Merge branch 'develop' of https://github.com/PaddlePaddle/Paddle into recv_op_python_with_guard
Showing
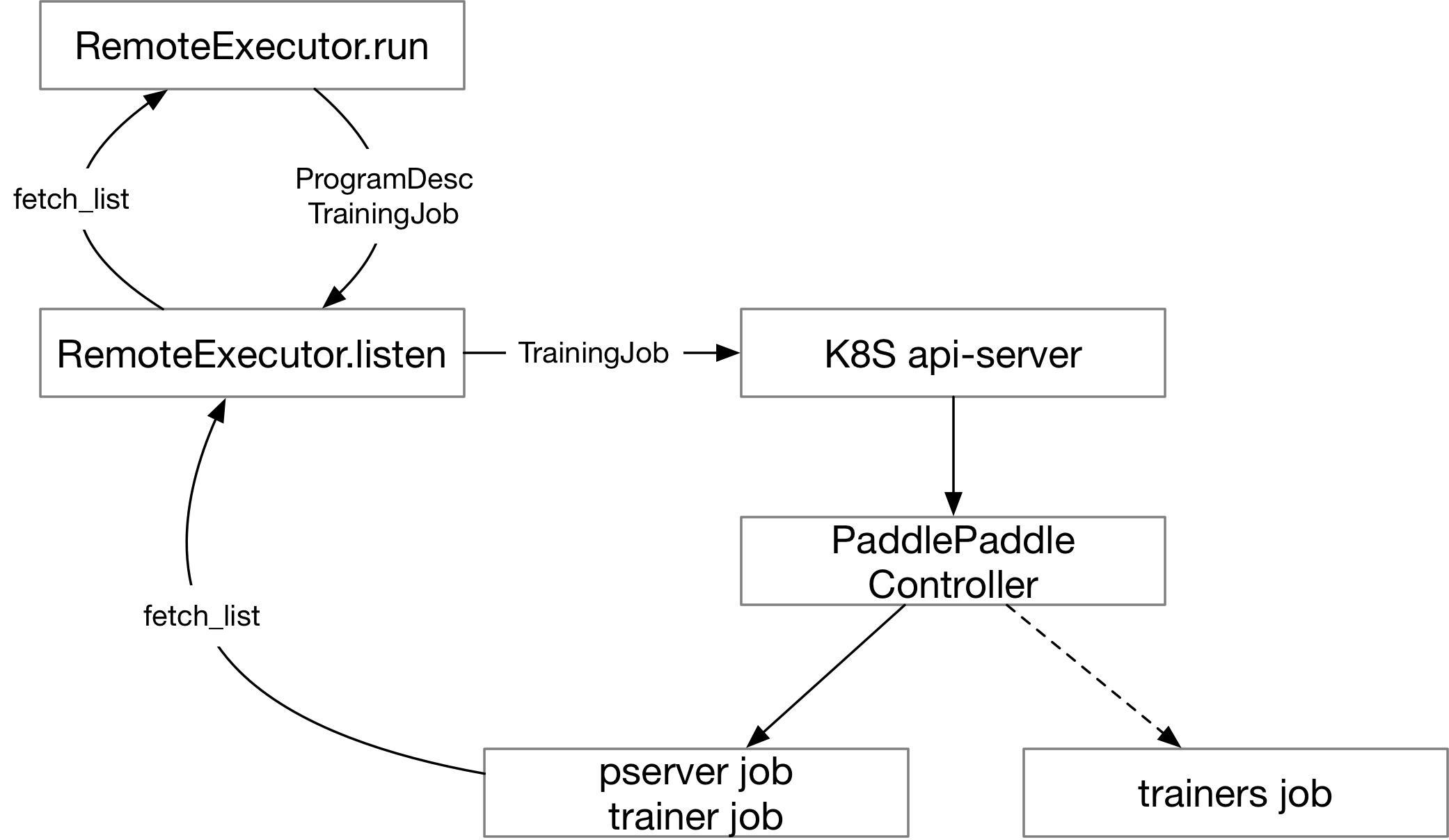
| W: | H:
| W: | H:
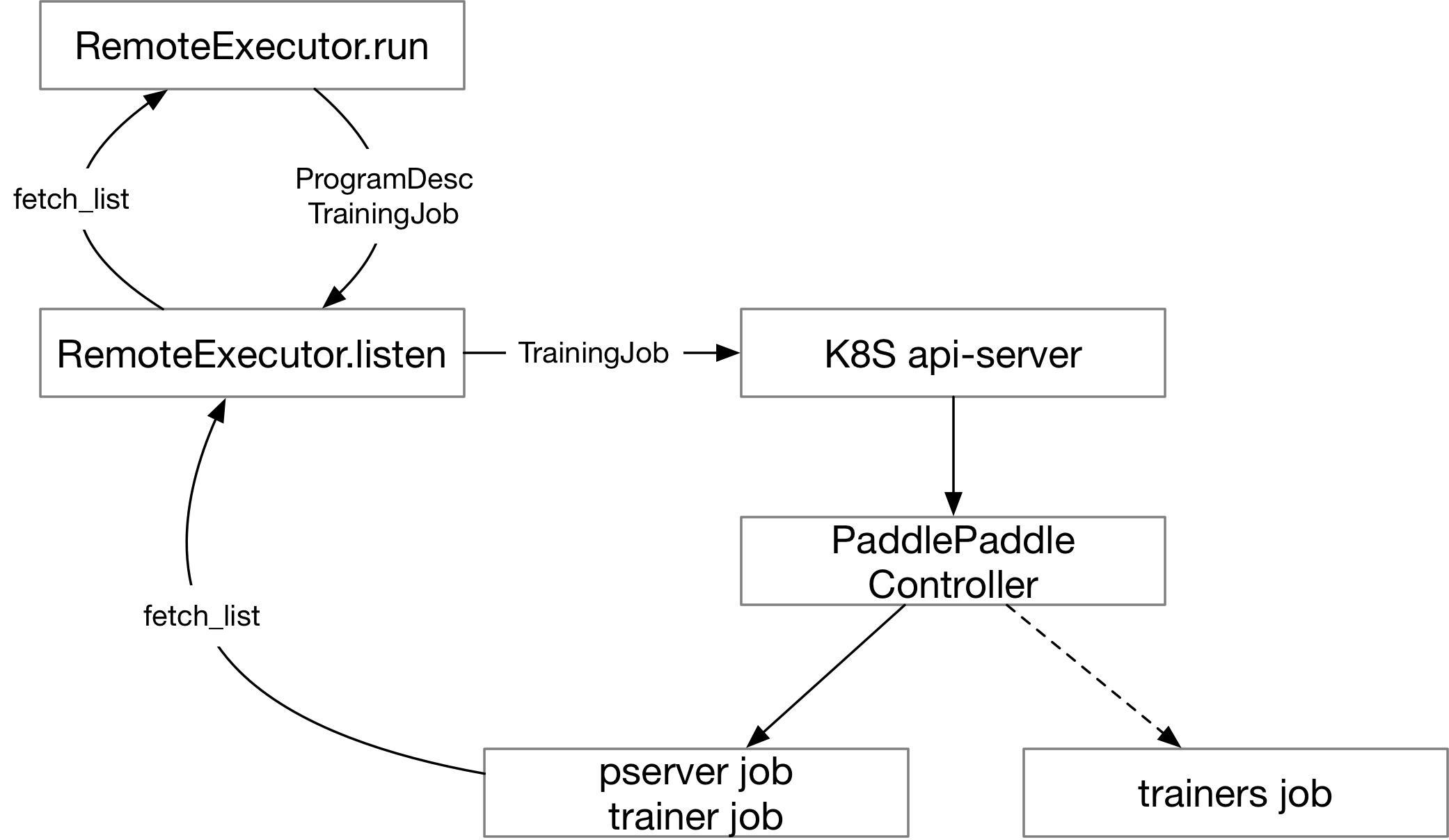
