翻译完成
Showing
dynamic_programming/编辑距离.md
已删除
100644 → 0
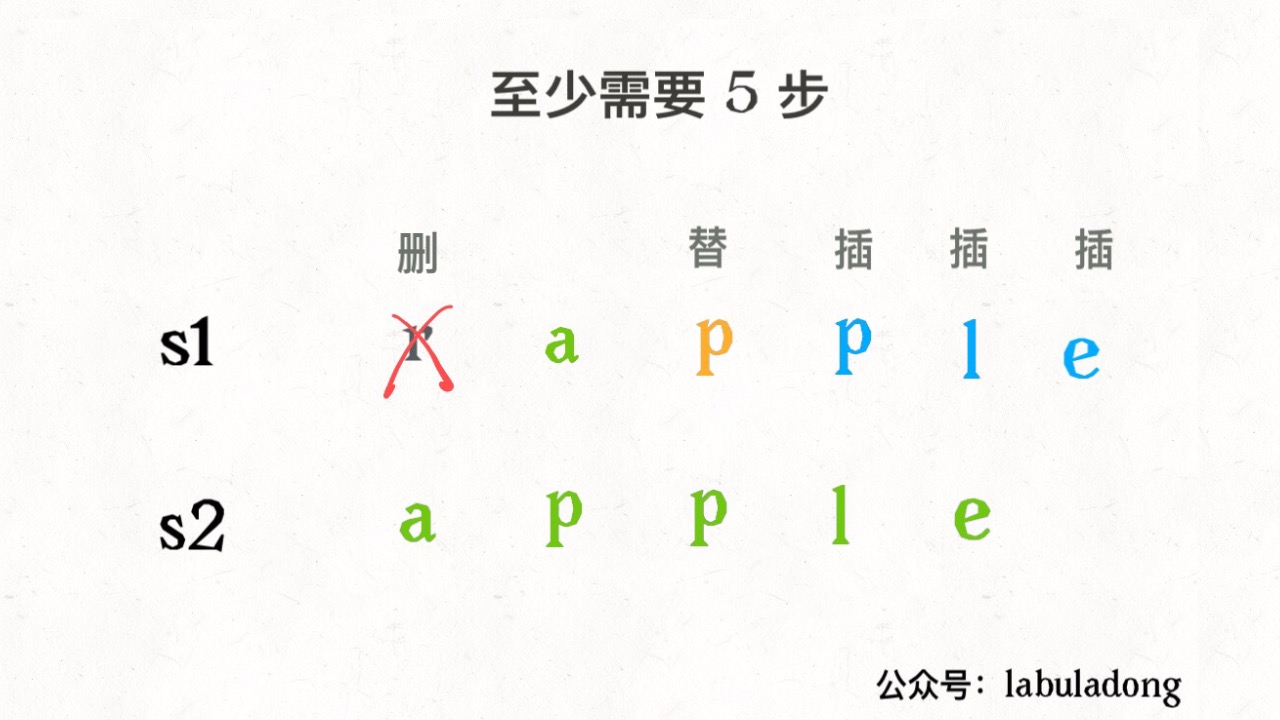
| W: | H:
| W: | H:
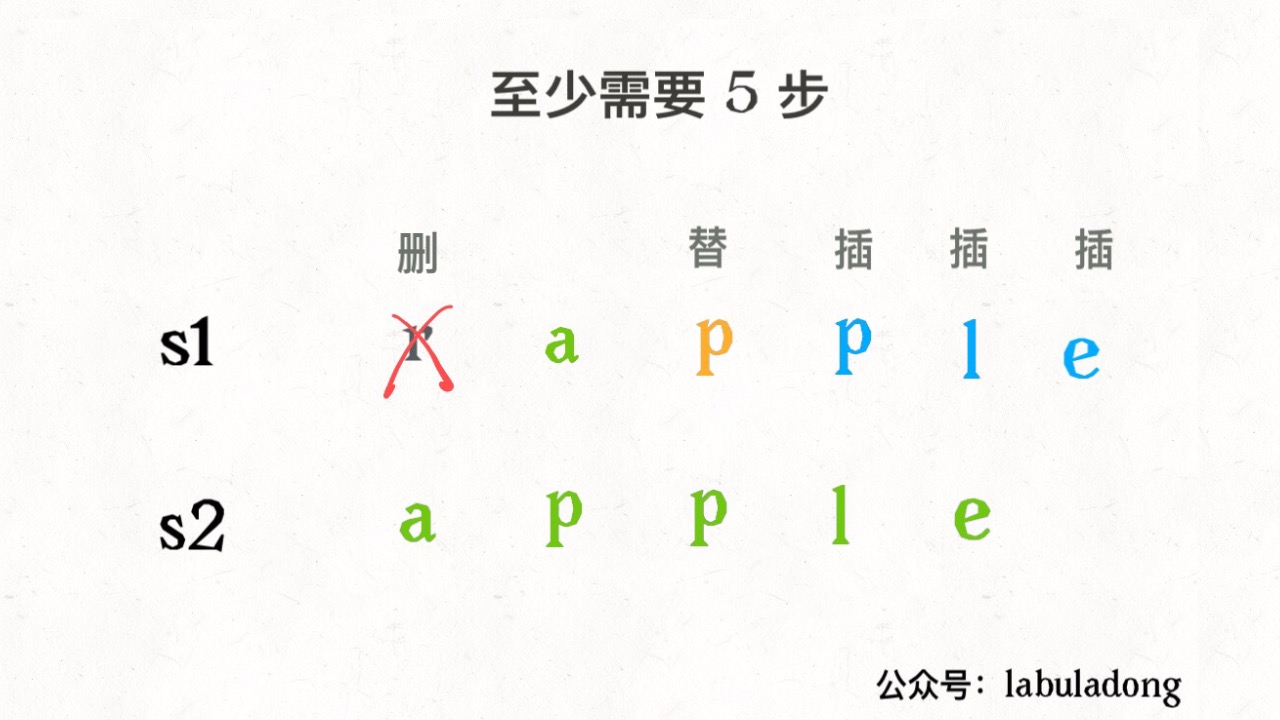
