Resolve conflicts
Showing
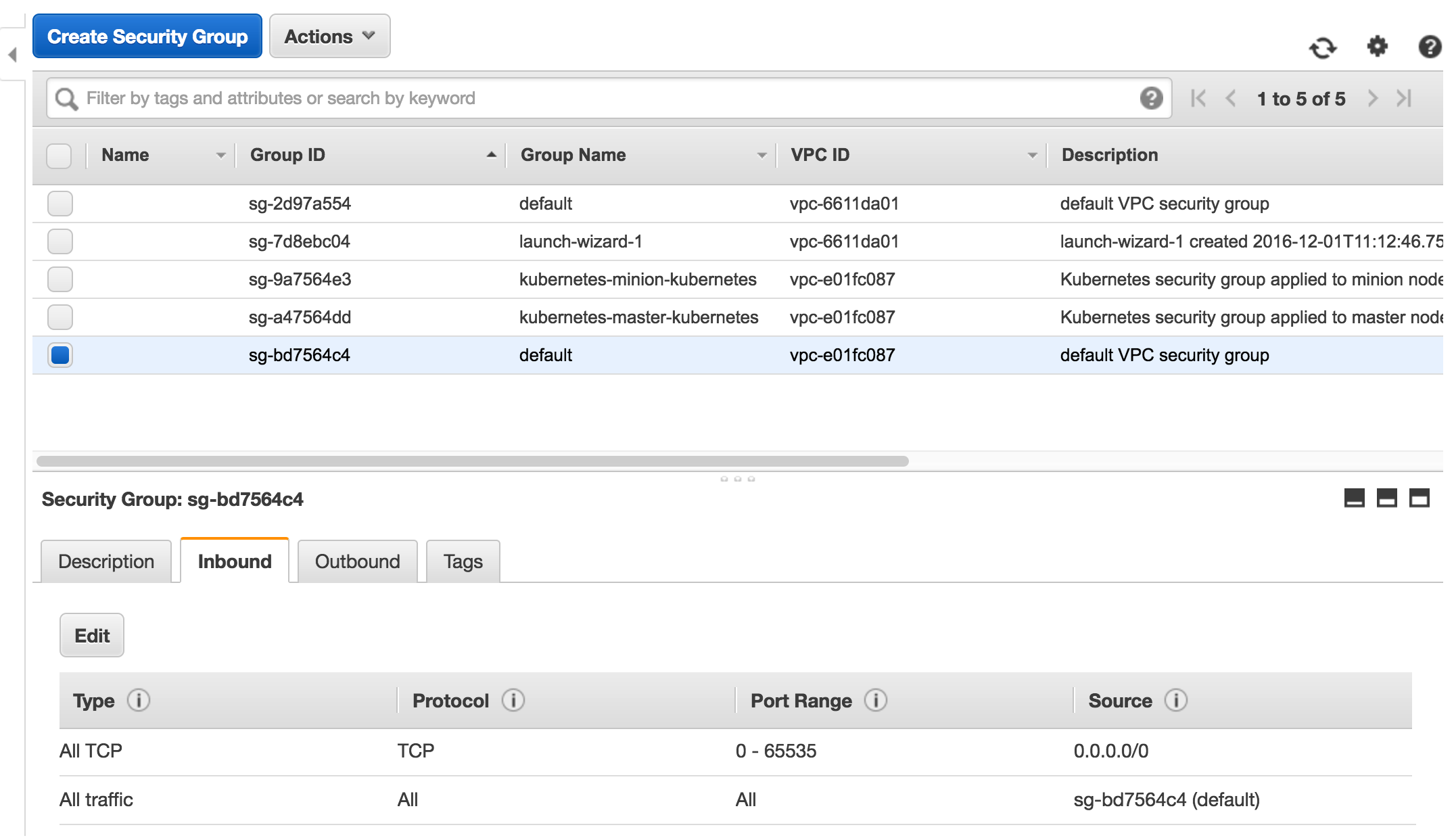
| W: | H:
| W: | H:
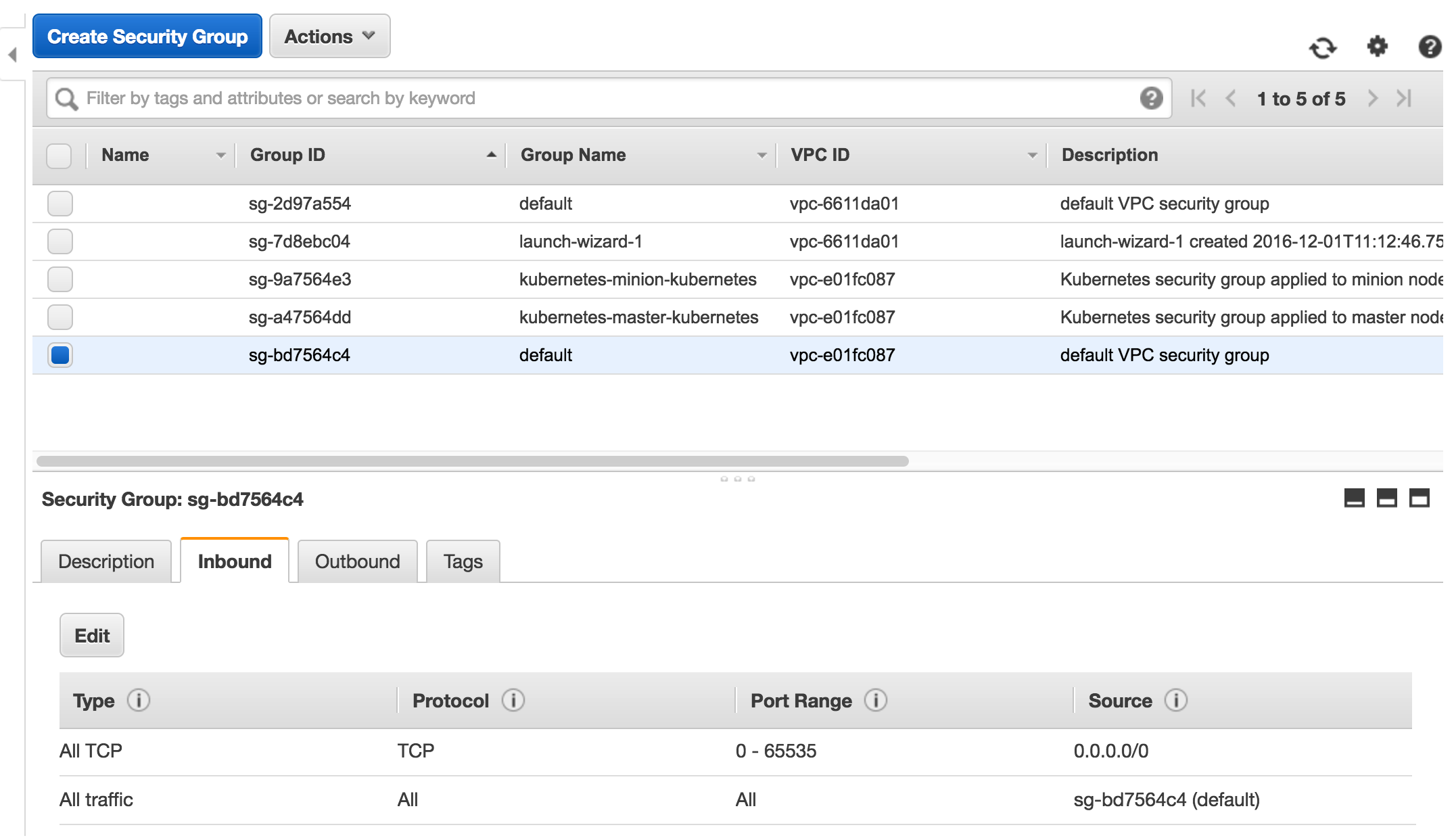
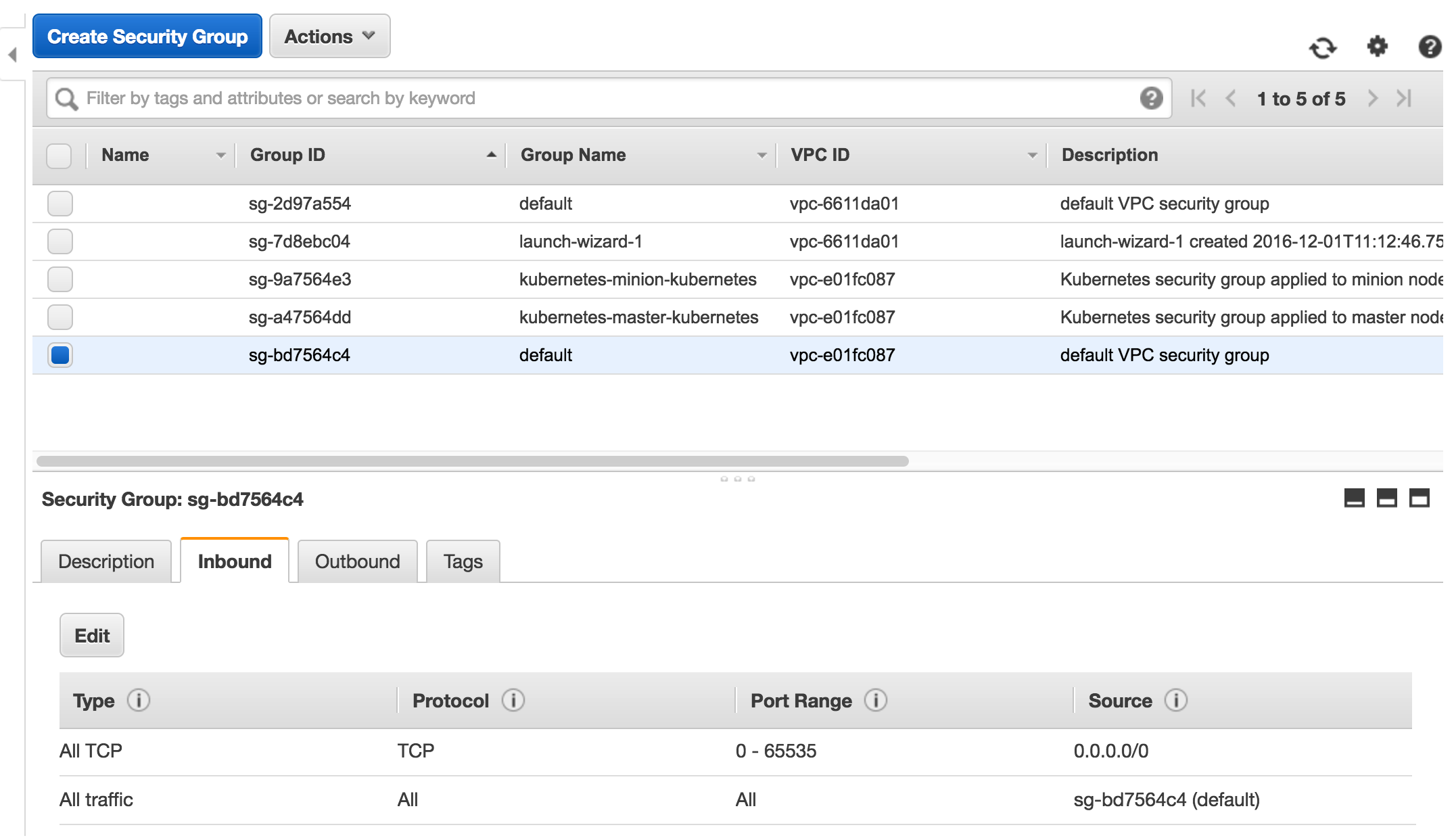
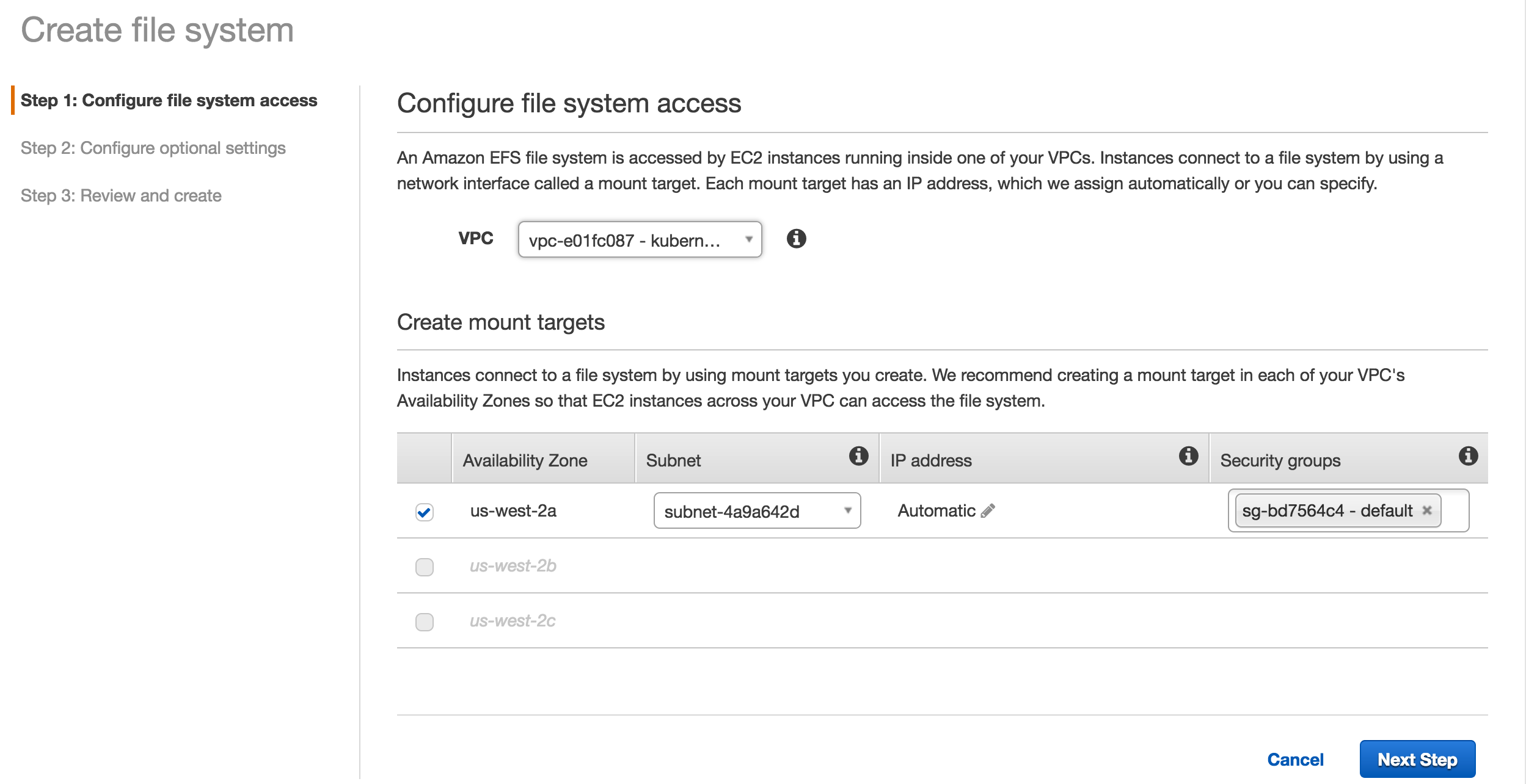
| W: | H:
| W: | H:
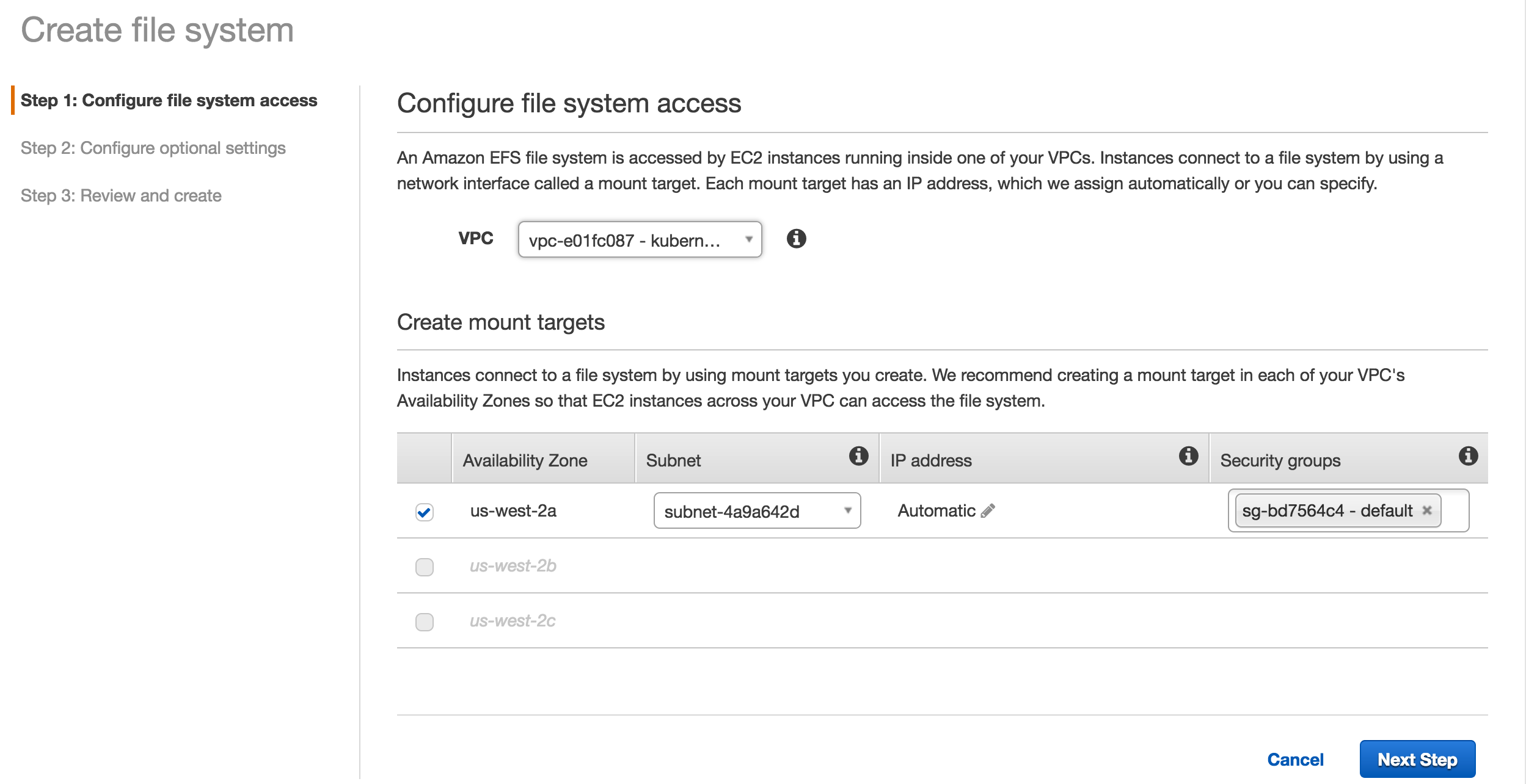
