diff --git a/configs/e2e/e2e_r50_vd_pg.yml b/configs/e2e/e2e_r50_vd_pg.yml
index 0a232f7a4f3b9ca214bbc6fd1840cec186c027e4..e4d868f98b5847fa064e14f87a69932806791320 100644
--- a/configs/e2e/e2e_r50_vd_pg.yml
+++ b/configs/e2e/e2e_r50_vd_pg.yml
@@ -59,8 +59,10 @@ Optimizer:
PostProcess:
name: PGPostProcess
score_thresh: 0.5
+ mode: fast # fast or slow two ways
Metric:
name: E2EMetric
+ gt_mat_dir: # the dir of gt_mat
character_dict_path: ppocr/utils/ic15_dict.txt
main_indicator: f_score_e2e
@@ -106,7 +108,7 @@ Eval:
order: 'hwc'
- ToCHWImage:
- KeepKeys:
- keep_keys: [ 'image', 'shape', 'polys', 'strs', 'tags' ]
+ keep_keys: [ 'image', 'shape', 'polys', 'strs', 'tags', 'img_id']
loader:
shuffle: False
drop_last: False
diff --git a/doc/doc_ch/inference.md b/doc/doc_ch/inference.md
index 1288d90692e154220b8ceb22cd7b6d98f53d3efb..f0f7401538a9f8940f671fdcc170aca6c003040d 100755
--- a/doc/doc_ch/inference.md
+++ b/doc/doc_ch/inference.md
@@ -13,7 +13,6 @@ inference 模型(`paddle.jit.save`保存的模型)
- [检测模型转inference模型](#检测模型转inference模型)
- [识别模型转inference模型](#识别模型转inference模型)
- [方向分类模型转inference模型](#方向分类模型转inference模型)
- - [端到端模型转inference模型](#端到端模型转inference模型)
- [二、文本检测模型推理](#文本检测模型推理)
- [1. 超轻量中文检测模型推理](#超轻量中文检测模型推理)
@@ -28,13 +27,10 @@ inference 模型(`paddle.jit.save`保存的模型)
- [4. 自定义文本识别字典的推理](#自定义文本识别字典的推理)
- [5. 多语言模型的推理](#多语言模型的推理)
-- [四、端到端模型推理](#端到端模型推理)
- - [1. PGNet端到端模型推理](#PGNet端到端模型推理)
-
-- [五、方向分类模型推理](#方向识别模型推理)
+- [四、方向分类模型推理](#方向识别模型推理)
- [1. 方向分类模型推理](#方向分类模型推理)
-- [六、文本检测、方向分类和文字识别串联推理](#文本检测、方向分类和文字识别串联推理)
+- [五、文本检测、方向分类和文字识别串联推理](#文本检测、方向分类和文字识别串联推理)
- [1. 超轻量中文OCR模型推理](#超轻量中文OCR模型推理)
- [2. 其他模型推理](#其他模型推理)
@@ -122,32 +118,6 @@ python3 tools/export_model.py -c configs/cls/cls_mv3.yml -o Global.pretrained_mo
├── inference.pdiparams.info # 分类inference模型的参数信息,可忽略
└── inference.pdmodel # 分类inference模型的program文件
```
-
-### 端到端模型转inference模型
-
-下载端到端模型:
-```
-wget -P ./ch_lite/ https://paddleocr.bj.bcebos.com/dygraph_v2.0/ch/ch_ppocr_mobile_v2.0_cls_train.tar && tar xf ./ch_lite/ch_ppocr_mobile_v2.0_cls_train.tar -C ./ch_lite/
-```
-
-端到端模型转inference模型与检测的方式相同,如下:
-```
-# -c 后面设置训练算法的yml配置文件
-# -o 配置可选参数
-# Global.pretrained_model 参数设置待转换的训练模型地址,不用添加文件后缀 .pdmodel,.pdopt或.pdparams。
-# Global.load_static_weights 参数需要设置为 False。
-# Global.save_inference_dir参数设置转换的模型将保存的地址。
-
-python3 tools/export_model.py -c configs/e2e/e2e_r50_vd_pg.yml -o Global.pretrained_model=./ch_lite/ch_ppocr_mobile_v2.0_cls_train/best_accuracy Global.load_static_weights=False Global.save_inference_dir=./inference/e2e/
-```
-
-转换成功后,在目录下有三个文件:
-```
-/inference/e2e/
- ├── inference.pdiparams # 分类inference模型的参数文件
- ├── inference.pdiparams.info # 分类inference模型的参数信息,可忽略
- └── inference.pdmodel # 分类inference模型的program文件
-```
## 二、文本检测模型推理
@@ -362,38 +332,8 @@ python3 tools/infer/predict_rec.py --image_dir="./doc/imgs_words/korean/1.jpg" -
Predicts of ./doc/imgs_words/korean/1.jpg:('바탕으로', 0.9948904)
```
-
-## 四、端到端模型推理
-
-端到端模型推理,默认使用PGNet模型的配置参数。当不使用PGNet模型时,在推理时,需要通过传入相应的参数进行算法适配,细节参考下文。
-
-### 1. PGNet端到端模型推理
-#### (1). 四边形文本检测模型(ICDAR2015)
-首先将PGNet端到端训练过程中保存的模型,转换成inference model。以基于Resnet50_vd骨干网络,在ICDAR2015英文数据集训练的模型为例([模型下载地址](https://paddleocr.bj.bcebos.com/dygraph_v2.0/pgnet/en_server_pgnetA.tar)),可以使用如下命令进行转换:
-```
-python3 tools/export_model.py -c configs/e2e/e2e_r50_vd_pg.yml -o Global.pretrained_model=./en_server_pgnetA/iter_epoch_450 Global.load_static_weights=False Global.save_inference_dir=./inference/e2e
-```
-**PGNet端到端模型推理,需要设置参数`--e2e_algorithm="PGNet"`**,可以执行如下命令:
-```
-python3 tools/infer/predict_e2e.py --e2e_algorithm="PGNet" --image_dir="./doc/imgs_en/img_10.jpg" --e2e_model_dir="./inference/e2e/" --e2e_pgnet_polygon=False
-```
-可视化文本检测结果默认保存到`./inference_results`文件夹里面,结果文件的名称前缀为'e2e_res'。结果示例如下:
-
-
-
-#### (2). 弯曲文本检测模型(Total-Text)
-和四边形文本检测模型共用一个推理模型
-**PGNet端到端模型推理,需要设置参数`--e2e_algorithm="PGNet"`,同时,还需要增加参数`--e2e_pgnet_polygon=True`,**可以执行如下命令:
-```
-python3.7 tools/infer/predict_e2e.py --e2e_algorithm="PGNet" --image_dir="./doc/imgs_en/img623.jpg" --e2e_model_dir="./inference/e2e/" --e2e_pgnet_polygon=True
-```
-可视化文本端到端结果默认保存到`./inference_results`文件夹里面,结果文件的名称前缀为'e2e_res'。结果示例如下:
-
-
-
-
-## 五、方向分类模型推理
+## 四、方向分类模型推理
下面将介绍方向分类模型推理。
@@ -418,7 +358,7 @@ Predicts of ./doc/imgs_words/ch/word_4.jpg:['0', 0.9999982]
```
-## 六、文本检测、方向分类和文字识别串联推理
+## 五、文本检测、方向分类和文字识别串联推理
### 1. 超轻量中文OCR模型推理
diff --git a/doc/doc_ch/multi_languages.md b/doc/doc_ch/multi_languages.md
index a8f7c2b77f64285e0edfbd22c248e84f0bb84d42..eec09535e7242f62cdebda97cee11086e45c1096 100644
--- a/doc/doc_ch/multi_languages.md
+++ b/doc/doc_ch/multi_languages.md
@@ -5,6 +5,25 @@
- 2021.4.9 支持**80种**语言的检测和识别
- 2021.4.9 支持**轻量高精度**英文模型检测识别
+PaddleOCR 旨在打造一套丰富、领先、且实用的OCR工具库,不仅提供了通用场景下的中英文模型,也提供了专门在英文场景下训练的模型,
+和覆盖[80个语言](#语种缩写)的小语种模型。
+
+其中英文模型支持,大小写字母和常见标点的检测识别,并优化了空格字符的识别:
+
+
+

+
+
+小语种模型覆盖了拉丁语系、阿拉伯语系、中文繁体、韩语、日语等等:
+
+
+

+

+
+
+
+本文档将简要介绍小语种模型的使用方法。
+
- [1 安装](#安装)
- [1.1 paddle 安装](#paddle安装)
- [1.2 paddleocr package 安装](#paddleocr_package_安装)
@@ -68,7 +87,11 @@ Paddleocr目前支持80个语种,可以通过修改--lang参数进行切换,
paddleocr --image_dir doc/imgs/japan_2.jpg --lang=japan
```
-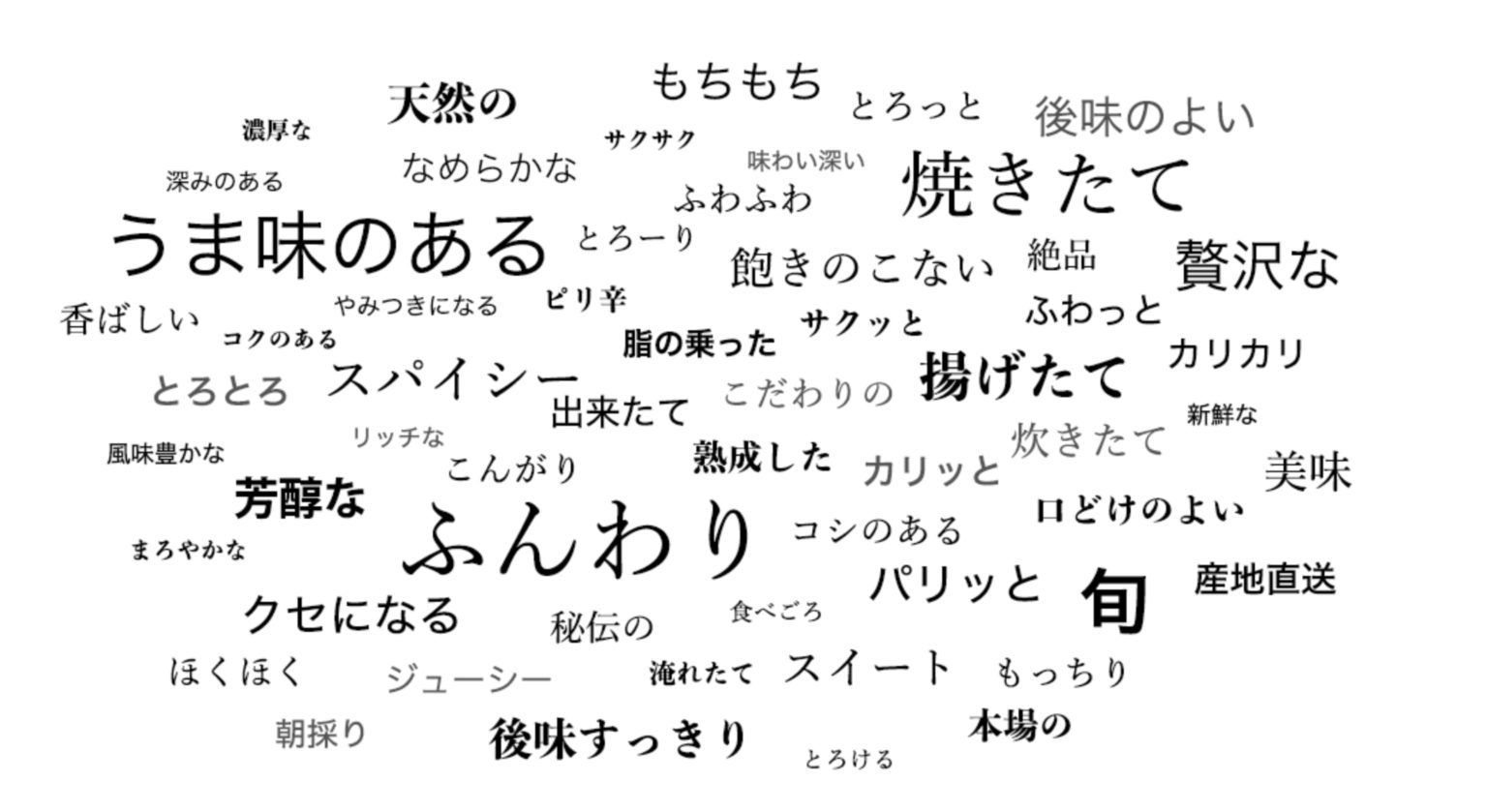
+
+
+
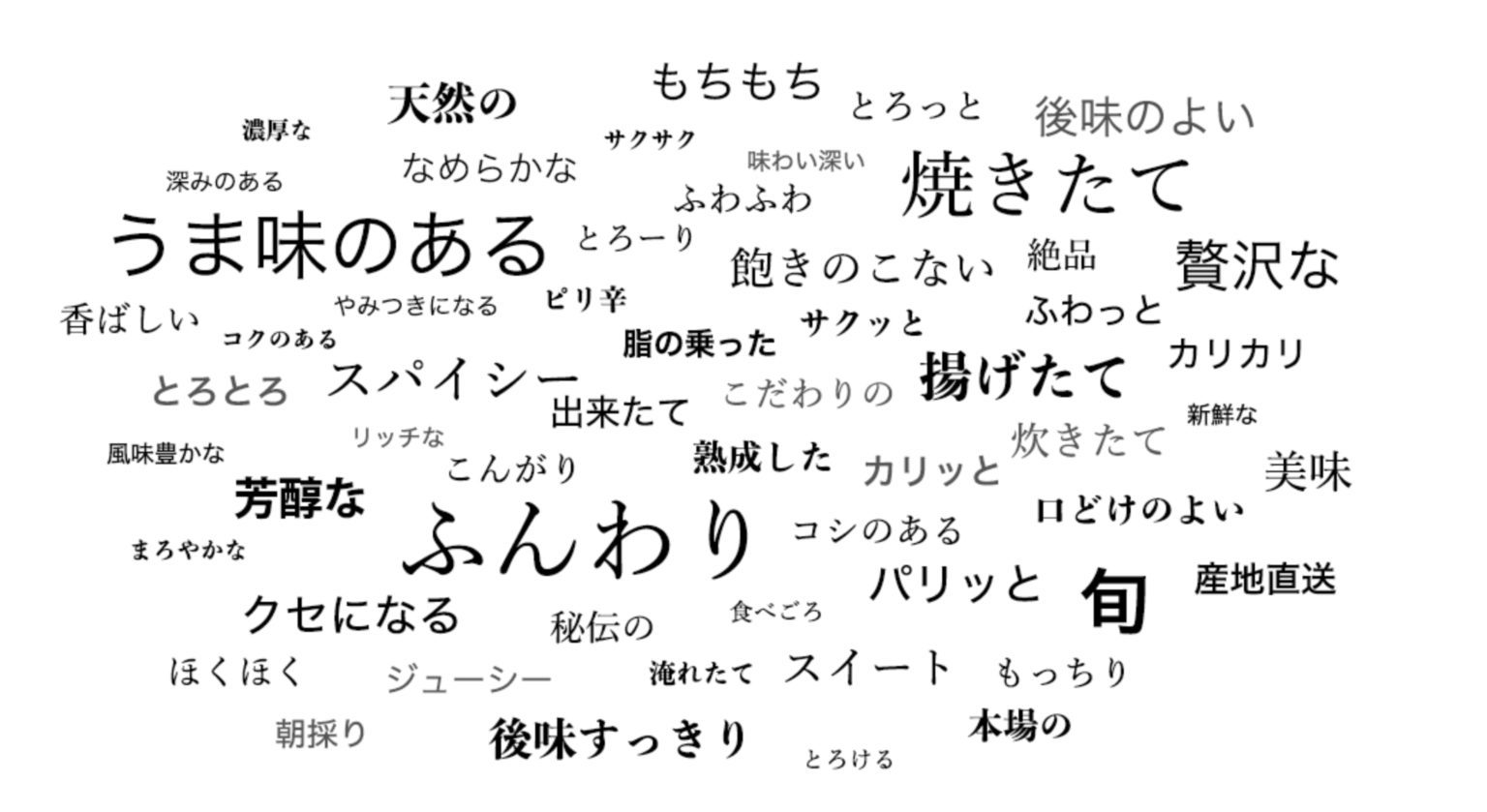
+
+
结果是一个list,每个item包含了文本框,文字和识别置信度
```text
@@ -138,8 +161,10 @@ im_show.save('result.jpg')
```
结果可视化:
-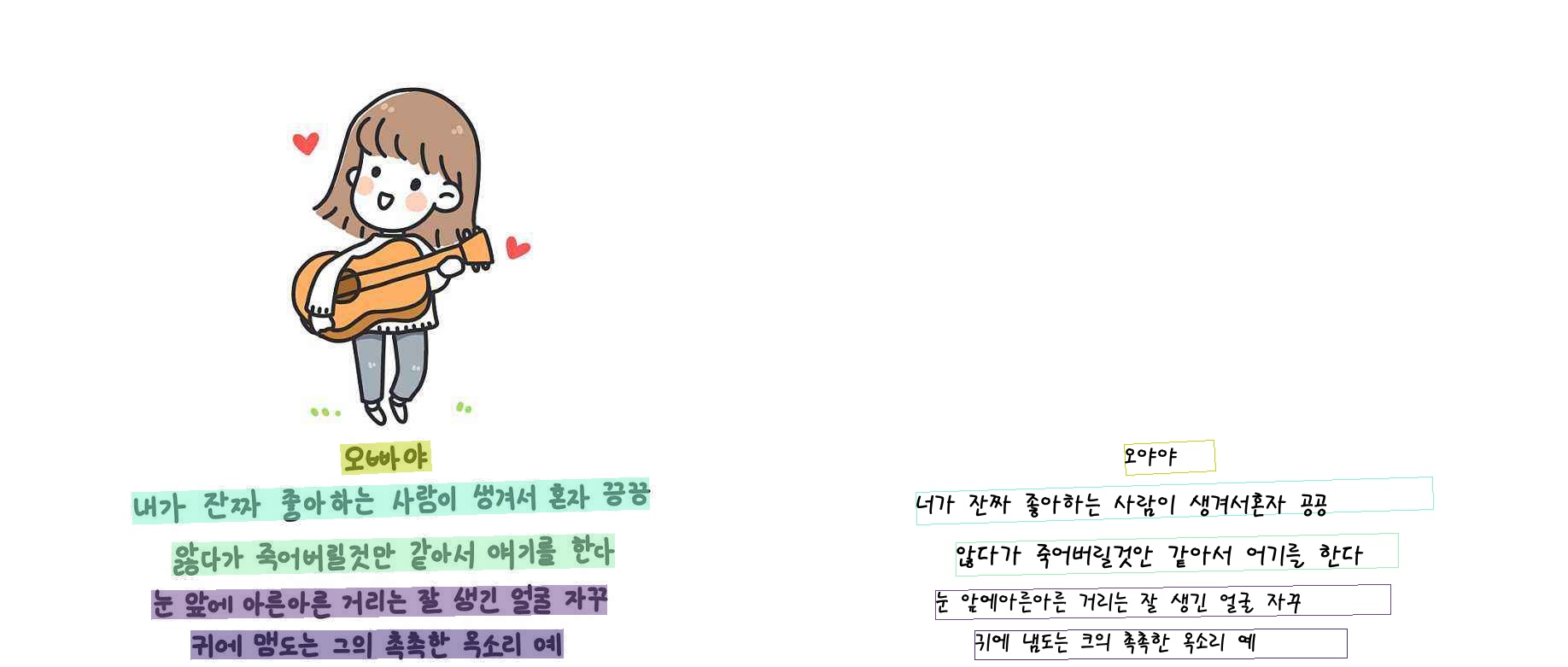
+
+
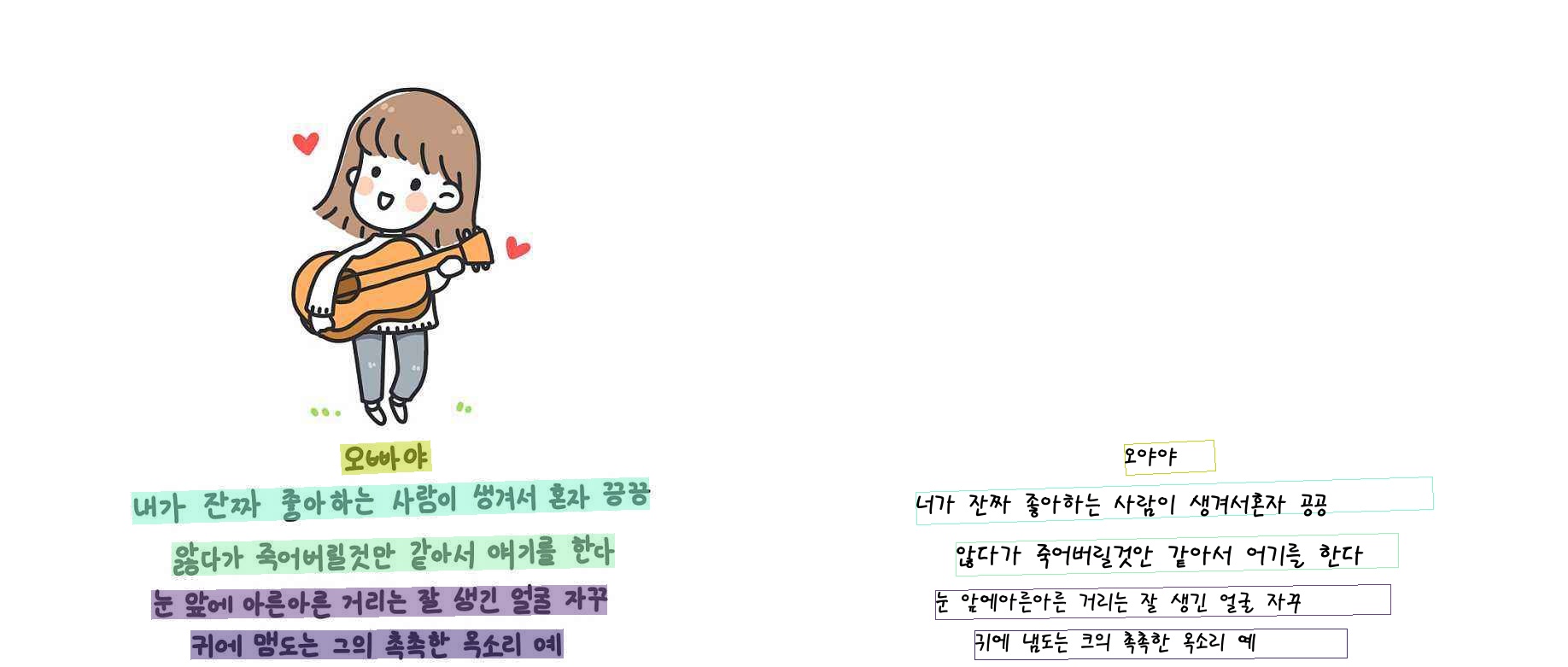
+
* 识别预测
@@ -152,7 +177,8 @@ for line in result:
print(line)
```
-
+
+
结果是一个tuple,只包含识别结果和识别置信度
@@ -187,7 +213,10 @@ im_show.save('result.jpg')
```
结果可视化 :
-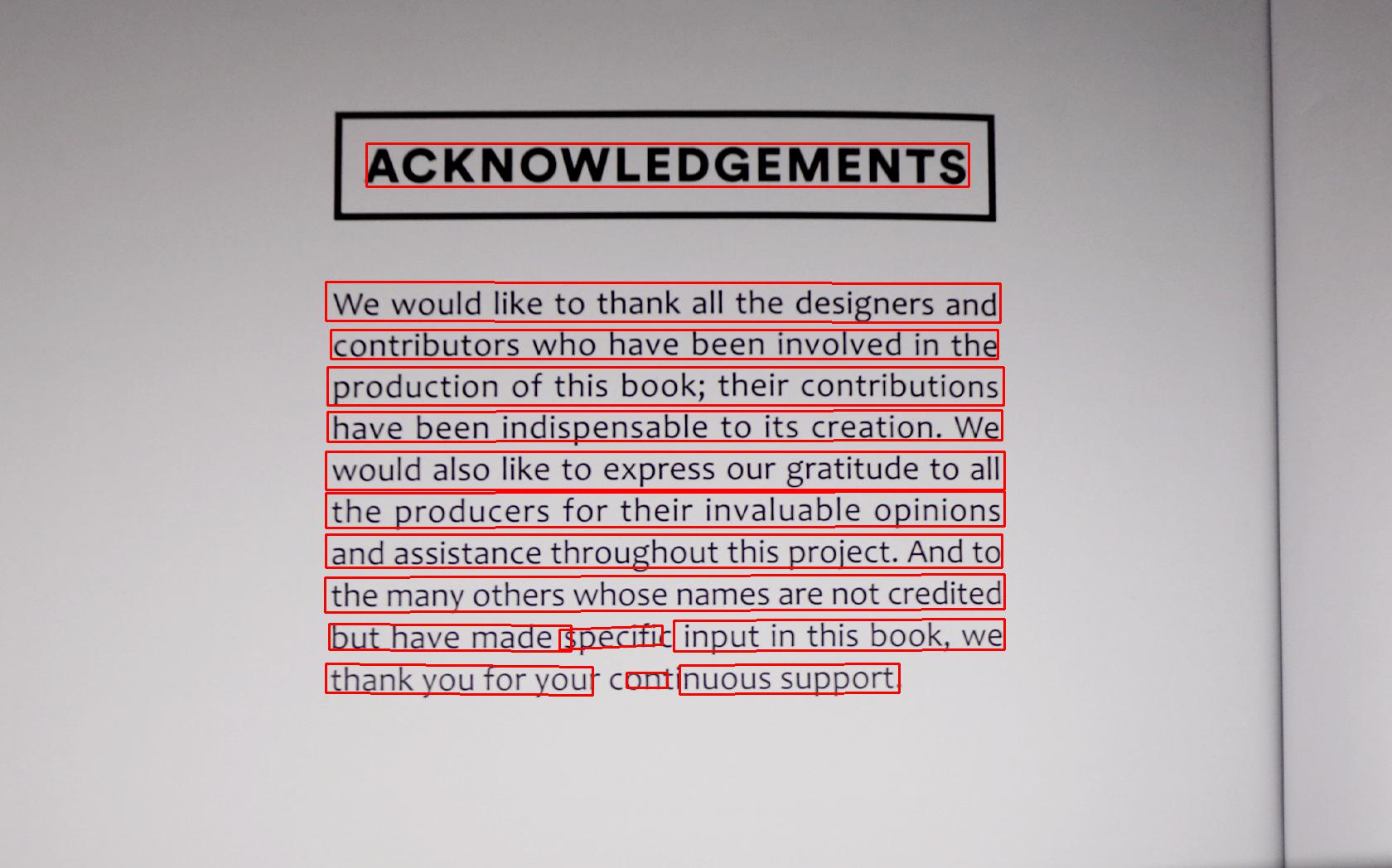
+
+
+
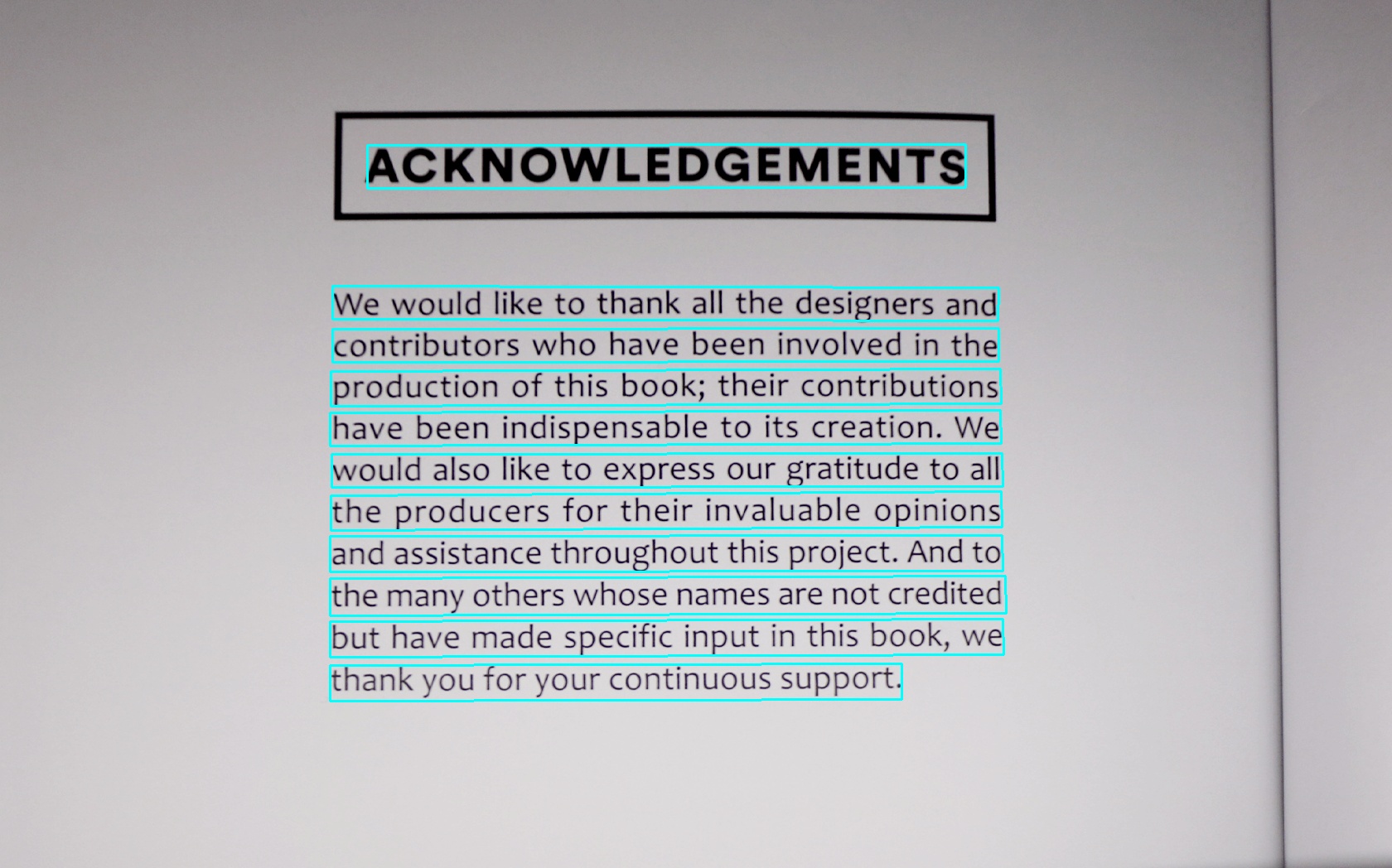
+
ppocr 还支持方向分类, 更多使用方式请参考:[whl包使用说明](https://github.com/PaddlePaddle/PaddleOCR/blob/release/2.0/doc/doc_ch/whl.md)。
@@ -233,7 +262,7 @@ ppocr 支持使用自己的数据进行自定义训练或finetune, 其中识别
|卡纳达文|Kannada |kn|
|泰米尔文|Tamil |ta|
|南非荷兰文 |Afrikaans |af|
-|阿塞拜疆文 |Azerbaijani |az|
+|阿塞拜疆文 |Azerbaijani |az|
|波斯尼亚文|Bosnian|bs|
|捷克文|Czech|cs|
|威尔士文 |Welsh |cy|
diff --git a/doc/doc_ch/pgnet.md b/doc/doc_ch/pgnet.md
index 4d3b8208777873dc7c0cdb87346eb950d3e3e2f4..265853860854317ab00f40b1f447edfad47dc557 100644
--- a/doc/doc_ch/pgnet.md
+++ b/doc/doc_ch/pgnet.md
@@ -2,7 +2,7 @@
- [一、简介](#简介)
- [二、环境配置](#环境配置)
- [三、快速使用](#快速使用)
-- [四、模型训练、评估、推理](#快速训练)
+- [四、模型训练、评估、推理](#模型训练、评估、推理)
## 一、简介
@@ -16,14 +16,31 @@ OCR算法可以分为两阶段算法和端对端的算法。二阶段OCR算法
- 提出基于图的修正模块(GRM)来进一步提高模型识别性能
- 精度更高,预测速度更快
-PGNet算法细节详见[论文](https://www.aaai.org/AAAI21Papers/AAAI-2885.WangP.pdf), 算法原理图如下所示:
+PGNet算法细节详见[论文](https://www.aaai.org/AAAI21Papers/AAAI-2885.WangP.pdf) ,算法原理图如下所示:

输入图像经过特征提取送入四个分支,分别是:文本边缘偏移量预测TBO模块,文本中心线预测TCL模块,文本方向偏移量预测TDO模块,以及文本字符分类图预测TCC模块。
其中TBO以及TCL的输出经过后处理后可以得到文本的检测结果,TCL、TDO、TCC负责文本识别。
+
其检测识别效果图如下:
+


+### 性能指标
+
+测试集: Total Text
+
+测试环境: NVIDIA Tesla V100-SXM2-16GB
+
+|PGNetA|det_precision|det_recall|det_f_score|e2e_precision|e2e_recall|e2e_f_score|FPS|下载|
+| --- | --- | --- | --- | --- | --- | --- | --- | --- |
+|Paper|85.30|86.80|86.1|-|-|61.7|38.20 (size=640)|-|
+|Ours|87.03|82.48|84.69|61.71|58.43|60.03|48.73 (size=768)|[下载链接](https://paddleocr.bj.bcebos.com/dygraph_v2.0/pgnet/en_server_pgnetA.tar)|
+
+*note:PaddleOCR里的PGNet实现针对预测速度做了优化,在精度下降可接受范围内,可以显著提升端对端预测速度*
+
+
+
## 二、环境配置
请先参考[快速安装](./installation.md)配置PaddleOCR运行环境。
@@ -49,24 +66,24 @@ wget https://paddleocr.bj.bcebos.com/dygraph_v2.0/pgnet/e2e_server_pgnetA_infer.
### 单张图像或者图像集合预测
```bash
# 预测image_dir指定的单张图像
-python3 tools/infer/predict_e2e.py --e2e_algorithm="PGNet" --image_dir="./doc/imgs_en/img623.jpg" --e2e_model_dir="./inference/e2e/" --e2e_pgnet_polygon=True
+python3 tools/infer/predict_e2e.py --e2e_algorithm="PGNet" --image_dir="./doc/imgs_en/img623.jpg" --e2e_model_dir="./inference/e2e_server_pgnetA_infer/" --e2e_pgnet_polygon=True
# 预测image_dir指定的图像集合
-python3 tools/infer/predict_e2e.py --e2e_algorithm="PGNet" --image_dir="./doc/imgs_en/" --e2e_model_dir="./inference/e2e/" --e2e_pgnet_polygon=True
+python3 tools/infer/predict_e2e.py --e2e_algorithm="PGNet" --image_dir="./doc/imgs_en/" --e2e_model_dir="./inference/e2e_server_pgnetA_infer/" --e2e_pgnet_polygon=True
# 如果想使用CPU进行预测,需设置use_gpu参数为False
-python3 tools/infer/predict_e2e.py --e2e_algorithm="PGNet" --image_dir="./doc/imgs_en/img623.jpg" --e2e_model_dir="./inference/e2e/" --e2e_pgnet_polygon=True --use_gpu=False
+python3 tools/infer/predict_e2e.py --e2e_algorithm="PGNet" --image_dir="./doc/imgs_en/img623.jpg" --e2e_model_dir="./inference/e2e_server_pgnetA_infer/" --e2e_pgnet_polygon=True --use_gpu=False
```
### 可视化结果
可视化文本检测结果默认保存到./inference_results文件夹里面,结果文件的名称前缀为'e2e_res'。结果示例如下:

-
+
## 四、模型训练、评估、推理
本节以totaltext数据集为例,介绍PaddleOCR中端到端模型的训练、评估与测试。
### 准备数据
-下载解压[totaltext](https://github.com/cs-chan/Total-Text-Dataset/blob/master/Dataset/README.md)数据集到PaddleOCR/train_data/目录,数据集组织结构:
+下载解压[totaltext](https://github.com/cs-chan/Total-Text-Dataset/blob/master/Dataset/README.md) 数据集到PaddleOCR/train_data/目录,数据集组织结构:
```
/PaddleOCR/train_data/total_text/train/
|- rgb/ # total_text数据集的训练数据
@@ -135,20 +152,20 @@ python3 tools/eval.py -c configs/e2e/e2e_r50_vd_pg.yml -o Global.checkpoints="{
### 模型预测
测试单张图像的端到端识别效果
```shell
-python3 tools/infer_e2e.py -c configs/e2e/e2e_r50_vd_pg.yml -o Global.infer_img="./doc/imgs_en/img_10.jpg" Global.pretrained_model="./output/det_db/best_accuracy" Global.load_static_weights=false
+python3 tools/infer_e2e.py -c configs/e2e/e2e_r50_vd_pg.yml -o Global.infer_img="./doc/imgs_en/img_10.jpg" Global.pretrained_model="./output/e2e_pgnet/best_accuracy" Global.load_static_weights=false
```
测试文件夹下所有图像的端到端识别效果
```shell
-python3 tools/infer_e2e.py -c configs/e2e/e2e_r50_vd_pg.yml -o Global.infer_img="./doc/imgs_en/" Global.pretrained_model="./output/det_db/best_accuracy" Global.load_static_weights=false
+python3 tools/infer_e2e.py -c configs/e2e/e2e_r50_vd_pg.yml -o Global.infer_img="./doc/imgs_en/" Global.pretrained_model="./output/e2e_pgnet/best_accuracy" Global.load_static_weights=false
```
### 预测推理
-#### (1).四边形文本检测模型(ICDAR2015)
+#### (1). 四边形文本检测模型(ICDAR2015)
首先将PGNet端到端训练过程中保存的模型,转换成inference model。以基于Resnet50_vd骨干网络,以英文数据集训练的模型为例[模型下载地址](https://paddleocr.bj.bcebos.com/dygraph_v2.0/pgnet/en_server_pgnetA.tar) ,可以使用如下命令进行转换:
```
wget https://paddleocr.bj.bcebos.com/dygraph_v2.0/pgnet/en_server_pgnetA.tar && tar xf en_server_pgnetA.tar
-python3 tools/export_model.py -c configs/e2e/e2e_r50_vd_pg.yml -o Global.pretrained_model=./en_server_pgnetA/iter_epoch_450 Global.load_static_weights=False Global.save_inference_dir=./inference/e2e
+python3 tools/export_model.py -c configs/e2e/e2e_r50_vd_pg.yml -o Global.pretrained_model=./en_server_pgnetA/best_accuracy Global.load_static_weights=False Global.save_inference_dir=./inference/e2e
```
**PGNet端到端模型推理,需要设置参数`--e2e_algorithm="PGNet"`**,可以执行如下命令:
```
@@ -158,7 +175,7 @@ python3 tools/infer/predict_e2e.py --e2e_algorithm="PGNet" --image_dir="./doc/im

-#### (2).弯曲文本检测模型(Total-Text)
+#### (2). 弯曲文本检测模型(Total-Text)
对于弯曲文本样例
**PGNet端到端模型推理,需要设置参数`--e2e_algorithm="PGNet"`,同时,还需要增加参数`--e2e_pgnet_polygon=True`,**可以执行如下命令:
diff --git a/doc/doc_en/multi_languages_en.md b/doc/doc_en/multi_languages_en.md
index d1c4583f66f611fbef7191d52d32e02187853c9b..3f4f02266f4708efb7fdd3e9211678af7028c9df 100644
--- a/doc/doc_en/multi_languages_en.md
+++ b/doc/doc_en/multi_languages_en.md
@@ -2,24 +2,44 @@
**Recent Update**
--2021.4.9 supports the detection and recognition of 80 languages
--2021.4.9 supports **lightweight high-precision** English model detection and recognition
-
--[1 Installation](#Install)
- -[1.1 paddle installation](#paddleinstallation)
- -[1.2 paddleocr package installation](#paddleocr_package_install)
-
--[2 Quick Use](#Quick_Use)
- -[2.1 Command line operation](#Command_line_operation)
- -[2.1.1 Prediction of the whole image](#bash_detection+recognition)
- -[2.1.2 Recognition](#bash_Recognition)
- -[2.1.3 Detection](#bash_detection)
- -[2.2 python script running](#python_Script_running)
- -[2.2.1 Whole image prediction](#python_detection+recognition)
- -[2.2.2 Recognition](#python_Recognition)
- -[2.2.3 Detection](#python_detection)
--[3 Custom Training](#Custom_Training)
--[4 Supported languages and abbreviations](#language_abbreviations)
+- 2021.4.9 supports the detection and recognition of 80 languages
+- 2021.4.9 supports **lightweight high-precision** English model detection and recognition
+
+PaddleOCR aims to create a rich, leading, and practical OCR tool library, which not only provides
+Chinese and English models in general scenarios, but also provides models specifically trained
+in English scenarios. And multilingual models covering [80 languages](#language_abbreviations).
+
+Among them, the English model supports the detection and recognition of uppercase and lowercase
+letters and common punctuation, and the recognition of space characters is optimized:
+
+
+

+
+
+The multilingual models cover Latin, Arabic, Traditional Chinese, Korean, Japanese, etc.:
+
+
+

+

+
+
+This document will briefly introduce how to use the multilingual model.