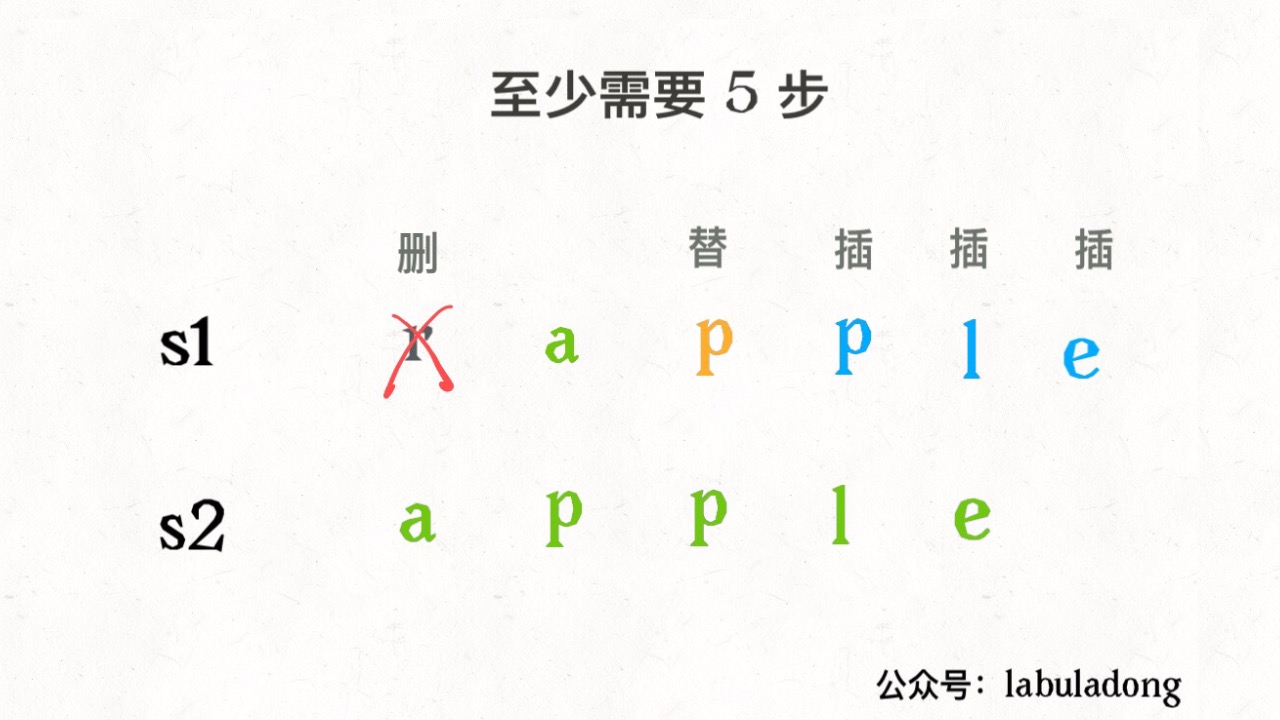
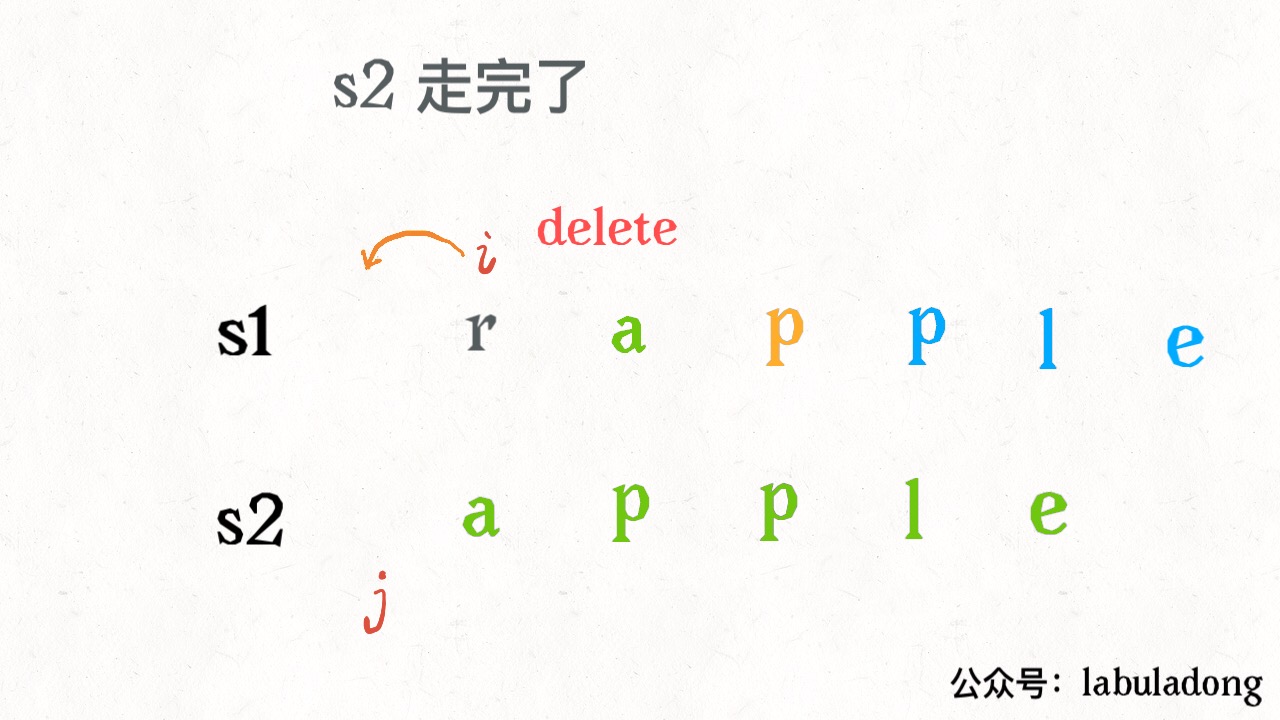
| W: | H:
| W: | H:
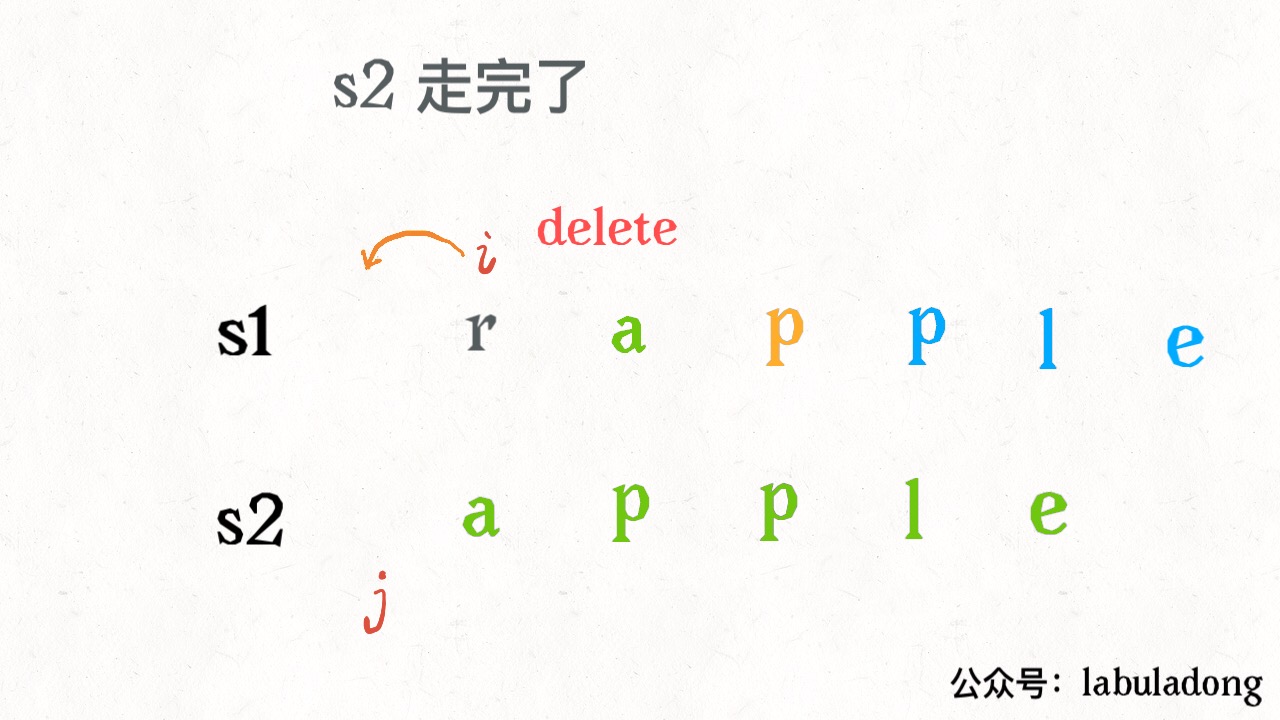
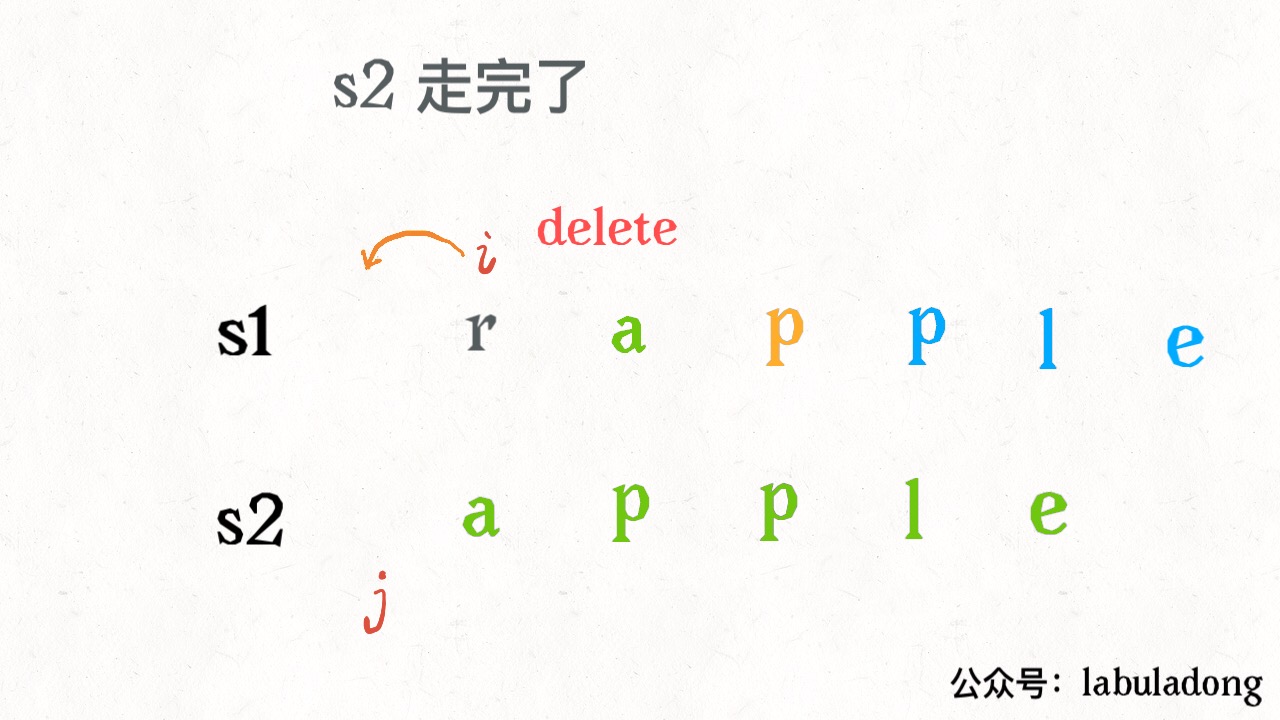
