@@ -36,7 +36,7 @@ One of the most important benefits of graph neural networks compared to other mo
<imgsrc="./docs/source/_static/message_passing_paradigm.png"alt="The basic idea of message passing paradigm"width="800">
As shown in the left of the following figure, to adapt general user-defined message aggregate functions, DGL uses the degree bucketing method to combine nodes with the same degree into a batch and then apply an aggregate function 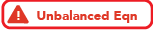 on each batch serially. For our PGL UDF aggregate function, we organize the message as a [LodTensor](http://www.paddlepaddle.org/documentation/docs/en/1.4/user_guides/howto/basic_concept/lod_tensor_en.html) in [PaddlePaddle](https://github.com/PaddlePaddle/Paddle) taking the message as variable length sequences. And we **utilize the features of LodTensor in Paddle to obtain fast parallel aggregation**.
As shown in the left of the following figure, to adapt general user-defined message aggregate functions, DGL uses the degree bucketing method to combine nodes with the same degree into a batch and then apply an aggregate function  on each batch serially. For our PGL UDF aggregate function, we organize the message as a [LodTensor](http://www.paddlepaddle.org/documentation/docs/en/1.4/user_guides/howto/basic_concept/lod_tensor_en.html) in [PaddlePaddle](https://github.com/PaddlePaddle/Paddle) taking the message as variable length sequences. And we **utilize the features of LodTensor in Paddle to obtain fast parallel aggregation**.
<imgsrc="./docs/source/_static/parallel_degree_bucketing.png"alt="The parallel degree bucketing of PGL"width="800">
@@ -6,54 +6,32 @@ information (e.g., text attributes) to efficiently generate node embeddings for
For purpose of high scalability, we use redis as distribute graph storage solution and training graphsage against redis server.
### Datasets(Quickstart)
The reddit dataset should be downloaded from [reddit_adj.npz](https://drive.google.com/open?id=174vb0Ws7Vxk_QTUtxqTgDHSQ4El4qDHt) and [reddit.npz](https://drive.google.com/open?id=19SphVl_Oe8SJ1r87Hr5a6znx3nJu1F2Jthe). The details for Reddit Dataset can be found [here](https://cs.stanford.edu/people/jure/pubs/graphsage-nips17.pdf).
The reddit dataset should be downloaded from [reddit_adj.npz](https://drive.google.com/open?id=174vb0Ws7Vxk_QTUtxqTgDHSQ4El4qDHt) and [reddit.npz](https://drive.google.com/open?id=19SphVl_Oe8SJ1r87Hr5a6znx3nJu1F2J). The details for Reddit Dataset can be found [here](https://cs.stanford.edu/people/jure/pubs/graphsage-nips17.pdf).
Alternatively, reddit dataset has been preprocessed and packed into docker image, which can be instantly pulled using following commands.