diff --git a/configs/rec/PP-OCRv3/multi_language/arabic_PP-OCRv3_rec.yml b/configs/rec/PP-OCRv3/multi_language/arabic_PP-OCRv3_rec.yml
index 0ad1ab0adc189102ff07094fcda92d4f9ea9c662..8c650bd826d127f25c907f97d20d1a52f67f9203 100644
--- a/configs/rec/PP-OCRv3/multi_language/arabic_PP-OCRv3_rec.yml
+++ b/configs/rec/PP-OCRv3/multi_language/arabic_PP-OCRv3_rec.yml
@@ -12,7 +12,7 @@ Global:
checkpoints:
save_inference_dir:
use_visualdl: false
- infer_img: doc/imgs_words/ch/word_1.jpg
+ infer_img: ./doc/imgs_words/arabic/ar_2.jpg
character_dict_path: ppocr/utils/dict/arabic_dict.txt
max_text_length: &max_text_length 25
infer_mode: false
diff --git a/doc/doc_ch/algorithm_overview.md b/doc/doc_ch/algorithm_overview.md
index 858dc02b9d21981ce3b465f33ce494b290db51fb..ecb0e9dfefbfdef2f8cea273c4e3de468aa29415 100755
--- a/doc/doc_ch/algorithm_overview.md
+++ b/doc/doc_ch/algorithm_overview.md
@@ -24,7 +24,7 @@ PaddleOCR将**持续新增**支持OCR领域前沿算法与模型,**欢迎广
### 1.1 文本检测算法
已支持的文本检测算法列表(戳链接获取使用教程):
-- [x] [DB](./algorithm_det_db.md)
+- [x] [DB与DB++](./algorithm_det_db.md)
- [x] [EAST](./algorithm_det_east.md)
- [x] [SAST](./algorithm_det_sast.md)
- [x] [PSENet](./algorithm_det_psenet.md)
@@ -41,6 +41,7 @@ PaddleOCR将**持续新增**支持OCR领域前沿算法与模型,**欢迎广
|SAST|ResNet50_vd|91.39%|83.77%|87.42%|[训练模型](https://paddleocr.bj.bcebos.com/dygraph_v2.0/en/det_r50_vd_sast_icdar15_v2.0_train.tar)|
|PSE|ResNet50_vd|85.81%|79.53%|82.55%|[训练模型](https://paddleocr.bj.bcebos.com/dygraph_v2.1/en_det/det_r50_vd_pse_v2.0_train.tar)|
|PSE|MobileNetV3|82.20%|70.48%|75.89%|[训练模型](https://paddleocr.bj.bcebos.com/dygraph_v2.1/en_det/det_mv3_pse_v2.0_train.tar)|
+|DB++|ResNet50|90.89%|82.66%|86.58%|[合成数据预训练模型](https://paddleocr.bj.bcebos.com/dygraph_v2.1/en_det/ResNet50_dcn_asf_synthtext_pretrained.pdparams)/[训练模型](https://paddleocr.bj.bcebos.com/dygraph_v2.1/en_det/det_r50_db%2B%2B_icdar15_train.tar)|
在Total-text文本检测公开数据集上,算法效果如下:
@@ -129,10 +130,10 @@ PaddleOCR将**持续新增**支持OCR领域前沿算法与模型,**欢迎广
已支持的关键信息抽取算法列表(戳链接获取使用教程):
-- [x] [VI-LayoutXLM](./algorithm_kie_vi_laoutxlm.md)
-- [x] [LayoutLM](./algorithm_kie_laoutxlm.md)
-- [x] [LayoutLMv2](./algorithm_kie_laoutxlm.md)
-- [x] [LayoutXLM](./algorithm_kie_laoutxlm.md)
+- [x] [VI-LayoutXLM](./algorithm_kie_vi_layoutxlm.md)
+- [x] [LayoutLM](./algorithm_kie_layoutxlm.md)
+- [x] [LayoutLMv2](./algorithm_kie_layoutxlm.md)
+- [x] [LayoutXLM](./algorithm_kie_layoutxlm.md)
- [x] [SDMGR](././algorithm_kie_sdmgr.md)
在wildreceipt发票公开数据集上,算法复现效果如下:
diff --git a/doc/doc_en/algorithm_det_db_en.md b/doc/doc_en/algorithm_det_db_en.md
index f5f333a039acded88f0f28d302821c5eb10d7402..fde344c3572f771e3e0fe5f9f62282cd1ae0a024 100644
--- a/doc/doc_en/algorithm_det_db_en.md
+++ b/doc/doc_en/algorithm_det_db_en.md
@@ -1,4 +1,4 @@
-# DB
+# DB && DB++
- [1. Introduction](#1)
- [2. Environment](#2)
@@ -21,13 +21,23 @@ Paper:
> Liao, Minghui and Wan, Zhaoyi and Yao, Cong and Chen, Kai and Bai, Xiang
> AAAI, 2020
+> [Real-Time Scene Text Detection with Differentiable Binarization and Adaptive Scale Fusion](https://arxiv.org/abs/2202.10304)
+> Liao, Minghui and Zou, Zhisheng and Wan, Zhaoyi and Yao, Cong and Bai, Xiang
+> TPAMI, 2022
+
On the ICDAR2015 dataset, the text detection result is as follows:
|Model|Backbone|Configuration|Precision|Recall|Hmean|Download|
| --- | --- | --- | --- | --- | --- | --- |
|DB|ResNet50_vd|[configs/det/det_r50_vd_db.yml](../../configs/det/det_r50_vd_db.yml)|86.41%|78.72%|82.38%|[trained model](https://paddleocr.bj.bcebos.com/dygraph_v2.0/en/det_r50_vd_db_v2.0_train.tar)|
|DB|MobileNetV3|[configs/det/det_mv3_db.yml](../../configs/det/det_mv3_db.yml)|77.29%|73.08%|75.12%|[trained model](https://paddleocr.bj.bcebos.com/dygraph_v2.0/en/det_mv3_db_v2.0_train.tar)|
+|DB++|ResNet50|[configs/det/det_r50_db++_ic15.yml](../../configs/det/det_r50_db++_ic15.yml)|90.89%|82.66%|86.58%|[pretrained model](https://paddleocr.bj.bcebos.com/dygraph_v2.1/en_det/ResNet50_dcn_asf_synthtext_pretrained.pdparams)/[trained model](https://paddleocr.bj.bcebos.com/dygraph_v2.1/en_det/det_r50_db%2B%2B_icdar15_train.tar)|
+
+On the TD_TR dataset, the text detection result is as follows:
+|Model|Backbone|Configuration|Precision|Recall|Hmean|Download|
+| --- | --- | --- | --- | --- | --- | --- |
+|DB++|ResNet50|[configs/det/det_r50_db++_td_tr.yml](../../configs/det/det_r50_db++_td_tr.yml)|92.92%|86.48%|89.58%|[pretrained model](https://paddleocr.bj.bcebos.com/dygraph_v2.1/en_det/ResNet50_dcn_asf_synthtext_pretrained.pdparams)/[trained model](https://paddleocr.bj.bcebos.com/dygraph_v2.1/en_det/det_r50_db%2B%2B_td_tr_train.tar)|
## 2. Environment
@@ -96,4 +106,12 @@ More deployment schemes supported for DB:
pages={11474--11481},
year={2020}
}
-```
\ No newline at end of file
+
+@article{liao2022real,
+ title={Real-Time Scene Text Detection with Differentiable Binarization and Adaptive Scale Fusion},
+ author={Liao, Minghui and Zou, Zhisheng and Wan, Zhaoyi and Yao, Cong and Bai, Xiang},
+ journal={IEEE Transactions on Pattern Analysis and Machine Intelligence},
+ year={2022},
+ publisher={IEEE}
+}
+```
diff --git a/doc/doc_en/algorithm_overview_en.md b/doc/doc_en/algorithm_overview_en.md
index 5bf569e3e1649cfabbe196be7e1a55d1caa3bf61..bca22f78482980bed18d6447d0cf07b27c26720d 100755
--- a/doc/doc_en/algorithm_overview_en.md
+++ b/doc/doc_en/algorithm_overview_en.md
@@ -22,7 +22,7 @@ Developers are welcome to contribute more algorithms! Please refer to [add new a
### 1.1 Text Detection Algorithms
Supported text detection algorithms (Click the link to get the tutorial):
-- [x] [DB](./algorithm_det_db_en.md)
+- [x] [DB && DB++](./algorithm_det_db_en.md)
- [x] [EAST](./algorithm_det_east_en.md)
- [x] [SAST](./algorithm_det_sast_en.md)
- [x] [PSENet](./algorithm_det_psenet_en.md)
@@ -39,6 +39,7 @@ On the ICDAR2015 dataset, the text detection result is as follows:
|SAST|ResNet50_vd|91.39%|83.77%|87.42%|[trained model](https://paddleocr.bj.bcebos.com/dygraph_v2.0/en/det_r50_vd_sast_icdar15_v2.0_train.tar)|
|PSE|ResNet50_vd|85.81%|79.53%|82.55%|[trianed model](https://paddleocr.bj.bcebos.com/dygraph_v2.1/en_det/det_r50_vd_pse_v2.0_train.tar)|
|PSE|MobileNetV3|82.20%|70.48%|75.89%|[trianed model](https://paddleocr.bj.bcebos.com/dygraph_v2.1/en_det/det_mv3_pse_v2.0_train.tar)|
+|DB++|ResNet50|90.89%|82.66%|86.58%|[pretrained model](https://paddleocr.bj.bcebos.com/dygraph_v2.1/en_det/ResNet50_dcn_asf_synthtext_pretrained.pdparams)/[trained model](https://paddleocr.bj.bcebos.com/dygraph_v2.1/en_det/det_r50_db%2B%2B_icdar15_train.tar)|