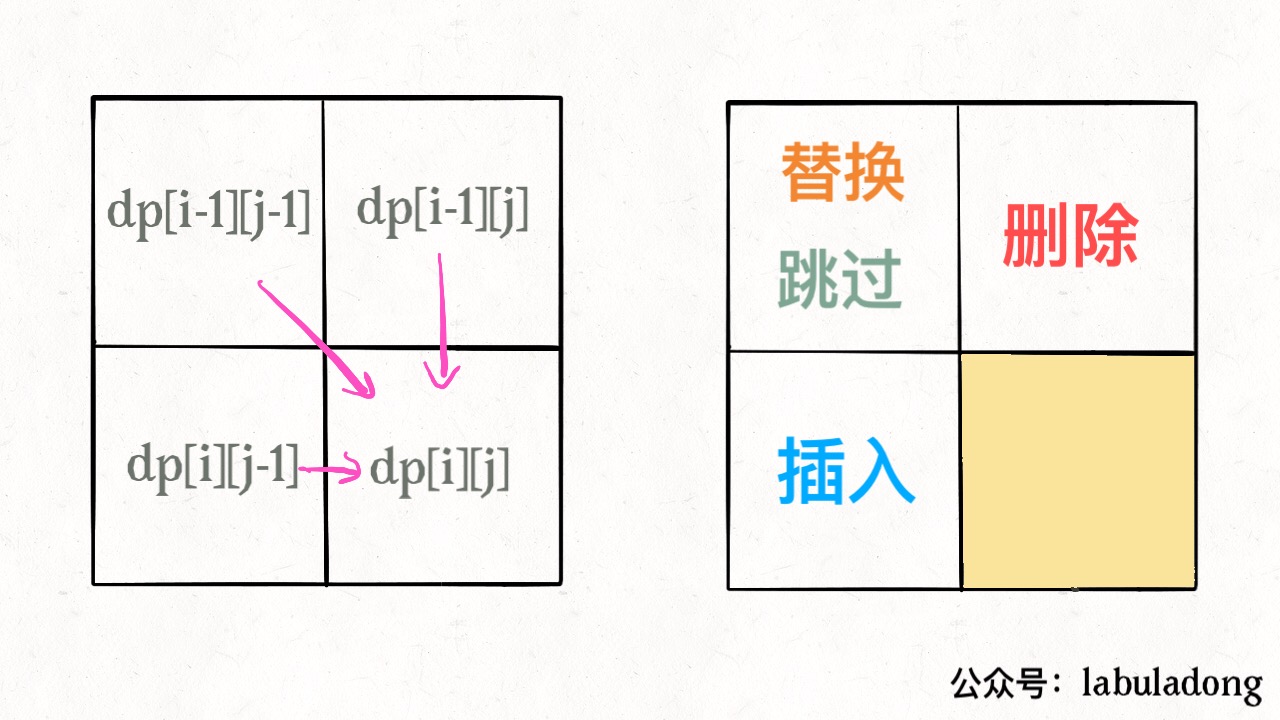
| W: | H:
| W: | H:
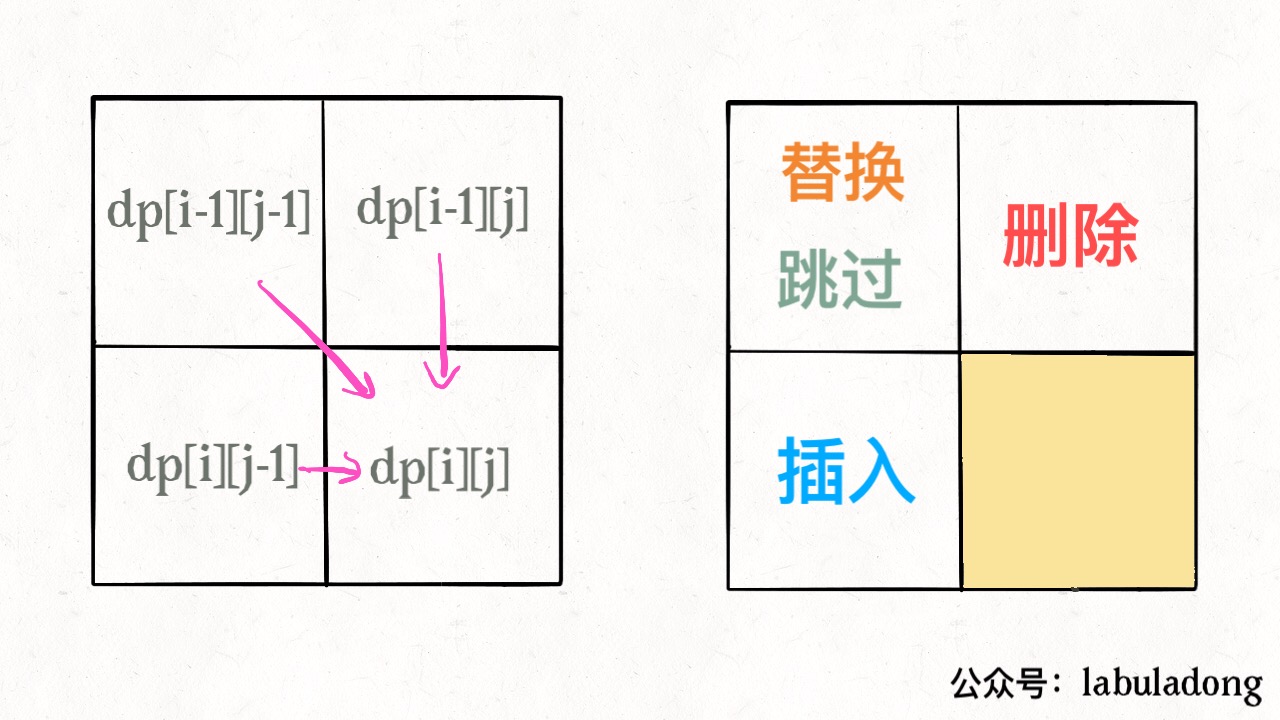
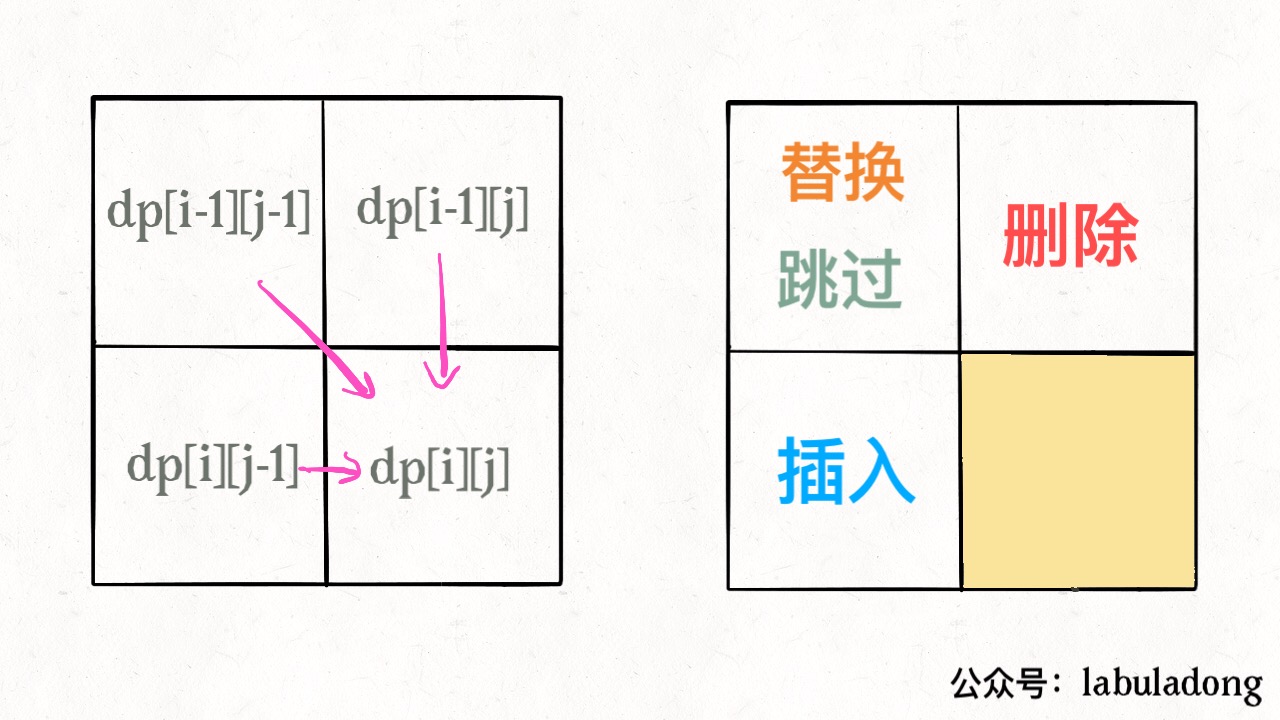
