Merge branch 'android_demo' of https://github.com/WenmuZhou/PaddleOCR into android_demo
Showing
doc/doc_ch/multi_languages.md
0 → 100644
doc/doc_en/multi_languages_en.md
0 → 100644
doc/doc_en/pgnet_en.md
0 → 100644
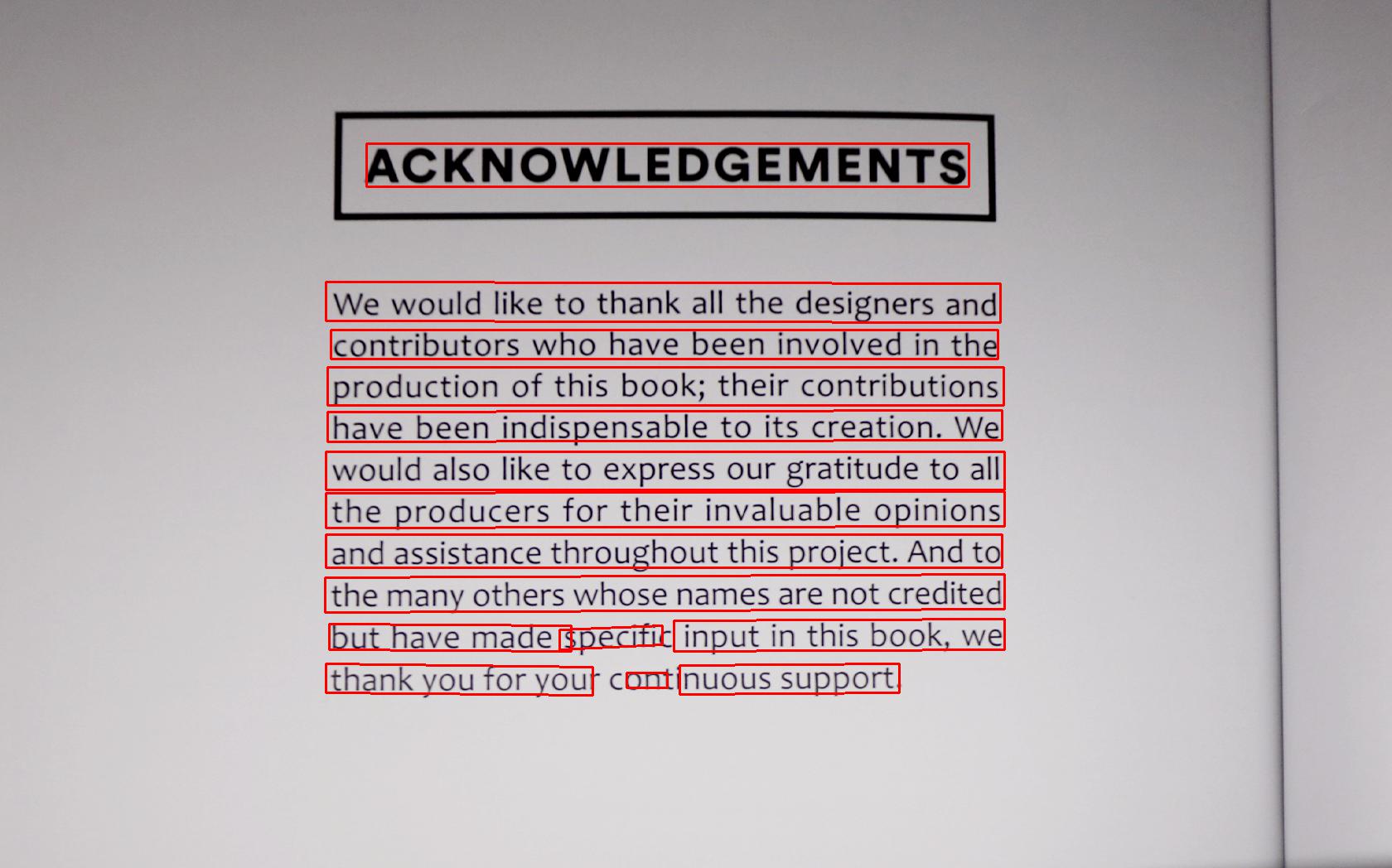
| W: | H:
| W: | H:
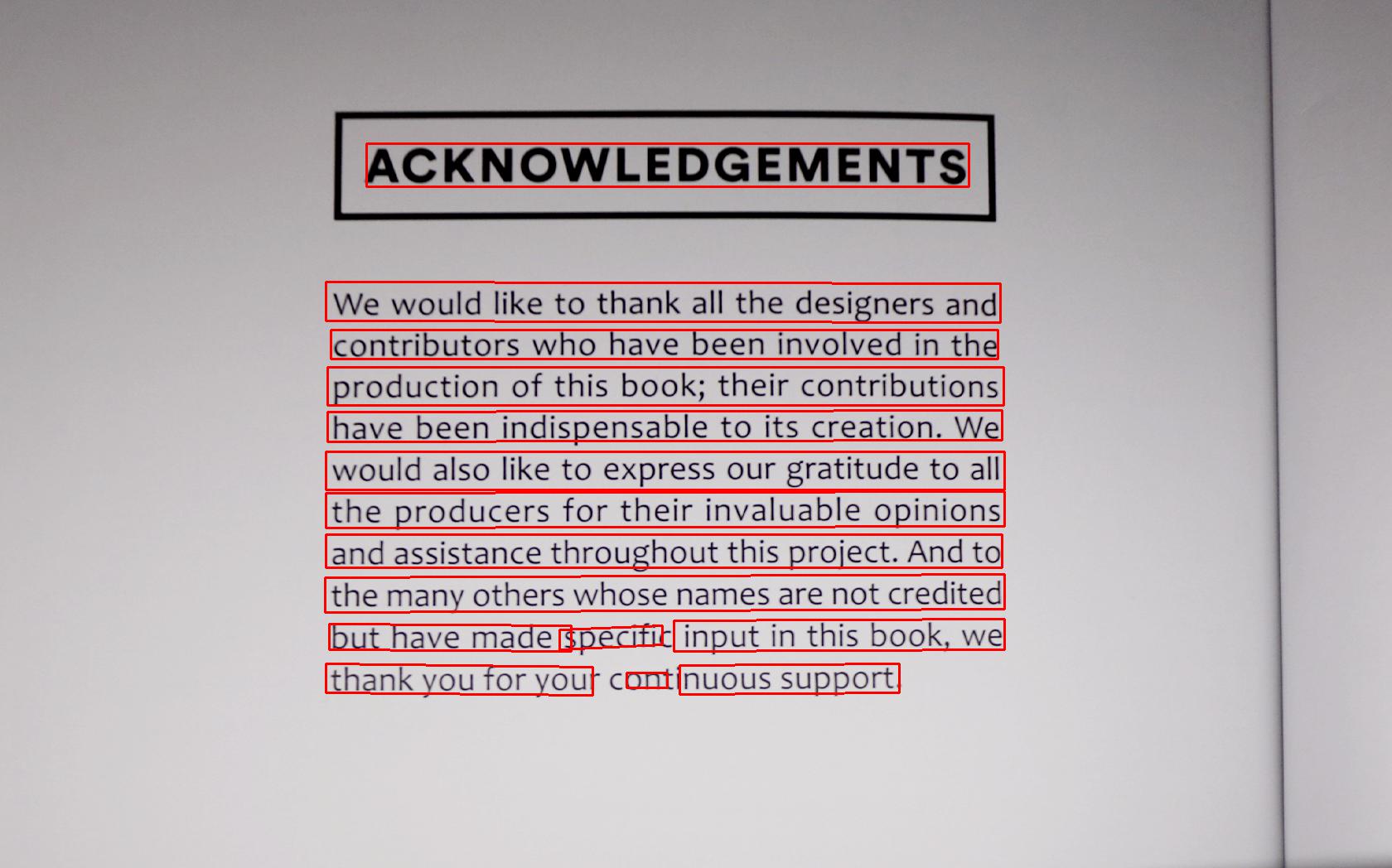
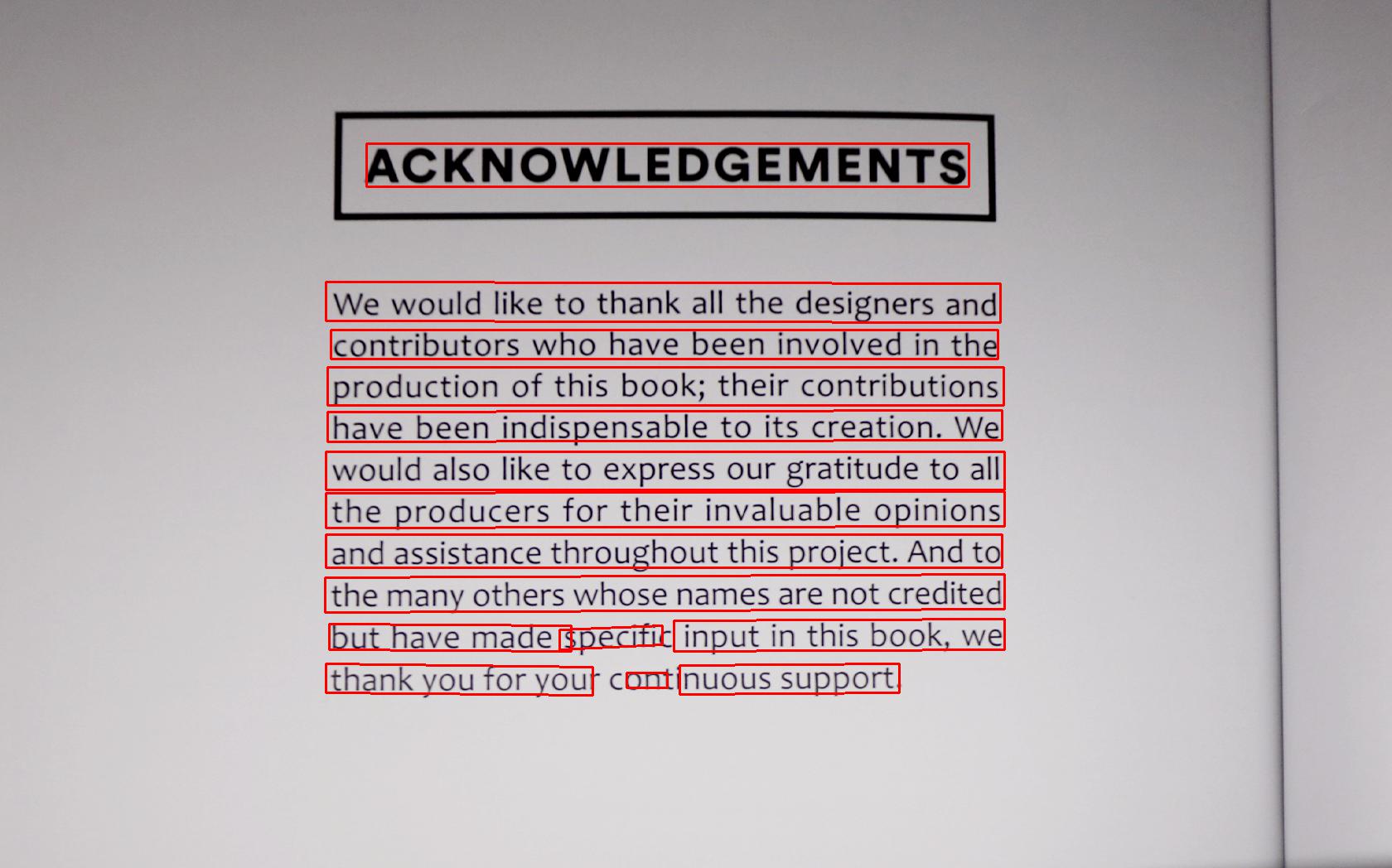
ppocr/utils/dict/arabic_dict.txt
0 → 100644
ppocr/utils/dict/latin_dict.txt
0 → 100644