On Total-Text dataset, the text detection result is as follows:
@@ -127,10 +128,10 @@ On the PubTabNet dataset, the algorithm result is as follows:
Supported KIE algorithms (Click the link to get the tutorial):
-- [x] [VI-LayoutXLM](./algorithm_kie_vi_laoutxlm_en.md)
-- [x] [LayoutLM](./algorithm_kie_laoutxlm_en.md)
-- [x] [LayoutLMv2](./algorithm_kie_laoutxlm_en.md)
-- [x] [LayoutXLM](./algorithm_kie_laoutxlm_en.md)
+- [x] [VI-LayoutXLM](./algorithm_kie_vi_layoutxlm_en.md)
+- [x] [LayoutLM](./algorithm_kie_layoutxlm_en.md)
+- [x] [LayoutLMv2](./algorithm_kie_layoutxlm_en.md)
+- [x] [LayoutXLM](./algorithm_kie_layoutxlm_en.md)
- [x] [SDMGR](./algorithm_kie_sdmgr_en.md)
On wildreceipt dataset, the algorithm result is as follows:
diff --git a/doc/overview_en.png b/doc/overview_en.png
deleted file mode 100644
index b44da4e9874d6a2162a8bb05ff1b479875bd65f3..0000000000000000000000000000000000000000
Binary files a/doc/overview_en.png and /dev/null differ
diff --git a/doc/ppocr_v3/svtr_tiny.jpg b/doc/ppocr_v3/svtr_tiny.jpg
deleted file mode 100644
index 26261047ef253e9802956f4c64449870d10de850..0000000000000000000000000000000000000000
Binary files a/doc/ppocr_v3/svtr_tiny.jpg and /dev/null differ
diff --git a/ppocr/postprocess/rec_postprocess.py b/ppocr/postprocess/rec_postprocess.py
index fc9fccfb143bf31ec66989e279d0bcc1c9baa5cc..f77631700648e84f28223cb14738e7b4ab679012 100644
--- a/ppocr/postprocess/rec_postprocess.py
+++ b/ppocr/postprocess/rec_postprocess.py
@@ -45,6 +45,27 @@ class BaseRecLabelDecode(object):
self.dict[char] = i
self.character = dict_character
+ if 'arabic' in character_dict_path:
+ self.reverse = True
+ else:
+ self.reverse = False
+
+ def pred_reverse(self, pred):
+ pred_re = []
+ c_current = ''
+ for c in pred:
+ if not bool(re.search('[a-zA-Z0-9 :*./%+-]', c)):
+ if c_current != '':
+ pred_re.append(c_current)
+ pred_re.append(c)
+ c_current = ''
+ else:
+ c_current += c
+ if c_current != '':
+ pred_re.append(c_current)
+
+ return ''.join(pred_re[::-1])
+
def add_special_char(self, dict_character):
return dict_character
@@ -73,6 +94,10 @@ class BaseRecLabelDecode(object):
conf_list = [0]
text = ''.join(char_list)
+
+ if self.reverse: # for arabic rec
+ text = self.pred_reverse(text)
+
result_list.append((text, np.mean(conf_list).tolist()))
return result_list
diff --git a/ppocr/utils/dict/arabic_dict.txt b/ppocr/utils/dict/arabic_dict.txt
index e97abf39274df77fbad066ee4635aebc6743140c..916d421c53bad563dfd980c1b64dcce07a3c9d24 100644
--- a/ppocr/utils/dict/arabic_dict.txt
+++ b/ppocr/utils/dict/arabic_dict.txt
@@ -1,4 +1,3 @@
-
!
#
$
diff --git a/ppstructure/docs/models_list_en.md b/ppstructure/docs/models_list_en.md
index 85531fb753c4e32f0cdc9296ab97a9faebbb0ebd..291d42f995fdd7fabc293a0e4df35c2249945fd2 100644
--- a/ppstructure/docs/models_list_en.md
+++ b/ppstructure/docs/models_list_en.md
@@ -13,7 +13,7 @@
|model name| description | inference model size |download|dict path|
| --- |---------------------------------------------------------------------------------------------------------------------------------------------------------| --- | --- | --- |
| picodet_lcnet_x1_0_fgd_layout | The layout analysis English model trained on the PubLayNet dataset based on PicoDet LCNet_x1_0 and FGD . the model can recognition 5 types of areas such as **Text, Title, Table, Picture and List** | 9.7M | [inference model](https://paddleocr.bj.bcebos.com/ppstructure/models/layout/picodet_lcnet_x1_0_fgd_layout_infer.tar) / [trained model](https://paddleocr.bj.bcebos.com/ppstructure/models/layout/picodet_lcnet_x1_0_fgd_layout.pdparams) | [PubLayNet dict](../../ppocr/utils/dict/layout_dict/layout_publaynet_dict.txt) |
-| ppyolov2_r50vd_dcn_365e_publaynet | The layout analysis English model trained on the PubLayNet dataset based on PP-YOLOv2 | 221M | [inference_moel]](https://paddle-model-ecology.bj.bcebos.com/model/layout-parser/ppyolov2_r50vd_dcn_365e_publaynet.tar) / [trained model](https://paddle-model-ecology.bj.bcebos.com/model/layout-parser/ppyolov2_r50vd_dcn_365e_publaynet_pretrained.pdparams) | sme as above |
+| ppyolov2_r50vd_dcn_365e_publaynet | The layout analysis English model trained on the PubLayNet dataset based on PP-YOLOv2 | 221M | [inference_moel](https://paddle-model-ecology.bj.bcebos.com/model/layout-parser/ppyolov2_r50vd_dcn_365e_publaynet.tar) / [trained model](https://paddle-model-ecology.bj.bcebos.com/model/layout-parser/ppyolov2_r50vd_dcn_365e_publaynet_pretrained.pdparams) | same as above |
| picodet_lcnet_x1_0_fgd_layout_cdla | The layout analysis Chinese model trained on the CDLA dataset, the model can recognition 10 types of areas such as **Table、Figure、Figure caption、Table、Table caption、Header、Footer、Reference、Equation** | 9.7M | [inference model](https://paddleocr.bj.bcebos.com/ppstructure/models/layout/picodet_lcnet_x1_0_fgd_layout_cdla_infer.tar) / [trained model](https://paddleocr.bj.bcebos.com/ppstructure/models/layout/picodet_lcnet_x1_0_fgd_layout_cdla.pdparams) | [CDLA dict](../../ppocr/utils/dict/layout_dict/layout_cdla_dict.txt) |
| picodet_lcnet_x1_0_fgd_layout_table | The layout analysis model trained on the table dataset, the model can detect tables in Chinese and English documents | 9.7M | [inference model](https://paddleocr.bj.bcebos.com/ppstructure/models/layout/picodet_lcnet_x1_0_fgd_layout_table_infer.tar) / [trained model](https://paddleocr.bj.bcebos.com/ppstructure/models/layout/picodet_lcnet_x1_0_fgd_layout_table.pdparams) | [Table dict](../../ppocr/utils/dict/layout_dict/layout_table_dict.txt) |
| ppyolov2_r50vd_dcn_365e_tableBank_word | The layout analysis model trained on the TableBank Word dataset based on PP-YOLOv2, the model can detect tables in English documents | 221M | [inference model](https://paddle-model-ecology.bj.bcebos.com/model/layout-parser/ppyolov2_r50vd_dcn_365e_tableBank_word.tar) | same as above |
diff --git a/ppstructure/kie/README.md b/ppstructure/kie/README.md
index adb19a3ca729821ab16bf8f0f8ec14c2376de1de..d9471fb18d140704fdeb76c321f8a001426f872d 100644
--- a/ppstructure/kie/README.md
+++ b/ppstructure/kie/README.md
@@ -242,9 +242,7 @@ For training, evaluation and inference tutorial for KIE models, please refer to
For training, evaluation and inference tutorial for text detection models, please refer to [text detection doc](../../doc/doc_en/detection_en.md).
-For training, evaluation and inference tutorial for text recognition models, please refer to [text recognition doc](../../doc/doc_en/recognition.md).