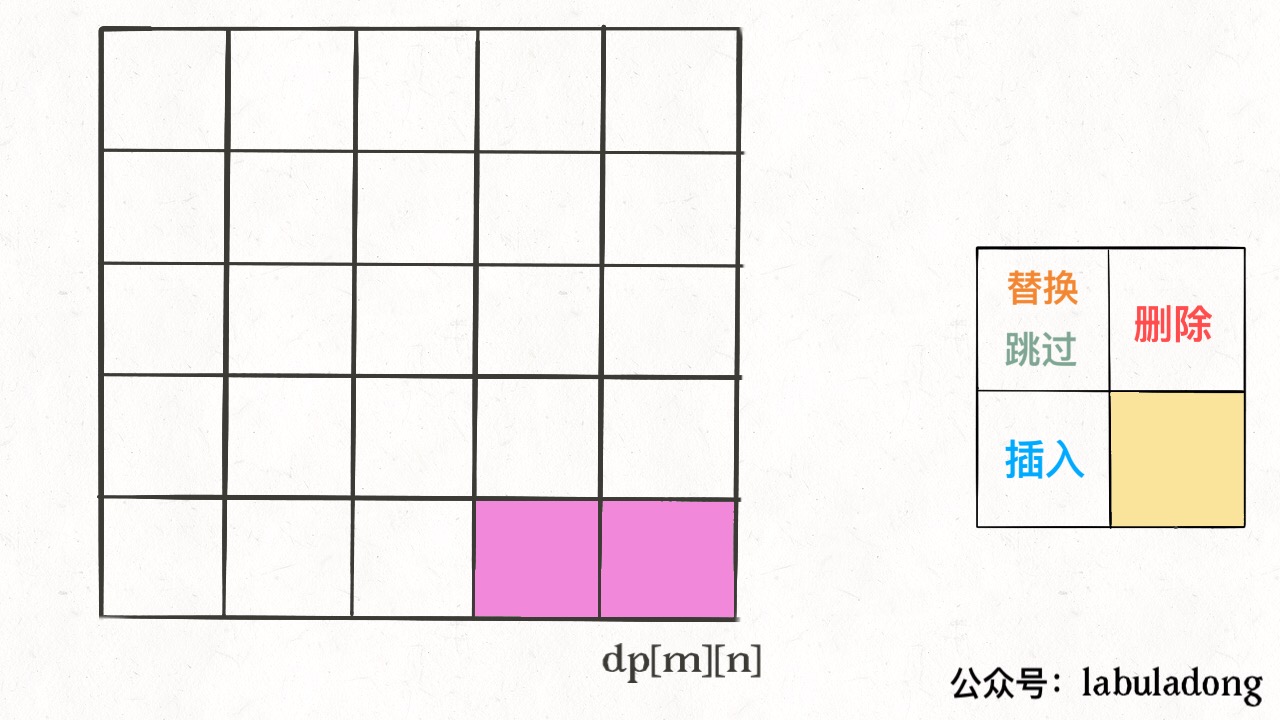
| W: | H:
| W: | H:
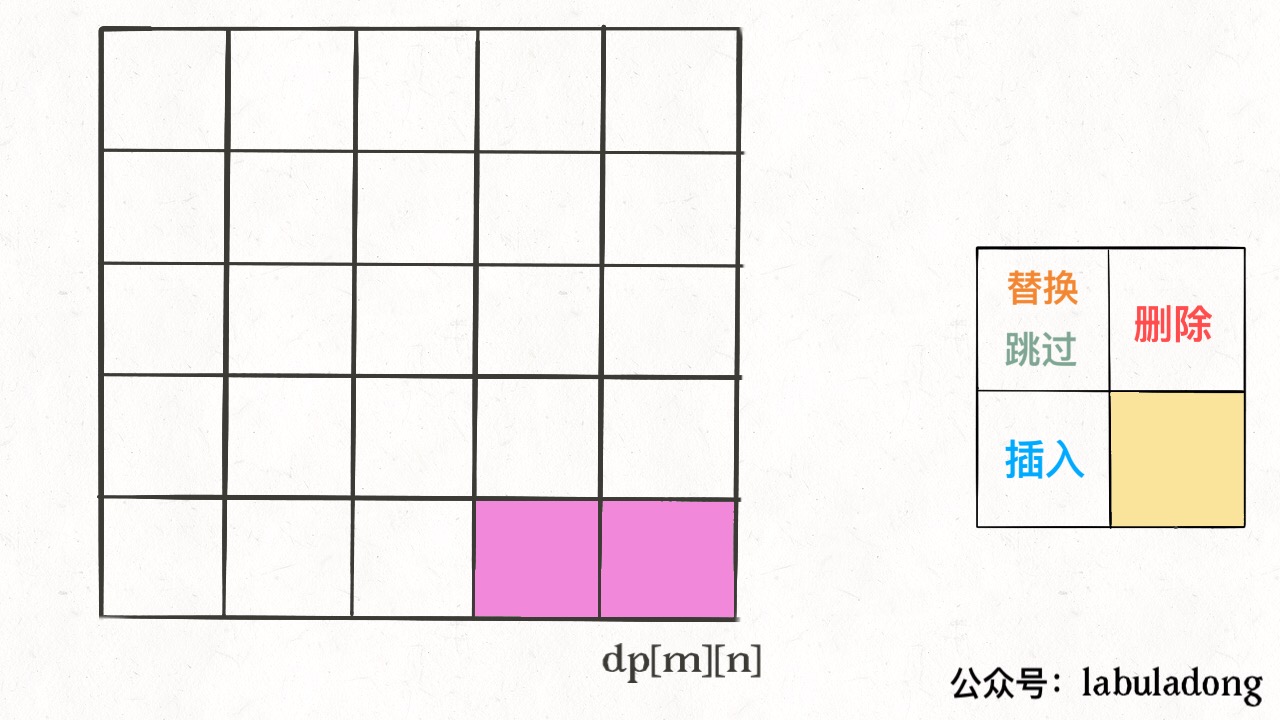
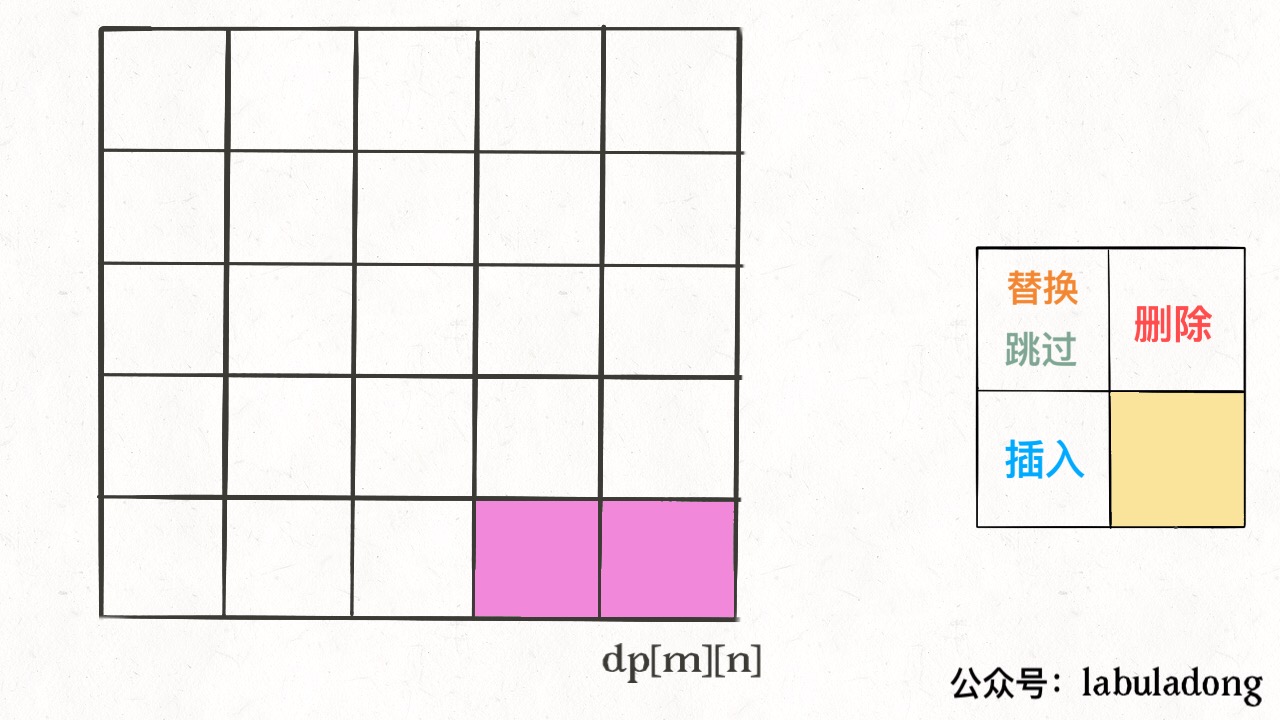
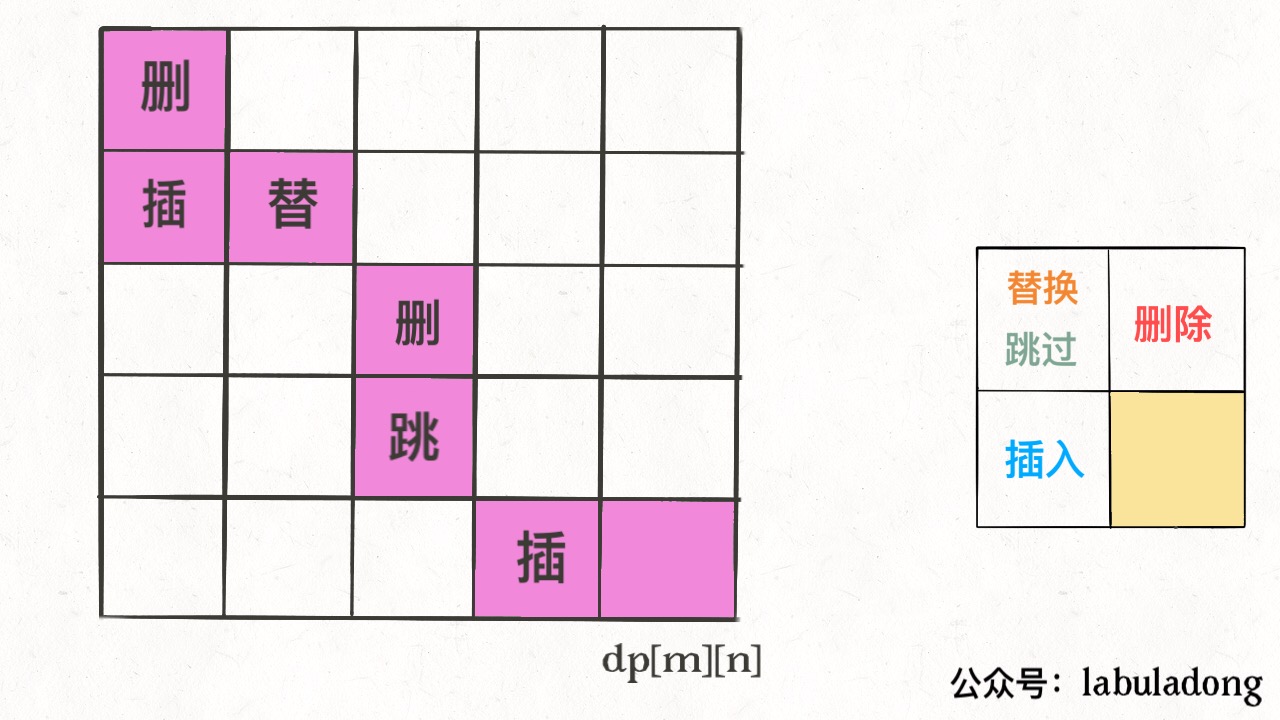
| W: | H:
| W: | H:
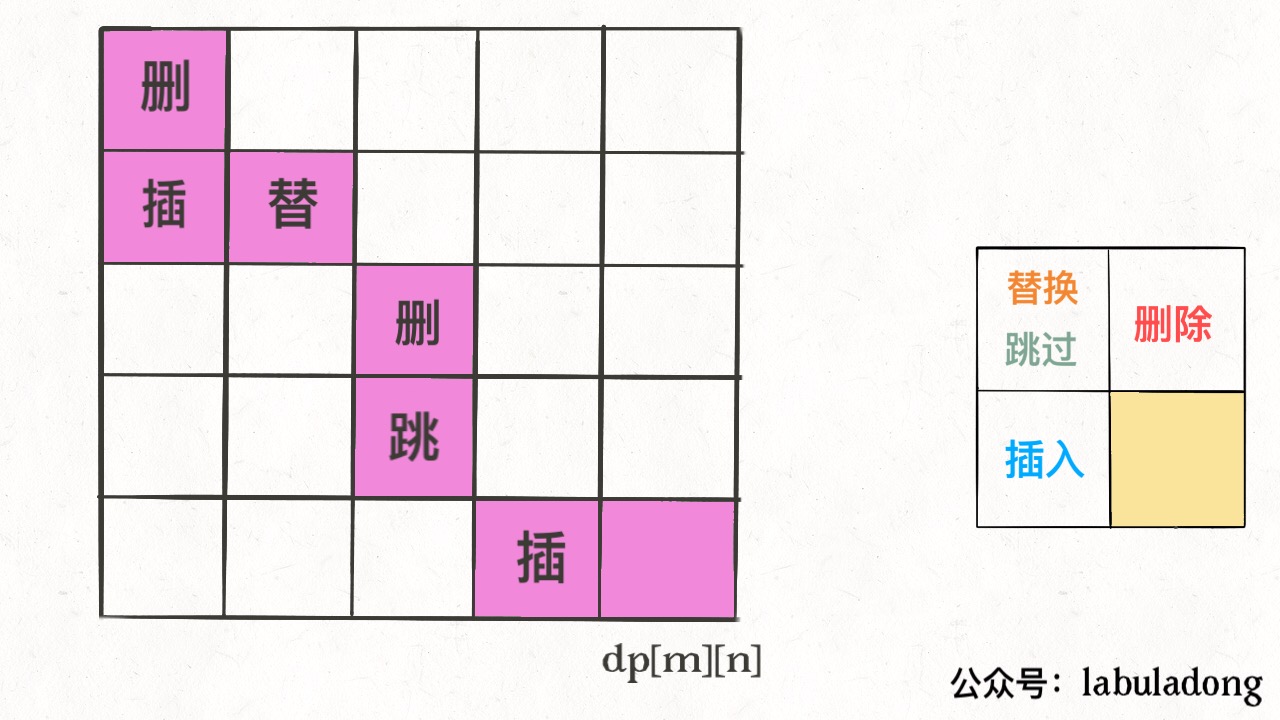
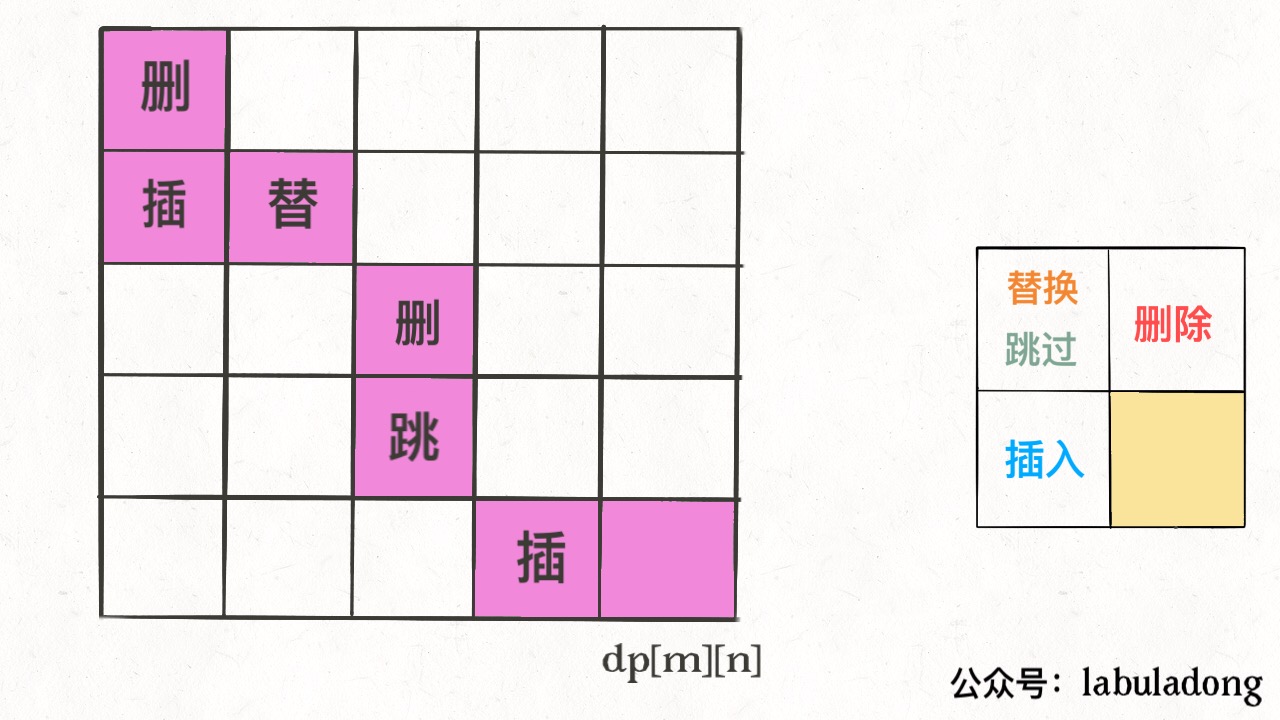
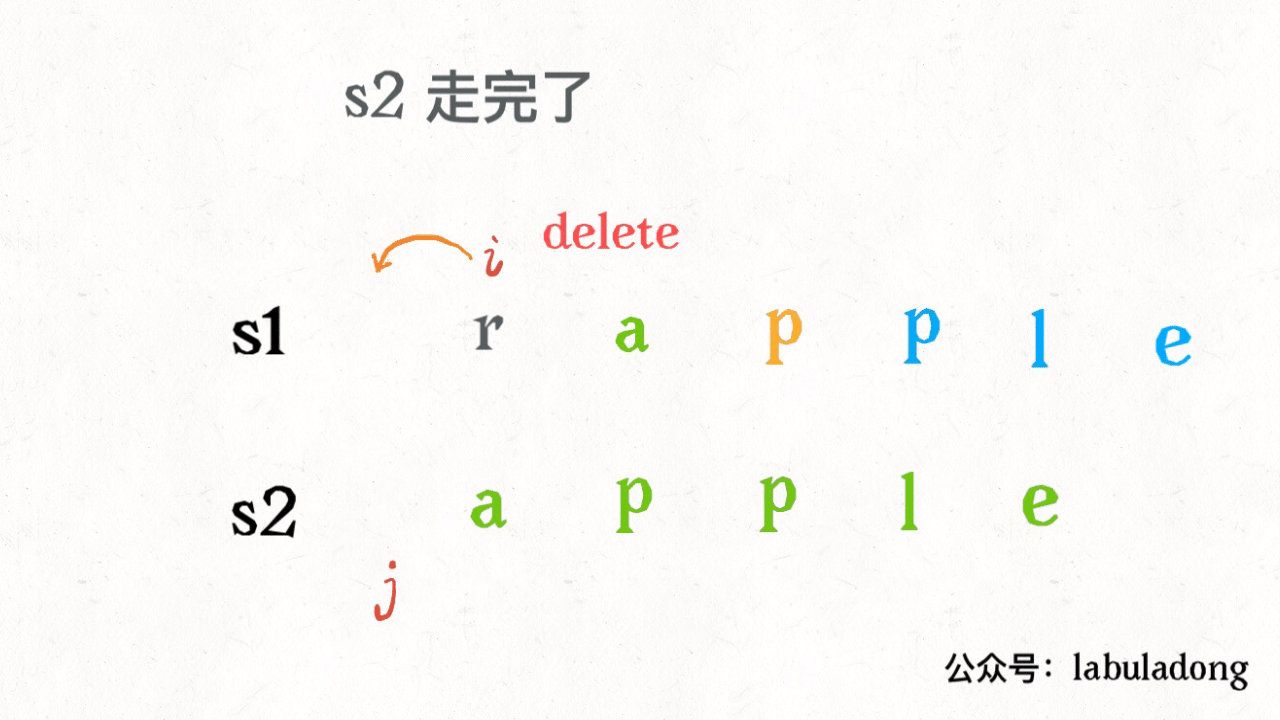
| W: | H:
| W: | H:
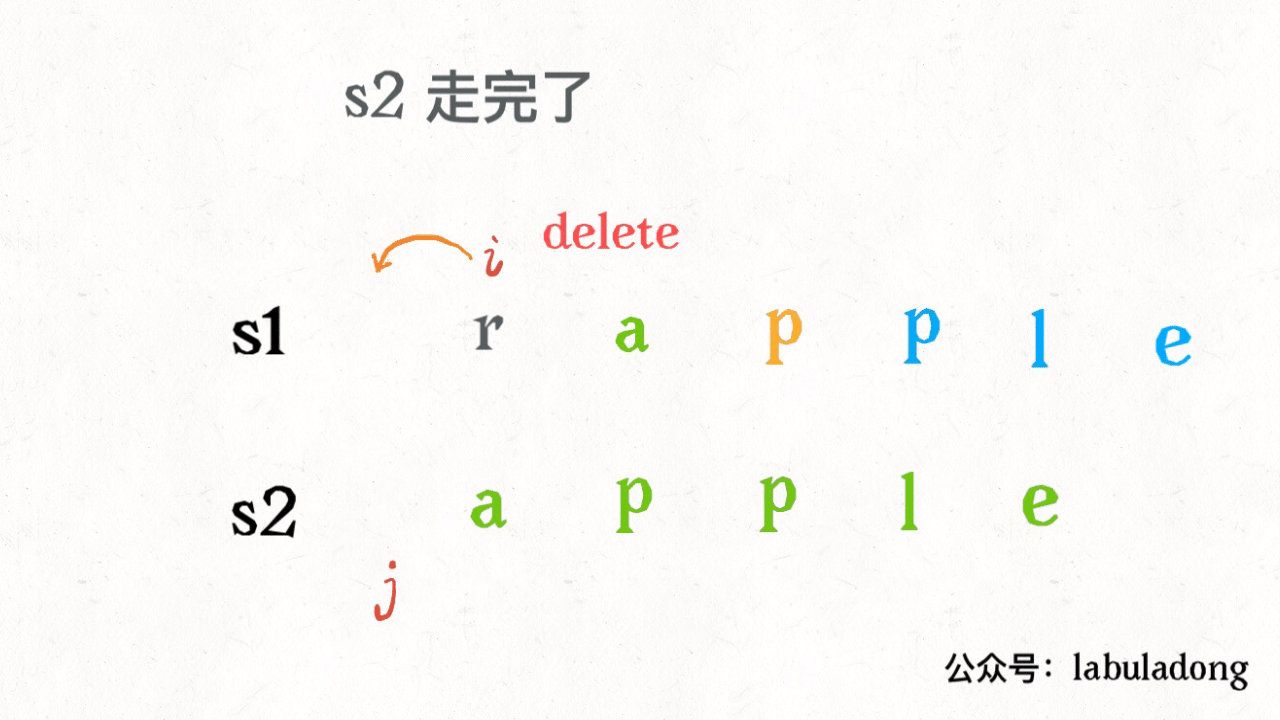
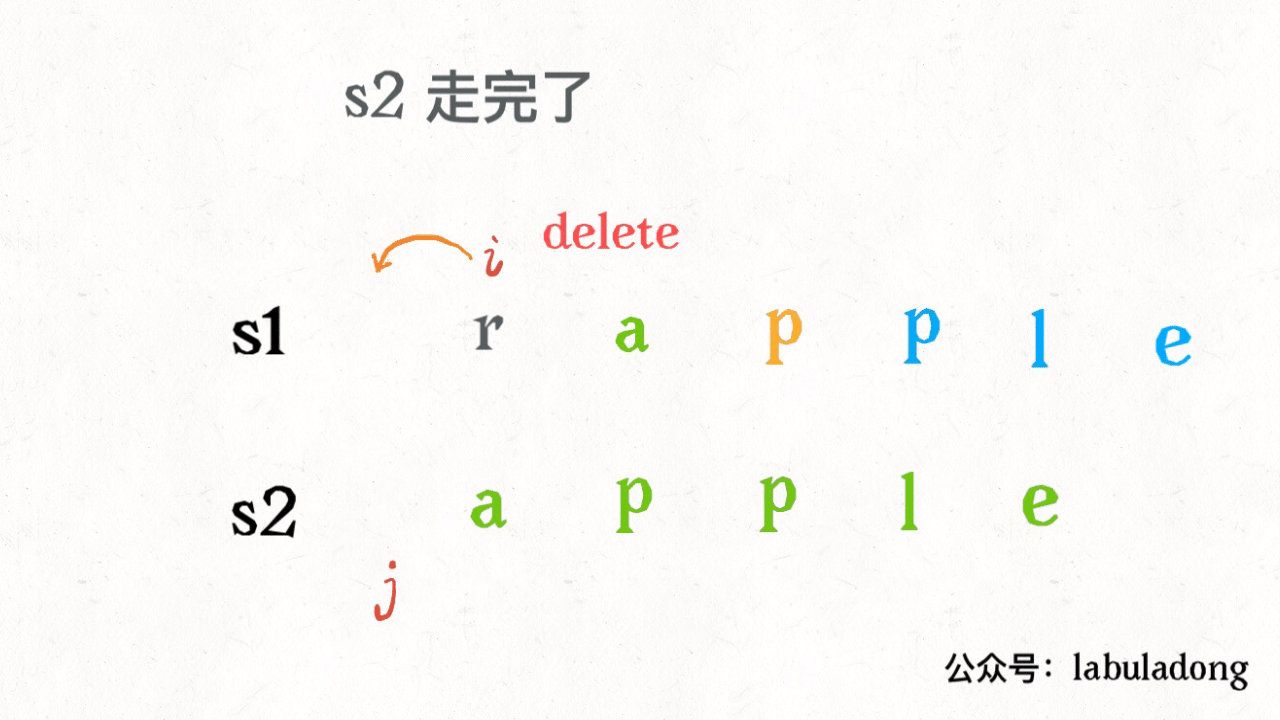
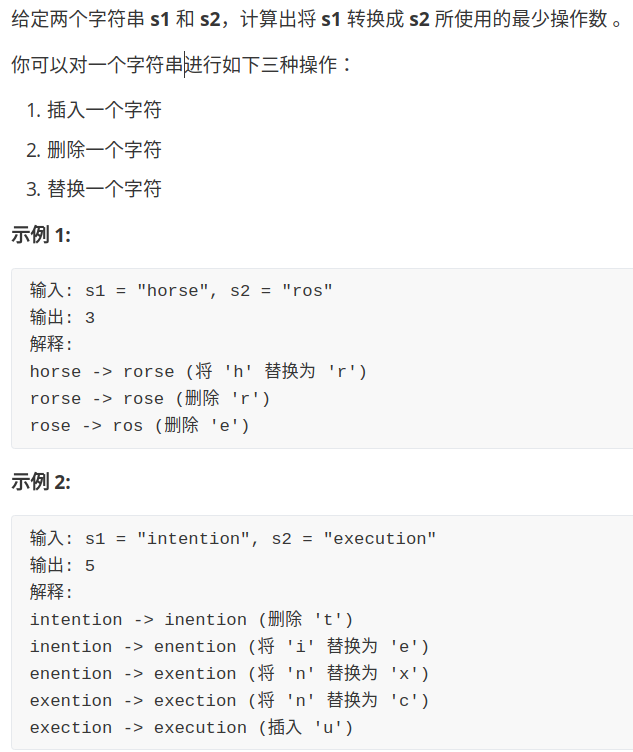
| W: | H:
| W: | H:
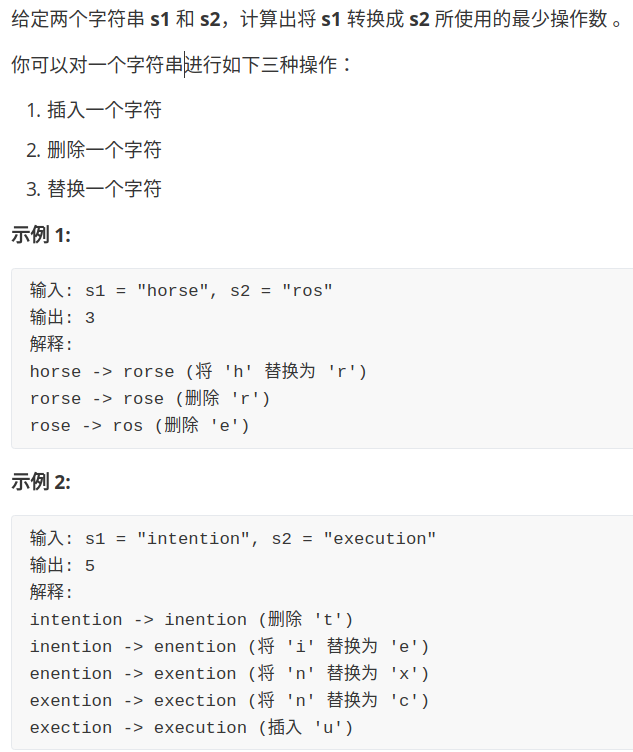
