Merge remote-tracking branch 'upstream/develop' into general-server-py3
Showing
doc/RUN_IN_DOCKER.md
0 → 100644
doc/RUN_IN_DOCKER_CN.md
0 → 100644
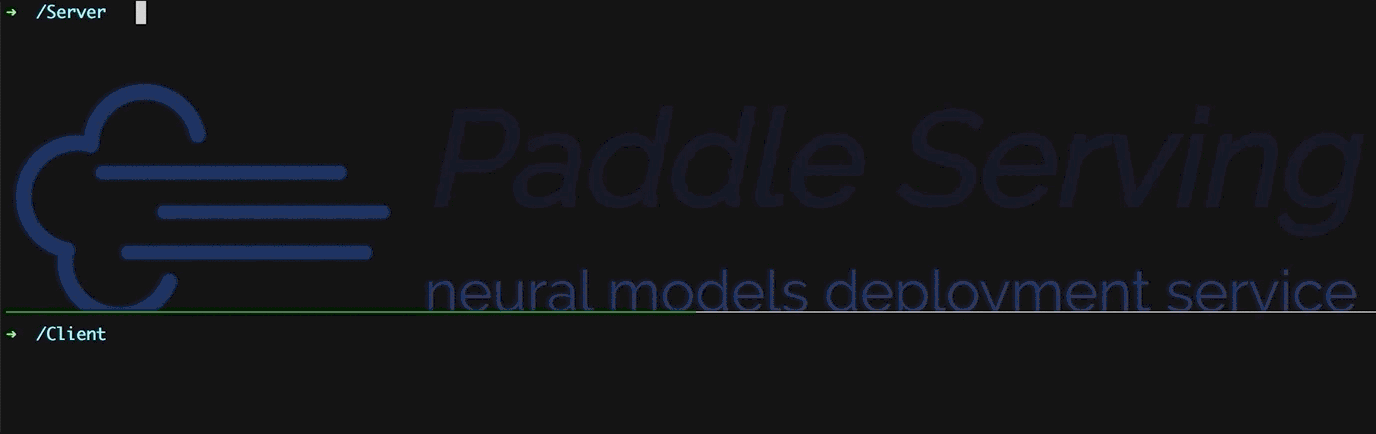
| W: | H:
| W: | H:
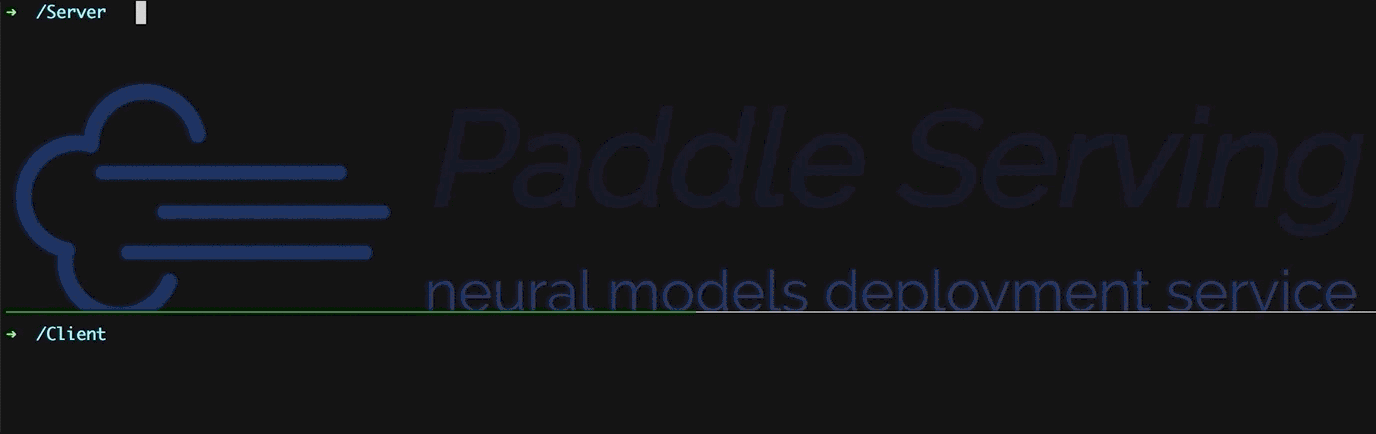
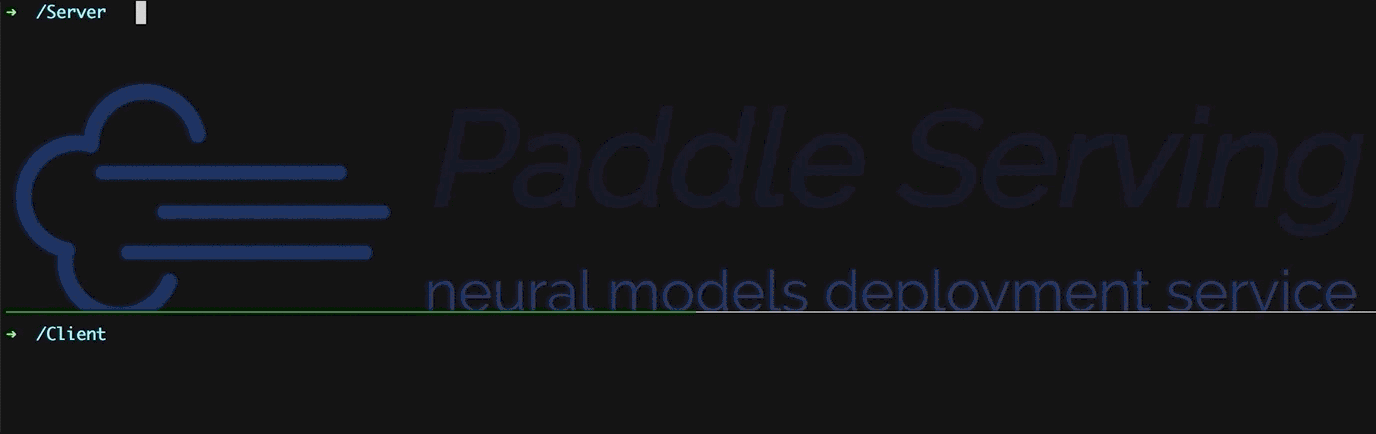
python/examples/lac/get_data.sh
0 → 100644
python/examples/lac/lac_client.py
0 → 100644
python/examples/lac/lac_reader.py
0 → 100644
python/examples/lac/utils.py
0 → 100644
tools/Dockerfile.devel
0 → 100644