+
+- [1 Installation](#Install)
+ - [1.1 paddle installation](#paddleinstallation)
+ - [1.2 paddleocr package installation](#paddleocr_package_install)
+
+- [2 Quick Use](#Quick_Use)
+ - [2.1 Command line operation](#Command_line_operation)
+ - [2.1.1 Prediction of the whole image](#bash_detection+recognition)
+ - [2.1.2 Recognition](#bash_Recognition)
+ - [2.1.3 Detection](#bash_detection)
+ - [2.2 python script running](#python_Script_running)
+ - [2.2.1 Whole image prediction](#python_detection+recognition)
+ - [2.2.2 Recognition](#python_Recognition)
+ - [2.2.3 Detection](#python_detection)
+- [3 Custom Training](#Custom_Training)
+- [4 Supported languages and abbreviations](#language_abbreviations)
## 1 Installation
@@ -69,7 +89,7 @@ The specific supported [language] (#language_abbreviations) can be viewed in the
paddleocr --image_dir doc/imgs/japan_2.jpg --lang=japan
```
-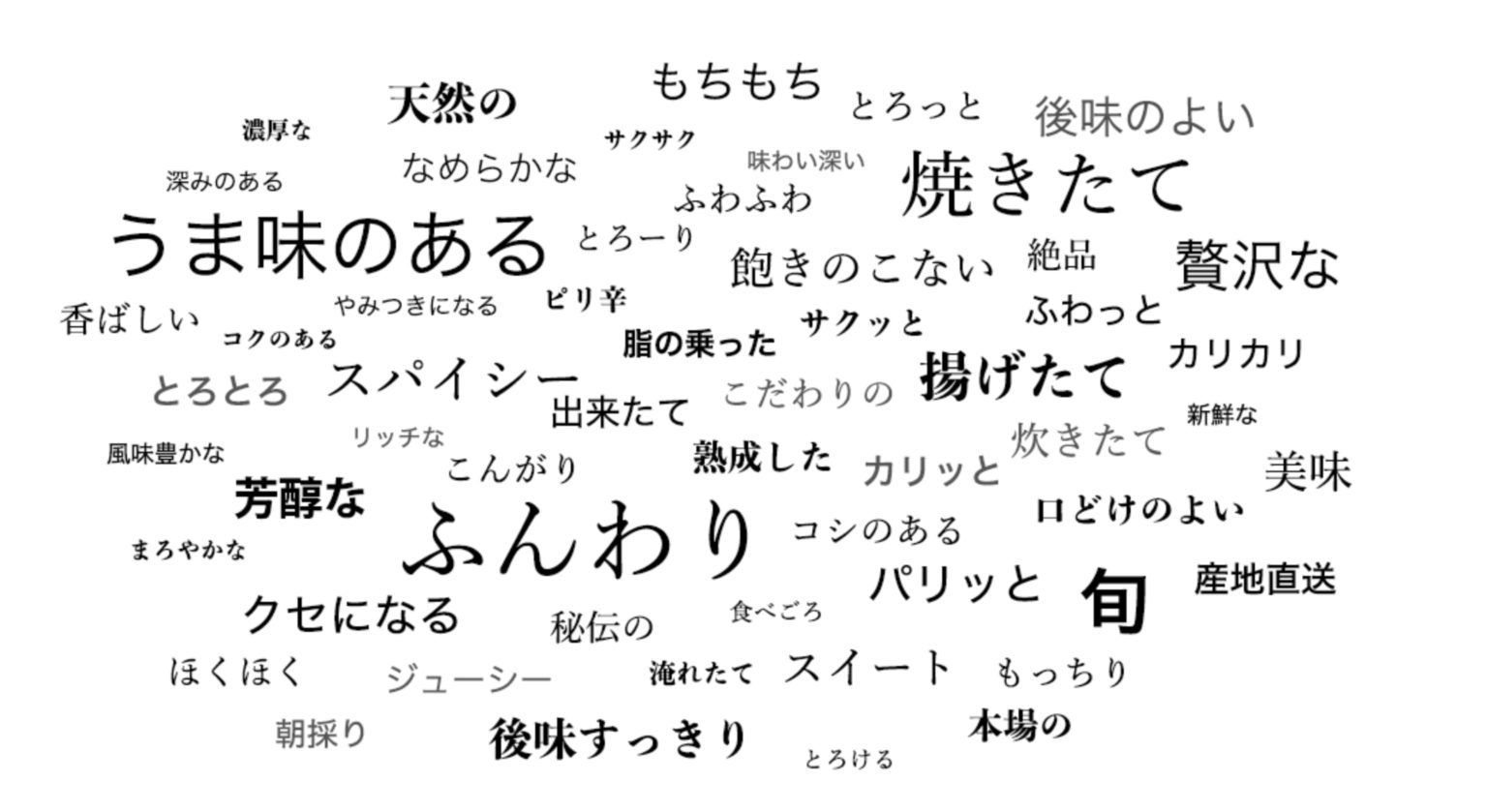
+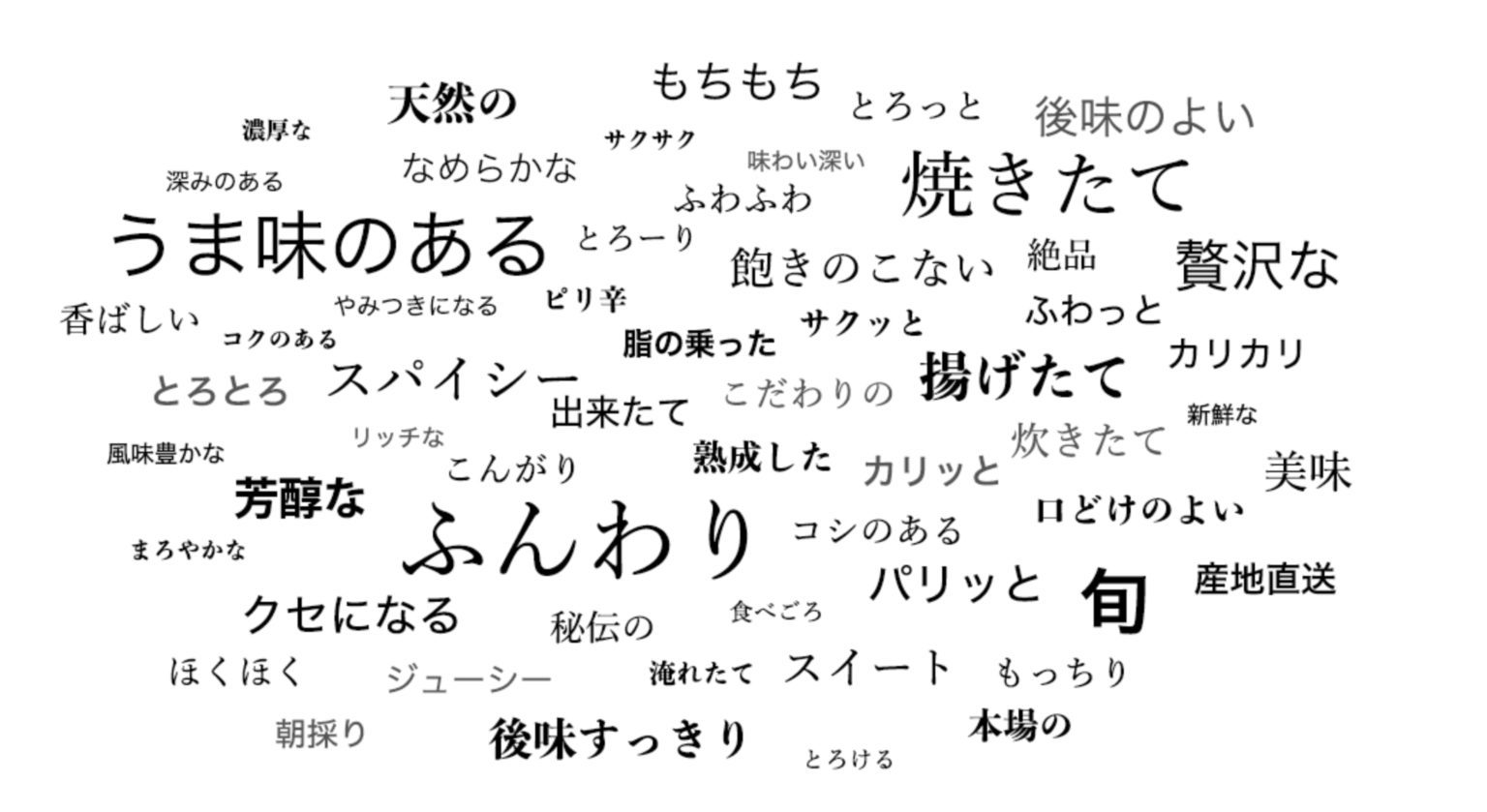
The result is a list, each item contains a text box, text and recognition confidence
```text
@@ -86,7 +106,7 @@ The result is a list, each item contains a text box, text and recognition confid
paddleocr --image_dir doc/imgs_words/japan/1.jpg --det false --lang=japan
```
-
+
The result is a tuple, which returns the recognition result and recognition confidence
@@ -139,7 +159,7 @@ im_show.save('result.jpg')
```
Visualization of results:
-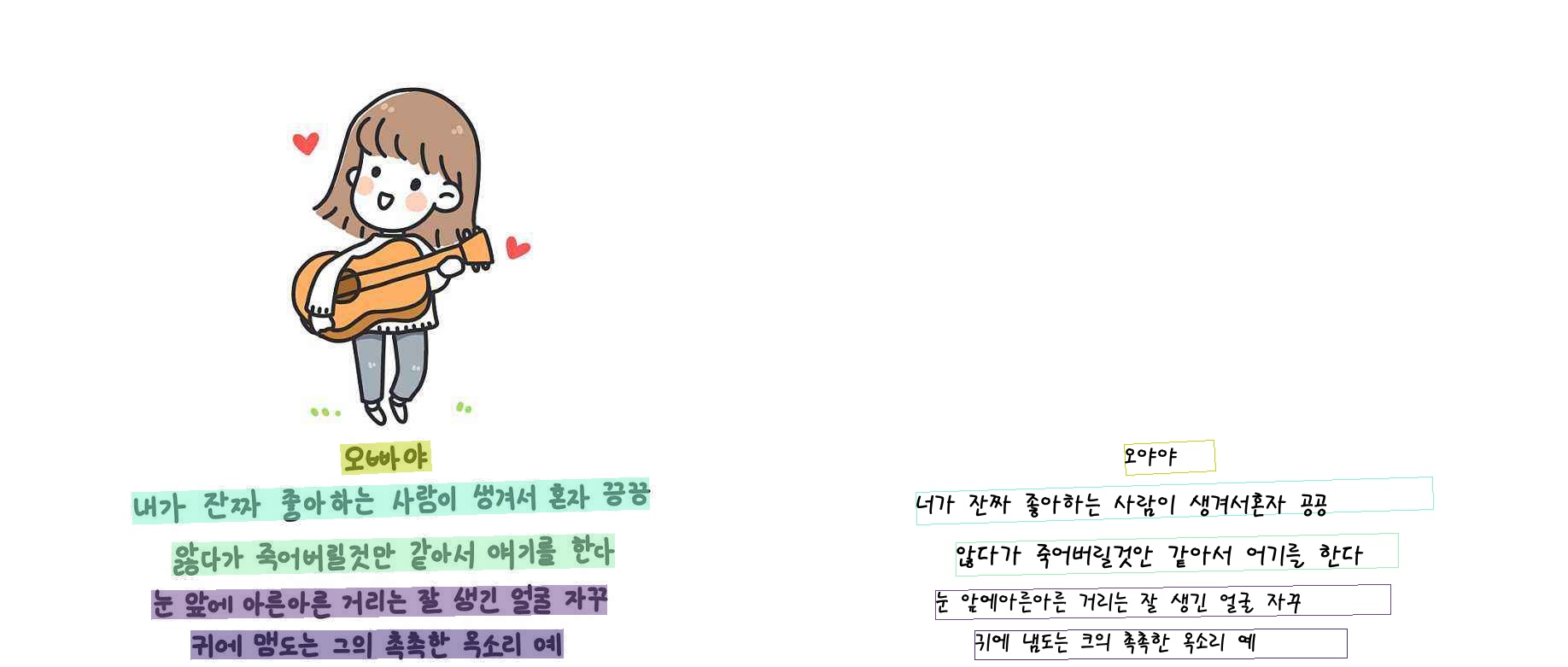
+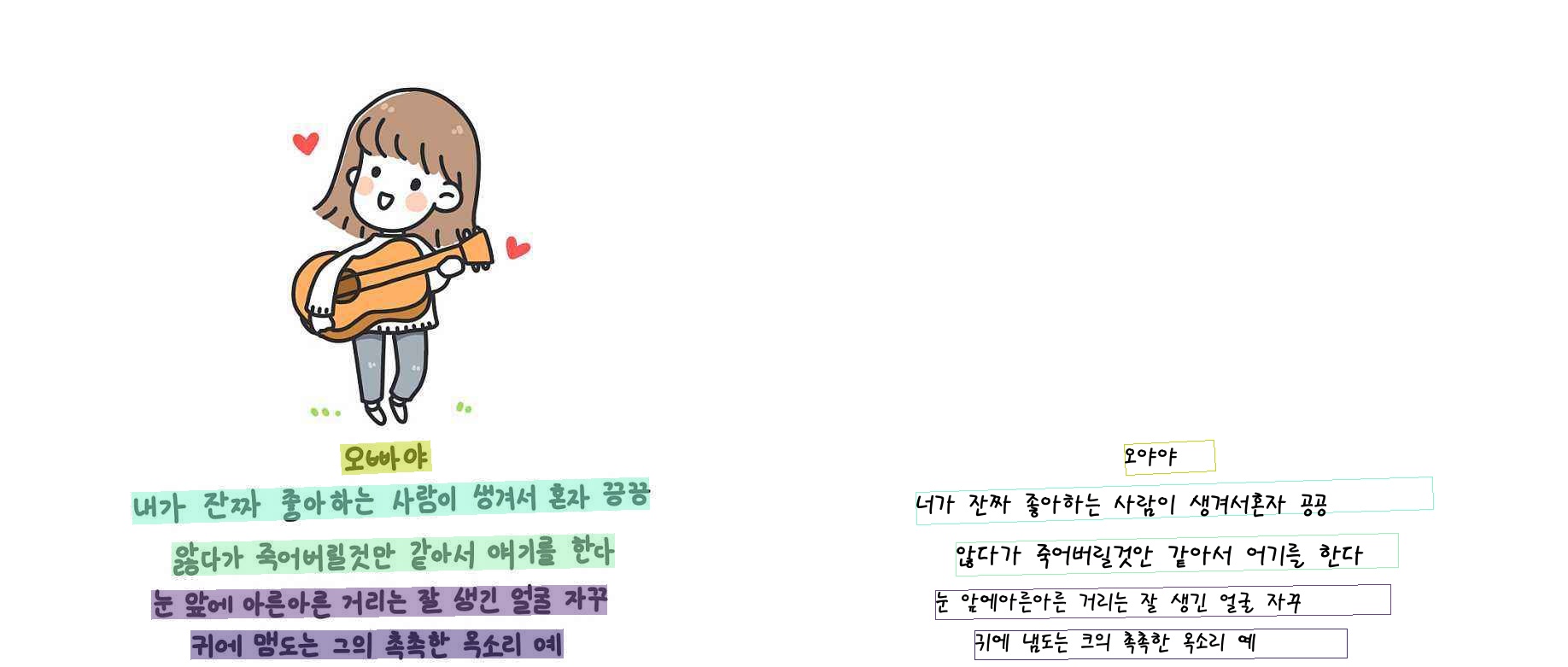
* Recognition
@@ -153,7 +173,7 @@ for line in result:
print(line)
```
-
+
The result is a tuple, which only contains the recognition result and recognition confidence
@@ -188,7 +208,7 @@ The result is a list, each item contains only text boxes
```
Visualization of results:
-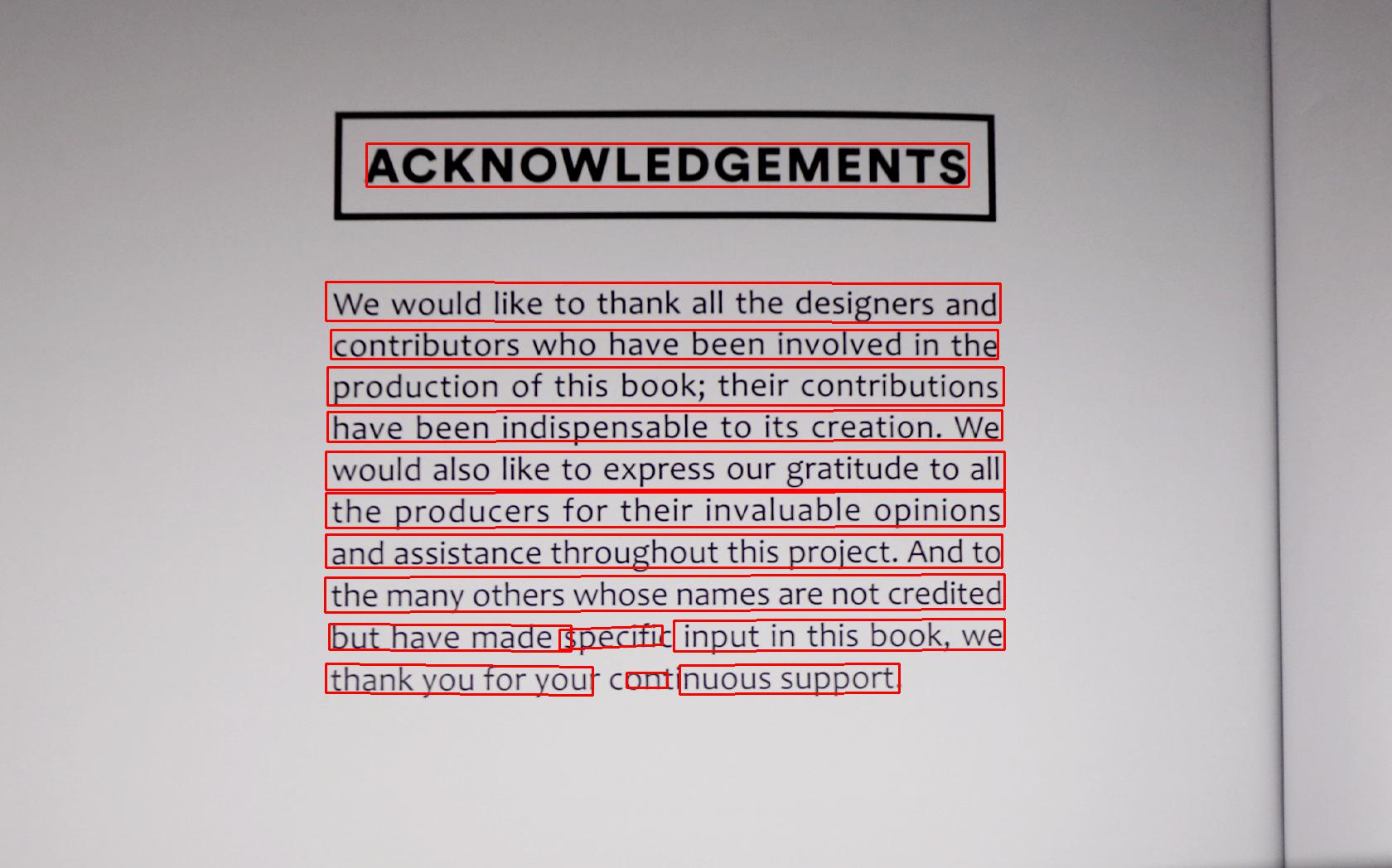
+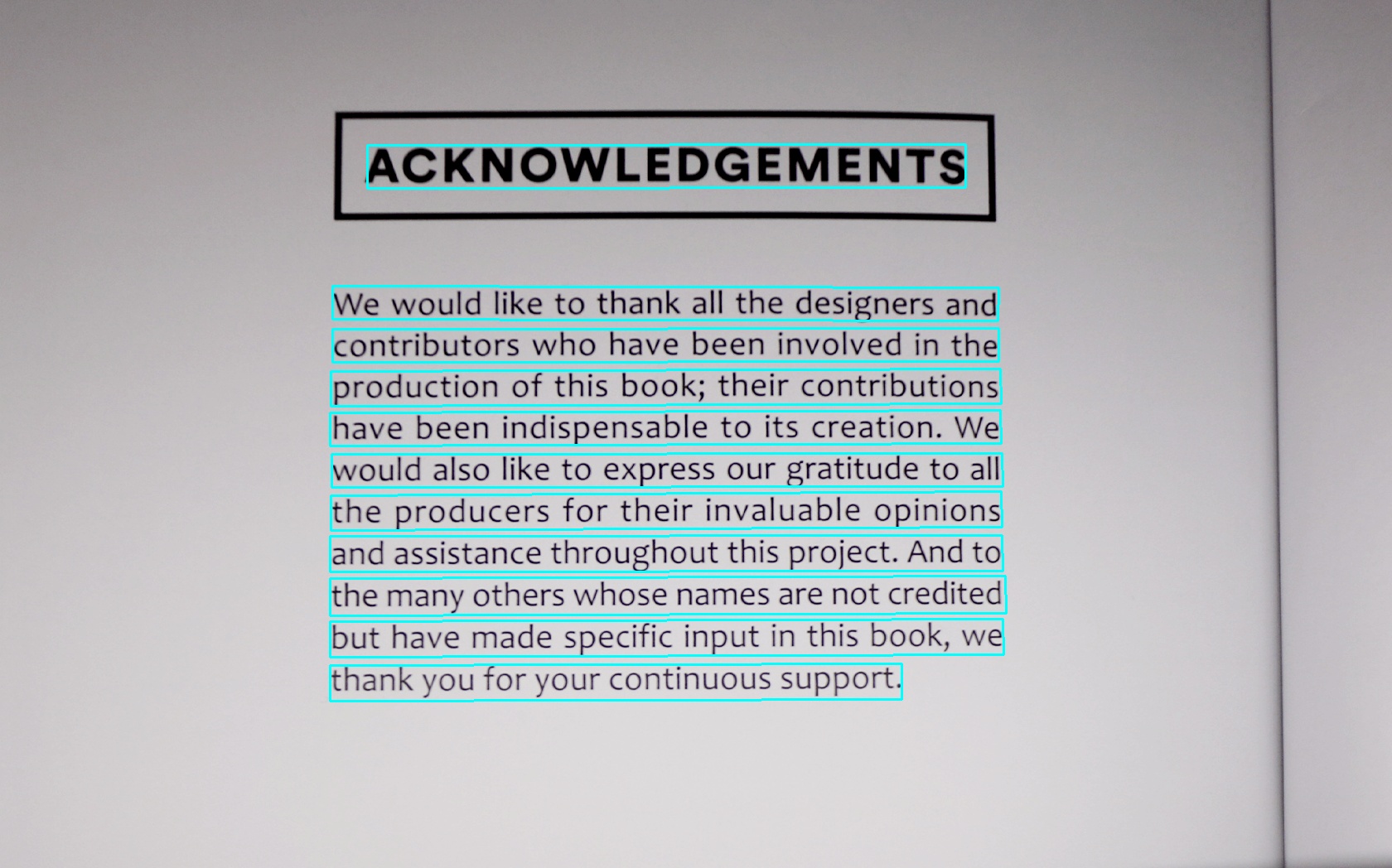
ppocr also supports direction classification. For more usage methods, please refer to: [whl package instructions](https://github.com/PaddlePaddle/PaddleOCR/blob/release/2.0/doc/doc_ch/whl.md).
diff --git a/doc/doc_en/pgnet_en.md b/doc/doc_en/pgnet_en.md
index 0f47f0e656f922e944710a746a6cd29ab6d46d8e..2ab1116ce7085e2e322b4be45ee5628c247040ea 100644
--- a/doc/doc_en/pgnet_en.md
+++ b/doc/doc_en/pgnet_en.md
@@ -15,7 +15,7 @@ In recent years, the end-to-end OCR algorithm has been well developed, including
- A graph based modification module (GRM) is proposed to further improve the performance of model recognition
- Higher accuracy and faster prediction speed
-For details of PGNet algorithm, please refer to [paper](https://www.aaai.org/AAAI21Papers/AAAI-2885.WangP.pdf), The schematic diagram of the algorithm is as follows:
+For details of PGNet algorithm, please refer to [paper](https://www.aaai.org/AAAI21Papers/AAAI-2885.WangP.pdf) ,The schematic diagram of the algorithm is as follows:

After feature extraction, the input image is sent to four branches: TBO module for text edge offset prediction, TCL module for text centerline prediction, TDO module for text direction offset prediction, and TCC module for text character classification graph prediction.
The output of TBO and TCL can get text detection results after post-processing, and TCL, TDO and TCC are responsible for text recognition.
@@ -23,6 +23,16 @@ The output of TBO and TCL can get text detection results after post-processing,
The results of detection and recognition are as follows:


+### Performance
+####Test set: Total Text
+
+####Test environment: NVIDIA Tesla V100-SXM2-16GB
+|PGNetA|det_precision|det_recall|det_f_score|e2e_precision|e2e_recall|e2e_f_score|FPS|download|
+| --- | --- | --- | --- | --- | --- | --- | --- | --- |
+|Paper|85.30|86.80|86.1|-|-|61.7|38.20 (size=640)|-|
+|Ours|87.03|82.48|84.69|61.71|58.43|60.03|48.73 (size=768)|[download link](https://paddleocr.bj.bcebos.com/dygraph_v2.0/pgnet/en_server_pgnetA.tar)|
+
+*note:PGNet in PaddleOCR optimizes the prediction speed, and can significantly improve the end-to-end prediction speed within the acceptable range of accuracy reduction*
## 2. Environment Configuration
@@ -49,13 +59,13 @@ After decompression, there should be the following file structure:
### Single image or image set prediction
```bash
# Prediction single image specified by image_dir
-python3 tools/infer/predict_e2e.py --e2e_algorithm="PGNet" --image_dir="./doc/imgs_en/img623.jpg" --e2e_model_dir="./inference/e2e/" --e2e_pgnet_polygon=True
+python3 tools/infer/predict_e2e.py --e2e_algorithm="PGNet" --image_dir="./doc/imgs_en/img623.jpg" --e2e_model_dir="./inference/e2e_server_pgnetA_infer/" --e2e_pgnet_polygon=True
# Prediction the collection of images specified by image_dir
-python3 tools/infer/predict_e2e.py --e2e_algorithm="PGNet" --image_dir="./doc/imgs_en/" --e2e_model_dir="./inference/e2e/" --e2e_pgnet_polygon=True
+python3 tools/infer/predict_e2e.py --e2e_algorithm="PGNet" --image_dir="./doc/imgs_en/" --e2e_model_dir="./inference/e2e_server_pgnetA_infer/" --e2e_pgnet_polygon=True
# If you want to use CPU for prediction, you need to set use_gpu parameter is false
-python3 tools/infer/predict_e2e.py --e2e_algorithm="PGNet" --image_dir="./doc/imgs_en/img623.jpg" --e2e_model_dir="./inference/e2e/" --e2e_pgnet_polygon=True --use_gpu=False
+python3 tools/infer/predict_e2e.py --e2e_algorithm="PGNet" --image_dir="./doc/imgs_en/img623.jpg" --e2e_model_dir="./inference/e2e_server_pgnetA_infer/" --e2e_pgnet_polygon=True --use_gpu=False
```
### Visualization results
The visualized end-to-end results are saved to the `./inference_results` folder by default, and the name of the result file is prefixed with 'e2e_res'. Examples of results are as follows:
@@ -141,12 +151,12 @@ python3 tools/eval.py -c configs/e2e/e2e_r50_vd_pg.yml -o Global.checkpoints="{
### Model Test
Test the end-to-end result on a single image:
```shell
-python3 tools/infer_e2e.py -c configs/e2e/e2e_r50_vd_pg.yml -o Global.infer_img="./doc/imgs_en/img_10.jpg" Global.pretrained_model="./output/det_db/best_accuracy" Global.load_static_weights=false
+python3 tools/infer_e2e.py -c configs/e2e/e2e_r50_vd_pg.yml -o Global.infer_img="./doc/imgs_en/img_10.jpg" Global.pretrained_model="./output/e2e_pgnet/best_accuracy" Global.load_static_weights=false