-
-If you want to finish the KIE tasks in your scene, and don't know what to prepare, please refer to [End cdoc](../../doc/doc_en/recognition.md).
+For training, evaluation and inference tutorial for text recognition models, please refer to [text recognition doc](../../doc/doc_en/recognition_en.md).
To complete the key information extraction task in your own scenario from data preparation to model selection, please refer to: [Guide to End-to-end KIE](./how_to_do_kie_en.md)。
diff --git a/ppstructure/layout/README.md b/ppstructure/layout/README.md
new file mode 100644
index 0000000000000000000000000000000000000000..01faa7b279c618602cafb8ef7d086753061ea559
--- /dev/null
+++ b/ppstructure/layout/README.md
@@ -0,0 +1,468 @@
+English | [简体中文](README_ch.md)
+
+# Layout analysis
+
+- [1. Introduction](#1-Introduction)
+- [2. Install](#2-Install)
+ - [2.1 Install PaddlePaddle](#21-Install-paddlepaddle)
+ - [2.2 Install PaddleDetection](#22-Install-paddledetection)
+- [3. Data preparation](#3-Data-preparation)
+ - [3.1 English data set](#31-English-data-set)
+ - [3.2 More datasets](#32-More-datasets)
+- [4. Start training](#4-Start-training)
+ - [4.1 Train](#41-Train)
+ - [4.2 FGD Distillation training](#42-FGD-Distillation-training)
+- [5. Model evaluation and prediction](#5-Model-evaluation-and-prediction)
+ - [5.1 Indicator evaluation](#51-Indicator-evaluation)
+ - [5.2 Test layout analysis results](#52-Test-layout-analysis-results)
+- [6 Model export and inference](#6-Model-export-and-inference)
+ - [6.1 Model export](#61-Model-export)
+ - [6.2 Model inference](#62-Model-inference)
+
+
+## 1. Introduction
+
+Layout analysis refers to the regional division of documents in the form of pictures and the positioning of key areas, such as text, title, table, picture, etc. The layout analysis algorithm is based on the lightweight model PP-picodet of [PaddleDetection]( https://github.com/PaddlePaddle/PaddleDetection )
+
+
+

+
+
+
+
+## 2. Install
+
+### 2.1. Install PaddlePaddle
+
+- **(1) Install PaddlePaddle**
+
+```bash
+python3 -m pip install --upgrade pip
+
+# GPU Install
+python3 -m pip install "paddlepaddle-gpu>=2.3" -i https://mirror.baidu.com/pypi/simple
+
+# CPU Install
+python3 -m pip install "paddlepaddle>=2.3" -i https://mirror.baidu.com/pypi/simple
+```
+For more requirements, please refer to the instructions in the [Install file](https://www.paddlepaddle.org.cn/install/quick)。
+
+### 2.2. Install PaddleDetection
+
+- **(1)Download PaddleDetection Source code**
+
+```bash
+git clone https://github.com/PaddlePaddle/PaddleDetection.git
+```
+
+- **(2)Install third-party libraries**
+
+```bash
+cd PaddleDetection
+python3 -m pip install -r requirements.txt
+```
+
+## 3. Data preparation
+
+If you want to experience the prediction process directly, you can skip data preparation and download the pre-training model.
+
+### 3.1. English data set
+
+Download document analysis data set [PubLayNet](https://developer.ibm.com/exchanges/data/all/publaynet/)(Dataset 96G),contains 5 classes:`{0: "Text", 1: "Title", 2: "List", 3:"Table", 4:"Figure"}`
+
+```
+# Download data
+wget https://dax-cdn.cdn.appdomain.cloud/dax-publaynet/1.0.0/publaynet.tar.gz
+# Decompress data
+tar -xvf publaynet.tar.gz
+```
+
+Uncompressed **directory structure:**
+
+```
+|-publaynet
+ |- test
+ |- PMC1277013_00004.jpg
+ |- PMC1291385_00002.jpg
+ | ...
+ |- train.json
+ |- train
+ |- PMC1291385_00002.jpg
+ |- PMC1277013_00004.jpg
+ | ...
+ |- val.json
+ |- val
+ |- PMC538274_00004.jpg
+ |- PMC539300_00004.jpg
+ | ...
+```
+
+**data distribution:**
+
+| File or Folder | Description | num |
+| :------------- | :------------- | ------- |
+| `train/` | Training set pictures | 335,703 |
+| `val/` | Verification set pictures | 11,245 |
+| `test/` | Test set pictures | 11,405 |
+| `train.json` | Training set annotation files | - |
+| `val.json` | Validation set dimension files | - |
+
+**Data Annotation**
+
+The JSON file contains the annotations of all images, and the data is stored in a dictionary nested manner.Contains the following keys:
+
+- info,represents the dimension file info。
+
+- licenses,represents the dimension file licenses。
+
+- images,represents the list of image information in the annotation file,each element is the information of an image。The information of one of the images is as follows:
+
+ ```
+ {
+ 'file_name': 'PMC4055390_00006.jpg', # file_name
+ 'height': 601, # image height
+ 'width': 792, # image width
+ 'id': 341427 # image id
+ }
+ ```
+
+- annotations, represents the list of annotation information of the target object in the annotation file,each element is the annotation information of a target object。The following is the annotation information of one of the target objects:
+
+ ```
+ {
+
+ 'segmentation': # Segmentation annotation of objects
+ 'area': 60518.099043117836, # Area of object
+ 'iscrowd': 0, # iscrowd
+ 'image_id': 341427, # image id
+ 'bbox': [50.58, 490.86, 240.15, 252.16], # bbox [x1,y1,w,h]
+ 'category_id': 1, # category_id
+ 'id': 3322348 # image id
+ }
+ ```
+
+### 3.2. More datasets
+
+We provide CDLA(Chinese layout analysis), TableBank(Table layout analysis)etc. data set download links,process to the JSON format of the above annotation file,that is, the training can be conducted in the same way。
+
+| dataset | 简介 |
+| ------------------------------------------------------------ | ------------------------------------------------------------ |
+| [cTDaR2019_cTDaR](https://cndplab-founder.github.io/cTDaR2019/) | For form detection (TRACKA) and form identification (TRACKB).Image types include historical data sets (beginning with cTDaR_t0, such as CTDAR_T00872.jpg) and modern data sets (beginning with cTDaR_t1, CTDAR_T10482.jpg). |
+| [IIIT-AR-13K](http://cvit.iiit.ac.in/usodi/iiitar13k.php) | Data sets constructed by manually annotating figures or pages from publicly available annual reports, containing 5 categories:table, figure, natural image, logo, and signature. |
+| [TableBank](https://github.com/doc-analysis/TableBank) | For table detection and recognition of large datasets, including Word and Latex document formats |
+| [CDLA](https://github.com/buptlihang/CDLA) | Chinese document layout analysis data set, for Chinese literature (paper) scenarios, including 10 categories:Table, Figure, Figure caption, Table, Table caption, Header, Footer, Reference, Equation |
+| [DocBank](https://github.com/doc-analysis/DocBank) | Large-scale dataset (500K document pages) constructed using weakly supervised methods for document layout analysis, containing 12 categories:Author, Caption, Date, Equation, Figure, Footer, List, Paragraph, Reference, Section, Table, Title |
+
+
+## 4. Start training
+
+Training scripts, evaluation scripts, and prediction scripts are provided, and the PubLayNet pre-training model is used as an example in this section.
+
+If you do not want training and directly experience the following process of model evaluation, prediction, motion to static, and inference, you can download the provided pre-trained model (PubLayNet dataset) and skip this part.
+
+```
+mkdir pretrained_model
+cd pretrained_model
+# Download PubLayNet pre-training model(Direct experience model evaluates, predicts, and turns static)
+wget https://paddleocr.bj.bcebos.com/ppstructure/models/layout/picodet_lcnet_x1_0_fgd_layout.pdparams
+# Download the PubLaynet inference model(Direct experience model reasoning)
+wget https://paddleocr.bj.bcebos.com/ppstructure/models/layout/picodet_lcnet_x1_0_fgd_layout_infer.tar
+```
+
+If the test image is Chinese, the pre-trained model of Chinese CDLA dataset can be downloaded to identify 10 types of document regions:Table, Figure, Figure caption, Table, Table caption, Header, Footer, Reference, Equation,Download the training model and inference model of Model 'picodet_lcnet_x1_0_fgd_layout_cdla' in [layout analysis model](https://github.com/PaddlePaddle/PaddleOCR/blob/dygraph/ppstructure/docs/models_list.md)。If only the table area in the image is detected, you can download the pre-trained model of the table dataset, and download the training model and inference model of the 'picodet_LCnet_x1_0_FGd_layout_table' model in [Layout Analysis model](https://github.com/PaddlePaddle/PaddleOCR/blob/dygraph/ppstructure/docs/models_list.md)
+
+### 4.1. Train
+
+Train:
+
+* Modify Profile
+
+If you want to train your own data set, you need to modify the data configuration and the number of categories in the configuration file.
