Merge branch 'develop' into develop
Showing
deploy/paddleserving/README.MD
0 → 120000
docs/en_tmp/faq_series/.gitkeep
0 → 100644
docs/en_tmp/images/.gitkeep
0 → 100644
docs/en_tmp/installation/.gitkeep
0 → 100644
docs/en_tmp/introduction/.gitkeep
0 → 100644
docs/en_tmp/models/.gitkeep
0 → 100644
docs/en_tmp/others/.gitkeep
0 → 100644
docs/en_tmp/quick_start/.gitkeep
0 → 100644
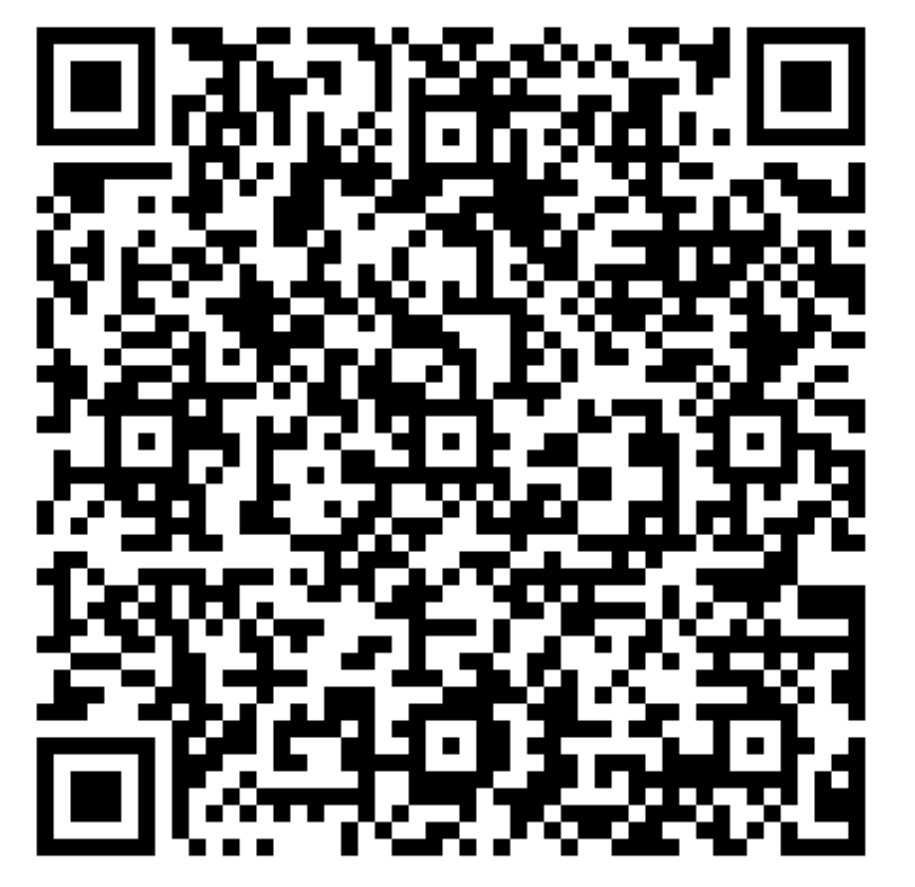
| W: | H:
| W: | H:
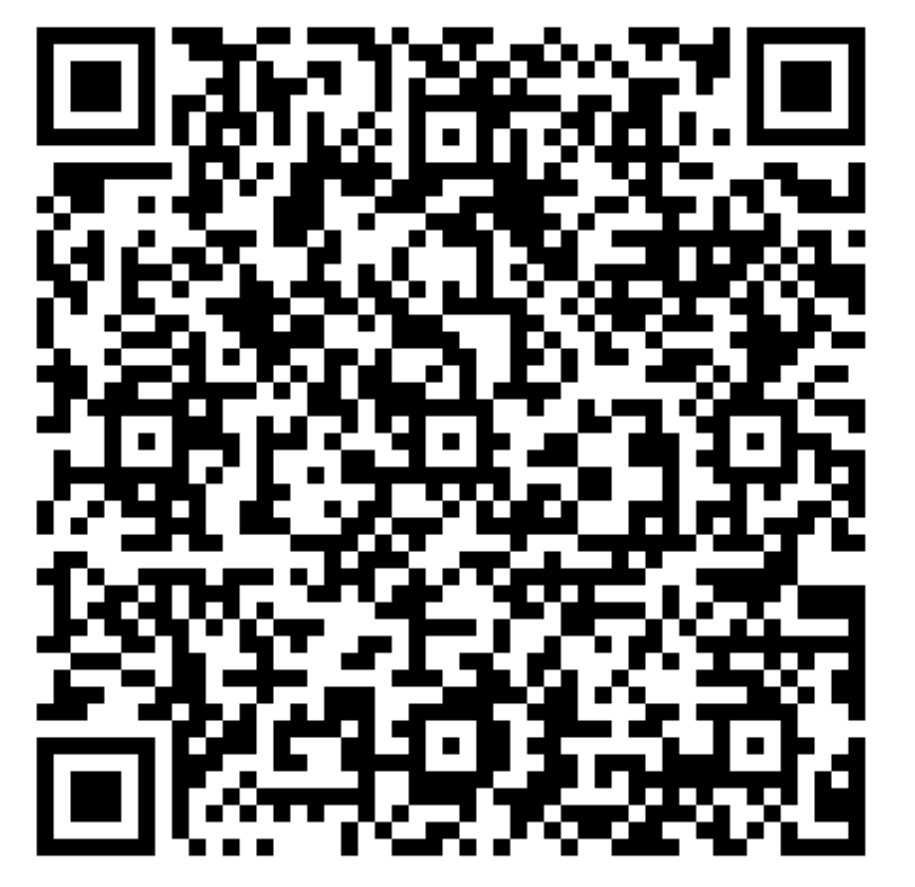
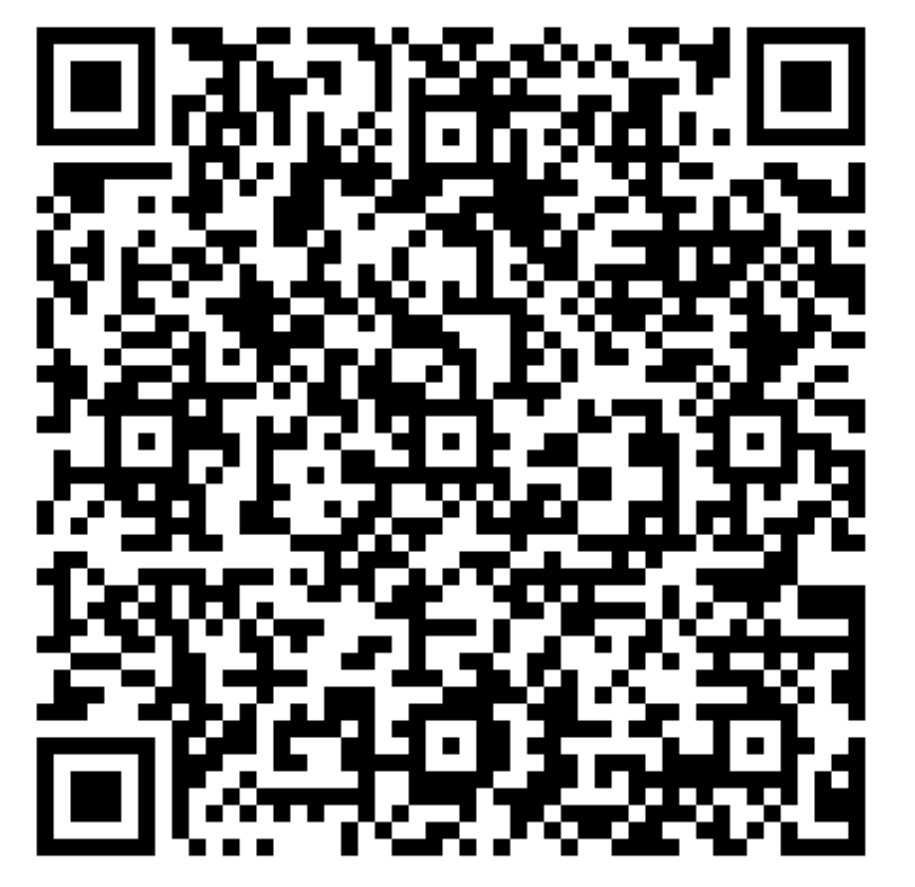