Merge branch 'develop' of https://github.com/PaddlePaddle/Paddle into lod_tensor_py
Showing
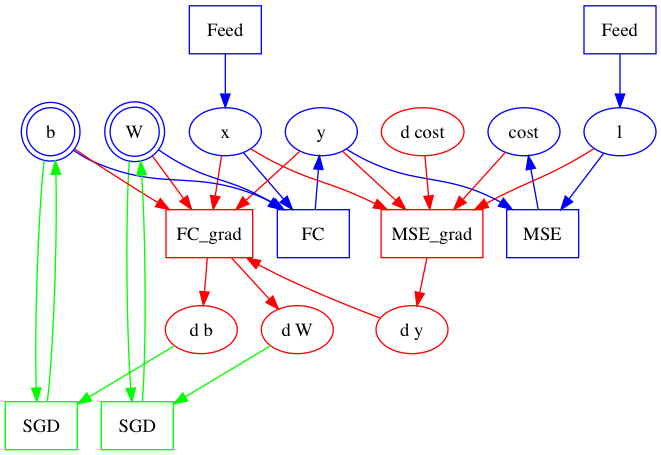
| W: | H:
| W: | H:
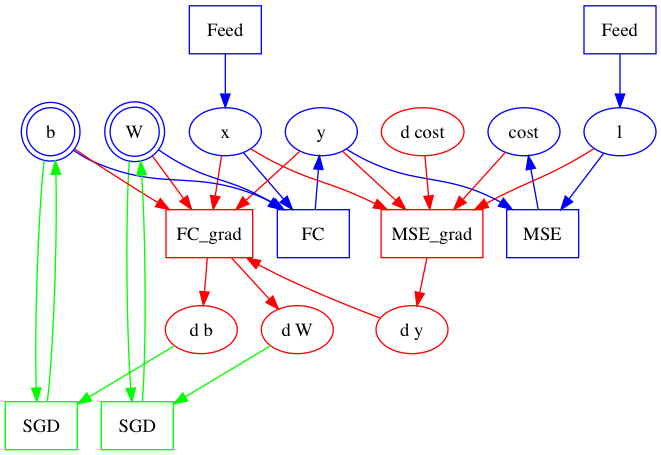
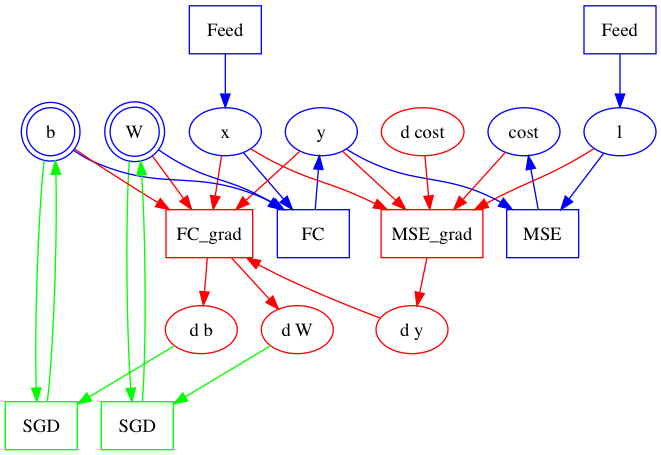
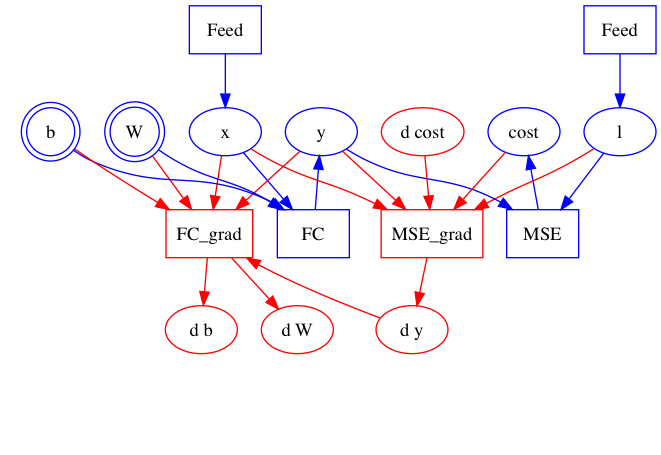
| W: | H:
| W: | H:
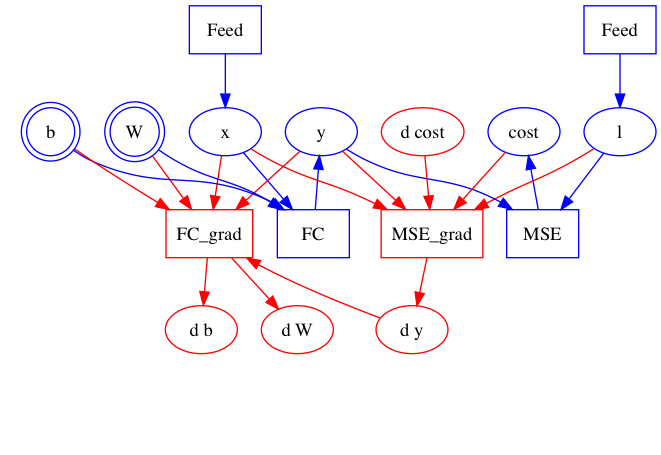
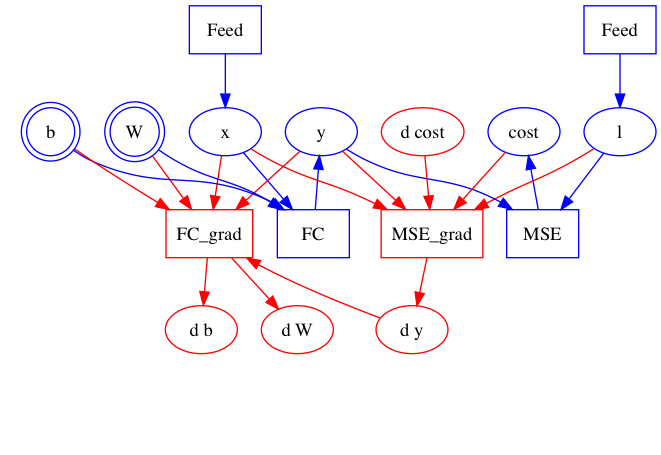
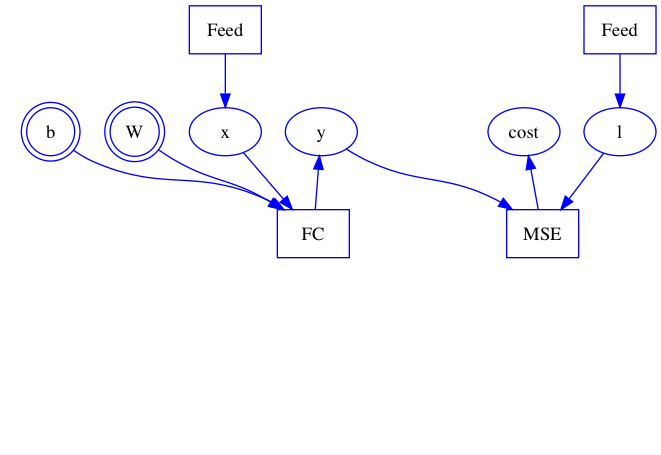
| W: | H:
| W: | H:
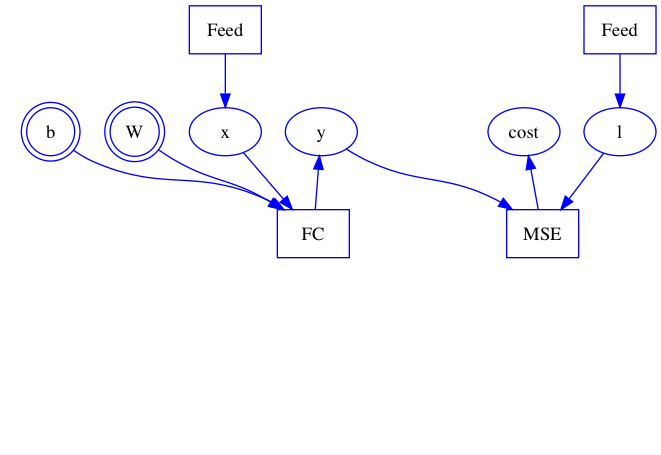
