Merge branch 'develop' of https://github.com/PaddlePaddle/Paddle into pixel_softmax_layer
Showing
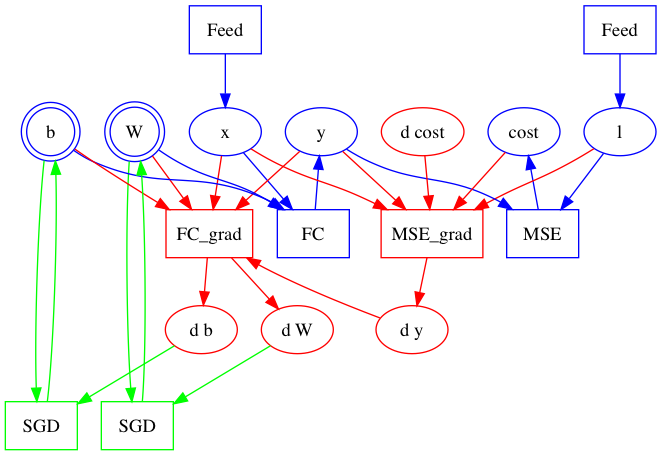
| W: | H:
| W: | H:
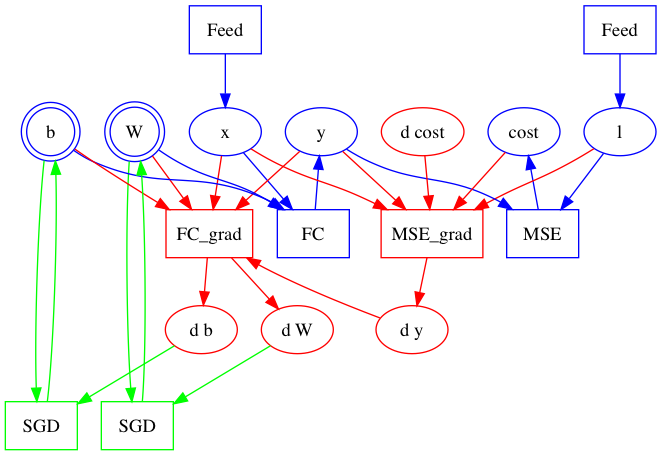
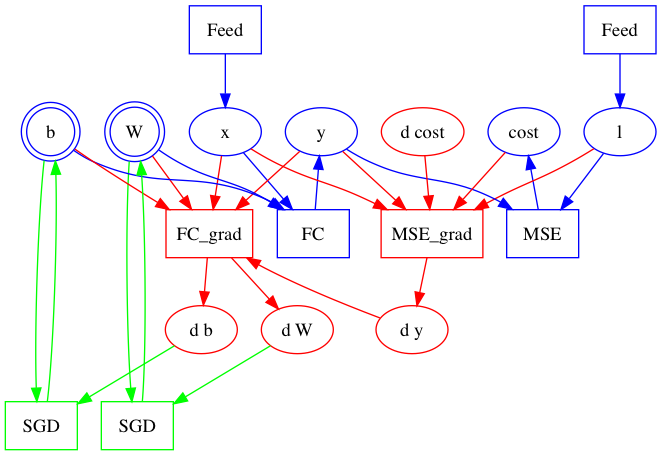
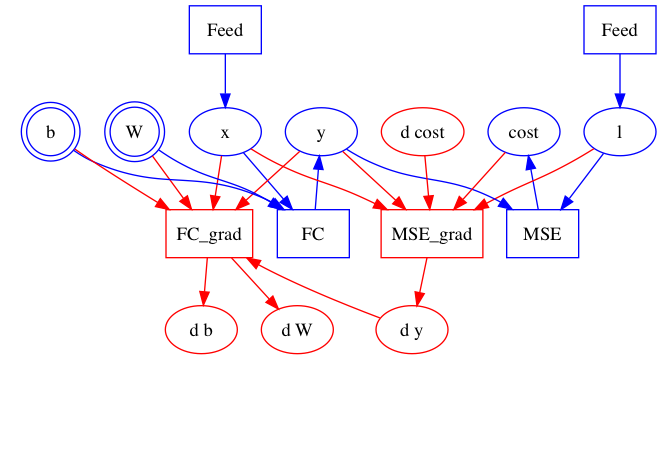
| W: | H:
| W: | H:
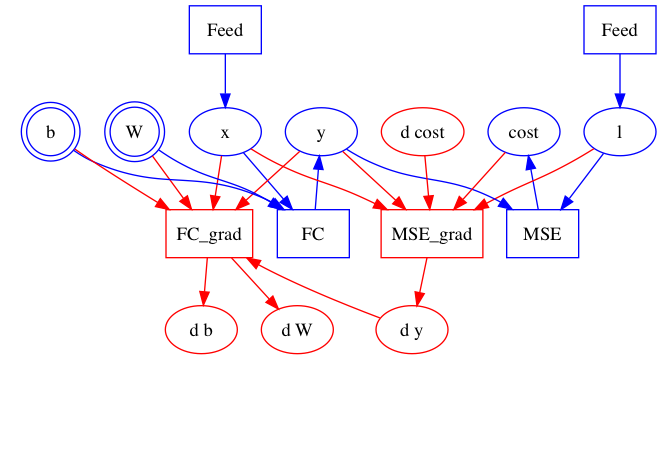