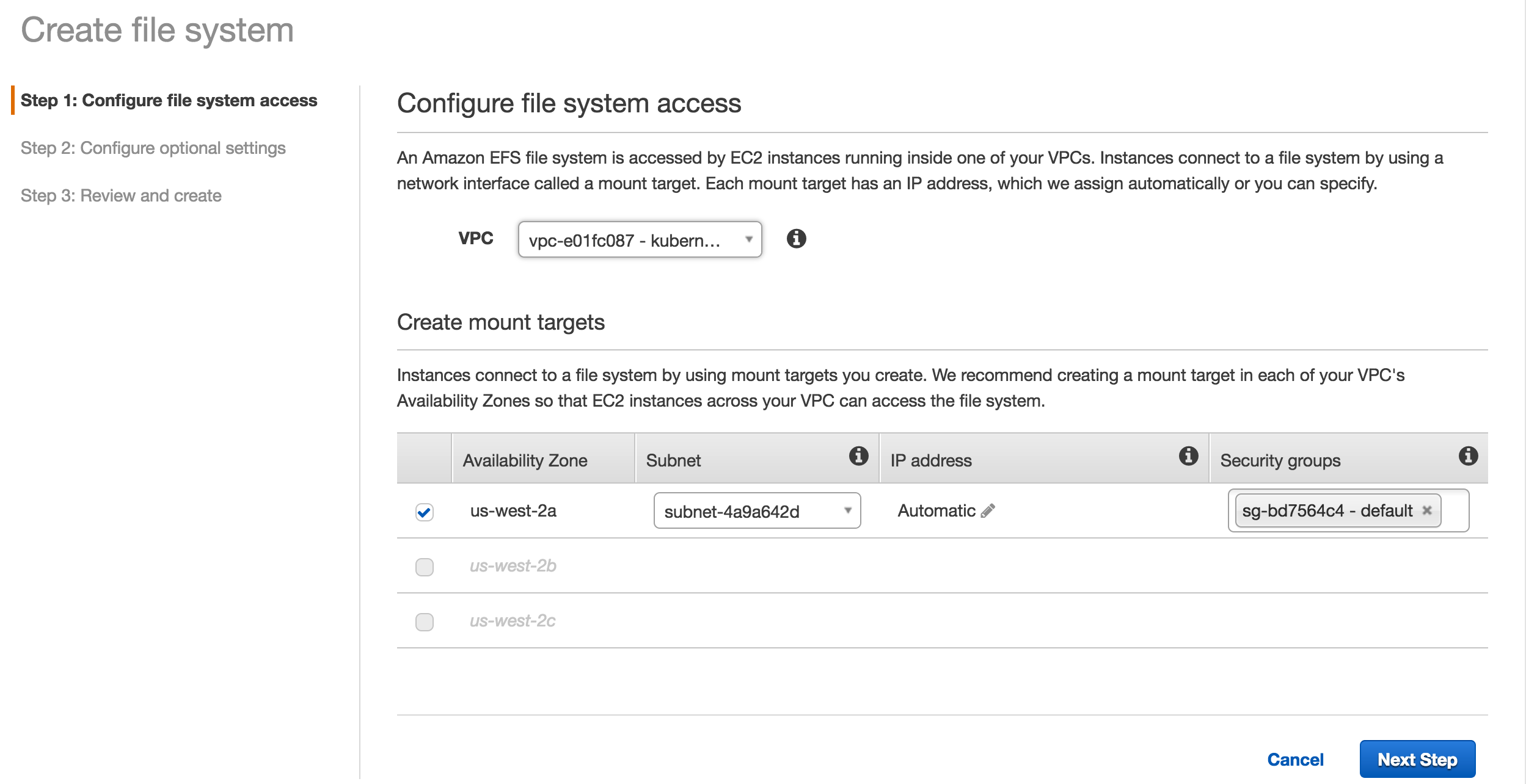
87.1 KB
paddle/function/MulOp.cpp
0 → 100644
paddle/function/MulOp.h
0 → 100644
paddle/function/MulOpGpu.cu
0 → 100644
paddle/function/MulOpTest.cpp
0 → 100644
python/paddle/v2/optimizer.py
0 → 100644