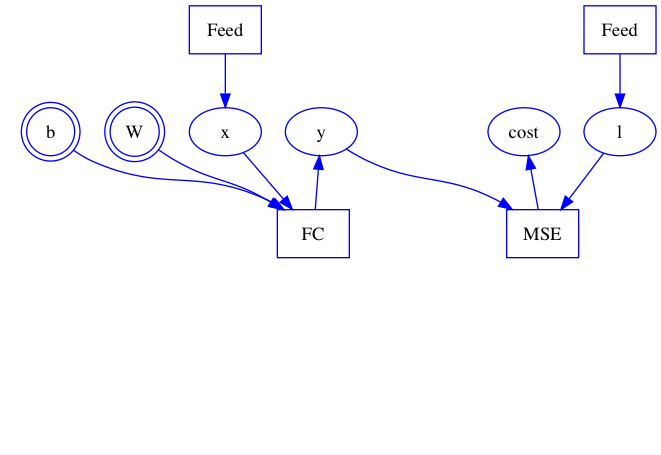
doc/design/var_desc.md
0 → 100644
paddle/function/GruFunctor.h
0 → 100644
paddle/function/SwitchOp.cpp
0 → 100644
paddle/function/SwitchOp.h
0 → 100644
paddle/function/SwitchOpGpu.cu
0 → 100644
paddle/function/SwitchOpTest.cpp
0 → 100644
此差异已折叠。
paddle/operators/identity_op.cc
0 → 100644
paddle/operators/math/im2col.cc
0 → 100644
paddle/operators/math/im2col.cu
0 → 100644
paddle/operators/math/im2col.h
0 → 100644
此差异已折叠。
此差异已折叠。
此差异已折叠。