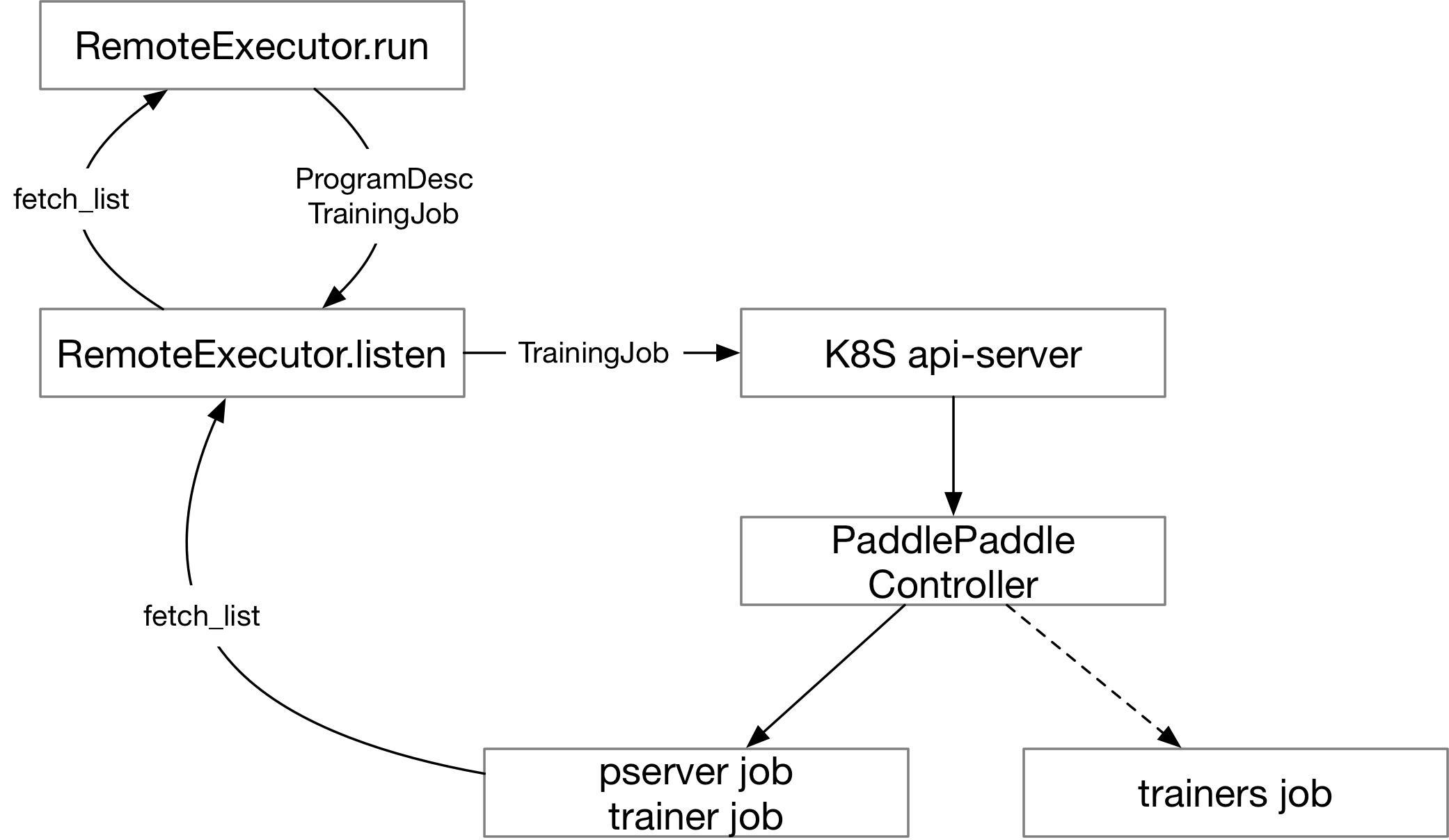
paddle/framework/channel.h
0 → 100644
paddle/operators/lstmp_op.cc
0 → 100644
paddle/operators/lstmp_op.cu
0 → 100644
paddle/operators/lstmp_op.h
0 → 100644
此差异已折叠。
paddle/operators/one_hot_op.cc
0 → 100644
paddle/operators/one_hot_op.cu
0 → 100644
paddle/operators/one_hot_op.h
0 → 100644
文件模式从 100755 更改为 100644
此差异已折叠。
此差异已折叠。
此差异已折叠。