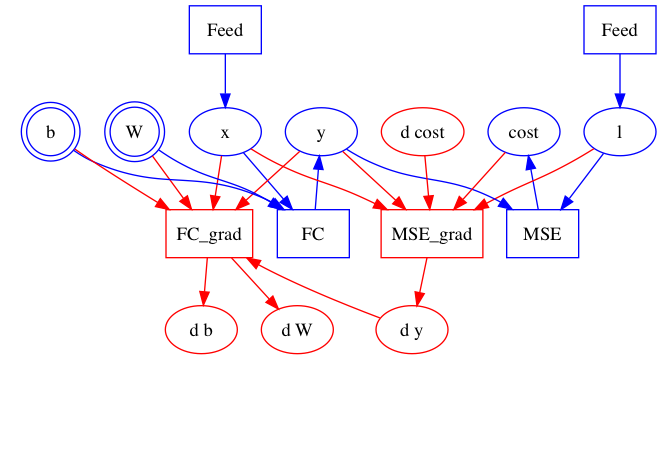
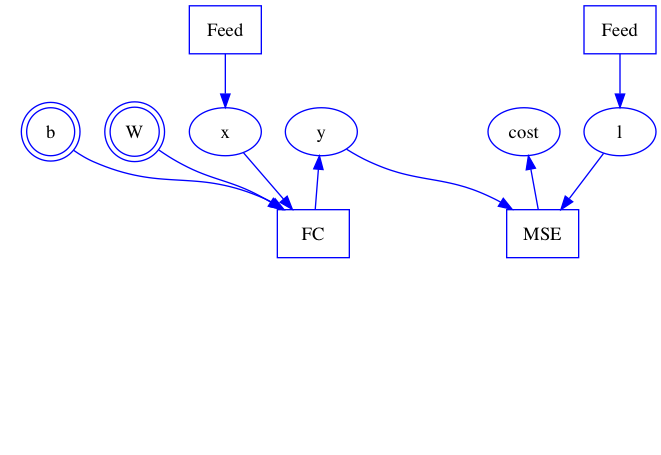
| W: | H:
| W: | H:
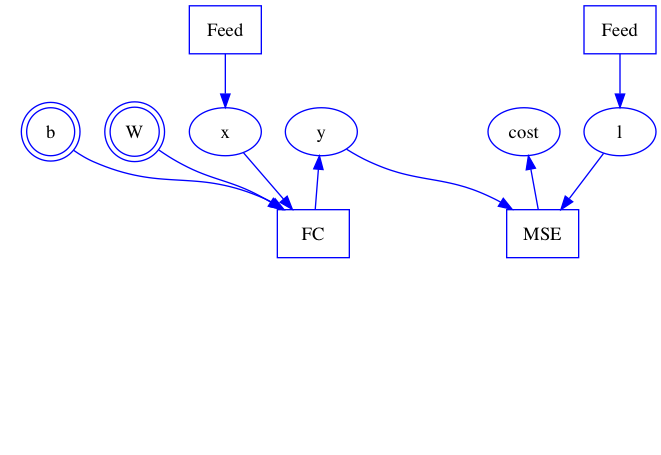
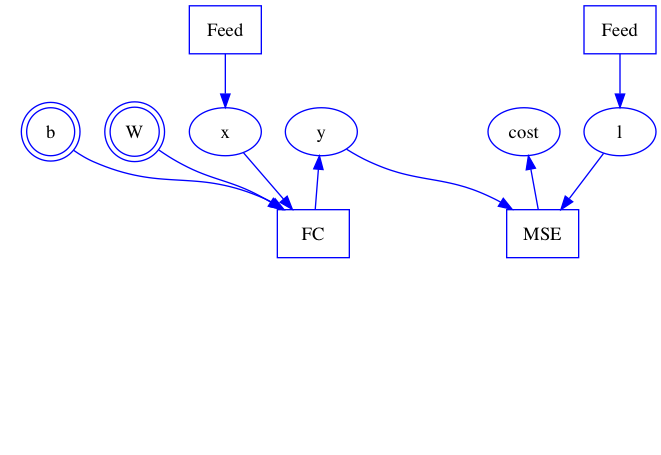
doc/howto/dev/use_eigen_cn.md
0 → 100644
paddle/operators/cos_sim_op.cc
0 → 100644
paddle/operators/cos_sim_op.h
0 → 100644
paddle/operators/identity_op.cc
0 → 100644