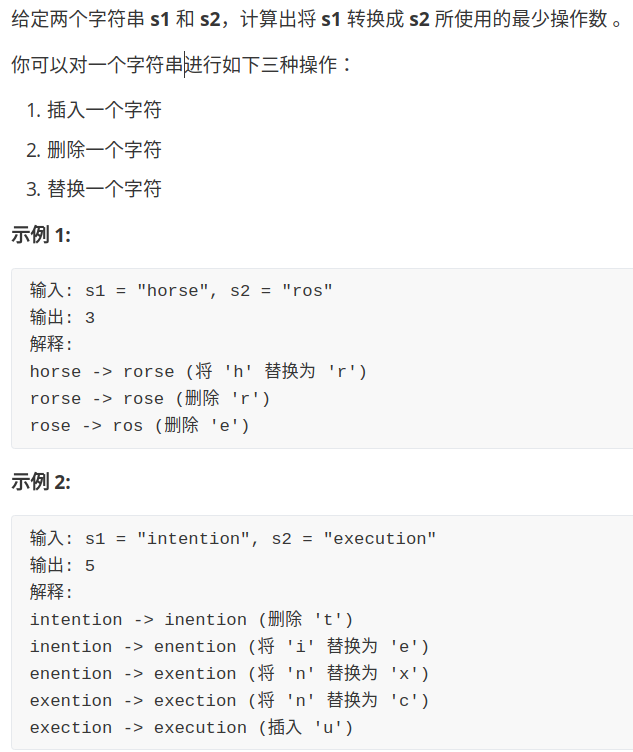
从无法访问的项目Fork
130.5 KB | W: | H:
124.7 KB | W: | H:
154.5 KB | W: | H:
142.1 KB | W: | H:
171.8 KB | W: | H:
155.4 KB | W: | H:
162.1 KB | W: | H:
146.1 KB | W: | H:
165.7 KB | W: | H:
148.7 KB | W: | H:
910.1 KB | W: | H:
311.9 KB | W: | H:
81.6 KB | W: | H:
46.8 KB | W: | H: