Merge branch 'develop' into cross_entropy_over_beam
Showing
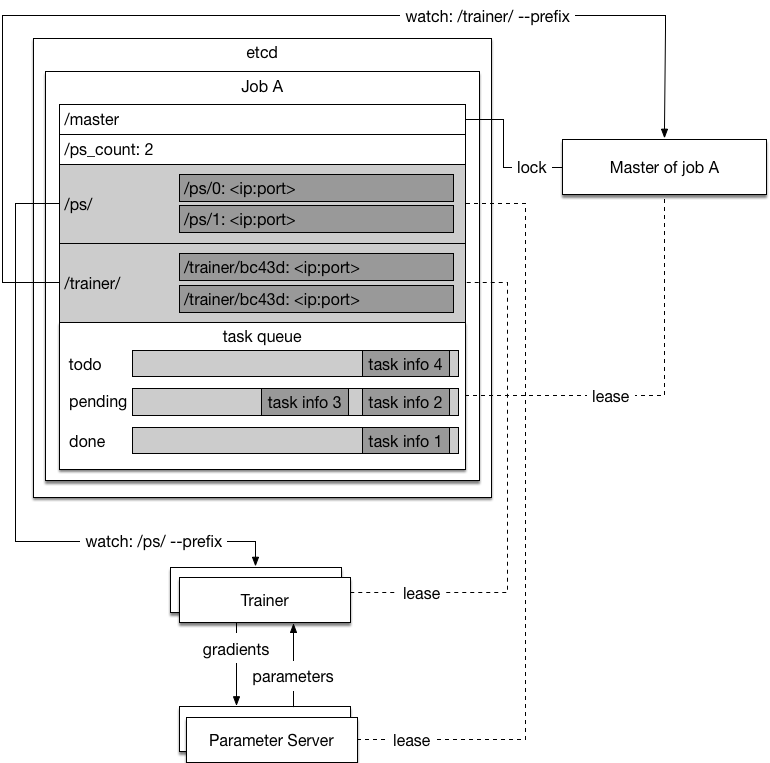
| W: | H:
| W: | H:
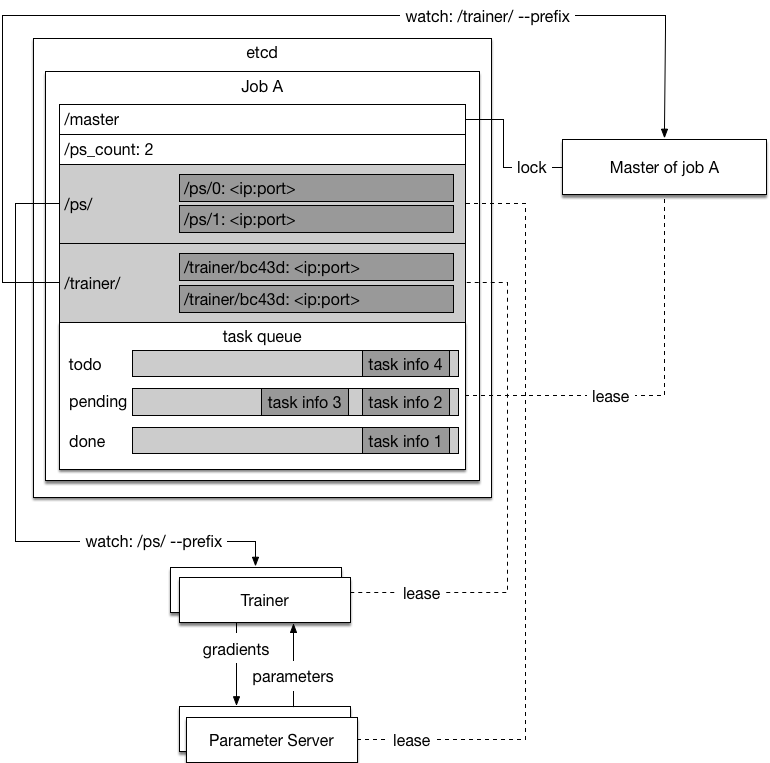
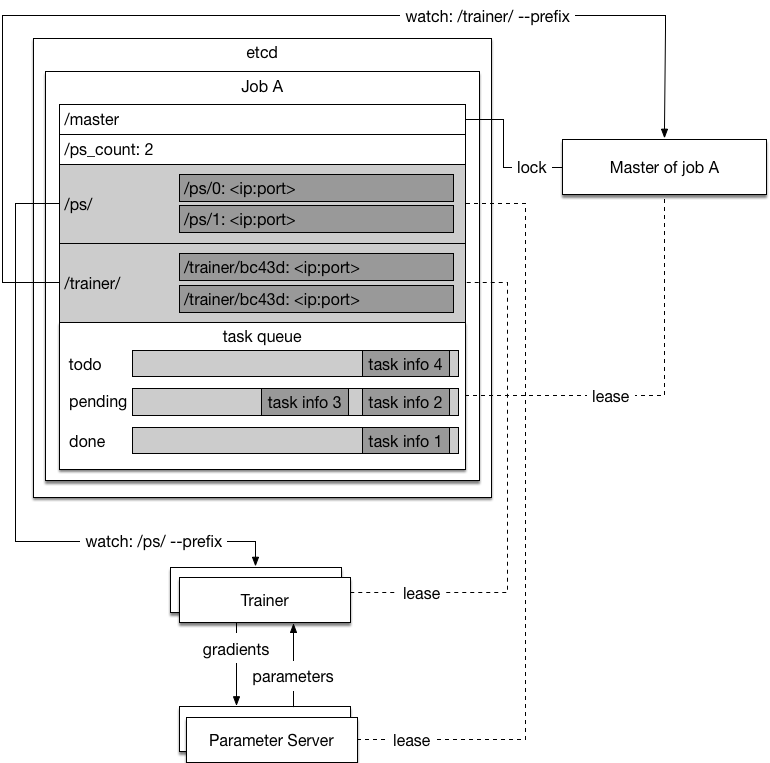
paddle/capi/export.map
0 → 100644
paddle/capi/export.sym
0 → 100644
paddle/operators/minus_op.cc
0 → 100644
paddle/operators/minus_op.cu
0 → 100644
paddle/operators/minus_op.h
0 → 100644
paddle/platform/cuda_helper.h
0 → 100644