+
+
+Using 'configs/picodet/legacy_model/application/layout_analysis/picodet_lcnet_x1_0_layout.yml' as an example, the change is as follows:
+
+```yaml
+metric: COCO
+# Number of categories
+num_classes: 5
+
+TrainDataset:
+ !COCODataSet
+ # Modify to your own training data directory
+ image_dir: train
+ # Modify to your own training data label file
+ anno_path: train.json
+ # Modify to your own training data root directory
+ dataset_dir: /root/publaynet/
+ data_fields: ['image', 'gt_bbox', 'gt_class', 'is_crowd']
+
+EvalDataset:
+ !COCODataSet
+ # Modify to your own validation data directory
+ image_dir: val
+ # Modify to your own validation data label file
+ anno_path: val.json
+ # Modify to your own validation data root
+ dataset_dir: /root/publaynet/
+
+TestDataset:
+ !ImageFolder
+ # Modify to your own test data label file
+ anno_path: /root/publaynet/val.json
+```
+
+* Start training. During training, PP picodet pre training model will be downloaded by default. There is no need to download in advance.
+
+```bash
+# GPU training supports single-card and multi-card training
+# The training log is automatically saved to the log directory
+
+# Single card training
+export CUDA_VISIBLE_DEVICES=0
+python3 tools/train.py \
+ -c configs/picodet/legacy_model/application/layout_analysis/picodet_lcnet_x1_0_layout.yml \
+ --eval
+
+# Multi-card training, with the -- GPUS parameter specifying the card number
+export CUDA_VISIBLE_DEVICES=0,1,2,3
+python3 -m paddle.distributed.launch --gpus '0,1,2,3' tools/train.py \
+ -c configs/picodet/legacy_model/application/layout_analysis/picodet_lcnet_x1_0_layout.yml \
+ --eval
+```
+
+**Attention:**If the video memory is out during training, adjust Batch_size in TrainReader and base_LR in LearningRate. The published config is obtained by 8-card training. If the number of GPU cards is changed to 1, then the base_LR needs to be reduced by 8 times.
+
+After starting training normally, you will see the following log output:
+
+```
+[08/15 04:02:30] ppdet.utils.checkpoint INFO: Finish loading model weights: /root/.cache/paddle/weights/LCNet_x1_0_pretrained.pdparams
+[08/15 04:02:46] ppdet.engine INFO: Epoch: [0] [ 0/1929] learning_rate: 0.040000 loss_vfl: 1.216707 loss_bbox: 1.142163 loss_dfl: 0.544196 loss: 2.903065 eta: 17 days, 13:50:26 batch_cost: 15.7452 data_cost: 2.9112 ips: 1.5243 images/s
+[08/15 04:03:19] ppdet.engine INFO: Epoch: [0] [ 20/1929] learning_rate: 0.064000 loss_vfl: 1.180627 loss_bbox: 0.939552 loss_dfl: 0.442436 loss: 2.628206 eta: 2 days, 12:18:53 batch_cost: 1.5770 data_cost: 0.0008 ips: 15.2184 images/s
+[08/15 04:03:47] ppdet.engine INFO: Epoch: [0] [ 40/1929] learning_rate: 0.088000 loss_vfl: 0.543321 loss_bbox: 1.071401 loss_dfl: 0.457817 loss: 2.057003 eta: 2 days, 0:07:03 batch_cost: 1.3190 data_cost: 0.0007 ips: 18.1954 images/s
+[08/15 04:04:12] ppdet.engine INFO: Epoch: [0] [ 60/1929] learning_rate: 0.112000 loss_vfl: 0.630989 loss_bbox: 0.859183 loss_dfl: 0.384702 loss: 1.883143 eta: 1 day, 19:01:29 batch_cost: 1.2177 data_cost: 0.0006 ips: 19.7087 images/s
+```
+
+- `--eval` indicates that the best model is saved as `output/picodet_lcnet_x1_0_layout/best_accuracy` by default during the evaluation process 。
+
+**Note that the configuration file for prediction / evaluation must be consistent with the training.**
+
+### 4.2. FGD Distillation Training
+
+PaddleDetection supports FGD-based [Focal and Global Knowledge Distillation for Detectors]( https://arxiv.org/abs/2111.11837v1) The training process of the target detection model of distillation, FGD distillation is divided into two parts `Focal` and `Global`. `Focal` Distillation separates the foreground and background of the image, allowing the student model to focus on the key pixels of the foreground and background features of the teacher model respectively;` Global`Distillation section reconstructs the relationships between different pixels and transfers them from the teacher to the student to compensate for the global information lost in `Focal`Distillation.
+
+Change the dataset and modify the data configuration and number of categories in the [TODO] configuration, referring to 4.1. Start training:
+
+```bash
+# Single Card Training
+export CUDA_VISIBLE_DEVICES=0
+python3 tools/train.py \
+ -c configs/picodet/legacy_model/application/layout_analysis/picodet_lcnet_x1_0_layout.yml \
+ --slim_config configs/picodet/legacy_model/application/layout_analysis/picodet_lcnet_x2_5_layout.yml \
+ --eval
+```
+
+- `-c`: Specify the model configuration file.
+- `--slim_config`: Specify the compression policy profile.
+
+## 5. Model evaluation and prediction
+
+### 5.1. Indicator evaluation
+
+ Model parameters in training are saved by default in `output/picodet_ Lcnet_ X1_ 0_ Under the layout` directory. When evaluating indicators, you need to set `weights` to point to the saved parameter file.Assessment datasets can be accessed via `configs/picodet/legacy_ Model/application/layout_ Analysis/picodet_ Lcnet_ X1_ 0_ Layout. Yml` . Modify `EvalDataset` : `img_dir`,`anno_ Path`and`dataset_dir` setting.
+
+```bash
+# GPU evaluation, weights as weights to be measured
+python3 tools/eval.py \
+ -c configs/picodet/legacy_model/application/layout_analysis/picodet_lcnet_x1_0_layout.yml \
+ -o weights=./output/picodet_lcnet_x1_0_layout/best_model
+```
+
+The following information will be printed out, such as mAP, AP0.5, etc.
+
+```py
+ Average Precision (AP) @[ IoU=0.50:0.95 | area= all | maxDets=100 ] = 0.935
+ Average Precision (AP) @[ IoU=0.50 | area= all | maxDets=100 ] = 0.979
+ Average Precision (AP) @[ IoU=0.75 | area= all | maxDets=100 ] = 0.956
+ Average Precision (AP) @[ IoU=0.50:0.95 | area= small | maxDets=100 ] = 0.404
+ Average Precision (AP) @[ IoU=0.50:0.95 | area=medium | maxDets=100 ] = 0.782
+ Average Precision (AP) @[ IoU=0.50:0.95 | area= large | maxDets=100 ] = 0.969
+ Average Recall (AR) @[ IoU=0.50:0.95 | area= all | maxDets= 1 ] = 0.539
+ Average Recall (AR) @[ IoU=0.50:0.95 | area= all | maxDets= 10 ] = 0.938
+ Average Recall (AR) @[ IoU=0.50:0.95 | area= all | maxDets=100 ] = 0.949
+ Average Recall (AR) @[ IoU=0.50:0.95 | area= small | maxDets=100 ] = 0.495
+ Average Recall (AR) @[ IoU=0.50:0.95 | area=medium | maxDets=100 ] = 0.818
+ Average Recall (AR) @[ IoU=0.50:0.95 | area= large | maxDets=100 ] = 0.978
+[08/15 07:07:09] ppdet.engine INFO: Total sample number: 11245, averge FPS: 24.405059207157436
+[08/15 07:07:09] ppdet.engine INFO: Best test bbox ap is 0.935.
+```
+
+If you use the provided pre-training model for evaluation or the FGD distillation training model, replace the `weights` model path and execute the following command for evaluation:
+
+```
+python3 tools/eval.py \
+ -c configs/picodet/legacy_model/application/layout_analysis/picodet_lcnet_x1_0_layout.yml \
+ --slim_config configs/picodet/legacy_model/application/layout_analysis/picodet_lcnet_x2_5_layout.yml \
+ -o weights=output/picodet_lcnet_x2_5_layout/best_model
+```
+
+- `-c`: Specify the model configuration file.
+- `--slim_config`: Specify the distillation policy profile.
+- `-o weights`: Specify the model path trained by the distillation algorithm.
+
+### 5.2. Test Layout Analysis Results
+
+
+The profile predicted to be used must be consistent with the training, for example, if you pass `python3 tools/train'. Py-c configs/picodet/legacy_ Model/application/layout_ Analysis/picodet_ Lcnet_ X1_ 0_ Layout. Yml` completed the training process for the model.
+
+With trained PaddleDetection model, you can use the following commands to make model predictions.
+
+```bash
+python3 tools/infer.py \
+ -c configs/picodet/legacy_model/application/layout_analysis/picodet_lcnet_x1_0_layout.yml \
+ -o weights='output/picodet_lcnet_x1_0_layout/best_model.pdparams' \
+ --infer_img='docs/images/layout.jpg' \
+ --output_dir=output_dir/ \
+ --draw_threshold=0.5
+```
+
+- `--infer_img`: Reasoning for a single picture can also be done via `--infer_ Dir`Inform all pictures in the file.
+- `--output_dir`: Specify the path to save the visualization results.
+- `--draw_threshold`:Specify the NMS threshold for drawing the result box.
+
+If you use the provided pre-training model for prediction or the FGD distillation training model, change the `weights` model path and execute the following command to make the prediction:
+
+```
+python3 tools/infer.py \
+ -c configs/picodet/legacy_model/application/layout_analysis/picodet_lcnet_x1_0_layout.yml \
+ --slim_config configs/picodet/legacy_model/application/layout_analysis/picodet_lcnet_x2_5_layout.yml \
+ -o weights='output/picodet_lcnet_x2_5_layout/best_model.pdparams' \
+ --infer_img='docs/images/layout.jpg' \
+ --output_dir=output_dir/ \
+ --draw_threshold=0.5
+```
+
+
+## 6. Model Export and Inference
+
+
+### 6.1 Model Export
+
+The inference model (the model saved by `paddle.jit.save`) is generally a solidified model saved after the model training is completed, and is mostly used to give prediction in deployment.
+
+The model saved during the training process is the checkpoints model, which saves the parameters of the model and is mostly used to resume training.
+
+Compared with the checkpoints model, the inference model will additionally save the structural information of the model. Therefore, it is easier to deploy because the model structure and model parameters are already solidified in the inference model file, and is suitable for integration with actual systems.
+
+Layout analysis model to inference model steps are as follows:
+
+```bash
+python3 tools/export_model.py \
+ -c configs/picodet/legacy_model/application/layout_analysis/picodet_lcnet_x1_0_layout.yml \
+ -o weights=output/picodet_lcnet_x1_0_layout/best_model \
+ --output_dir=output_inference/
+```
+
+* If no post-export processing is required, specify:`-o export.benchmark=True`(If -o already exists, delete -o here)
+* If you do not need to export NMS, specify:`-o export.nms=False`
+
+After successful conversion, there are three files in the directory:
+
+```
+output_inference/picodet_lcnet_x1_0_layout/
+ ├── model.pdiparams # inference Parameter file for model
+ ├── model.pdiparams.info # inference Model parameter information, ignorable
+ └── model.pdmodel # inference Model Structure File for Model
+```
+
+If you change the `weights` model path using the provided pre-training model to the Inference model, or using the FGD distillation training model, the model to inference model steps are as follows:
+
+```bash
+python3 tools/export_model.py \
+ -c configs/picodet/legacy_model/application/layout_analysis/picodet_lcnet_x1_0_layout.yml \
+ --slim_config configs/picodet/legacy_model/application/layout_analysis/picodet_lcnet_x2_5_layout.yml \
+ -o weights=./output/picodet_lcnet_x2_5_layout/best_model \
+ --output_dir=output_inference/
+```
+
+### 6.2 Model inference
+
+Replace model_with the provided inference training model for inference or the FGD distillation training `model_dir`Inference model path, execute the following commands for inference:
+
+```bash
+python3 deploy/python/infer.py \
+ --model_dir=output_inference/picodet_lcnet_x1_0_layout/ \
+ --image_file=docs/images/layout.jpg \
+ --device=CPU
+```
+
+- --device:Specify the GPU or CPU device
+
+When model inference is complete, you will see the following log output:
+
+```
+------------------------------------------
+----------- Model Configuration -----------
+Model Arch: PicoDet
+Transform Order:
+--transform op: Resize
+--transform op: NormalizeImage
+--transform op: Permute
+--transform op: PadStride
+--------------------------------------------
+class_id:0, confidence:0.9921, left_top:[20.18,35.66],right_bottom:[341.58,600.99]
+class_id:0, confidence:0.9914, left_top:[19.77,611.42],right_bottom:[341.48,901.82]
+class_id:0, confidence:0.9904, left_top:[369.36,375.10],right_bottom:[691.29,600.59]
+class_id:0, confidence:0.9835, left_top:[369.60,608.60],right_bottom:[691.38,736.72]
+class_id:0, confidence:0.9830, left_top:[369.58,805.38],right_bottom:[690.97,901.80]
+class_id:0, confidence:0.9716, left_top:[383.68,271.44],right_bottom:[688.93,335.39]
+class_id:0, confidence:0.9452, left_top:[370.82,34.48],right_bottom:[688.10,63.54]
+class_id:1, confidence:0.8712, left_top:[370.84,771.03],right_bottom:[519.30,789.13]
+class_id:3, confidence:0.9856, left_top:[371.28,67.85],right_bottom:[685.73,267.72]
+save result to: output/layout.jpg
+Test iter 0
+------------------ Inference Time Info ----------------------
+total_time(ms): 2196.0, img_num: 1
+average latency time(ms): 2196.00, QPS: 0.455373
+preprocess_time(ms): 2172.50, inference_time(ms): 11.90, postprocess_time(ms): 11.60
+```
+
+- Model:model structure
+- Transform Order:Preprocessing operation
+- class_id, confidence, left_top, right_bottom:Indicates category id, confidence level, upper left coordinate, lower right coordinate, respectively
+- save result to:Save path of visual layout analysis results, default save to ./output folder
+- inference time info:Inference time, where preprocess_time represents the preprocessing time, Inference_time represents the model prediction time, and postprocess_time represents the post-processing time
+
+The result of visualization layout is shown in the following figure
+
+
+

+
+
+
+
+## Citations
+
+```
+@inproceedings{zhong2019publaynet,
+ title={PubLayNet: largest dataset ever for document layout analysis},
+ author={Zhong, Xu and Tang, Jianbin and Yepes, Antonio Jimeno},
+ booktitle={2019 International Conference on Document Analysis and Recognition (ICDAR)},
+ year={2019},
+ volume={},
+ number={},
+ pages={1015-1022},
+ doi={10.1109/ICDAR.2019.00166},
+ ISSN={1520-5363},
+ month={Sep.},
+ organization={IEEE}
+}
+
+@inproceedings{yang2022focal,
+ title={Focal and global knowledge distillation for detectors},
+ author={Yang, Zhendong and Li, Zhe and Jiang, Xiaohu and Gong, Yuan and Yuan, Zehuan and Zhao, Danpei and Yuan, Chun},
+ booktitle={Proceedings of the IEEE/CVF Conference on Computer Vision and Pattern Recognition},
+ pages={4643--4652},
+ year={2022}
+}
+```
diff --git a/ppstructure/layout/README_ch.md b/ppstructure/layout/README_ch.md
index f8d1978e25d7fb17cfd3fcb363b4ce981e19c8dc..49c10c7e7726a35dadbc936e94c9ab5b55628e82 100644
--- a/ppstructure/layout/README_ch.md
+++ b/ppstructure/layout/README_ch.md
@@ -1,3 +1,7 @@
+简体中文 | [English](README.md)
+
+# 版面分析
+
- [1. 简介](#1-简介)
- [2. 安装](#2-安装)
- [2.1 安装PaddlePaddle](#21-安装paddlepaddle)
@@ -15,8 +19,6 @@
- [6.1 模型导出](#61-模型导出)
- [6.2 模型推理](#62-模型推理)
-# 版面分析
-
## 1. 简介
版面分析指的是对图片形式的文档进行区域划分,定位其中的关键区域,如文字、标题、表格、图片等。版面分析算法基于[PaddleDetection](https://github.com/PaddlePaddle/PaddleDetection)的轻量模型PP-PicoDet进行开发。
@@ -37,10 +39,10 @@
python3 -m pip install --upgrade pip
# GPU安装
-python3 -m pip install "paddlepaddle-gpu>=2.2" -i https://mirror.baidu.com/pypi/simple
+python3 -m pip install "paddlepaddle-gpu>=2.3" -i https://mirror.baidu.com/pypi/simple
# CPU安装
-python3 -m pip install "paddlepaddle>=2.2" -i https://mirror.baidu.com/pypi/simple
+python3 -m pip install "paddlepaddle>=2.3" -i https://mirror.baidu.com/pypi/simple
```
更多需求,请参照[安装文档](https://www.paddlepaddle.org.cn/install/quick)中的说明进行操作。
diff --git a/ppstructure/pdf2word/icons/chinese.png b/ppstructure/pdf2word/icons/chinese.png
new file mode 100644
index 0000000000000000000000000000000000000000..328e2fff73bd75188fa888aae45c8cb4ca844f57
Binary files /dev/null and b/ppstructure/pdf2word/icons/chinese.png differ
diff --git a/ppstructure/pdf2word/icons/english.png b/ppstructure/pdf2word/icons/english.png
new file mode 100644
index 0000000000000000000000000000000000000000..536c4a910ae6fc05040f4958477a34aeae891ea0
Binary files /dev/null and b/ppstructure/pdf2word/icons/english.png differ
diff --git a/ppstructure/pdf2word/icons/folder-open.png b/ppstructure/pdf2word/icons/folder-open.png
new file mode 100644
index 0000000000000000000000000000000000000000..ab5f55f5a4819add116113b55f717f7a21aeafdd
Binary files /dev/null and b/ppstructure/pdf2word/icons/folder-open.png differ
diff --git a/ppstructure/pdf2word/icons/folder-plus.png b/ppstructure/pdf2word/icons/folder-plus.png
new file mode 100644
index 0000000000000000000000000000000000000000..01ce6c10b0ed3e3975edbebbc8e886f846fabe8d
Binary files /dev/null and b/ppstructure/pdf2word/icons/folder-plus.png differ
diff --git a/ppstructure/pdf2word/pdf2word.md b/ppstructure/pdf2word/pdf2word.md
new file mode 100644
index 0000000000000000000000000000000000000000..564df4063e101e028afbea5c3acab8946196d31d
--- /dev/null
+++ b/ppstructure/pdf2word/pdf2word.md
@@ -0,0 +1,28 @@
+# PDF2WORD
+
+PDF2WORD是PaddleOCR社区开发者[whjdark](https://github.com/whjdark) 基于PP-Structure智能文档分析模型实现的PDF转换Word应用程序,提供可直接安装的exe,方便windows用户运行
+
+## 1.使用
+
+### 应用程序
+
+1. 下载与安装:针对Windows用户,根据[软件下载]()一节下载软件后,运行 `pdf2word.exe` 。若您下载的是lite版本,安装过程中会在线下载环境依赖、模型等必要资源,安装时间较长,请确保网络畅通。serve版本打包了相关依赖,安装时间较短,可按需下载。
+
+2. 转换:由于PP-Structure根据中英文数据分别进行适配,在转换相应文件时可**根据文档语言进行相应选择**。
+
+### 脚本运行
+
+首次运行需要将切换路径到 `/ppstructure/pdf2word` ,然后运行代码
+
+```
+python pdf2word.py
+```
+
+## 2.软件下载
+
+如需获取已打包程序,可以扫描下方二维码,关注公众号填写问卷后,加入PaddleOCR官方交流群免费获取20G OCR学习大礼包,内含OCR场景应用集合(包含数码管、液晶屏、车牌、高精度SVTR模型等7个垂类模型)、《动手学OCR》电子书、课程回放视频、前沿论文等重磅资料
+
+
+
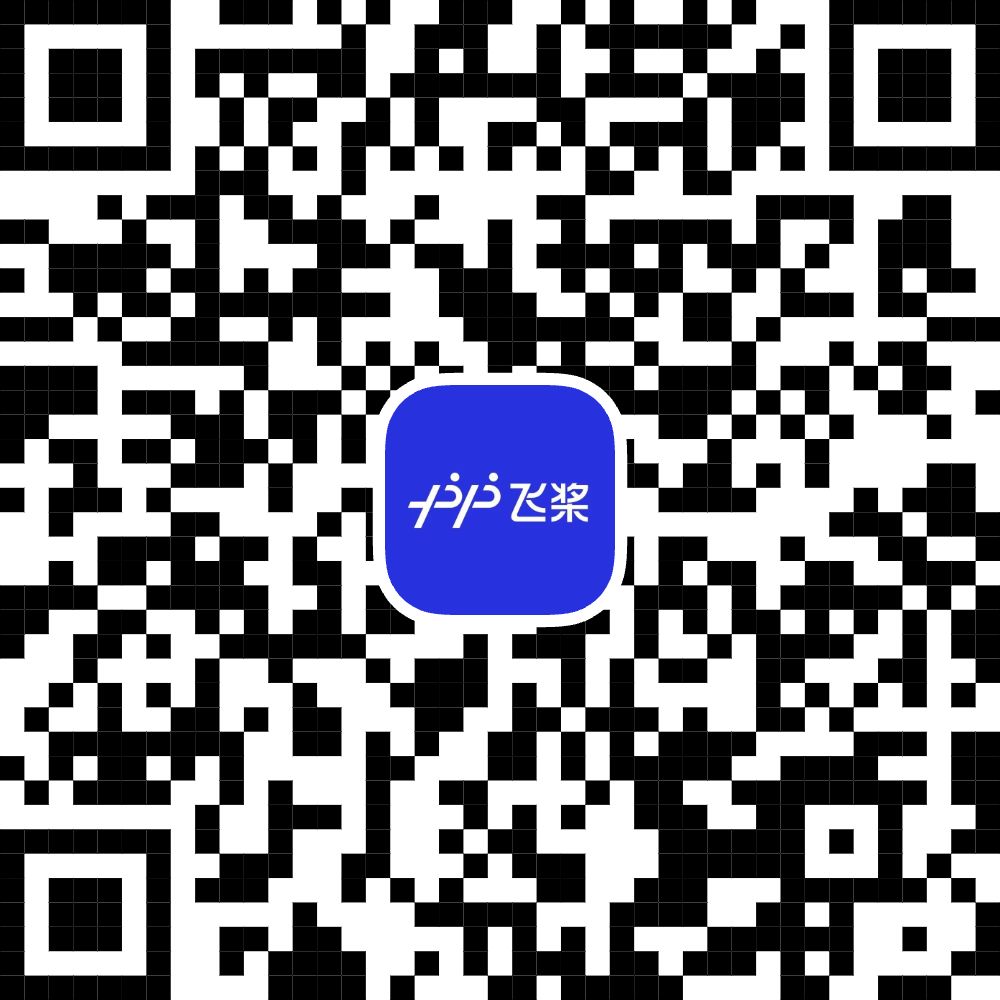
+
+
diff --git a/ppstructure/pdf2word/pdf2word.py b/ppstructure/pdf2word/pdf2word.py
new file mode 100644
index 0000000000000000000000000000000000000000..b05886f62a871fa4f41b81f4292a848e859e2eb7
--- /dev/null
+++ b/ppstructure/pdf2word/pdf2word.py
@@ -0,0 +1,441 @@
+import sys
+import tarfile
+import os
+import time
+import datetime
+import functools
+import cv2
+import platform
+import numpy as np
+from qtpy.QtWidgets import QApplication, QWidget, QPushButton, QProgressBar, \
+ QGridLayout, QMessageBox, QLabel, QFileDialog
+from qtpy.QtCore import Signal, QThread, QObject
+from qtpy.QtGui import QImage, QPixmap, QIcon
+
+file = os.path.dirname(os.path.abspath(__file__))
+root = os.path.abspath(os.path.join(file, '../../'))
+sys.path.append(file)
+sys.path.insert(0, root)
+
+from ppstructure.predict_system import StructureSystem, save_structure_res
+from ppstructure.utility import parse_args, draw_structure_result
+from ppocr.utils.network import download_with_progressbar
+from ppstructure.recovery.recovery_to_doc import sorted_layout_boxes, convert_info_docx
+# from ScreenShotWidget import ScreenShotWidget
+
+__APPNAME__ = "pdf2word"
+__VERSION__ = "0.1.1"
+
+URLs_EN = {
+ # 下载超英文轻量级PP-OCRv3模型的检测模型并解压
+ "en_PP-OCRv3_det_infer": "https://paddleocr.bj.bcebos.com/PP-OCRv3/english/en_PP-OCRv3_det_infer.tar",
+ # 下载英文轻量级PP-OCRv3模型的识别模型并解压
+ "en_PP-OCRv3_rec_infer": "https://paddleocr.bj.bcebos.com/PP-OCRv3/english/en_PP-OCRv3_rec_infer.tar",
+ # 下载超轻量级英文表格英文模型并解压
+ "en_ppstructure_mobile_v2.0_SLANet_infer": "https://paddleocr.bj.bcebos.com/ppstructure/models/slanet/en_ppstructure_mobile_v2.0_SLANet_infer.tar",
+ # 英文版面分析模型
+ "picodet_lcnet_x1_0_fgd_layout_infer": "https://paddleocr.bj.bcebos.com/ppstructure/models/layout/picodet_lcnet_x1_0_fgd_layout_infer.tar",
+}
+DICT_EN = {
+ "rec_char_dict_path": "en_dict.txt",
+ "layout_dict_path": "layout_publaynet_dict.txt",
+}
+
+URLs_CN = {
+ # 下载超中文轻量级PP-OCRv3模型的检测模型并解压
+ "cn_PP-OCRv3_det_infer": "https://paddleocr.bj.bcebos.com/PP-OCRv3/chinese/ch_PP-OCRv3_det_infer.tar",
+ # 下载中文轻量级PP-OCRv3模型的识别模型并解压
+ "cn_PP-OCRv3_rec_infer": "https://paddleocr.bj.bcebos.com/PP-OCRv3/chinese/ch_PP-OCRv3_rec_infer.tar",
+ # 下载超轻量级英文表格英文模型并解压
+ "cn_ppstructure_mobile_v2.0_SLANet_infer": "https://paddleocr.bj.bcebos.com/ppstructure/models/slanet/en_ppstructure_mobile_v2.0_SLANet_infer.tar",
+ # 中文版面分析模型
+ "picodet_lcnet_x1_0_fgd_layout_cdla_infer": "https://paddleocr.bj.bcebos.com/ppstructure/models/layout/picodet_lcnet_x1_0_fgd_layout_cdla_infer.tar",
+}
+DICT_CN = {
+ "rec_char_dict_path": "ppocr_keys_v1.txt",
+ "layout_dict_path": "layout_cdla_dict.txt",
+}
+
+
+
+def QImageToCvMat(incomingImage) -> np.array:
+ '''
+ Converts a QImage into an opencv MAT format
+ '''
+
+ incomingImage = incomingImage.convertToFormat(QImage.Format.Format_RGBA8888)
+
+ width = incomingImage.width()
+ height = incomingImage.height()
+
+ ptr = incomingImage.bits()
+ ptr.setsize(height * width * 4)
+ arr = np.frombuffer(ptr, np.uint8).reshape((height, width, 4))
+ return arr
+
+
+def readImage(image_file) -> list:
+ if os.path.basename(image_file)[-3:] in ['pdf']:
+ import fitz
+ from PIL import Image
+ imgs = []
+ with fitz.open(image_file) as pdf:
+ for pg in range(0, pdf.pageCount):
+ page = pdf[pg]
+ mat = fitz.Matrix(2, 2)
+ pm = page.getPixmap(matrix=mat, alpha=False)
+
+ # if width or height > 2000 pixels, don't enlarge the image
+ if pm.width > 2000 or pm.height > 2000:
+ pm = page.getPixmap(matrix=fitz.Matrix(1, 1), alpha=False)
+
+ img = Image.frombytes("RGB", [pm.width, pm.height], pm.samples)
+ img = cv2.cvtColor(np.array(img), cv2.COLOR_RGB2BGR)
+ imgs.append(img)
+ else:
+ img = cv2.imread(image_file, cv2.IMREAD_COLOR)
+ if img is not None:
+ imgs = [img]
+
+ return imgs
+
+
+class Worker(QThread):
+ progressBarValue = Signal(int)
+ endsignal = Signal()
+ loopFlag = True
+
+ def __init__(self, predictors, save_pdf, vis_font_path):
+ super(Worker, self).__init__()
+ self.predictors = predictors
+ self.save_pdf = save_pdf
+ self.vis_font_path = vis_font_path
+ self.lang = 'EN'
+ self.imagePaths = []
+ self.outputDir = None
+ self.setStackSize(1024*1024)
+
+ def setImagePath(self, imagePaths):
+ self.imagePaths = imagePaths
+
+ def setLang(self, lang):
+ self.lang = lang
+
+ def setOutputDir(self, outputDir):
+ self.outputDir = outputDir
+
+ def predictAndSave(self, imgs, img_name):
+ all_res = []
+ for index, img in enumerate(imgs):
+ res, time_dict = self.predictors[self.lang](img)
+
+ # save output
+ save_structure_res(res, self.outputDir, img_name)
+ draw_img = draw_structure_result(img, res, self.vis_font_path)
+ img_save_path = os.path.join(self.outputDir, img_name, 'show_{}.jpg'.format(index))
+ if res != []:
+ cv2.imwrite(img_save_path, draw_img)
+
+ # recovery
+ h, w, _ = img.shape
+ res = sorted_layout_boxes(res, w)
+ all_res += res
+
+ try:
+ convert_info_docx(img, all_res, self.outputDir, img_name, self.save_pdf)
+ except Exception as ex:
+ print(self,
+ "error in layout recovery image:{}, err msg: {}".format(
+ img_name, ex))
+
+ print('result save to {}'.format(self.outputDir))
+
+ def run(self):
+ try:
+ findex = 0
+ os.makedirs(self.outputDir, exist_ok=True)
+ for i, image_file in enumerate(self.imagePaths):
+ if self.loopFlag == True:
+ imgs = readImage(image_file)
+ if len(imgs) == 0:
+ continue
+ img_name = os.path.basename(image_file).split('.')[0]
+ os.makedirs(os.path.join(self.outputDir, img_name), exist_ok=True)
+ self.predictAndSave(imgs, img_name)
+ findex += 1
+ self.progressBarValue.emit(findex)
+ else:
+ break
+ self.endsignal.emit()
+ self.exec()
+ except Exception as e:
+ print(e)
+ raise
+
+
+class APP_Image2Doc(QWidget):
+ def __init__(self):
+ super().__init__()
+ self.setFixedHeight(90)
+ self.setFixedWidth(400)
+
+ # settings
+ self.imagePaths = []
+# self.screenShotWg = ScreenShotWidget()
+ self.screenShot = None
+ self.save_pdf = False
+ self.output_dir = None
+ self.vis_font_path = os.path.join(root,
+ "doc", "fonts", "simfang.ttf")
+
+ # ProgressBar
+ self.pb = QProgressBar()
+ self.pb.setRange(0, 100)
+ self.pb.setValue(0)
+
+ # 初始化界面
+ self.setupUi()
+
+ # 下载模型
+ self.downloadModels(URLs_EN)
+ self.downloadModels(URLs_CN)
+
+ # 初始化模型
+ predictors = {
+ 'EN': self.initPredictor('EN'),
+ 'CN': self.initPredictor('CN'),
+ }
+
+ # 设置工作进程
+ self._thread = Worker(predictors, self.save_pdf, self.vis_font_path)
+ self._thread.progressBarValue.connect(self.handleProgressBarSingal)
+ self._thread.endsignal.connect(self.handleEndsignalSignal)
+ self._thread.finished.connect(QObject.deleteLater)
+ self.time_start = 0 # save start time
+
+ def setupUi(self):
+ self.setObjectName("MainWindow")
+ self.setWindowTitle(__APPNAME__ + " " + __VERSION__)
+
+ layout = QGridLayout()
+
+ self.openFileButton = QPushButton("打开文件")
+ self.openFileButton.setIcon(QIcon(QPixmap("./icons/folder-plus.png")))
+ layout.addWidget(self.openFileButton, 0, 0, 1, 1)
+ self.openFileButton.clicked.connect(self.handleOpenFileSignal)
+
+ # screenShotButton = QPushButton("截图识别")
+ # layout.addWidget(screenShotButton, 0, 1, 1, 1)
+ # screenShotButton.clicked.connect(self.screenShotSlot)
+ # screenShotButton.setEnabled(False) # temporarily disenble
+
+ self.startCNButton = QPushButton("中文转换")
+ self.startCNButton.setIcon(QIcon(QPixmap("./icons/chinese.png")))
+ layout.addWidget(self.startCNButton, 0, 1, 1, 1)
+ self.startCNButton.clicked.connect(
+ functools.partial(self.handleStartSignal, 'CN'))
+
+ self.startENButton = QPushButton("英文转换")
+ self.startENButton.setIcon(QIcon(QPixmap("./icons/english.png")))
+ layout.addWidget(self.startENButton, 0, 2, 1, 1)
+ self.startENButton.clicked.connect(
+ functools.partial(self.handleStartSignal, 'EN'))
+
+ self.showResultButton = QPushButton("显示结果")
+ self.showResultButton.setIcon(QIcon(QPixmap("./icons/folder-open.png")))
+ layout.addWidget(self.showResultButton, 0, 3, 1, 1)
+ self.showResultButton.clicked.connect(self.handleShowResultSignal)
+
+ # ProgressBar
+ layout.addWidget(self.pb, 2, 0, 1, 4)
+ # time estimate label
+ self.timeEstLabel = QLabel(
+ ("Time Left: --"))
+ layout.addWidget(self.timeEstLabel, 3, 0, 1, 4)
+
+ self.setLayout(layout)
+
+ def downloadModels(self, URLs):
+ # using custom model
+ tar_file_name_list = [
+ 'inference.pdiparams',
+ 'inference.pdiparams.info',
+ 'inference.pdmodel',
+ 'model.pdiparams',
+ 'model.pdiparams.info',
+ 'model.pdmodel'
+ ]
+ model_path = os.path.join(root, 'inference')
+ os.makedirs(model_path, exist_ok=True)
+
+ # download and unzip models
+ for name in URLs.keys():
+ url = URLs[name]
+ print("Try downloading file: {}".format(url))
+ tarname = url.split('/')[-1]
+ tarpath = os.path.join(model_path, tarname)
+ if os.path.exists(tarpath):
+ print("File have already exist. skip")
+ else:
+ try:
+ download_with_progressbar(url, tarpath)
+ except Exception as e:
+ print("Error occurred when downloading file, error message:")
+ print(e)
+
+ # unzip model tar
+ try:
+ with tarfile.open(tarpath, 'r') as tarObj:
+ storage_dir = os.path.join(model_path, name)
+ os.makedirs(storage_dir, exist_ok=True)
+ for member in tarObj.getmembers():
+ filename = None
+ for tar_file_name in tar_file_name_list:
+ if tar_file_name in member.name:
+ filename = tar_file_name
+ if filename is None:
+ continue
+ file = tarObj.extractfile(member)
+ with open(
+ os.path.join(storage_dir, filename),
+ 'wb') as f:
+ f.write(file.read())
+ except Exception as e:
+ print("Error occurred when unziping file, error message:")
+ print(e)
+
+ def initPredictor(self, lang='EN'):
+ # init predictor args
+ args = parse_args()
+ args.table_max_len = 488
+ args.ocr = True
+ args.recovery = True
+ args.save_pdf = self.save_pdf
+ args.table_char_dict_path = os.path.join(root,
+ "ppocr", "utils", "dict", "table_structure_dict.txt")
+ if lang == 'EN':
+ args.det_model_dir = os.path.join(root, # 此处从这里找到模型存放位置
+ "inference", "en_PP-OCRv3_det_infer")
+ args.rec_model_dir = os.path.join(root,
+ "inference", "en_PP-OCRv3_rec_infer")
+ args.table_model_dir = os.path.join(root,
+ "inference", "en_ppstructure_mobile_v2.0_SLANet_infer")
+ args.output = os.path.join(root, "output") # 结果保存路径
+ args.layout_model_dir = os.path.join(root,
+ "inference", "picodet_lcnet_x1_0_fgd_layout_infer")
+ lang_dict = DICT_EN
+ elif lang == 'CN':
+ args.det_model_dir = os.path.join(root, # 此处从这里找到模型存放位置
+ "inference", "cn_PP-OCRv3_det_infer")
+ args.rec_model_dir = os.path.join(root,
+ "inference", "cn_PP-OCRv3_rec_infer")
+ args.table_model_dir = os.path.join(root,
+ "inference", "cn_ppstructure_mobile_v2.0_SLANet_infer")
+ args.output = os.path.join(root, "output") # 结果保存路径
+ args.layout_model_dir = os.path.join(root,
+ "inference", "picodet_lcnet_x1_0_fgd_layout_cdla_infer")
+ lang_dict = DICT_CN
+ else:
+ raise ValueError("Unsupported language")
+ args.rec_char_dict_path = os.path.join(root,
+ "ppocr", "utils",
+ lang_dict['rec_char_dict_path'])
+ args.layout_dict_path = os.path.join(root,
+ "ppocr", "utils", "dict", "layout_dict",
+ lang_dict['layout_dict_path'])
+ # init predictor
+ return StructureSystem(args)
+
+ def handleOpenFileSignal(self):
+ '''
+ 可以多选图像文件
+ '''
+ selectedFiles = QFileDialog.getOpenFileNames(self,
+ "多文件选择", "/", "图片文件 (*.png *.jpeg *.jpg *.bmp *.pdf)")[0]
+ if len(selectedFiles) > 0:
+ self.imagePaths = selectedFiles
+ self.screenShot = None # discard screenshot temp image
+ self.pb.setRange(0, len(self.imagePaths))
+ self.pb.setValue(0)
+
+# def screenShotSlot(self):
+# '''
+# 选定图像文件和截图的转换过程只能同时进行一个
+# 截图只能同时转换一个
+# '''
+# self.screenShotWg.start()
+# if self.screenShotWg.captureImage:
+# self.screenShot = self.screenShotWg.captureImage
+# self.imagePaths.clear() # discard openfile temp list
+# self.pb.setRange(0, 1)
+# self.pb.setValue(0)
+
+ def handleStartSignal(self, lang):
+ if self.screenShot: # for screenShot
+ img_name = 'screenshot_' + time.strftime("%Y%m%d%H%M%S", time.localtime())
+ image = QImageToCvMat(self.screenShot)
+ self.predictAndSave(image, img_name, lang)
+ # update Progress Bar
+ self.pb.setValue(1)
+ QMessageBox.information(self,
+ u'Information', "文档提取完成")
+ elif len(self.imagePaths) > 0 : # for image file selection
+ # Must set image path list and language before start
+ self.output_dir = os.path.join(
+ os.path.dirname(self.imagePaths[0]), "output") # output_dir shold be same as imagepath
+ self._thread.setOutputDir(self.output_dir)
+ self._thread.setImagePath(self.imagePaths)
+ self._thread.setLang(lang)
+ # disenble buttons
+ self.openFileButton.setEnabled(False)
+ self.startCNButton.setEnabled(False)
+ self.startENButton.setEnabled(False)
+ # 启动工作进程
+ self._thread.start()
+ self.time_start = time.time() # log start time
+ QMessageBox.information(self,
+ u'Information', "开始转换")
+ else:
+ QMessageBox.warning(self,
+ u'Information', "请选择要识别的文件或截图")
+
+ def handleShowResultSignal(self):
+ if self.output_dir is None:
+ return
+ if os.path.exists(self.output_dir):
+ if platform.system() == 'Windows':
+ os.startfile(self.output_dir)
+ else:
+ os.system('open ' + os.path.normpath(self.output_dir))
+ else:
+ QMessageBox.information(self,
+ u'Information', "输出文件不存在")
+
+ def handleProgressBarSingal(self, i):
+ self.pb.setValue(i)
+ # calculate time left of recognition
+ lenbar = self.pb.maximum()
+ avg_time = (time.time() - self.time_start) / i # Use average time to prevent time fluctuations
+ time_left = str(datetime.timedelta(seconds=avg_time * (lenbar - i))).split(".")[0] # Remove microseconds
+ self.timeEstLabel.setText(f"Time Left: {time_left}") # show time left
+
+ def handleEndsignalSignal(self):
+ # enble buttons
+ self.openFileButton.setEnabled(True)
+ self.startCNButton.setEnabled(True)
+ self.startENButton.setEnabled(True)
+ QMessageBox.information(self, u'Information', "转换结束")
+
+
+def main():
+ app = QApplication(sys.argv)
+
+ window = APP_Image2Doc() # 创建对象
+ window.show() # 全屏显示窗口
+
+ QApplication.processEvents()
+ sys.exit(app.exec())
+
+
+if __name__ == "__main__":
+ main()
diff --git a/ppstructure/recovery/README.md b/ppstructure/recovery/README.md
index 59aef707dd67799bb46dc18dc58f883c502c8b86..b1eaae46df87499d11f196d02d17d0690ffd0f16 100644
--- a/ppstructure/recovery/README.md
+++ b/ppstructure/recovery/README.md
@@ -66,7 +66,7 @@ git clone https://gitee.com/paddlepaddle/PaddleOCR
- **(2) Install recovery's `requirements`**
-The layout restoration is exported as docx and PDF files, so python-docx and docx2pdf API need to be installed, and fitz and PyMuPDF apis need to be installed to process the input files in pdf format.
+The layout restoration is exported as docx and PDF files, so python-docx and docx2pdf API need to be installed, and PyMuPDF api([requires Python >= 3.7](https://pypi.org/project/PyMuPDF/)) need to be installed to process the input files in pdf format.
```bash
python3 -m pip install -r ppstructure/recovery/requirements.txt
diff --git a/ppstructure/recovery/README_ch.md b/ppstructure/recovery/README_ch.md
index ae3b7ed82464f513af585542ef8e92d66f2c8756..cd99f7f725f4a3d275ab920e9fe0125a74a995e5 100644
--- a/ppstructure/recovery/README_ch.md
+++ b/ppstructure/recovery/README_ch.md
@@ -68,7 +68,7 @@ git clone https://gitee.com/paddlepaddle/PaddleOCR
- **(2)安装recovery的`requirements`**
-版面恢复导出为docx、pdf文件,所以需要安装python-docx、docx2pdf API,同时处理pdf格式的输入文件,需要安装fitz、PyMuPDF API。
+版面恢复导出为docx、pdf文件,所以需要安装python-docx、docx2pdf API,同时处理pdf格式的输入文件,需要安装PyMuPDF API([要求Python >= 3.7](https://pypi.org/project/PyMuPDF/))。
```bash
python3 -m pip install -r ppstructure/recovery/requirements.txt
diff --git a/ppstructure/recovery/requirements.txt b/ppstructure/recovery/requirements.txt
index b118a41e516ec20e5807030649943e5f7d848107..25e8cdbb0d58b0a243b176f563c66717d6f4c112 100644
--- a/ppstructure/recovery/requirements.txt
+++ b/ppstructure/recovery/requirements.txt
@@ -1,5 +1,4 @@
python-docx
docx2pdf
-fitz
-PyMuPDF==1.16.14
+PyMuPDF
beautifulsoup4
\ No newline at end of file