```
Test the end-to-end result on all images in the folder:
```shell
-python3 tools/infer_e2e.py -c configs/e2e/e2e_r50_vd_pg.yml -o Global.infer_img="./doc/imgs_en/" Global.pretrained_model="./output/det_db/best_accuracy" Global.load_static_weights=false
+python3 tools/infer_e2e.py -c configs/e2e/e2e_r50_vd_pg.yml -o Global.infer_img="./doc/imgs_en/" Global.pretrained_model="./output/e2e_pgnet/best_accuracy" Global.load_static_weights=false
```
### Model inference
@@ -154,7 +164,7 @@ python3 tools/infer_e2e.py -c configs/e2e/e2e_r50_vd_pg.yml -o Global.infer_img=
First, convert the model saved in the PGNet end-to-end training process into an inference model. In the first stage of training based on composite dataset, the model of English data set training is taken as an example[model download link](https://paddleocr.bj.bcebos.com/dygraph_v2.0/pgnet/en_server_pgnetA.tar), you can use the following command to convert:
```
wget https://paddleocr.bj.bcebos.com/dygraph_v2.0/pgnet/en_server_pgnetA.tar && tar xf en_server_pgnetA.tar
-python3 tools/export_model.py -c configs/e2e/e2e_r50_vd_pg.yml -o Global.pretrained_model=./en_server_pgnetA/iter_epoch_450 Global.load_static_weights=False Global.save_inference_dir=./inference/e2e
+python3 tools/export_model.py -c configs/e2e/e2e_r50_vd_pg.yml -o Global.pretrained_model=./en_server_pgnetA/best_accuracy Global.load_static_weights=False Global.save_inference_dir=./inference/e2e
```
**For PGNet quadrangle end-to-end model inference, you need to set the parameter `--e2e_algorithm="PGNet"`**, run the following command:
```
diff --git a/doc/imgs_results/multi_lang/en_1.jpg b/doc/imgs_results/multi_lang/en_1.jpg
new file mode 100644
index 0000000000000000000000000000000000000000..2dc84d3f04610effa7888e81848b015443e8091f
Binary files /dev/null and b/doc/imgs_results/multi_lang/en_1.jpg differ
diff --git a/doc/imgs_results/multi_lang/en_2.jpg b/doc/imgs_results/multi_lang/en_2.jpg
new file mode 100644
index 0000000000000000000000000000000000000000..455ec98ed337c6af9c45f2861b8078b31abb4054
Binary files /dev/null and b/doc/imgs_results/multi_lang/en_2.jpg differ
diff --git a/doc/imgs_results/multi_lang/en_3.jpg b/doc/imgs_results/multi_lang/en_3.jpg
new file mode 100644
index 0000000000000000000000000000000000000000..36eb063d78466a9000306dab11507e0a43664568
Binary files /dev/null and b/doc/imgs_results/multi_lang/en_3.jpg differ
diff --git a/doc/imgs_results/multi_lang/french_0.jpg b/doc/imgs_results/multi_lang/french_0.jpg
new file mode 100644
index 0000000000000000000000000000000000000000..3c2abe6304b93e3025dd19b75980c548f70bd3c7
Binary files /dev/null and b/doc/imgs_results/multi_lang/french_0.jpg differ
diff --git a/doc/imgs_results/multi_lang/japan_2.jpg b/doc/imgs_results/multi_lang/japan_2.jpg
new file mode 100644
index 0000000000000000000000000000000000000000..7038ba2effab289b2895ea8d0fddaa365149ff80
Binary files /dev/null and b/doc/imgs_results/multi_lang/japan_2.jpg differ
diff --git a/ppocr/data/imaug/label_ops.py b/ppocr/data/imaug/label_ops.py
index 47e0cbf07d8bd8b6ad838fa2d211345c65a6751a..cbb110090cfff3ebee4b30b009f88fc9aaba1617 100644
--- a/ppocr/data/imaug/label_ops.py
+++ b/ppocr/data/imaug/label_ops.py
@@ -200,18 +200,16 @@ class E2ELabelEncode(BaseRecLabelEncode):
self.pad_num = len(self.dict) # the length to pad
def __call__(self, data):
- text_label_index_list, temp_text = [], []
texts = data['strs']
+ temp_texts = []
for text in texts:
text = text.lower()
- temp_text = []
- for c_ in text:
- if c_ in self.dict:
- temp_text.append(self.dict[c_])
- temp_text = temp_text + [self.pad_num] * (self.max_text_len -
- len(temp_text))
- text_label_index_list.append(temp_text)
- data['strs'] = np.array(text_label_index_list)
+ text = self.encode(text)
+ if text is None:
+ return None
+ text = text + [self.pad_num] * (self.max_text_len - len(text))
+ temp_texts.append(text)
+ data['strs'] = np.array(temp_texts)
return data
diff --git a/ppocr/data/pgnet_dataset.py b/ppocr/data/pgnet_dataset.py
index ae0638350ad02f10202a67bc6cd531daf742f984..543dbe79ef6ff548c91b4e17bf6424797cdeeea7 100644
--- a/ppocr/data/pgnet_dataset.py
+++ b/ppocr/data/pgnet_dataset.py
@@ -64,9 +64,6 @@ class PGDataSet(Dataset):
for line in f.readlines():
poly_str, txt = line.strip().split('\t')
poly = list(map(float, poly_str.split(',')))
- if self.mode.lower() == "eval":
- while len(poly) < 100:
- poly.append(-1)
text_polys.append(
np.array(
poly, dtype=np.float32).reshape(-1, 2))
@@ -139,23 +136,21 @@ class PGDataSet(Dataset):
try:
if self.data_format == 'icdar':
im_path = os.path.join(data_path, 'rgb', data_line)
- if self.mode.lower() == "eval":
- poly_path = os.path.join(data_path, 'poly_gt',
- data_line.split('.')[0] + '.txt')
- else:
- poly_path = os.path.join(data_path, 'poly',
- data_line.split('.')[0] + '.txt')
+ poly_path = os.path.join(data_path, 'poly',
+ data_line.split('.')[0] + '.txt')
text_polys, text_tags, text_strs = self.extract_polys(poly_path)
else:
image_dir = os.path.join(os.path.dirname(data_path), 'image')
im_path, text_polys, text_tags, text_strs = self.extract_info_textnet(
data_line, image_dir)
+ img_id = int(data_line.split(".")[0][3:])
data = {
'img_path': im_path,
'polys': text_polys,
'tags': text_tags,
- 'strs': text_strs
+ 'strs': text_strs,
+ 'img_id': img_id
}
with open(data['img_path'], 'rb') as f:
img = f.read()
diff --git a/ppocr/metrics/e2e_metric.py b/ppocr/metrics/e2e_metric.py
index 684d77421c659d4150ea4a28a99b4ae43d678b69..8a604192fa455071202eec157e3832e2804bfdfd 100644
--- a/ppocr/metrics/e2e_metric.py
+++ b/ppocr/metrics/e2e_metric.py
@@ -19,58 +19,29 @@ from __future__ import print_function
__all__ = ['E2EMetric']
from ppocr.utils.e2e_metric.Deteval import get_socre, combine_results
-from ppocr.utils.e2e_utils.extract_textpoint import get_dict
+from ppocr.utils.e2e_utils.extract_textpoint_slow import get_dict
class E2EMetric(object):
def __init__(self,
+ gt_mat_dir,
character_dict_path,
main_indicator='f_score_e2e',
**kwargs):
+ self.gt_mat_dir = gt_mat_dir
self.label_list = get_dict(character_dict_path)
self.max_index = len(self.label_list)
self.main_indicator = main_indicator
self.reset()
def __call__(self, preds, batch, **kwargs):
- temp_gt_polyons_batch = batch[2]
- temp_gt_strs_batch = batch[3]
- ignore_tags_batch = batch[4]
- gt_polyons_batch = []
- gt_strs_batch = []
-
- temp_gt_polyons_batch = temp_gt_polyons_batch[0].tolist()
- for temp_list in temp_gt_polyons_batch:
- t = []
- for index in temp_list:
- if index[0] != -1 and index[1] != -1:
- t.append(index)
- gt_polyons_batch.append(t)
-
- temp_gt_strs_batch = temp_gt_strs_batch[0].tolist()
- for temp_list in temp_gt_strs_batch:
- t = ""
- for index in temp_list:
- if index < self.max_index:
- t += self.label_list[index]
- gt_strs_batch.append(t)
-
- for pred, gt_polyons, gt_strs, ignore_tags in zip(
- [preds], [gt_polyons_batch], [gt_strs_batch], ignore_tags_batch):
- # prepare gt
- gt_info_list = [{
- 'points': gt_polyon,
- 'text': gt_str,
- 'ignore': ignore_tag
- } for gt_polyon, gt_str, ignore_tag in
- zip(gt_polyons, gt_strs, ignore_tags)]
- # prepare det
- e2e_info_list = [{
- 'points': det_polyon,
- 'text': pred_str
- } for det_polyon, pred_str in zip(pred['points'], pred['strs'])]
- result = get_socre(gt_info_list, e2e_info_list)
- self.results.append(result)
+ img_id = batch[5][0]
+ e2e_info_list = [{
+ 'points': det_polyon,
+ 'text': pred_str
+ } for det_polyon, pred_str in zip(preds['points'], preds['strs'])]
+ result = get_socre(self.gt_mat_dir, img_id, e2e_info_list)
+ self.results.append(result)
def get_metric(self):
metircs = combine_results(self.results)
diff --git a/ppocr/postprocess/pg_postprocess.py b/ppocr/postprocess/pg_postprocess.py
index d9c0048f20ff46850ab8a26554af31532c73efd6..0b1455181fddb0adb5347406bb2eb3093ee6fb30 100644
--- a/ppocr/postprocess/pg_postprocess.py
+++ b/ppocr/postprocess/pg_postprocess.py
@@ -22,10 +22,7 @@ import sys
__dir__ = os.path.dirname(__file__)
sys.path.append(__dir__)
sys.path.append(os.path.join(__dir__, '..'))
-
-from ppocr.utils.e2e_utils.extract_textpoint import *
-from ppocr.utils.e2e_utils.visual import *
-import paddle
+from ppocr.utils.e2e_utils.pgnet_pp_utils import PGNet_PostProcess
class PGPostProcess(object):
@@ -33,10 +30,12 @@ class PGPostProcess(object):
The post process for PGNet.
"""
- def __init__(self, character_dict_path, valid_set, score_thresh, **kwargs):
- self.Lexicon_Table = get_dict(character_dict_path)
+ def __init__(self, character_dict_path, valid_set, score_thresh, mode,
+ **kwargs):
+ self.character_dict_path = character_dict_path
self.valid_set = valid_set
self.score_thresh = score_thresh
+ self.mode = mode
# c++ la-nms is faster, but only support python 3.5
self.is_python35 = False
@@ -44,112 +43,10 @@ class PGPostProcess(object):
self.is_python35 = True
def __call__(self, outs_dict, shape_list):
- p_score = outs_dict['f_score']
- p_border = outs_dict['f_border']
- p_char = outs_dict['f_char']
- p_direction = outs_dict['f_direction']
- if isinstance(p_score, paddle.Tensor):
- p_score = p_score[0].numpy()
- p_border = p_border[0].numpy()
- p_direction = p_direction[0].numpy()
- p_char = p_char[0].numpy()
+ post = PGNet_PostProcess(self.character_dict_path, self.valid_set,
+ self.score_thresh, outs_dict, shape_list)
+ if self.mode == 'fast':
+ data = post.pg_postprocess_fast()
else:
- p_score = p_score[0]
- p_border = p_border[0]
- p_direction = p_direction[0]
- p_char = p_char[0]
- src_h, src_w, ratio_h, ratio_w = shape_list[0]
- is_curved = self.valid_set == "totaltext"
- instance_yxs_list = generate_pivot_list(
- p_score,
- p_char,
- p_direction,
- score_thresh=self.score_thresh,
- is_backbone=True,
- is_curved=is_curved)
- p_char = paddle.to_tensor(np.expand_dims(p_char, axis=0))
- char_seq_idx_set = []
- for i in range(len(instance_yxs_list)):
- gather_info_lod = paddle.to_tensor(instance_yxs_list[i])
- f_char_map = paddle.transpose(p_char, [0, 2, 3, 1])
- feature_seq = paddle.gather_nd(f_char_map, gather_info_lod)
- feature_seq = np.expand_dims(feature_seq.numpy(), axis=0)
- feature_len = [len(feature_seq[0])]
- featyre_seq = paddle.to_tensor(feature_seq)
- feature_len = np.array([feature_len]).astype(np.int64)
- length = paddle.to_tensor(feature_len)
- seq_pred = paddle.fluid.layers.ctc_greedy_decoder(
- input=featyre_seq, blank=36, input_length=length)
- seq_pred_str = seq_pred[0].numpy().tolist()[0]
- seq_len = seq_pred[1].numpy()[0][0]
- temp_t = []
- for c in seq_pred_str[:seq_len]:
- temp_t.append(c)
- char_seq_idx_set.append(temp_t)
- seq_strs = []
- for char_idx_set in char_seq_idx_set:
- pr_str = ''.join([self.Lexicon_Table[pos] for pos in char_idx_set])
- seq_strs.append(pr_str)
- poly_list = []
- keep_str_list = []
- all_point_list = []
- all_point_pair_list = []
- for yx_center_line, keep_str in zip(instance_yxs_list, seq_strs):
- if len(yx_center_line) == 1:
- yx_center_line.append(yx_center_line[-1])
-
- offset_expand = 1.0
- if self.valid_set == 'totaltext':
- offset_expand = 1.2
-
- point_pair_list = []
- for batch_id, y, x in yx_center_line:
- offset = p_border[:, y, x].reshape(2, 2)
- if offset_expand != 1.0:
- offset_length = np.linalg.norm(
- offset, axis=1, keepdims=True)
- expand_length = np.clip(
- offset_length * (offset_expand - 1),
- a_min=0.5,
- a_max=3.0)
- offset_detal = offset / offset_length * expand_length
- offset = offset + offset_detal
- ori_yx = np.array([y, x], dtype=np.float32)
- point_pair = (ori_yx + offset)[:, ::-1] * 4.0 / np.array(
- [ratio_w, ratio_h]).reshape(-1, 2)
- point_pair_list.append(point_pair)
-
- all_point_list.append([
- int(round(x * 4.0 / ratio_w)),
- int(round(y * 4.0 / ratio_h))
- ])
- all_point_pair_list.append(point_pair.round().astype(np.int32)
- .tolist())
-
- detected_poly, pair_length_info = point_pair2poly(point_pair_list)
- detected_poly = expand_poly_along_width(
- detected_poly, shrink_ratio_of_width=0.2)
- detected_poly[:, 0] = np.clip(
- detected_poly[:, 0], a_min=0, a_max=src_w)
- detected_poly[:, 1] = np.clip(
- detected_poly[:, 1], a_min=0, a_max=src_h)
-
- if len(keep_str) < 2:
- continue
-
- keep_str_list.append(keep_str)
- if self.valid_set == 'partvgg':
- middle_point = len(detected_poly) // 2
- detected_poly = detected_poly[
- [0, middle_point - 1, middle_point, -1], :]
- poly_list.append(detected_poly)
- elif self.valid_set == 'totaltext':
- poly_list.append(detected_poly)
- else:
- print('--> Not supported format.')
- exit(-1)
- data = {
- 'points': poly_list,
- 'strs': keep_str_list,
- }
+ data = post.pg_postprocess_slow()
return data
diff --git a/ppocr/utils/e2e_metric/Deteval.py b/ppocr/utils/e2e_metric/Deteval.py
index 8033a9ff9f1f55200d43472f405d5805e238085b..e30a498eaf2e24f7a337ee48536466e7c4f0d91c 100755
--- a/ppocr/utils/e2e_metric/Deteval.py
+++ b/ppocr/utils/e2e_metric/Deteval.py
@@ -13,10 +13,11 @@
# limitations under the License.
import numpy as np
+import scipy.io as io
from ppocr.utils.e2e_metric.polygon_fast import iod, area_of_intersection, area
-def get_socre(gt_dict, pred_dict):
+def get_socre(gt_dir, img_id, pred_dict):
allInputs = 1
def input_reading_mod(pred_dict):
@@ -30,31 +31,9 @@ def get_socre(gt_dict, pred_dict):
det.append([point, text])
return det
- def gt_reading_mod(gt_dict):
- """This helper reads groundtruths from mat files"""
- gt = []
- n = len(gt_dict)
- for i in range(n):
- points = gt_dict[i]['points']
- h = len(points)
- text = gt_dict[i]['text']
- xx = [
- np.array(
- ['x:'], dtype=' y, x
+ sorted_point, sorted_direction = sort_part_with_direction(pos_list,
+ point_direction)
+
+ point_num = len(sorted_point)
+ if point_num >= 16:
+ middle_num = point_num // 2
+ first_part_point = sorted_point[:middle_num]
+ first_point_direction = sorted_direction[:middle_num]
+ sorted_fist_part_point, sorted_fist_part_direction = sort_part_with_direction(
+ first_part_point, first_point_direction)
+
+ last_part_point = sorted_point[middle_num:]
+ last_point_direction = sorted_direction[middle_num:]
+ sorted_last_part_point, sorted_last_part_direction = sort_part_with_direction(
+ last_part_point, last_point_direction)
+ sorted_point = sorted_fist_part_point + sorted_last_part_point
+ sorted_direction = sorted_fist_part_direction + sorted_last_part_direction
+
+ return sorted_point, np.array(sorted_direction)
+
+
+def add_id(pos_list, image_id=0):
+ """
+ Add id for gather feature, for inference.
+ """
+ new_list = []
+ for item in pos_list:
+ new_list.append((image_id, item[0], item[1]))
+ return new_list
+
+
+def sort_and_expand_with_direction(pos_list, f_direction):
+ """
+ f_direction: h x w x 2
+ pos_list: [[y, x], [y, x], [y, x] ...]
+ """
+ h, w, _ = f_direction.shape
+ sorted_list, point_direction = sort_with_direction(pos_list, f_direction)
+
+ point_num = len(sorted_list)
+ sub_direction_len = max(point_num // 3, 2)
+ left_direction = point_direction[:sub_direction_len, :]
+ right_dirction = point_direction[point_num - sub_direction_len:, :]
+
+ left_average_direction = -np.mean(left_direction, axis=0, keepdims=True)
+ left_average_len = np.linalg.norm(left_average_direction)
+ left_start = np.array(sorted_list[0])
+ left_step = left_average_direction / (left_average_len + 1e-6)
+
+ right_average_direction = np.mean(right_dirction, axis=0, keepdims=True)
+ right_average_len = np.linalg.norm(right_average_direction)
+ right_step = right_average_direction / (right_average_len + 1e-6)
+ right_start = np.array(sorted_list[-1])
+
+ append_num = max(
+ int((left_average_len + right_average_len) / 2.0 * 0.15), 1)
+ left_list = []
+ right_list = []
+ for i in range(append_num):
+ ly, lx = np.round(left_start + left_step * (i + 1)).flatten().astype(
+ 'int32').tolist()
+ if ly < h and lx < w and (ly, lx) not in left_list:
+ left_list.append((ly, lx))
+ ry, rx = np.round(right_start + right_step * (i + 1)).flatten().astype(
+ 'int32').tolist()
+ if ry < h and rx < w and (ry, rx) not in right_list:
+ right_list.append((ry, rx))
+
+ all_list = left_list[::-1] + sorted_list + right_list
+ return all_list
+
+
+def sort_and_expand_with_direction_v2(pos_list, f_direction, binary_tcl_map):
+ """
+ f_direction: h x w x 2
+ pos_list: [[y, x], [y, x], [y, x] ...]
+ binary_tcl_map: h x w
+ """
+ h, w, _ = f_direction.shape
+ sorted_list, point_direction = sort_with_direction(pos_list, f_direction)
+
+ point_num = len(sorted_list)
+ sub_direction_len = max(point_num // 3, 2)
+ left_direction = point_direction[:sub_direction_len, :]
+ right_dirction = point_direction[point_num - sub_direction_len:, :]
+
+ left_average_direction = -np.mean(left_direction, axis=0, keepdims=True)
+ left_average_len = np.linalg.norm(left_average_direction)
+ left_start = np.array(sorted_list[0])
+ left_step = left_average_direction / (left_average_len + 1e-6)
+
+ right_average_direction = np.mean(right_dirction, axis=0, keepdims=True)
+ right_average_len = np.linalg.norm(right_average_direction)
+ right_step = right_average_direction / (right_average_len + 1e-6)
+ right_start = np.array(sorted_list[-1])
+
+ append_num = max(
+ int((left_average_len + right_average_len) / 2.0 * 0.15), 1)
+ max_append_num = 2 * append_num
+
+ left_list = []
+ right_list = []
+ for i in range(max_append_num):
+ ly, lx = np.round(left_start + left_step * (i + 1)).flatten().astype(
+ 'int32').tolist()
+ if ly < h and lx < w and (ly, lx) not in left_list:
+ if binary_tcl_map[ly, lx] > 0.5:
+ left_list.append((ly, lx))
+ else:
+ break
+
+ for i in range(max_append_num):
+ ry, rx = np.round(right_start + right_step * (i + 1)).flatten().astype(
+ 'int32').tolist()
+ if ry < h and rx < w and (ry, rx) not in right_list:
+ if binary_tcl_map[ry, rx] > 0.5:
+ right_list.append((ry, rx))
+ else:
+ break
+
+ all_list = left_list[::-1] + sorted_list + right_list
+ return all_list
+
+
+def point_pair2poly(point_pair_list):
+ """
+ Transfer vertical point_pairs into poly point in clockwise.
+ """
+ point_num = len(point_pair_list) * 2
+ point_list = [0] * point_num
+ for idx, point_pair in enumerate(point_pair_list):
+ point_list[idx] = point_pair[0]
+ point_list[point_num - 1 - idx] = point_pair[1]
+ return np.array(point_list).reshape(-1, 2)
+
+
+def shrink_quad_along_width(quad, begin_width_ratio=0., end_width_ratio=1.):
+ ratio_pair = np.array(
+ [[begin_width_ratio], [end_width_ratio]], dtype=np.float32)
+ p0_1 = quad[0] + (quad[1] - quad[0]) * ratio_pair
+ p3_2 = quad[3] + (quad[2] - quad[3]) * ratio_pair
+ return np.array([p0_1[0], p0_1[1], p3_2[1], p3_2[0]])
+
+
+def expand_poly_along_width(poly, shrink_ratio_of_width=0.3):
+ """
+ expand poly along width.
+ """
+ point_num = poly.shape[0]
+ left_quad = np.array(
+ [poly[0], poly[1], poly[-2], poly[-1]], dtype=np.float32)
+ left_ratio = -shrink_ratio_of_width * np.linalg.norm(left_quad[0] - left_quad[3]) / \
+ (np.linalg.norm(left_quad[0] - left_quad[1]) + 1e-6)
+ left_quad_expand = shrink_quad_along_width(left_quad, left_ratio, 1.0)
+ right_quad = np.array(
+ [
+ poly[point_num // 2 - 2], poly[point_num // 2 - 1],
+ poly[point_num // 2], poly[point_num // 2 + 1]
+ ],
+ dtype=np.float32)
+ right_ratio = 1.0 + shrink_ratio_of_width * np.linalg.norm(right_quad[0] - right_quad[3]) / \
+ (np.linalg.norm(right_quad[0] - right_quad[1]) + 1e-6)
+ right_quad_expand = shrink_quad_along_width(right_quad, 0.0, right_ratio)
+ poly[0] = left_quad_expand[0]
+ poly[-1] = left_quad_expand[-1]
+ poly[point_num // 2 - 1] = right_quad_expand[1]
+ poly[point_num // 2] = right_quad_expand[2]
+ return poly
+
+
+def restore_poly(instance_yxs_list, seq_strs, p_border, ratio_w, ratio_h, src_w,
+ src_h, valid_set):
+ poly_list = []
+ keep_str_list = []
+ for yx_center_line, keep_str in zip(instance_yxs_list, seq_strs):
+ if len(keep_str) < 2:
+ print('--> too short, {}'.format(keep_str))
+ continue
+
+ offset_expand = 1.0
+ if valid_set == 'totaltext':
+ offset_expand = 1.2
+
+ point_pair_list = []
+ for y, x in yx_center_line:
+ offset = p_border[:, y, x].reshape(2, 2) * offset_expand
+ ori_yx = np.array([y, x], dtype=np.float32)
+ point_pair = (ori_yx + offset)[:, ::-1] * 4.0 / np.array(
+ [ratio_w, ratio_h]).reshape(-1, 2)
+ point_pair_list.append(point_pair)
+
+ detected_poly = point_pair2poly(point_pair_list)
+ detected_poly = expand_poly_along_width(
+ detected_poly, shrink_ratio_of_width=0.2)
+ detected_poly[:, 0] = np.clip(detected_poly[:, 0], a_min=0, a_max=src_w)
+ detected_poly[:, 1] = np.clip(detected_poly[:, 1], a_min=0, a_max=src_h)
+
+ keep_str_list.append(keep_str)
+ if valid_set == 'partvgg':
+ middle_point = len(detected_poly) // 2
+ detected_poly = detected_poly[
+ [0, middle_point - 1, middle_point, -1], :]
+ poly_list.append(detected_poly)
+ elif valid_set == 'totaltext':
+ poly_list.append(detected_poly)
+ else:
+ print('--> Not supported format.')
+ exit(-1)
+ return poly_list, keep_str_list
+
+
+def generate_pivot_list_fast(p_score,
+ p_char_maps,
+ f_direction,
+ Lexicon_Table,
+ score_thresh=0.5):
+ """
+ return center point and end point of TCL instance; filter with the char maps;
+ """
+ p_score = p_score[0]
+ f_direction = f_direction.transpose(1, 2, 0)
+ p_tcl_map = (p_score > score_thresh) * 1.0
+ skeleton_map = thin(p_tcl_map.astype(np.uint8))
+ instance_count, instance_label_map = cv2.connectedComponents(
+ skeleton_map.astype(np.uint8), connectivity=8)
+
+ # get TCL Instance
+ all_pos_yxs = []
+ if instance_count > 0:
+ for instance_id in range(1, instance_count):
+ pos_list = []
+ ys, xs = np.where(instance_label_map == instance_id)
+ pos_list = list(zip(ys, xs))
+
+ if len(pos_list) < 3:
+ continue
+
+ pos_list_sorted = sort_and_expand_with_direction_v2(
+ pos_list, f_direction, p_tcl_map)
+ all_pos_yxs.append(pos_list_sorted)
+
+ p_char_maps = p_char_maps.transpose([1, 2, 0])
+ decoded_str, keep_yxs_list = ctc_decoder_for_image(
+ all_pos_yxs, logits_map=p_char_maps, Lexicon_Table=Lexicon_Table)
+ return keep_yxs_list, decoded_str
+
+
+def extract_main_direction(pos_list, f_direction):
+ """
+ f_direction: h x w x 2
+ pos_list: [[y, x], [y, x], [y, x] ...]
+ """
+ pos_list = np.array(pos_list)
+ point_direction = f_direction[pos_list[:, 0], pos_list[:, 1]]
+ point_direction = point_direction[:, ::-1] # x, y -> y, x
+ average_direction = np.mean(point_direction, axis=0, keepdims=True)
+ average_direction = average_direction / (
+ np.linalg.norm(average_direction) + 1e-6)
+ return average_direction
+
+
+def sort_by_direction_with_image_id_deprecated(pos_list, f_direction):
+ """
+ f_direction: h x w x 2
+ pos_list: [[id, y, x], [id, y, x], [id, y, x] ...]
+ """
+ pos_list_full = np.array(pos_list).reshape(-1, 3)
+ pos_list = pos_list_full[:, 1:]
+ point_direction = f_direction[pos_list[:, 0], pos_list[:, 1]] # x, y
+ point_direction = point_direction[:, ::-1] # x, y -> y, x
+ average_direction = np.mean(point_direction, axis=0, keepdims=True)
+ pos_proj_leng = np.sum(pos_list * average_direction, axis=1)
+ sorted_list = pos_list_full[np.argsort(pos_proj_leng)].tolist()
+ return sorted_list
+
+
+def sort_by_direction_with_image_id(pos_list, f_direction):
+ """
+ f_direction: h x w x 2
+ pos_list: [[y, x], [y, x], [y, x] ...]
+ """
+
+ def sort_part_with_direction(pos_list_full, point_direction):
+ pos_list_full = np.array(pos_list_full).reshape(-1, 3)
+ pos_list = pos_list_full[:, 1:]
+ point_direction = np.array(point_direction).reshape(-1, 2)
+ average_direction = np.mean(point_direction, axis=0, keepdims=True)
+ pos_proj_leng = np.sum(pos_list * average_direction, axis=1)
+ sorted_list = pos_list_full[np.argsort(pos_proj_leng)].tolist()
+ sorted_direction = point_direction[np.argsort(pos_proj_leng)].tolist()
+ return sorted_list, sorted_direction
+
+ pos_list = np.array(pos_list).reshape(-1, 3)
+ point_direction = f_direction[pos_list[:, 1], pos_list[:, 2]] # x, y
+ point_direction = point_direction[:, ::-1] # x, y -> y, x
+ sorted_point, sorted_direction = sort_part_with_direction(pos_list,
+ point_direction)
+
+ point_num = len(sorted_point)
+ if point_num >= 16:
+ middle_num = point_num // 2
+ first_part_point = sorted_point[:middle_num]
+ first_point_direction = sorted_direction[:middle_num]
+ sorted_fist_part_point, sorted_fist_part_direction = sort_part_with_direction(
+ first_part_point, first_point_direction)
+
+ last_part_point = sorted_point[middle_num:]
+ last_point_direction = sorted_direction[middle_num:]
+ sorted_last_part_point, sorted_last_part_direction = sort_part_with_direction(
+ last_part_point, last_point_direction)
+ sorted_point = sorted_fist_part_point + sorted_last_part_point
+ sorted_direction = sorted_fist_part_direction + sorted_last_part_direction
+
+ return sorted_point
diff --git a/ppocr/utils/e2e_utils/extract_textpoint.py b/ppocr/utils/e2e_utils/extract_textpoint_slow.py
similarity index 88%
rename from ppocr/utils/e2e_utils/extract_textpoint.py
rename to ppocr/utils/e2e_utils/extract_textpoint_slow.py
index 975ca16174f2ee1c7f985a5eb9ae1ec66aa7ca28..db0c30e67bea472da6c7ed5176b1c70f0ab1cbc6 100644
--- a/ppocr/utils/e2e_utils/extract_textpoint.py
+++ b/ppocr/utils/e2e_utils/extract_textpoint_slow.py
@@ -35,6 +35,64 @@ def get_dict(character_dict_path):
return dict_character
+def point_pair2poly(point_pair_list):
+ """
+ Transfer vertical point_pairs into poly point in clockwise.
+ """
+ pair_length_list = []
+ for point_pair in point_pair_list:
+ pair_length = np.linalg.norm(point_pair[0] - point_pair[1])
+ pair_length_list.append(pair_length)
+ pair_length_list = np.array(pair_length_list)
+ pair_info = (pair_length_list.max(), pair_length_list.min(),
+ pair_length_list.mean())
+
+ point_num = len(point_pair_list) * 2
+ point_list = [0] * point_num
+ for idx, point_pair in enumerate(point_pair_list):
+ point_list[idx] = point_pair[0]
+ point_list[point_num - 1 - idx] = point_pair[1]
+ return np.array(point_list).reshape(-1, 2), pair_info
+
+
+def shrink_quad_along_width(quad, begin_width_ratio=0., end_width_ratio=1.):
+ """
+ Generate shrink_quad_along_width.
+ """
+ ratio_pair = np.array(
+ [[begin_width_ratio], [end_width_ratio]], dtype=np.float32)
+ p0_1 = quad[0] + (quad[1] - quad[0]) * ratio_pair
+ p3_2 = quad[3] + (quad[2] - quad[3]) * ratio_pair
+ return np.array([p0_1[0], p0_1[1], p3_2[1], p3_2[0]])
+
+
+def expand_poly_along_width(poly, shrink_ratio_of_width=0.3):
+ """
+ expand poly along width.
+ """
+ point_num = poly.shape[0]
+ left_quad = np.array(
+ [poly[0], poly[1], poly[-2], poly[-1]], dtype=np.float32)
+ left_ratio = -shrink_ratio_of_width * np.linalg.norm(left_quad[0] - left_quad[3]) / \
+ (np.linalg.norm(left_quad[0] - left_quad[1]) + 1e-6)
+ left_quad_expand = shrink_quad_along_width(left_quad, left_ratio, 1.0)
+ right_quad = np.array(
+ [
+ poly[point_num // 2 - 2], poly[point_num // 2 - 1],
+ poly[point_num // 2], poly[point_num // 2 + 1]
+ ],
+ dtype=np.float32)
+ right_ratio = 1.0 + \
+ shrink_ratio_of_width * np.linalg.norm(right_quad[0] - right_quad[3]) / \
+ (np.linalg.norm(right_quad[0] - right_quad[1]) + 1e-6)
+ right_quad_expand = shrink_quad_along_width(right_quad, 0.0, right_ratio)
+ poly[0] = left_quad_expand[0]
+ poly[-1] = left_quad_expand[-1]
+ poly[point_num // 2 - 1] = right_quad_expand[1]
+ poly[point_num // 2] = right_quad_expand[2]
+ return poly
+
+
def softmax(logits):
"""
logits: N x d
@@ -399,13 +457,13 @@ def generate_pivot_list_horizontal(p_score,
return center_pos_yxs, end_points_yxs
-def generate_pivot_list(p_score,
- p_char_maps,
- f_direction,
- score_thresh=0.5,
- is_backbone=False,
- is_curved=True,
- image_id=0):
+def generate_pivot_list_slow(p_score,
+ p_char_maps,
+ f_direction,
+ score_thresh=0.5,
+ is_backbone=False,
+ is_curved=True,
+ image_id=0):
"""
Warp all the function together.
"""
diff --git a/ppocr/utils/e2e_utils/pgnet_pp_utils.py b/ppocr/utils/e2e_utils/pgnet_pp_utils.py
new file mode 100644
index 0000000000000000000000000000000000000000..64bfd372cc75ab533ce3ef46216a33345dae40c4
--- /dev/null
+++ b/ppocr/utils/e2e_utils/pgnet_pp_utils.py
@@ -0,0 +1,181 @@
+# Copyright (c) 2021 PaddlePaddle Authors. All Rights Reserved.
+#
+# Licensed under the Apache License, Version 2.0 (the "License");
+# you may not use this file except in compliance with the License.
+# You may obtain a copy of the License at
+#
+# http://www.apache.org/licenses/LICENSE-2.0
+#
+# Unless required by applicable law or agreed to in writing, software
+# distributed under the License is distributed on an "AS IS" BASIS,
+# WITHOUT WARRANTIES OR CONDITIONS OF ANY KIND, either express or implied.
+# See the License for the specific language governing permissions and
+# limitations under the License.
+
+from __future__ import absolute_import
+from __future__ import division
+from __future__ import print_function
+import paddle
+import os
+import sys
+
+__dir__ = os.path.dirname(__file__)
+sys.path.append(__dir__)
+sys.path.append(os.path.join(__dir__, '..'))
+from extract_textpoint_slow import *
+from extract_textpoint_fast import generate_pivot_list_fast, restore_poly
+
+
+class PGNet_PostProcess(object):
+ # two different post-process
+ def __init__(self, character_dict_path, valid_set, score_thresh, outs_dict,
+ shape_list):
+ self.Lexicon_Table = get_dict(character_dict_path)
+ self.valid_set = valid_set
+ self.score_thresh = score_thresh
+ self.outs_dict = outs_dict
+ self.shape_list = shape_list
+
+ def pg_postprocess_fast(self):
+ p_score = self.outs_dict['f_score']
+ p_border = self.outs_dict['f_border']
+ p_char = self.outs_dict['f_char']
+ p_direction = self.outs_dict['f_direction']
+ if isinstance(p_score, paddle.Tensor):
+ p_score = p_score[0].numpy()
+ p_border = p_border[0].numpy()
+ p_direction = p_direction[0].numpy()
+ p_char = p_char[0].numpy()
+ else:
+ p_score = p_score[0]
+ p_border = p_border[0]
+ p_direction = p_direction[0]
+ p_char = p_char[0]
+
+ src_h, src_w, ratio_h, ratio_w = self.shape_list[0]
+ instance_yxs_list, seq_strs = generate_pivot_list_fast(
+ p_score,
+ p_char,
+ p_direction,
+ self.Lexicon_Table,
+ score_thresh=self.score_thresh)
+ poly_list, keep_str_list = restore_poly(instance_yxs_list, seq_strs,
+ p_border, ratio_w, ratio_h,
+ src_w, src_h, self.valid_set)
+ data = {
+ 'points': poly_list,
+ 'strs': keep_str_list,
+ }
+ return data
+
+ def pg_postprocess_slow(self):
+ p_score = self.outs_dict['f_score']
+ p_border = self.outs_dict['f_border']
+ p_char = self.outs_dict['f_char']
+ p_direction = self.outs_dict['f_direction']
+ if isinstance(p_score, paddle.Tensor):
+ p_score = p_score[0].numpy()
+ p_border = p_border[0].numpy()
+ p_direction = p_direction[0].numpy()
+ p_char = p_char[0].numpy()
+ else:
+ p_score = p_score[0]
+ p_border = p_border[0]
+ p_direction = p_direction[0]
+ p_char = p_char[0]
+ src_h, src_w, ratio_h, ratio_w = self.shape_list[0]
+ is_curved = self.valid_set == "totaltext"
+ instance_yxs_list = generate_pivot_list_slow(
+ p_score,
+ p_char,
+ p_direction,
+ score_thresh=self.score_thresh,
+ is_backbone=True,
+ is_curved=is_curved)
+ p_char = paddle.to_tensor(np.expand_dims(p_char, axis=0))
+ char_seq_idx_set = []
+ for i in range(len(instance_yxs_list)):
+ gather_info_lod = paddle.to_tensor(instance_yxs_list[i])
+ f_char_map = paddle.transpose(p_char, [0, 2, 3, 1])
+ feature_seq = paddle.gather_nd(f_char_map, gather_info_lod)
+ feature_seq = np.expand_dims(feature_seq.numpy(), axis=0)
+ feature_len = [len(feature_seq[0])]
+ featyre_seq = paddle.to_tensor(feature_seq)
+ feature_len = np.array([feature_len]).astype(np.int64)
+ length = paddle.to_tensor(feature_len)
+ seq_pred = paddle.fluid.layers.ctc_greedy_decoder(
+ input=featyre_seq, blank=36, input_length=length)
+ seq_pred_str = seq_pred[0].numpy().tolist()[0]
+ seq_len = seq_pred[1].numpy()[0][0]
+ temp_t = []
+ for c in seq_pred_str[:seq_len]:
+ temp_t.append(c)
+ char_seq_idx_set.append(temp_t)
+ seq_strs = []
+ for char_idx_set in char_seq_idx_set:
+ pr_str = ''.join([self.Lexicon_Table[pos] for pos in char_idx_set])
+ seq_strs.append(pr_str)
+ poly_list = []
+ keep_str_list = []
+ all_point_list = []
+ all_point_pair_list = []
+ for yx_center_line, keep_str in zip(instance_yxs_list, seq_strs):
+ if len(yx_center_line) == 1:
+ yx_center_line.append(yx_center_line[-1])
+
+ offset_expand = 1.0
+ if self.valid_set == 'totaltext':
+ offset_expand = 1.2
+
+ point_pair_list = []
+ for batch_id, y, x in yx_center_line:
+ offset = p_border[:, y, x].reshape(2, 2)
+ if offset_expand != 1.0:
+ offset_length = np.linalg.norm(
+ offset, axis=1, keepdims=True)
+ expand_length = np.clip(
+ offset_length * (offset_expand - 1),
+ a_min=0.5,
+ a_max=3.0)
+ offset_detal = offset / offset_length * expand_length
+ offset = offset + offset_detal
+ ori_yx = np.array([y, x], dtype=np.float32)
+ point_pair = (ori_yx + offset)[:, ::-1] * 4.0 / np.array(
+ [ratio_w, ratio_h]).reshape(-1, 2)
+ point_pair_list.append(point_pair)
+
+ all_point_list.append([
+ int(round(x * 4.0 / ratio_w)),
+ int(round(y * 4.0 / ratio_h))
+ ])
+ all_point_pair_list.append(point_pair.round().astype(np.int32)
+ .tolist())
+
+ detected_poly, pair_length_info = point_pair2poly(point_pair_list)
+ detected_poly = expand_poly_along_width(
+ detected_poly, shrink_ratio_of_width=0.2)
+ detected_poly[:, 0] = np.clip(
+ detected_poly[:, 0], a_min=0, a_max=src_w)
+ detected_poly[:, 1] = np.clip(
+ detected_poly[:, 1], a_min=0, a_max=src_h)
+
+ if len(keep_str) < 2:
+ continue
+
+ keep_str_list.append(keep_str)
+ detected_poly = np.round(detected_poly).astype('int32')
+ if self.valid_set == 'partvgg':
+ middle_point = len(detected_poly) // 2
+ detected_poly = detected_poly[
+ [0, middle_point - 1, middle_point, -1], :]
+ poly_list.append(detected_poly)
+ elif self.valid_set == 'totaltext':
+ poly_list.append(detected_poly)
+ else:
+ print('--> Not supported format.')
+ exit(-1)
+ data = {
+ 'points': poly_list,
+ 'strs': keep_str_list,
+ }
+ return data
diff --git a/ppocr/utils/en_dict.txt b/ppocr/utils/en_dict.txt
new file mode 100644
index 0000000000000000000000000000000000000000..7677d31b9d3f08eef2823c2cf051beeab1f0470b
--- /dev/null
+++ b/ppocr/utils/en_dict.txt
@@ -0,0 +1,95 @@
+0
+1
+2
+3
+4
+5
+6
+7
+8
+9
+:
+;
+<
+=
+>
+?
+@
+A
+B
+C
+D
+E
+F
+G
+H
+I
+J
+K
+L
+M
+N
+O
+P
+Q
+R
+S
+T
+U
+V
+W
+X
+Y
+Z
+[
+\
+]
+^
+_
+`
+a
+b
+c
+d
+e
+f
+g
+h
+i
+j
+k
+l
+m
+n
+o
+p
+q
+r
+s
+t
+u
+v
+w
+x
+y
+z
+{
+|
+}
+~
+!
+"
+#
+$
+%
+&
+'
+(
+)
+*
++
+,
+-
+.
+/
+
diff --git a/tools/infer/predict_e2e.py b/tools/infer/predict_e2e.py
index a5c57914173b7d44c9479f7bb120e4ff409b91e3..8b94f24a9ffa16ca5683795afa6392fa23c24a94 100755
--- a/tools/infer/predict_e2e.py
+++ b/tools/infer/predict_e2e.py
@@ -66,6 +66,7 @@ class TextE2E(object):
postprocess_params["score_thresh"] = args.e2e_pgnet_score_thresh
postprocess_params["character_dict_path"] = args.e2e_char_dict_path
postprocess_params["valid_set"] = args.e2e_pgnet_valid_set
+ postprocess_params["mode"] = args.e2e_pgnet_mode
self.e2e_pgnet_polygon = args.e2e_pgnet_polygon
else:
logger.info("unknown e2e_algorithm:{}".format(self.e2e_algorithm))
diff --git a/tools/infer/utility.py b/tools/infer/utility.py
index b273eaf3258421d5c5c30c132f99e78f9f0999ba..9fa51d80b41ae80abe206ee779accd44d49cebba 100755
--- a/tools/infer/utility.py
+++ b/tools/infer/utility.py
@@ -86,6 +86,7 @@ def parse_args():
"--e2e_char_dict_path", type=str, default="./ppocr/utils/ic15_dict.txt")
parser.add_argument("--e2e_pgnet_valid_set", type=str, default='totaltext')
parser.add_argument("--e2e_pgnet_polygon", type=bool, default=True)
+ parser.add_argument("--e2e_pgnet_mode", type=str, default='fast')
# params for text classifier
parser.add_argument("--use_angle_cls", type=str2bool, default=False)