Merge branch 'dygraph' of https://github.com/PaddlePaddle/PaddleOCR into lock_seed
Showing
4.1 KB
4.1 KB
configs/rec/rec_mtb_nrtr.yml
0 → 100644
deploy/cpp_infer/tools/run.sh
已删除
100755 → 0
| W: | H:
| W: | H:
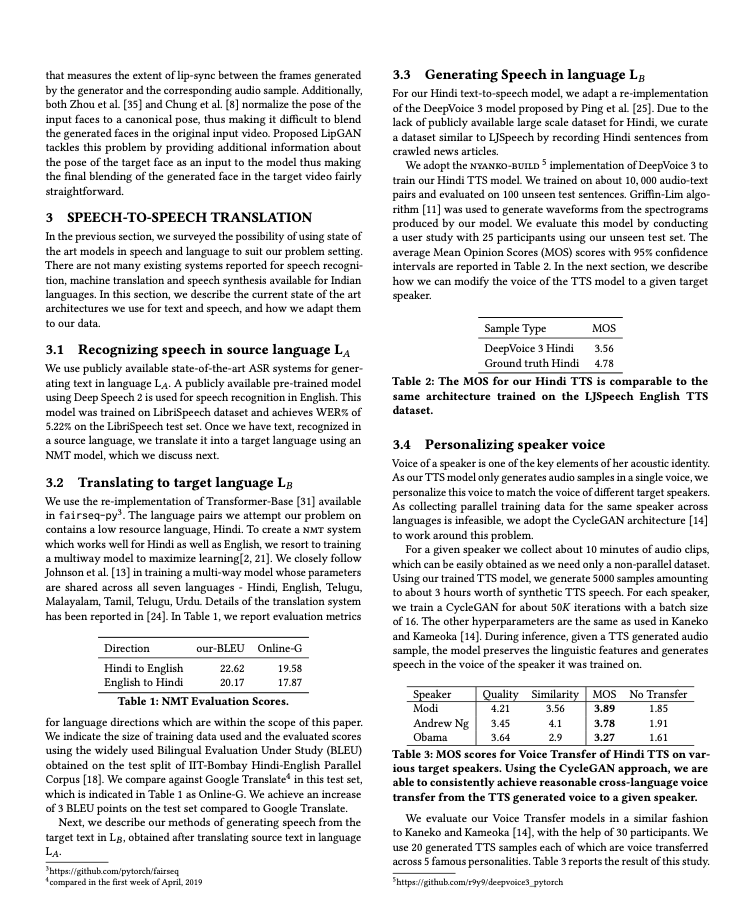
| W: | H:
| W: | H:
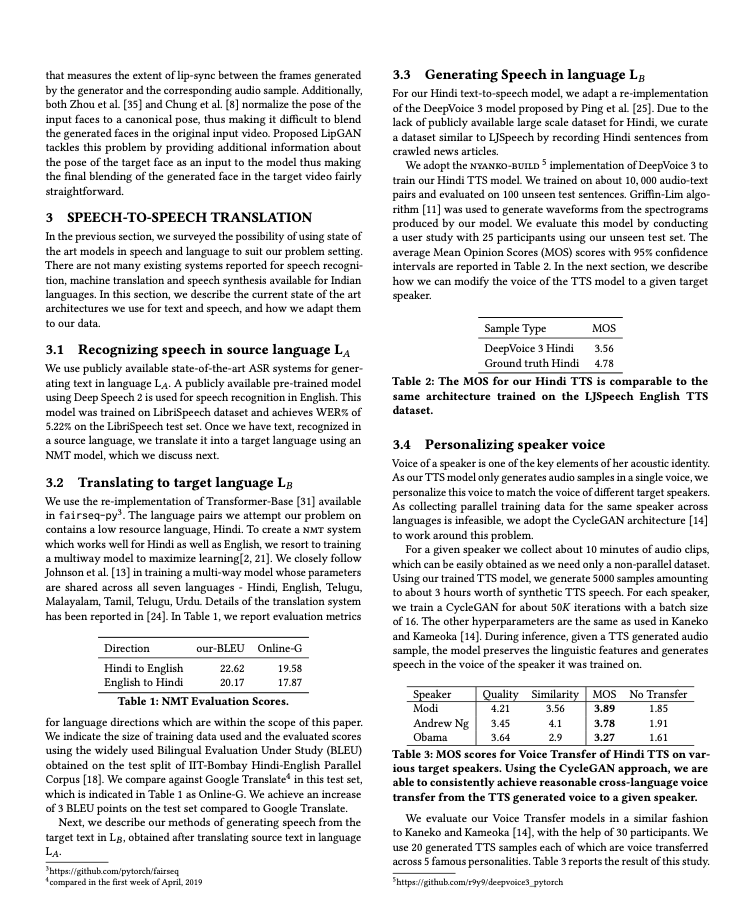
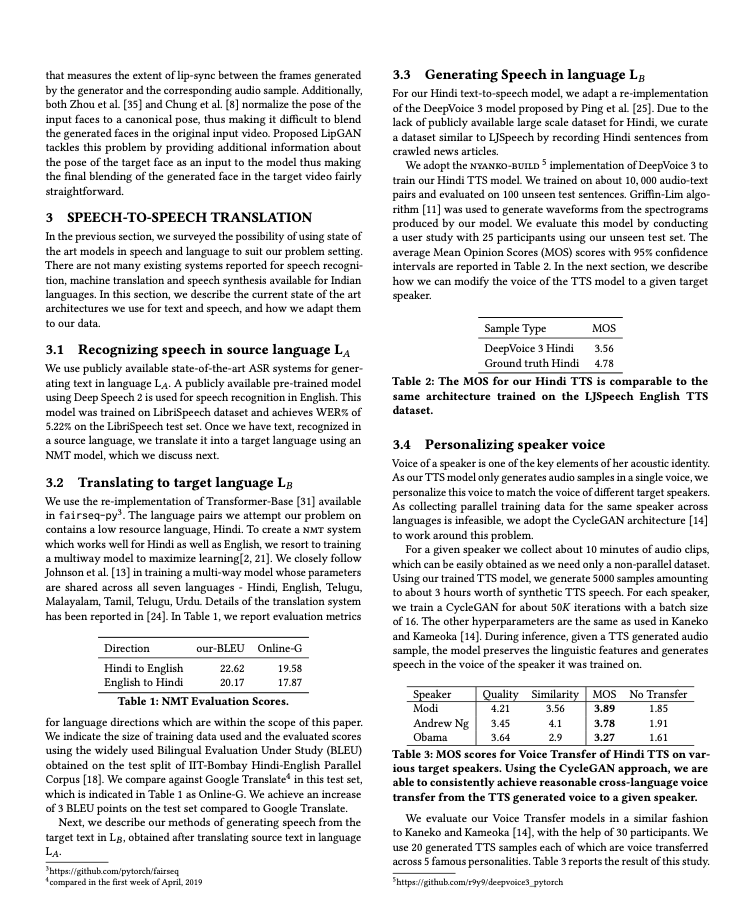
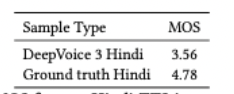
| W: | H:
| W: | H:
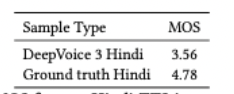
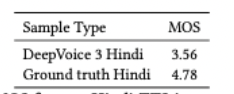
ppocr/losses/rec_nrtr_loss.py
0 → 100644
此差异已折叠。
... | ... | @@ -7,4 +7,7 @@ tqdm |
numpy | ||
visualdl | ||
python-Levenshtein | ||
opencv-contrib-python==4.4.0.46 | ||
\ No newline at end of file | ||
opencv-contrib-python==4.4.0.46 | ||
lxml | ||
premailer | ||
openpyxl | ||
\ No newline at end of file |
tests/ocr_det_server_params.txt
0 → 100644
tests/readme.md
0 → 100644