refine demo dataprovider and some tiny fix
ISSUE=4597359 git-svn-id: https://svn.baidu.com/idl/trunk/paddle@1432 1ad973e4-5ce8-4261-8a94-b56d1f490c56
Showing
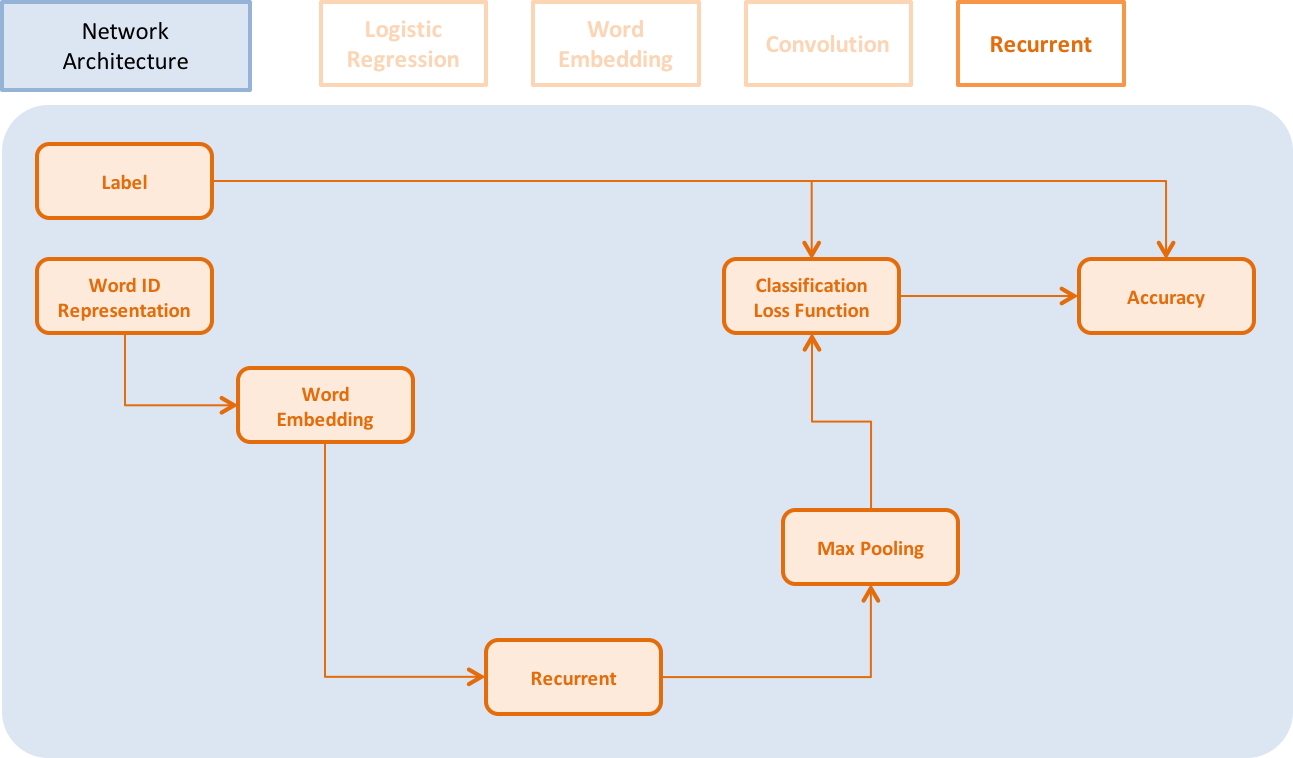
| W: | H:
| W: | H:
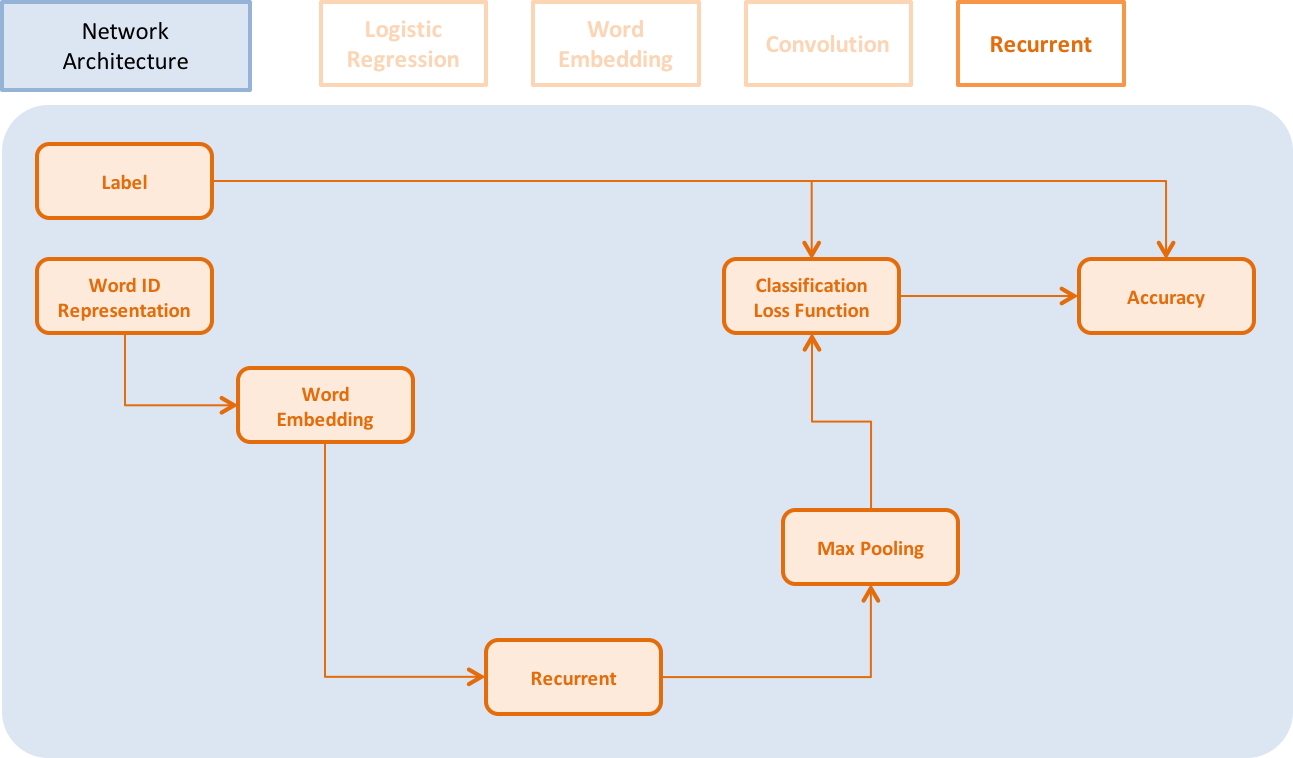
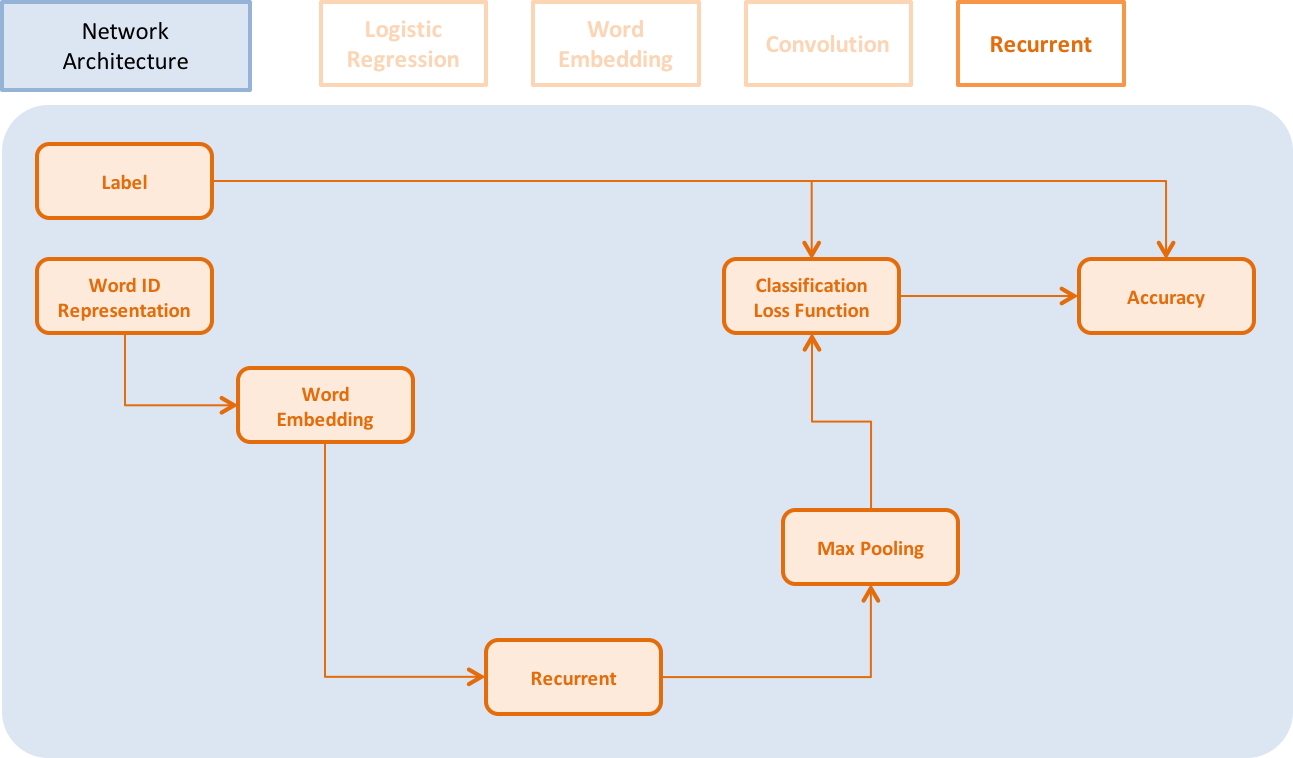
doc/layer.md
0 → 100644
ISSUE=4597359 git-svn-id: https://svn.baidu.com/idl/trunk/paddle@1432 1ad973e4-5ce8-4261-8a94-b56d1f490c56
70.3 KB | W: | H:
57.1 KB | W: | H: