diff --git a/PPOCRLabel/README.md b/PPOCRLabel/README.md
index 9c483e1feff5a07467f7aa90343391888004bce7..8a3914e55c94d5477346ad86342dc6c4e0f3f9ed 100644
--- a/PPOCRLabel/README.md
+++ b/PPOCRLabel/README.md
@@ -103,7 +103,7 @@ python PPOCRLabel.py --kie True # [KIE mode] for [detection + recognition + keyw
```
#### 1.2.3 Build and Install the Whl Package Locally
-Compile and install a new whl package, where 1.0.2 is the version number, you can specify the new version in 'setup.py'.
+Compile and install a new whl package, where 2.1.2 is the version number, you can specify the new version in 'setup.py'.
```bash
cd ./PPOCRLabel
python3 setup.py bdist_wheel
diff --git a/PPOCRLabel/README_ch.md b/PPOCRLabel/README_ch.md
index 784ab0985c4f83dcf5e03b0f5752d9825dd3a031..91214dff6271635de26d3e7cfa2e36db84c9e038 100644
--- a/PPOCRLabel/README_ch.md
+++ b/PPOCRLabel/README_ch.md
@@ -101,7 +101,7 @@ python PPOCRLabel.py --lang ch
#### 1.2.3 本地构建whl包并安装
-编译与安装新的whl包,其中1.0.2为版本号,可在 `setup.py` 中指定新版本。
+编译与安装新的whl包,其中2.1.2为版本号,可在 `setup.py` 中指定新版本。
```bash
cd ./PPOCRLabel
diff --git "a/applications/\344\270\255\346\226\207\350\241\250\346\240\274\350\257\206\345\210\253.md" "b/applications/\344\270\255\346\226\207\350\241\250\346\240\274\350\257\206\345\210\253.md"
new file mode 100644
index 0000000000000000000000000000000000000000..af7cc96b70410c614ef39e91c229d705c8bd400a
--- /dev/null
+++ "b/applications/\344\270\255\346\226\207\350\241\250\346\240\274\350\257\206\345\210\253.md"
@@ -0,0 +1,472 @@
+# 智能运营:通用中文表格识别
+
+- [1. 背景介绍](#1-背景介绍)
+- [2. 中文表格识别](#2-中文表格识别)
+- [2.1 环境准备](#21-环境准备)
+- [2.2 准备数据集](#22-准备数据集)
+ - [2.2.1 划分训练测试集](#221-划分训练测试集)
+ - [2.2.2 查看数据集](#222-查看数据集)
+- [2.3 训练](#23-训练)
+- [2.4 验证](#24-验证)
+- [2.5 训练引擎推理](#25-训练引擎推理)
+- [2.6 模型导出](#26-模型导出)
+- [2.7 预测引擎推理](#27-预测引擎推理)
+- [2.8 表格识别](#28-表格识别)
+- [3. 表格属性识别](#3-表格属性识别)
+- [3.1 代码、环境、数据准备](#31-代码环境数据准备)
+ - [3.1.1 代码准备](#311-代码准备)
+ - [3.1.2 环境准备](#312-环境准备)
+ - [3.1.3 数据准备](#313-数据准备)
+- [3.2 表格属性识别训练](#32-表格属性识别训练)
+- [3.3 表格属性识别推理和部署](#33-表格属性识别推理和部署)
+ - [3.3.1 模型转换](#331-模型转换)
+ - [3.3.2 模型推理](#332-模型推理)
+
+## 1. 背景介绍
+
+中文表格识别在金融行业有着广泛的应用,如保险理赔、财报分析和信息录入等领域。当前,金融行业的表格识别主要以手动录入为主,开发一种自动表格识别成为丞待解决的问题。
+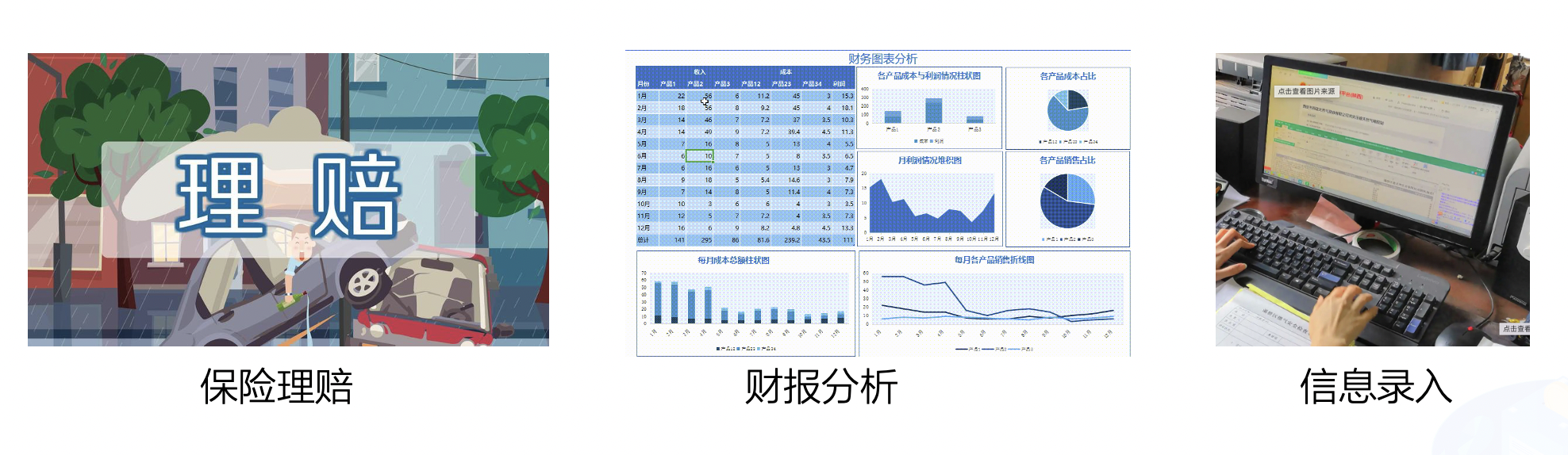
+
+
+在金融行业中,表格图像主要有清单类的单元格密集型表格,申请表类的大单元格表格,拍照表格和倾斜表格四种主要形式。
+
+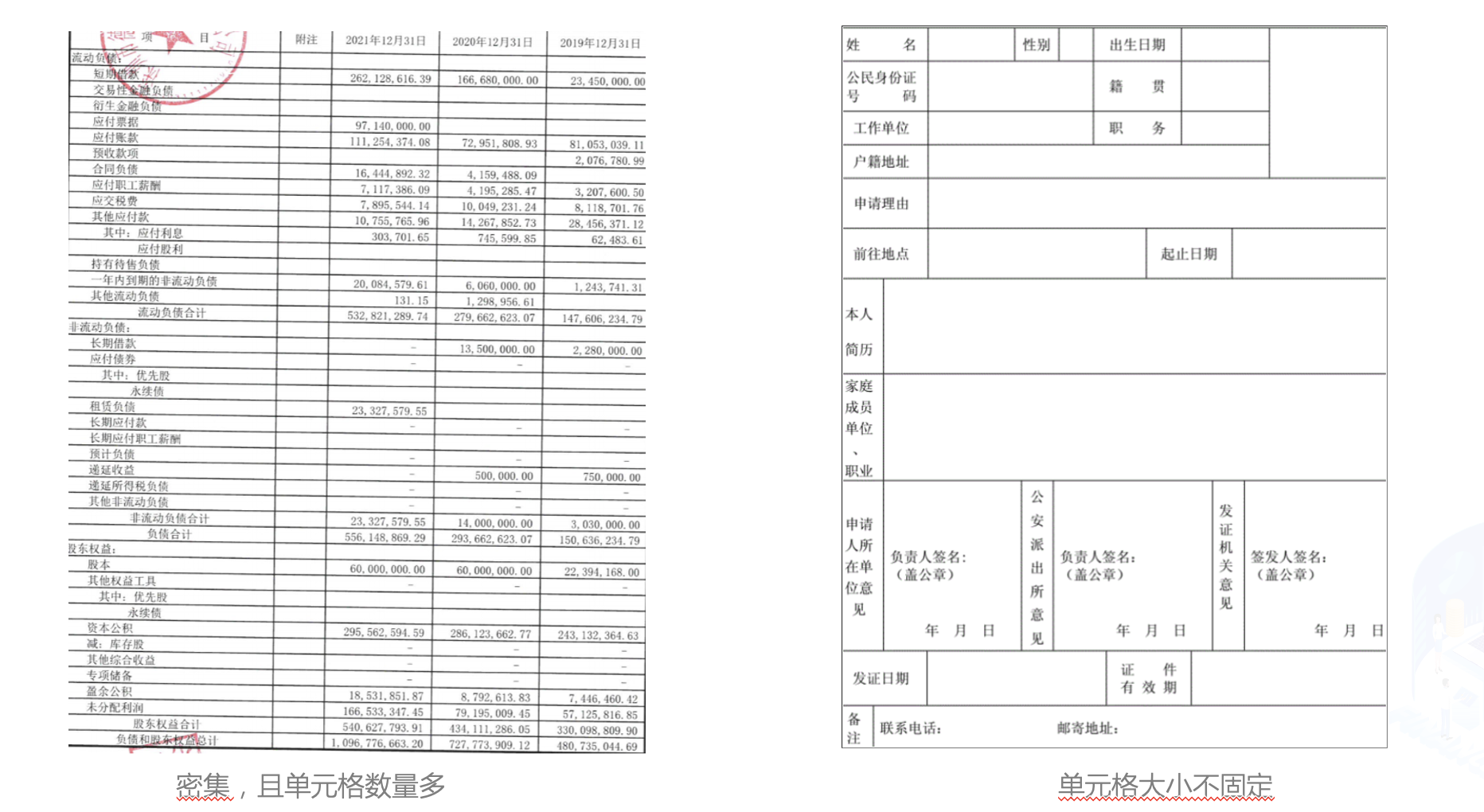
+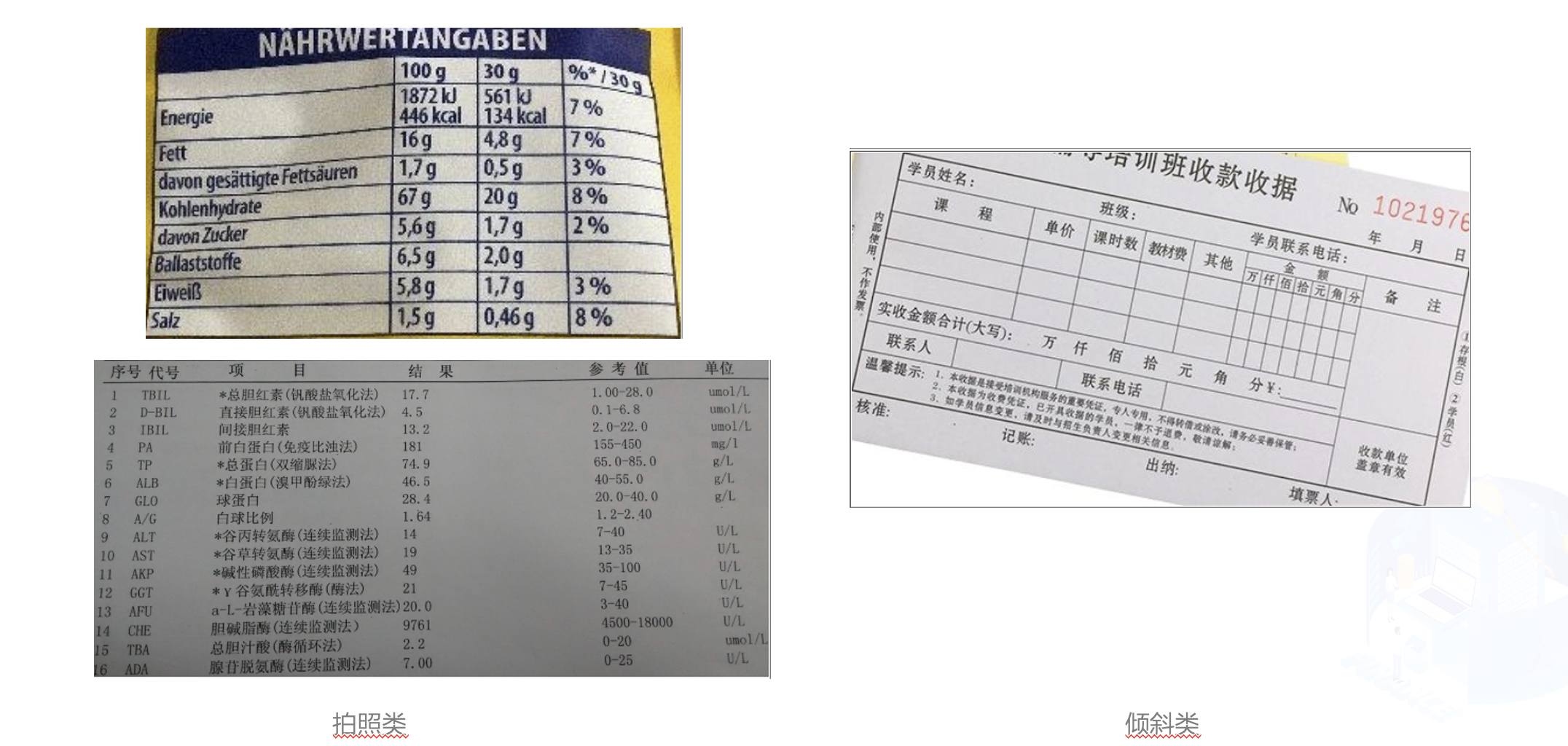
+
+
+当前的表格识别算法不能很好的处理这些场景下的表格图像。在本例中,我们使用PP-Structurev2最新发布的表格识别模型SLANet来演示如何进行中文表格是识别。同时,为了方便作业流程,我们使用表格属性识别模型对表格图像的属性进行识别,对表格的难易程度进行判断,加快人工进行校对速度。
+
+本项目AI Studio链接:https://aistudio.baidu.com/aistudio/projectdetail/4588067
+
+## 2. 中文表格识别
+### 2.1 环境准备
+
+
+```python
+# 下载PaddleOCR代码
+! git clone -b dygraph https://gitee.com/paddlepaddle/PaddleOCR
+```
+
+
+```python
+# 安装PaddleOCR环境
+! pip install -r PaddleOCR/requirements.txt --force-reinstall
+! pip install protobuf==3.19
+```
+
+### 2.2 准备数据集
+
+本例中使用的数据集采用表格[生成工具](https://github.com/WenmuZhou/TableGeneration)制作。
+
+使用如下命令对数据集进行解压,并查看数据集大小
+
+
+```python
+! cd data/data165849 && tar -xf table_gen_dataset.tar && cd -
+! wc -l data/data165849/table_gen_dataset/gt.txt
+```
+
+#### 2.2.1 划分训练测试集
+
+使用下述命令将数据集划分为训练集和测试集, 这里将90%划分为训练集,10%划分为测试集
+
+
+```python
+import random
+with open('/home/aistudio/data/data165849/table_gen_dataset/gt.txt') as f:
+ lines = f.readlines()
+random.shuffle(lines)
+train_len = int(len(lines)*0.9)
+train_list = lines[:train_len]
+val_list = lines[train_len:]
+
+# 保存结果
+with open('/home/aistudio/train.txt','w',encoding='utf-8') as f:
+ f.writelines(train_list)
+with open('/home/aistudio/val.txt','w',encoding='utf-8') as f:
+ f.writelines(val_list)
+```
+
+划分完成后,数据集信息如下
+
+|类型|数量|图片地址|标注文件路径|
+|---|---|---|---|
+|训练集|18000|/home/aistudio/data/data165849/table_gen_dataset|/home/aistudio/train.txt|
+|测试集|2000|/home/aistudio/data/data165849/table_gen_dataset|/home/aistudio/val.txt|
+
+#### 2.2.2 查看数据集
+
+
+```python
+import cv2
+import os, json
+import numpy as np
+from matplotlib import pyplot as plt
+%matplotlib inline
+
+def parse_line(data_dir, line):
+ data_line = line.strip("\n")
+ info = json.loads(data_line)
+ file_name = info['filename']
+ cells = info['html']['cells'].copy()
+ structure = info['html']['structure']['tokens'].copy()
+
+ img_path = os.path.join(data_dir, file_name)
+ if not os.path.exists(img_path):
+ print(img_path)
+ return None
+ data = {
+ 'img_path': img_path,
+ 'cells': cells,
+ 'structure': structure,
+ 'file_name': file_name
+ }
+ return data
+
+def draw_bbox(img_path, points, color=(255, 0, 0), thickness=2):
+ if isinstance(img_path, str):
+ img_path = cv2.imread(img_path)
+ img_path = img_path.copy()
+ for point in points:
+ cv2.polylines(img_path, [point.astype(int)], True, color, thickness)
+ return img_path
+
+
+def rebuild_html(data):
+ html_code = data['structure']
+ cells = data['cells']
+ to_insert = [i for i, tag in enumerate(html_code) if tag in ('
', '>')]
+
+ for i, cell in zip(to_insert[::-1], cells[::-1]):
+ if cell['tokens']:
+ text = ''.join(cell['tokens'])
+ # skip empty text
+ sp_char_list = ['', ' ', '\u2028', ' ', '', ' ']
+ text_remove_style = skip_char(text, sp_char_list)
+ if len(text_remove_style) == 0:
+ continue
+ html_code.insert(i + 1, text)
+
+ html_code = ''.join(html_code)
+ return html_code
+
+
+def skip_char(text, sp_char_list):
+ """
+ skip empty cell
+ @param text: text in cell
+ @param sp_char_list: style char and special code
+ @return:
+ """
+ for sp_char in sp_char_list:
+ text = text.replace(sp_char, '')
+ return text
+
+save_dir = '/home/aistudio/vis'
+os.makedirs(save_dir, exist_ok=True)
+image_dir = '/home/aistudio/data/data165849/'
+html_str = ''
+
+# 解析标注信息并还原html表格
+data = parse_line(image_dir, val_list[0])
+
+img = cv2.imread(data['img_path'])
+img_name = ''.join(os.path.basename(data['file_name']).split('.')[:-1])
+img_save_name = os.path.join(save_dir, img_name)
+boxes = [np.array(x['bbox']) for x in data['cells']]
+show_img = draw_bbox(data['img_path'], boxes)
+cv2.imwrite(img_save_name + '_show.jpg', show_img)
+
+html = rebuild_html(data)
+html_str += html
+html_str += '
'
+
+# 显示标注的html字符串
+from IPython.core.display import display, HTML
+display(HTML(html_str))
+# 显示单元格坐标
+plt.figure(figsize=(15,15))
+plt.imshow(show_img)
+plt.show()
+```
+
+### 2.3 训练
+
+这里选用PP-Structurev2中的表格识别模型[SLANet](https://github.com/PaddlePaddle/PaddleOCR/blob/dygraph/configs/table/SLANet.yml)
+
+SLANet是PP-Structurev2全新推出的表格识别模型,相比PP-Structurev1中TableRec-RARE,在速度不变的情况下精度提升4.7%。TEDS提升2%
+
+
+|算法|Acc|[TEDS(Tree-Edit-Distance-based Similarity)](https://github.com/ibm-aur-nlp/PubTabNet/tree/master/src)|Speed|
+| --- | --- | --- | ---|
+| EDD[2] |x| 88.3% |x|
+| TableRec-RARE(ours) | 71.73%| 93.88% |779ms|
+| SLANet(ours) | 76.31%| 95.89%|766ms|
+
+进行训练之前先使用如下命令下载预训练模型
+
+
+```python
+# 进入PaddleOCR工作目录
+os.chdir('/home/aistudio/PaddleOCR')
+# 下载英文预训练模型
+! wget -nc -P ./pretrain_models/ https://paddleocr.bj.bcebos.com/ppstructure/models/slanet/en_ppstructure_mobile_v2.0_SLANet_train.tar --no-check-certificate
+! cd ./pretrain_models/ && tar xf en_ppstructure_mobile_v2.0_SLANet_train.tar && cd ../
+```
+
+使用如下命令即可启动训练,需要修改的配置有
+
+|字段|修改值|含义|
+|---|---|---|
+|Global.pretrained_model|./pretrain_models/en_ppstructure_mobile_v2.0_SLANet_train/best_accuracy.pdparams|指向英文表格预训练模型地址|
+|Global.eval_batch_step|562|模型多少step评估一次,一般设置为一个epoch总的step数|
+|Optimizer.lr.name|Const|学习率衰减器 |
+|Optimizer.lr.learning_rate|0.0005|学习率设为之前的0.05倍 |
+|Train.dataset.data_dir|/home/aistudio/data/data165849|指向训练集图片存放目录 |
+|Train.dataset.label_file_list|/home/aistudio/data/data165849/table_gen_dataset/train.txt|指向训练集标注文件 |
+|Train.loader.batch_size_per_card|32|训练时每张卡的batch_size |
+|Train.loader.num_workers|1|训练集多进程数据读取的进程数,在aistudio中需要设为1 |
+|Eval.dataset.data_dir|/home/aistudio/data/data165849|指向测试集图片存放目录 |
+|Eval.dataset.label_file_list|/home/aistudio/data/data165849/table_gen_dataset/val.txt|指向测试集标注文件 |
+|Eval.loader.batch_size_per_card|32|测试时每张卡的batch_size |
+|Eval.loader.num_workers|1|测试集多进程数据读取的进程数,在aistudio中需要设为1 |
+
+
+已经修改好的配置存储在 `/home/aistudio/SLANet_ch.yml`
+
+
+```python
+import os
+os.chdir('/home/aistudio/PaddleOCR')
+! python3 tools/train.py -c /home/aistudio/SLANet_ch.yml
+```
+
+大约在7个epoch后达到最高精度 97.49%
+
+### 2.4 验证
+
+训练完成后,可使用如下命令在测试集上评估最优模型的精度
+
+
+```python
+! python3 tools/eval.py -c /home/aistudio/SLANet_ch.yml -o Global.checkpoints=/home/aistudio/PaddleOCR/output/SLANet_ch/best_accuracy.pdparams
+```
+
+### 2.5 训练引擎推理
+使用如下命令可使用训练引擎对单张图片进行推理
+
+
+```python
+import os;os.chdir('/home/aistudio/PaddleOCR')
+! python3 tools/infer_table.py -c /home/aistudio/SLANet_ch.yml -o Global.checkpoints=/home/aistudio/PaddleOCR/output/SLANet_ch/best_accuracy.pdparams Global.infer_img=/home/aistudio/data/data165849/table_gen_dataset/img/no_border_18298_G7XZH93DDCMATGJQ8RW2.jpg
+```
+
+
+```python
+import cv2
+from matplotlib import pyplot as plt
+%matplotlib inline
+
+# 显示原图
+show_img = cv2.imread('/home/aistudio/data/data165849/table_gen_dataset/img/no_border_18298_G7XZH93DDCMATGJQ8RW2.jpg')
+plt.figure(figsize=(15,15))
+plt.imshow(show_img)
+plt.show()
+
+# 显示预测的单元格
+show_img = cv2.imread('/home/aistudio/PaddleOCR/output/infer/no_border_18298_G7XZH93DDCMATGJQ8RW2.jpg')
+plt.figure(figsize=(15,15))
+plt.imshow(show_img)
+plt.show()
+```
+
+### 2.6 模型导出
+
+使用如下命令可将模型导出为inference模型
+
+
+```python
+! python3 tools/export_model.py -c /home/aistudio/SLANet_ch.yml -o Global.checkpoints=/home/aistudio/PaddleOCR/output/SLANet_ch/best_accuracy.pdparams Global.save_inference_dir=/home/aistudio/SLANet_ch/infer
+```
+
+### 2.7 预测引擎推理
+使用如下命令可使用预测引擎对单张图片进行推理
+
+
+
+```python
+os.chdir('/home/aistudio/PaddleOCR/ppstructure')
+! python3 table/predict_structure.py \
+ --table_model_dir=/home/aistudio/SLANet_ch/infer \
+ --table_char_dict_path=../ppocr/utils/dict/table_structure_dict.txt \
+ --image_dir=/home/aistudio/data/data165849/table_gen_dataset/img/no_border_18298_G7XZH93DDCMATGJQ8RW2.jpg \
+ --output=../output/inference
+```
+
+
+```python
+# 显示原图
+show_img = cv2.imread('/home/aistudio/data/data165849/table_gen_dataset/img/no_border_18298_G7XZH93DDCMATGJQ8RW2.jpg')
+plt.figure(figsize=(15,15))
+plt.imshow(show_img)
+plt.show()
+
+# 显示预测的单元格
+show_img = cv2.imread('/home/aistudio/PaddleOCR/output/inference/no_border_18298_G7XZH93DDCMATGJQ8RW2.jpg')
+plt.figure(figsize=(15,15))
+plt.imshow(show_img)
+plt.show()
+```
+
+### 2.8 表格识别
+
+在表格结构模型训练完成后,可结合OCR检测识别模型,对表格内容进行识别。
+
+首先下载PP-OCRv3文字检测识别模型
+
+
+```python
+# 下载PP-OCRv3文本检测识别模型并解压
+! wget -nc -P ./inference/ https://paddleocr.bj.bcebos.com/PP-OCRv3/chinese/ch_PP-OCRv3_det_slim_infer.tar --no-check-certificate
+! wget -nc -P ./inference/ https://paddleocr.bj.bcebos.com/PP-OCRv3/chinese/ch_PP-OCRv3_rec_slim_infer.tar --no-check-certificate
+! cd ./inference/ && tar xf ch_PP-OCRv3_det_slim_infer.tar && tar xf ch_PP-OCRv3_rec_slim_infer.tar && cd ../
+```
+
+模型下载完成后,使用如下命令进行表格识别
+
+
+```python
+import os;os.chdir('/home/aistudio/PaddleOCR/ppstructure')
+! python3 table/predict_table.py \
+ --det_model_dir=inference/ch_PP-OCRv3_det_slim_infer \
+ --rec_model_dir=inference/ch_PP-OCRv3_rec_slim_infer \
+ --table_model_dir=/home/aistudio/SLANet_ch/infer \
+ --rec_char_dict_path=../ppocr/utils/ppocr_keys_v1.txt \
+ --table_char_dict_path=../ppocr/utils/dict/table_structure_dict.txt \
+ --image_dir=/home/aistudio/data/data165849/table_gen_dataset/img/no_border_18298_G7XZH93DDCMATGJQ8RW2.jpg \
+ --output=../output/table
+```
+
+
+```python
+# 显示原图
+show_img = cv2.imread('/home/aistudio/data/data165849/table_gen_dataset/img/no_border_18298_G7XZH93DDCMATGJQ8RW2.jpg')
+plt.figure(figsize=(15,15))
+plt.imshow(show_img)
+plt.show()
+
+# 显示预测结果
+from IPython.core.display import display, HTML
+display(HTML('alleadersh 不贰过,推 从自己参与浙江数 。另一方 AnSha 自己越 共商共建工作协商 w.east 抓好改革试点任务 Edime ImisesElec 怀天下”。 22.26 31.61 4.30 794.94 ip Profundi :2019年12月1 Horspro 444.48 2.41 87 679.98 iehaiTrain 组长蒋蕊 Toafterdec 203.43 23.54 4 4266.62 Tyint roudlyRol 谢您的好意,我知道 ErChows 48.90 1031 6 NaFlint 一辈的 aterreclam 7823.86 9829.23 7.96 3068 家上下游企业,5 Tr 景象。当地球上的我们 Urelaw 799.62 354.96 12.98 33 赛事( uestCh 复制的业务模式并 Listicjust 9.23 92 53.22 Ca Iskole 扶贫"之名引导 Papua 7191.90 1.65 3.62 48 避讳 ir 但由于 Fficeof 0.22 6.37 7.17 3397.75 ndaTurk 百处遗址 gMa 1288.34 2053.66 2.29 885.45
'))
+```
+
+## 3. 表格属性识别
+### 3.1 代码、环境、数据准备
+#### 3.1.1 代码准备
+首先,我们需要准备训练表格属性的代码,PaddleClas集成了PULC方案,该方案可以快速获得一个在CPU上用时2ms的属性识别模型。PaddleClas代码可以clone下载得到。获取方式如下:
+
+
+
+```python
+! git clone -b develop https://gitee.com/paddlepaddle/PaddleClas
+```
+
+#### 3.1.2 环境准备
+其次,我们需要安装训练PaddleClas相关的依赖包
+
+
+```python
+! pip install -r PaddleClas/requirements.txt --force-reinstall
+! pip install protobuf==3.20.0
+```
+
+
+#### 3.1.3 数据准备
+
+最后,准备训练数据。在这里,我们一共定义了表格的6个属性,分别是表格来源、表格数量、表格颜色、表格清晰度、表格有无干扰、表格角度。其可视化如下:
+
+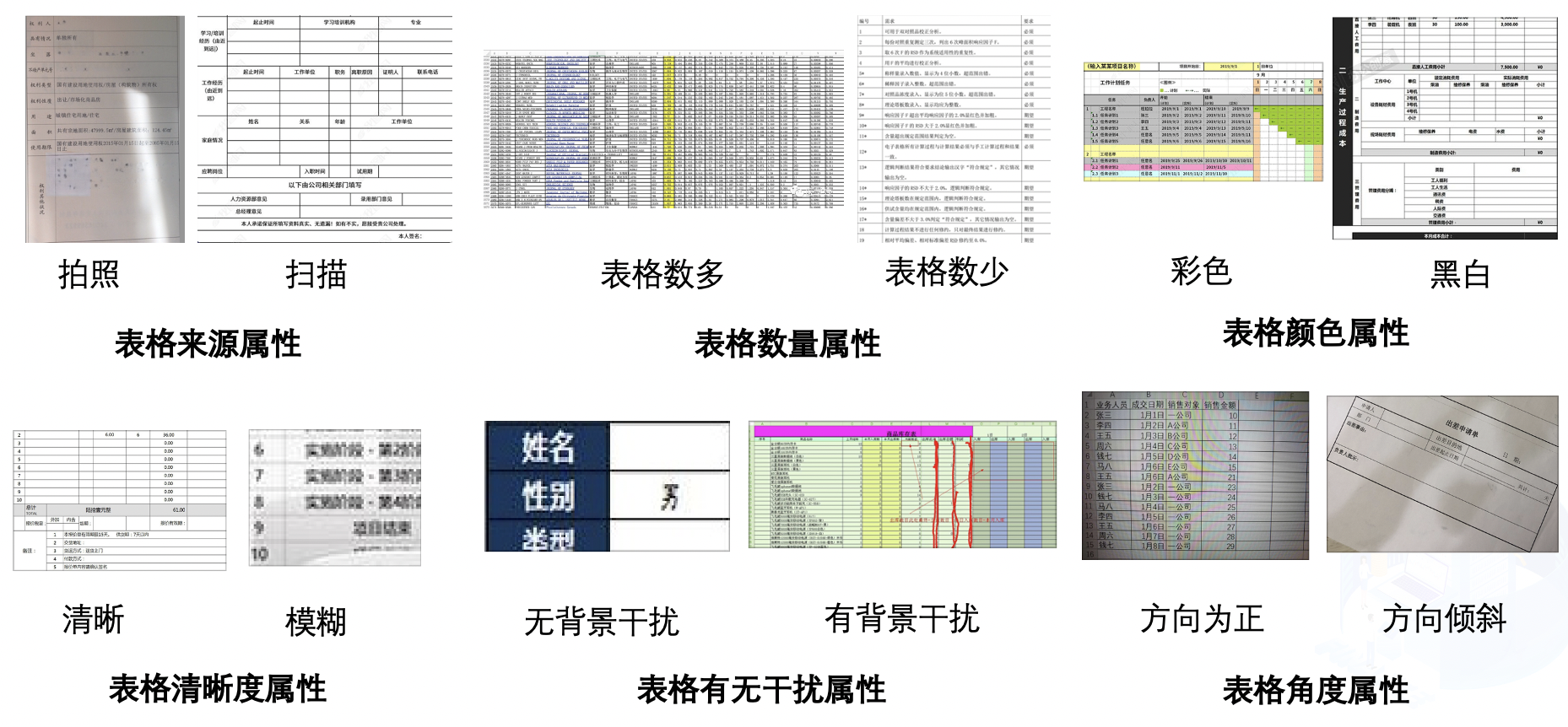
+
+这里,我们提供了一个表格属性的demo子集,可以快速迭代体验。下载方式如下:
+
+
+```python
+%cd PaddleClas/dataset
+!wget https://paddleclas.bj.bcebos.com/data/PULC/table_attribute.tar
+!tar -xf table_attribute.tar
+%cd ../PaddleClas/dataset
+%cd ../
+```
+
+### 3.2 表格属性识别训练
+表格属性训练整体pipelinie如下:
+
+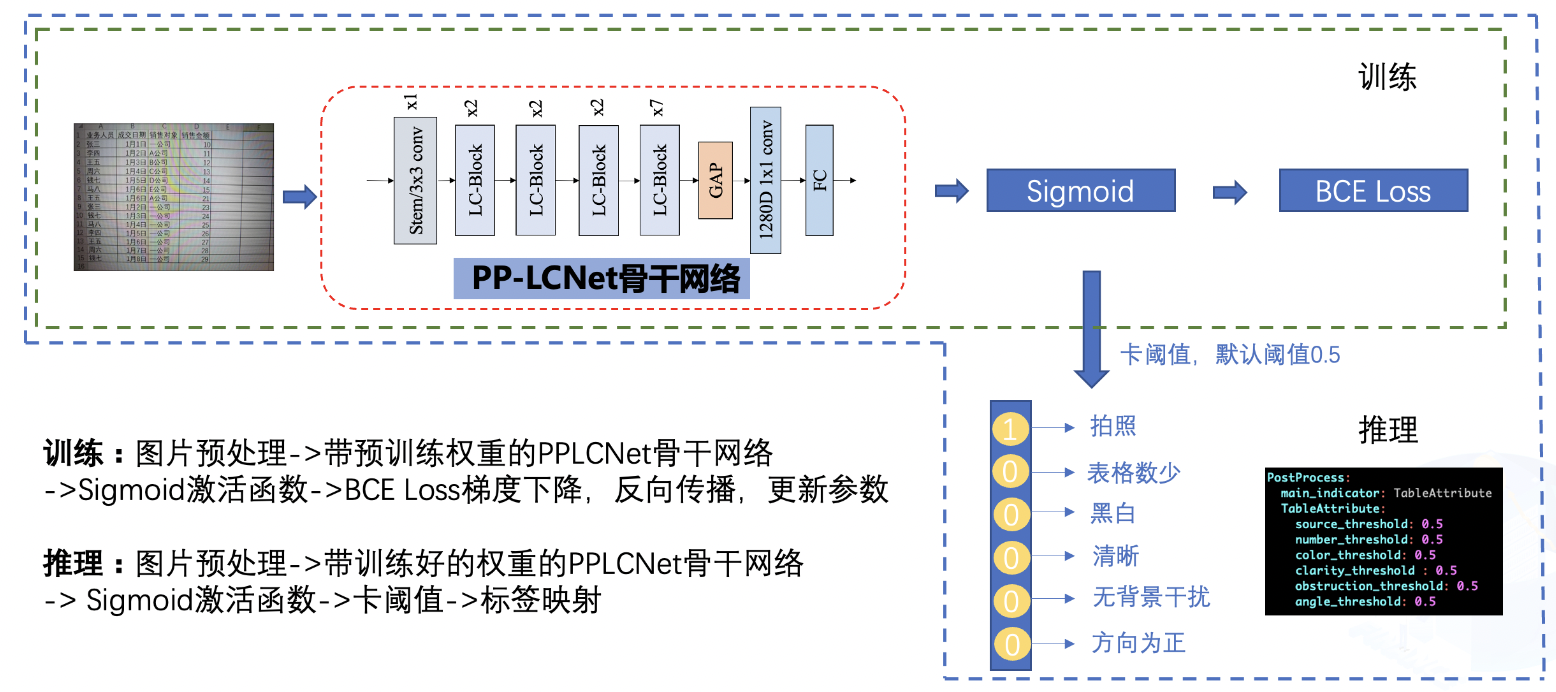
+
+1.训练过程中,图片经过预处理之后,送入到骨干网络之中,骨干网络将抽取表格图片的特征,最终该特征连接输出的FC层,FC层经过Sigmoid激活函数后和真实标签做交叉熵损失函数,优化器通过对该损失函数做梯度下降来更新骨干网络的参数,经过多轮训练后,骨干网络的参数可以对为止图片做很好的预测;
+
+2.推理过程中,图片经过预处理之后,送入到骨干网络之中,骨干网络加载学习好的权重后对该表格图片做出预测,预测的结果为一个6维向量,该向量中的每个元素反映了每个属性对应的概率值,通过对该值进一步卡阈值之后,得到最终的输出,最终的输出描述了该表格的6个属性。
+
+当准备好相关的数据之后,可以一键启动表格属性的训练,训练代码如下:
+
+
+```python
+
+!python tools/train.py -c ./ppcls/configs/PULC/table_attribute/PPLCNet_x1_0.yaml -o Global.device=cpu -o Global.epochs=10
+```
+
+### 3.3 表格属性识别推理和部署
+#### 3.3.1 模型转换
+当训练好模型之后,需要将模型转换为推理模型进行部署。转换脚本如下:
+
+
+```python
+!python tools/export_model.py -c ppcls/configs/PULC/table_attribute/PPLCNet_x1_0.yaml -o Global.pretrained_model=output/PPLCNet_x1_0/best_model
+```
+
+执行以上命令之后,会在当前目录上生成`inference`文件夹,该文件夹中保存了当前精度最高的推理模型。
+
+#### 3.3.2 模型推理
+安装推理需要的paddleclas包, 此时需要通过下载安装paddleclas的develop的whl包
+
+
+
+```python
+!pip install https://paddleclas.bj.bcebos.com/whl/paddleclas-0.0.0-py3-none-any.whl
+```
+
+进入`deploy`目录下即可对模型进行推理
+
+
+```python
+%cd deploy/
+```
+
+推理命令如下:
+
+
+```python
+!python python/predict_cls.py -c configs/PULC/table_attribute/inference_table_attribute.yaml -o Global.inference_model_dir="../inference" -o Global.infer_imgs="../dataset/table_attribute/Table_val/val_9.jpg"
+!python python/predict_cls.py -c configs/PULC/table_attribute/inference_table_attribute.yaml -o Global.inference_model_dir="../inference" -o Global.infer_imgs="../dataset/table_attribute/Table_val/val_3253.jpg"
+```
+
+推理的表格图片:
+
+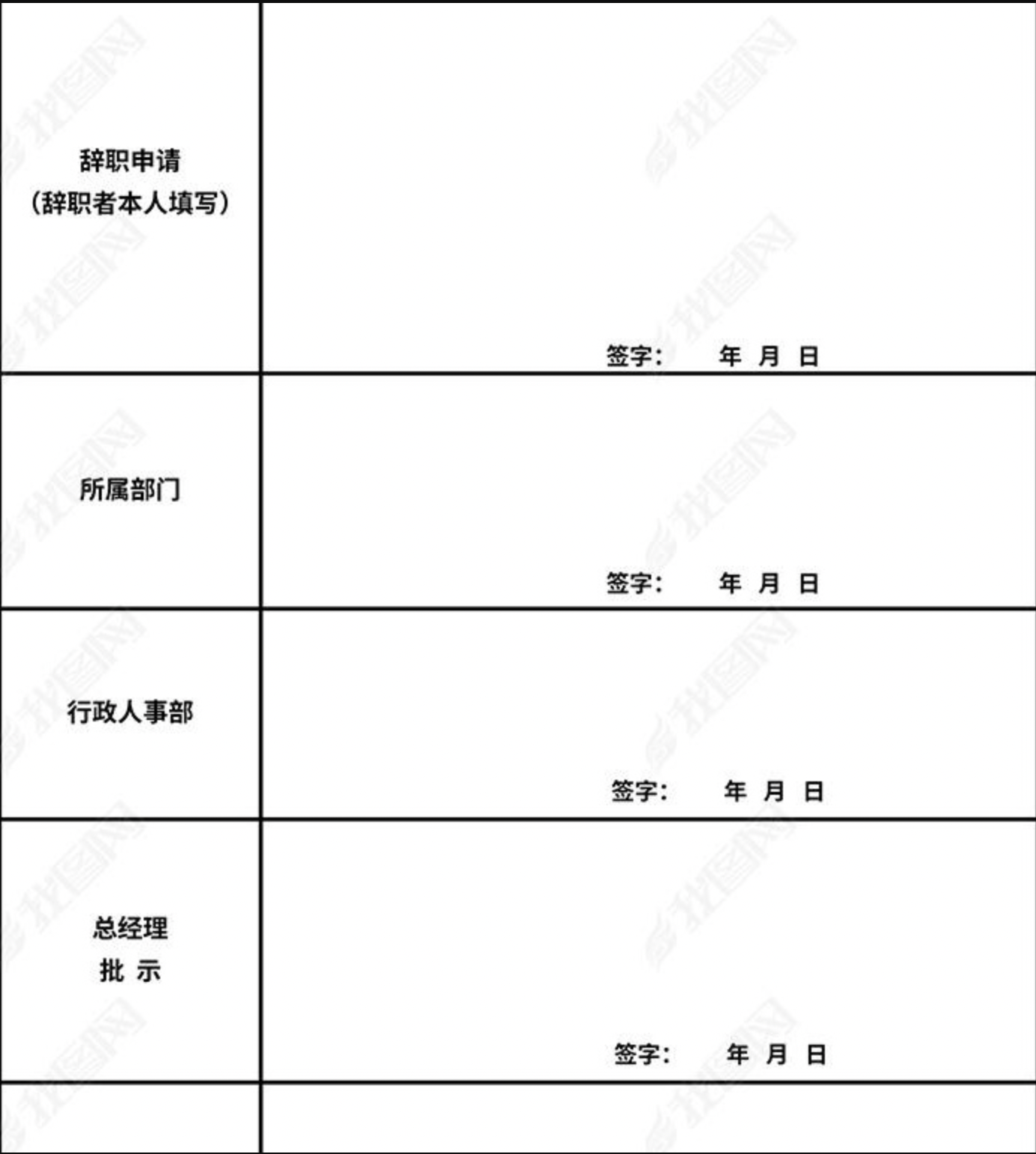
+
+预测结果如下:
+```
+val_9.jpg: {'attributes': ['Scanned', 'Little', 'Black-and-White', 'Clear', 'Without-Obstacles', 'Horizontal'], 'output': [1, 1, 1, 1, 1, 1]}
+```
+
+
+推理的表格图片:
+
+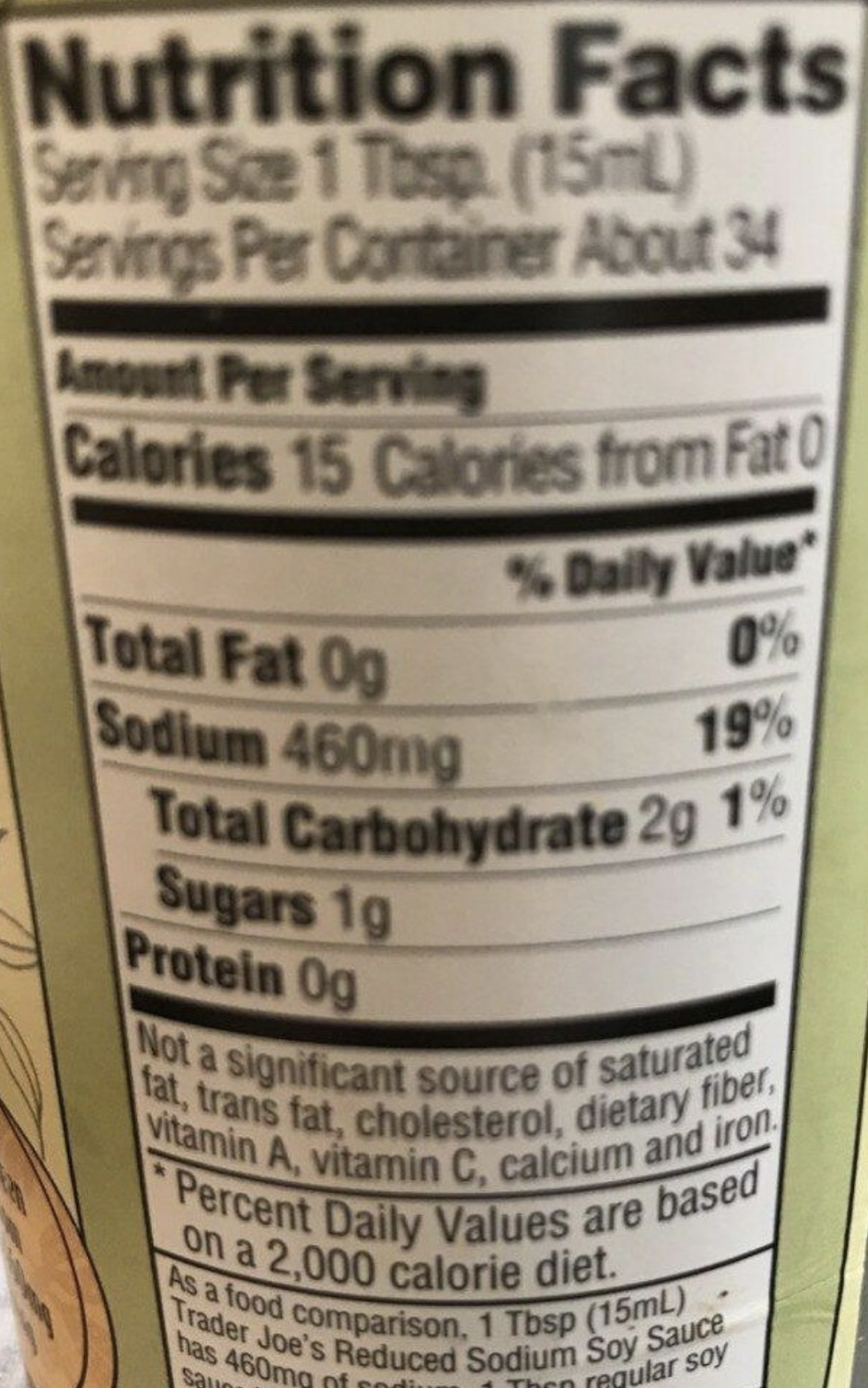
+
+预测结果如下:
+```
+val_3253.jpg: {'attributes': ['Photo', 'Little', 'Black-and-White', 'Blurry', 'Without-Obstacles', 'Tilted'], 'output': [0, 1, 1, 0, 1, 0]}
+```
+
+对比两张图片可以发现,第一张图片比较清晰,表格属性的结果也偏向于比较容易识别,我们可以更相信表格识别的结果,第二张图片比较模糊,且存在倾斜现象,表格识别可能存在错误,需要我们人工进一步校验。通过表格的属性识别能力,可以进一步将“人工”和“智能”很好的结合起来,为表格识别能力的落地的精度提供保障。
diff --git "a/applications/\345\215\260\347\253\240\345\274\257\346\233\262\346\226\207\345\255\227\350\257\206\345\210\253.md" "b/applications/\345\215\260\347\253\240\345\274\257\346\233\262\346\226\207\345\255\227\350\257\206\345\210\253.md"
new file mode 100644
index 0000000000000000000000000000000000000000..48b13ca39dccd271739dbddf17ed1ab1ed3b91ae
--- /dev/null
+++ "b/applications/\345\215\260\347\253\240\345\274\257\346\233\262\346\226\207\345\255\227\350\257\206\345\210\253.md"
@@ -0,0 +1,1034 @@
+# 印章弯曲文字识别
+
+- [1. 项目介绍](#1-----)
+- [2. 环境搭建](#2-----)
+ * [2.1 准备PaddleDetection环境](#21---paddledetection--)
+ * [2.2 准备PaddleOCR环境](#22---paddleocr--)
+- [3. 数据集准备](#3------)
+ * [3.1 数据标注](#31-----)
+ * [3.2 数据处理](#32-----)
+- [4. 印章检测实践](#4-------)
+- [5. 印章文字识别实践](#5---------)
+ * [5.1 端对端印章文字识别实践](#51------------)
+ * [5.2 两阶段印章文字识别实践](#52------------)
+ + [5.2.1 印章文字检测](#521-------)
+ + [5.2.2 印章文字识别](#522-------)
+
+
+# 1. 项目介绍
+
+弯曲文字识别在OCR任务中有着广泛的应用,比如:自然场景下的招牌,艺术文字,以及常见的印章文字识别。
+
+在本项目中,将以印章识别任务为例,介绍如何使用PaddleDetection和PaddleOCR完成印章检测和印章文字识别任务。
+
+项目难点:
+1. 缺乏训练数据
+2. 图像质量参差不齐,图像模糊,文字不清晰
+
+针对以上问题,本项目选用PaddleOCR里的PPOCRLabel工具完成数据标注。基于PaddleDetection完成印章区域检测,然后通过PaddleOCR里的端对端OCR算法和两阶段OCR算法分别完成印章文字识别任务。不同任务的精度效果如下:
+
+
+| 任务 | 训练数据数量 | 精度 |
+| -------- | - | -------- |
+| 印章检测 | 1000 | 95% |
+| 印章文字识别-端对端OCR方法 | 700 | 47% |
+| 印章文字识别-两阶段OCR方法 | 700 | 55% |
+
+点击进入 [AI Studio 项目](https://aistudio.baidu.com/aistudio/projectdetail/4586113)
+
+# 2. 环境搭建
+
+本项目需要准备PaddleDetection和PaddleOCR的项目运行环境,其中PaddleDetection用于实现印章检测任务,PaddleOCR用于实现文字识别任务
+
+
+## 2.1 准备PaddleDetection环境
+
+下载PaddleDetection代码:
+```
+!git clone https://github.com/PaddlePaddle/PaddleDetection.git
+# 如果克隆github代码较慢,请从gitee上克隆代码
+#git clone https://gitee.com/PaddlePaddle/PaddleDetection.git
+```
+
+安装PaddleDetection依赖
+```
+!cd PaddleDetection && pip install -r requirements.txt
+```
+
+## 2.2 准备PaddleOCR环境
+
+下载PaddleOCR代码:
+```
+!git clone https://github.com/PaddlePaddle/PaddleOCR.git
+# 如果克隆github代码较慢,请从gitee上克隆代码
+#git clone https://gitee.com/PaddlePaddle/PaddleOCR.git
+```
+
+安装PaddleOCR依赖
+```
+!cd PaddleOCR && git checkout dygraph && pip install -r requirements.txt
+```
+
+# 3. 数据集准备
+
+## 3.1 数据标注
+
+本项目中使用[PPOCRLabel](https://github.com/PaddlePaddle/PaddleOCR/tree/release/2.6/PPOCRLabel)工具标注印章检测数据,标注内容包括印章的位置以及印章中文字的位置和文字内容。
+
+[标注好的8000张印章数据集下载地址](https://aistudio.baidu.com/aistudio/datasetdetail/154271)
+
+注:PPOCRLabel的使用方法参考[文档](https://github.com/PaddlePaddle/PaddleOCR/tree/release/2.6/PPOCRLabel)。
+
+PPOCRlabel标注印章数据步骤:
+- 打开数据集所在文件夹
+- 按下快捷键Q进行4点(多点)标注——针对印章文本识别,
+ - 印章弯曲文字包围框采用偶数点标注(比如4点,8点,16点),按照阅读顺序,以16点标注为例,从文字左上方开始标注->到文字右上方标注8个点->到文字右下方->文字左下方8个点,一共8个点,形成包围曲线,参考下图。如果文字弯曲程度不高,为了减小标注工作量,可以采用4点、8点标注,需要注意的是,文字上下点数相同。(总点数尽量不要超过18个)
+ - 对于需要识别的印章中非弯曲文字,采用4点框标注即可
+ - 对应包围框的文字部分默认是”待识别”,需要修改为包围框内的具体文字内容
+- 快捷键W进行矩形标注——针对印章区域检测,印章检测区域保证标注框包围整个印章,包围框对应文字可以设置为'印章区域',方便后续处理。
+- 针对印章中的水平文字可以视情况考虑矩形或四点标注:保证按行标注即可。如果背景文字与印章文字比较接近,标注时尽量避开背景文字。
+- 标注完成后修改右侧文本结果,确认无误后点击下方check(或CTRL+V),确认本张图片的标注。
+- 所有图片标注完成后,在顶部菜单栏点击File -> Export Label导出label.txt。
+
+标注完成后,可视化效果如下:
+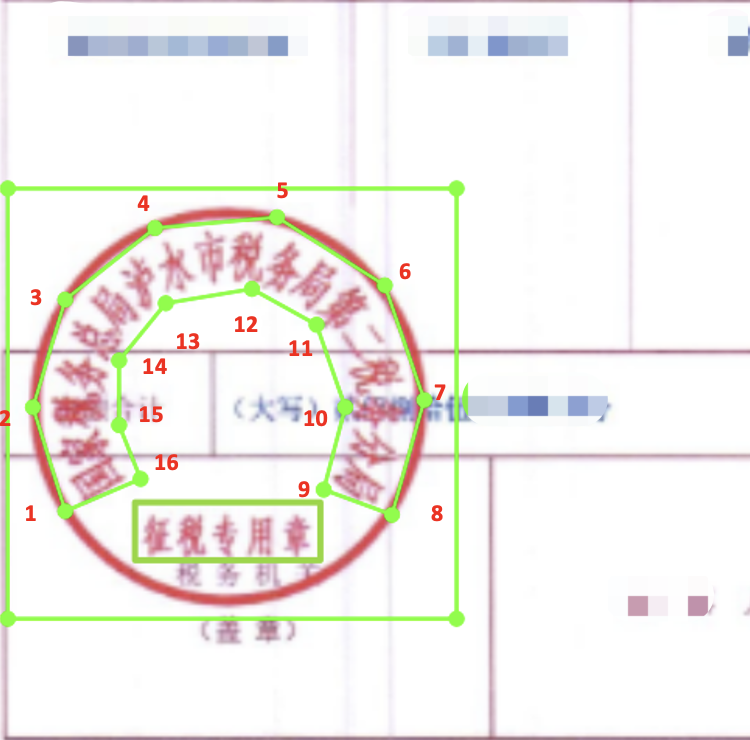
+
+数据标注完成后,标签中包含印章检测的标注和印章文字识别的标注,如下所示:
+```
+img/1.png [{"transcription": "印章区域", "points": [[87, 245], [214, 245], [214, 369], [87, 369]], "difficult": false}, {"transcription": "国家税务总局泸水市税务局第二税务分局", "points": [[110, 314], [116, 290], [131, 275], [152, 273], [170, 277], [181, 289], [186, 303], [186, 312], [201, 311], [198, 289], [189, 272], [175, 259], [152, 252], [124, 257], [100, 280], [94, 312]], "difficult": false}, {"transcription": "征税专用章", "points": [[117, 334], [183, 334], [183, 352], [117, 352]], "difficult": false}]
+```
+标注中包含表示'印章区域'的坐标和'印章文字'坐标以及文字内容。
+
+
+
+## 3.2 数据处理
+
+标注时为了方便标注,没有区分印章区域的标注框和文字区域的标注框,可以通过python代码完成标签的划分。
+
+在本项目的'/home/aistudio/work/seal_labeled_datas'目录下,存放了标注的数据示例,如下:
+
+
+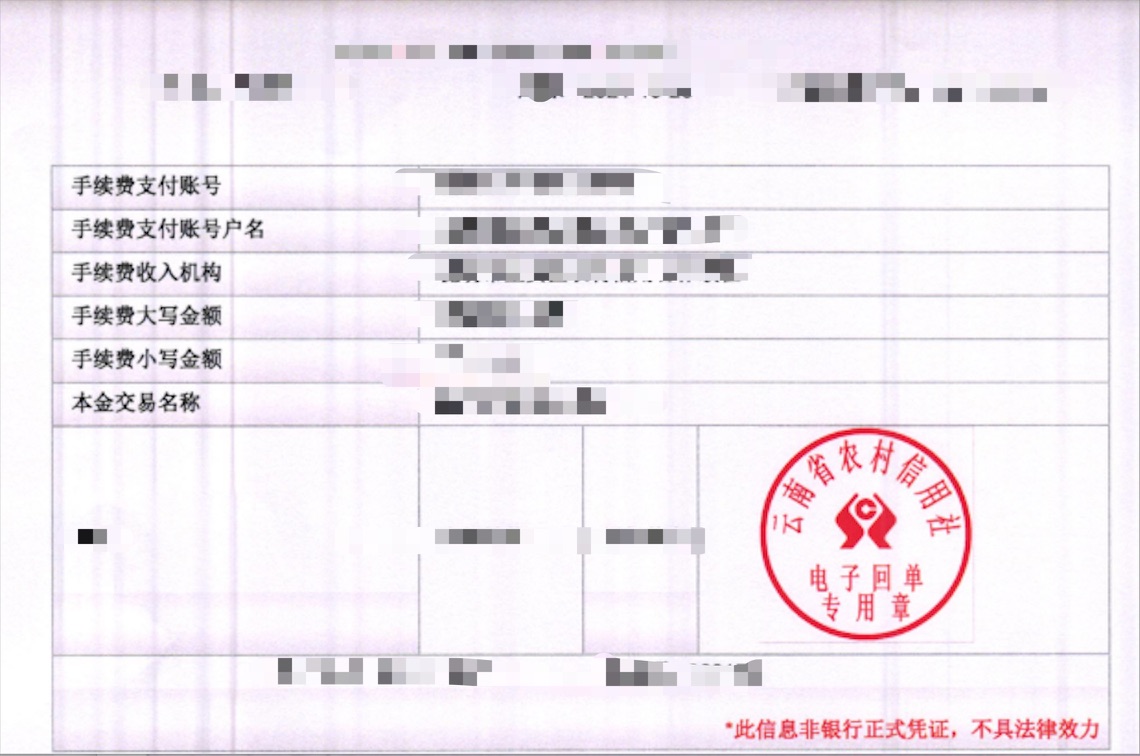
+
+标签文件'/home/aistudio/work/seal_labeled_datas/Label.txt'中的标注内容如下:
+
+```
+img/test1.png [{"transcription": "待识别", "points": [[408, 232], [537, 232], [537, 352], [408, 352]], "difficult": false}, {"transcription": "电子回单", "points": [[437, 305], [504, 305], [504, 322], [437, 322]], "difficult": false}, {"transcription": "云南省农村信用社", "points": [[417, 290], [434, 295], [438, 281], [446, 267], [455, 261], [472, 258], [489, 264], [498, 277], [502, 295], [526, 289], [518, 267], [503, 249], [475, 232], [446, 239], [429, 255], [418, 275]], "difficult": false}, {"transcription": "专用章", "points": [[437, 319], [503, 319], [503, 338], [437, 338]], "difficult": false}]
+```
+
+
+为了方便训练,我们需要通过python代码将用于训练印章检测和训练印章文字识别的标注区分开。
+
+
+```
+import numpy as np
+import json
+import cv2
+import os
+from shapely.geometry import Polygon
+
+
+def poly2box(poly):
+ xmin = np.min(np.array(poly)[:, 0])
+ ymin = np.min(np.array(poly)[:, 1])
+ xmax = np.max(np.array(poly)[:, 0])
+ ymax = np.max(np.array(poly)[:, 1])
+ return np.array([[xmin, ymin], [xmax, ymin], [xmax, ymax], [xmin, ymax]])
+
+
+def draw_text_det_res(dt_boxes, src_im, color=(255, 255, 0)):
+ for box in dt_boxes:
+ box = np.array(box).astype(np.int32).reshape(-1, 2)
+ cv2.polylines(src_im, [box], True, color=color, thickness=2)
+ return src_im
+
+class LabelDecode(object):
+ def __init__(self, **kwargs):
+ pass
+
+ def __call__(self, data):
+ label = json.loads(data['label'])
+
+ nBox = len(label)
+ seal_boxes = self.get_seal_boxes(label)
+
+ gt_label = []
+
+ for seal_box in seal_boxes:
+ seal_anno = {'seal_box': seal_box}
+ boxes, txts, txt_tags = [], [], []
+
+ for bno in range(0, nBox):
+ box = label[bno]['points']
+ txt = label[bno]['transcription']
+ try:
+ ints = self.get_intersection(box, seal_box)
+ except Exception as E:
+ print(E)
+ continue
+
+ if abs(Polygon(box).area - self.get_intersection(box, seal_box)) < 1e-3 and \
+ abs(Polygon(box).area - self.get_union(box, seal_box)) > 1e-3:
+
+ boxes.append(box)
+ txts.append(txt)
+ if txt in ['*', '###', '待识别']:
+ txt_tags.append(True)
+ else:
+ txt_tags.append(False)
+
+ seal_anno['polys'] = boxes
+ seal_anno['texts'] = txts
+ seal_anno['ignore_tags'] = txt_tags
+
+ gt_label.append(seal_anno)
+
+ return gt_label
+
+ def get_seal_boxes(self, label):
+
+ nBox = len(label)
+ seal_box = []
+ for bno in range(0, nBox):
+ box = label[bno]['points']
+ if len(box) == 4:
+ seal_box.append(box)
+
+ if len(seal_box) == 0:
+ return None
+
+ seal_box = self.valid_seal_box(seal_box)
+ return seal_box
+
+
+ def is_seal_box(self, box, boxes):
+ is_seal = True
+ for poly in boxes:
+ if list(box.shape()) != list(box.shape.shape()):
+ if abs(Polygon(box).area - self.get_intersection(box, poly)) < 1e-3:
+ return False
+ else:
+ if np.sum(np.array(box) - np.array(poly)) < 1e-3:
+ # continue when the box is same with poly
+ continue
+ if abs(Polygon(box).area - self.get_intersection(box, poly)) < 1e-3:
+ return False
+ return is_seal
+
+
+ def valid_seal_box(self, boxes):
+ if len(boxes) == 1:
+ return boxes
+
+ new_boxes = []
+ flag = True
+ for k in range(0, len(boxes)):
+ flag = True
+ tmp_box = boxes[k]
+ for i in range(0, len(boxes)):
+ if k == i: continue
+ if abs(Polygon(tmp_box).area - self.get_intersection(tmp_box, boxes[i])) < 1e-3:
+ flag = False
+ continue
+ if flag:
+ new_boxes.append(tmp_box)
+
+ return new_boxes
+
+
+ def get_union(self, pD, pG):
+ return Polygon(pD).union(Polygon(pG)).area
+
+ def get_intersection_over_union(self, pD, pG):
+ return get_intersection(pD, pG) / get_union(pD, pG)
+
+ def get_intersection(self, pD, pG):
+ return Polygon(pD).intersection(Polygon(pG)).area
+
+ def expand_points_num(self, boxes):
+ max_points_num = 0
+ for box in boxes:
+ if len(box) > max_points_num:
+ max_points_num = len(box)
+ ex_boxes = []
+ for box in boxes:
+ ex_box = box + [box[-1]] * (max_points_num - len(box))
+ ex_boxes.append(ex_box)
+ return ex_boxes
+
+
+def gen_extract_label(data_dir, label_file, seal_gt, seal_ppocr_gt):
+ label_decode_func = LabelDecode()
+ gts = open(label_file, "r").readlines()
+
+ seal_gt_list = []
+ seal_ppocr_list = []
+
+ for idx, line in enumerate(gts):
+ img_path, label = line.strip().split("\t")
+ data = {'label': label, 'img_path':img_path}
+ res = label_decode_func(data)
+ src_img = cv2.imread(os.path.join(data_dir, img_path))
+ if res is None:
+ print("ERROR! res is None!")
+ continue
+
+ anno = []
+ for i, gt in enumerate(res):
+ # print(i, box, type(box), )
+ anno.append({'polys': gt['seal_box'], 'cls':1})
+
+ seal_gt_list.append(f"{img_path}\t{json.dumps(anno)}\n")
+ seal_ppocr_list.append(f"{img_path}\t{json.dumps(res)}\n")
+
+ if not os.path.exists(os.path.dirname(seal_gt)):
+ os.makedirs(os.path.dirname(seal_gt))
+ if not os.path.exists(os.path.dirname(seal_ppocr_gt)):
+ os.makedirs(os.path.dirname(seal_ppocr_gt))
+
+ with open(seal_gt, "w") as f:
+ f.writelines(seal_gt_list)
+ f.close()
+
+ with open(seal_ppocr_gt, 'w') as f:
+ f.writelines(seal_ppocr_list)
+ f.close()
+
+def vis_seal_ppocr(data_dir, label_file, save_dir):
+
+ datas = open(label_file, 'r').readlines()
+ for idx, line in enumerate(datas):
+ img_path, label = line.strip().split('\t')
+ img_path = os.path.join(data_dir, img_path)
+
+ label = json.loads(label)
+ src_im = cv2.imread(img_path)
+ if src_im is None:
+ continue
+
+ for anno in label:
+ seal_box = anno['seal_box']
+ txt_boxes = anno['polys']
+
+ # vis seal box
+ src_im = draw_text_det_res([seal_box], src_im, color=(255, 255, 0))
+ src_im = draw_text_det_res(txt_boxes, src_im, color=(255, 0, 0))
+
+ save_path = os.path.join(save_dir, os.path.basename(img_path))
+ if not os.path.exists(save_dir):
+ os.makedirs(save_dir)
+ # print(src_im.shape)
+ cv2.imwrite(save_path, src_im)
+
+
+def draw_html(img_dir, save_name):
+ import glob
+
+ images_dir = glob.glob(img_dir + "/*")
+ print(len(images_dir))
+
+ html_path = save_name
+ with open(html_path, 'w') as html:
+ html.write('\n\n')
+ html.write('\n')
+ html.write(" ")
+
+ html.write("\n")
+ html.write(f' \n GT')
+
+ for i, filename in enumerate(sorted(images_dir)):
+ if filename.endswith("txt"): continue
+ print(filename)
+
+ base = "{}".format(filename)
+ if True:
+ html.write(" \n")
+ html.write(f' {filename}\n GT')
+ html.write(' GT 310\n ' % (base))
+ html.write(" \n")
+
+ html.write('\n')
+ html.write('
\n')
+ html.write('\n\n')
+ print("ok")
+
+
+def crop_seal_from_img(label_file, data_dir, save_dir, save_gt_path):
+
+ if not os.path.exists(save_dir):
+ os.makedirs(save_dir)
+
+ datas = open(label_file, 'r').readlines()
+ all_gts = []
+ count = 0
+ for idx, line in enumerate(datas):
+ img_path, label = line.strip().split('\t')
+ img_path = os.path.join(data_dir, img_path)
+
+ label = json.loads(label)
+ src_im = cv2.imread(img_path)
+ if src_im is None:
+ continue
+
+ for c, anno in enumerate(label):
+ seal_poly = anno['seal_box']
+ txt_boxes = anno['polys']
+ txts = anno['texts']
+ ignore_tags = anno['ignore_tags']
+
+ box = poly2box(seal_poly)
+ img_crop = src_im[box[0][1]:box[2][1], box[0][0]:box[2][0], :]
+
+ save_path = os.path.join(save_dir, f"{idx}_{c}.jpg")
+ cv2.imwrite(save_path, np.array(img_crop))
+
+ img_gt = []
+ for i in range(len(txts)):
+ txt_boxes_crop = np.array(txt_boxes[i])
+ txt_boxes_crop[:, 1] -= box[0, 1]
+ txt_boxes_crop[:, 0] -= box[0, 0]
+ img_gt.append({'transcription': txts[i], "points": txt_boxes_crop.tolist(), "ignore_tag": ignore_tags[i]})
+
+ if len(img_gt) >= 1:
+ count += 1
+ save_gt = f"{os.path.basename(save_path)}\t{json.dumps(img_gt)}\n"
+
+ all_gts.append(save_gt)
+
+ print(f"The num of all image: {len(all_gts)}, and the number of useful image: {count}")
+ if not os.path.exists(os.path.dirname(save_gt_path)):
+ os.makedirs(os.path.dirname(save_gt_path))
+
+ with open(save_gt_path, "w") as f:
+ f.writelines(all_gts)
+ f.close()
+ print("Done")
+
+
+
+if __name__ == "__main__":
+
+ # 数据处理
+ gen_extract_label("./seal_labeled_datas", "./seal_labeled_datas/Label.txt", "./seal_ppocr_gt/seal_det_img.txt", "./seal_ppocr_gt/seal_ppocr_img.txt")
+ vis_seal_ppocr("./seal_labeled_datas", "./seal_ppocr_gt/seal_ppocr_img.txt", "./seal_ppocr_gt/seal_ppocr_vis/")
+ draw_html("./seal_ppocr_gt/seal_ppocr_vis/", "./vis_seal_ppocr.html")
+ seal_ppocr_img_label = "./seal_ppocr_gt/seal_ppocr_img.txt"
+ crop_seal_from_img(seal_ppocr_img_label, "./seal_labeled_datas/", "./seal_img_crop", "./seal_img_crop/label.txt")
+
+```
+
+处理完成后,生成的文件如下:
+```
+├── seal_img_crop/
+│ ├── 0_0.jpg
+│ ├── ...
+│ └── label.txt
+├── seal_ppocr_gt/
+│ ├── seal_det_img.txt
+│ ├── seal_ppocr_img.txt
+│ └── seal_ppocr_vis/
+│ ├── test1.png
+│ ├── ...
+└── vis_seal_ppocr.html
+
+```
+其中`seal_img_crop/label.txt`文件为印章识别标签文件,其内容格式为:
+```
+0_0.jpg [{"transcription": "\u7535\u5b50\u56de\u5355", "points": [[29, 73], [96, 73], [96, 90], [29, 90]], "ignore_tag": false}, {"transcription": "\u4e91\u5357\u7701\u519c\u6751\u4fe1\u7528\u793e", "points": [[9, 58], [26, 63], [30, 49], [38, 35], [47, 29], [64, 26], [81, 32], [90, 45], [94, 63], [118, 57], [110, 35], [95, 17], [67, 0], [38, 7], [21, 23], [10, 43]], "ignore_tag": false}, {"transcription": "\u4e13\u7528\u7ae0", "points": [[29, 87], [95, 87], [95, 106], [29, 106]], "ignore_tag": false}]
+```
+可以直接用于PaddleOCR的PGNet算法的训练。
+
+`seal_ppocr_gt/seal_det_img.txt`为印章检测标签文件,其内容格式为:
+```
+img/test1.png [{"polys": [[408, 232], [537, 232], [537, 352], [408, 352]], "cls": 1}]
+```
+为了使用PaddleDetection工具完成印章检测模型的训练,需要将`seal_det_img.txt`转换为COCO或者VOC的数据标注格式。
+
+可以直接使用下述代码将印章检测标注转换成VOC格式。
+
+
+```
+import numpy as np
+import json
+import cv2
+import os
+from shapely.geometry import Polygon
+
+seal_train_gt = "./seal_ppocr_gt/seal_det_img.txt"
+# 注:仅用于示例,实际使用中需要分别转换训练集和测试集的标签
+seal_valid_gt = "./seal_ppocr_gt/seal_det_img.txt"
+
+def gen_main_train_txt(mode='train'):
+ if mode == "train":
+ file_path = seal_train_gt
+ if mode in ['valid', 'test']:
+ file_path = seal_valid_gt
+
+ save_path = f"./seal_VOC/ImageSets/Main/{mode}.txt"
+ save_train_path = f"./seal_VOC/{mode}.txt"
+ if not os.path.exists(os.path.dirname(save_path)):
+ os.makedirs(os.path.dirname(save_path))
+
+ datas = open(file_path, 'r').readlines()
+ img_names = []
+ train_names = []
+ for line in datas:
+ img_name = line.strip().split('\t')[0]
+ img_name = os.path.basename(img_name)
+ (i_name, extension) = os.path.splitext(img_name)
+ t_name = 'JPEGImages/'+str(img_name)+' '+'Annotations/'+str(i_name)+'.xml\n'
+ train_names.append(t_name)
+ img_names.append(i_name + "\n")
+
+ with open(save_train_path, "w") as f:
+ f.writelines(train_names)
+ f.close()
+
+ with open(save_path, "w") as f:
+ f.writelines(img_names)
+ f.close()
+
+ print(f"{mode} save done")
+
+
+def gen_xml_label(mode='train'):
+ if mode == "train":
+ file_path = seal_train_gt
+ if mode in ['valid', 'test']:
+ file_path = seal_valid_gt
+
+ datas = open(file_path, 'r').readlines()
+ img_names = []
+ train_names = []
+ anno_path = "./seal_VOC/Annotations"
+ img_path = "./seal_VOC/JPEGImages"
+
+ if not os.path.exists(anno_path):
+ os.makedirs(anno_path)
+ if not os.path.exists(img_path):
+ os.makedirs(img_path)
+
+ for idx, line in enumerate(datas):
+ img_name, label = line.strip().split('\t')
+ img = cv2.imread(os.path.join("./seal_labeled_datas", img_name))
+ cv2.imwrite(os.path.join(img_path, os.path.basename(img_name)), img)
+ height, width, c = img.shape
+ img_name = os.path.basename(img_name)
+ (i_name, extension) = os.path.splitext(img_name)
+ label = json.loads(label)
+
+ xml_file = open(("./seal_VOC/Annotations" + '/' + i_name + '.xml'), 'w')
+ xml_file.write('\n')
+ xml_file.write(' seal_VOC \n')
+ xml_file.write(' ' + str(img_name) + ' \n')
+ xml_file.write(' ' + 'Annotations/' + str(img_name) + ' \n')
+ xml_file.write(' \n')
+ xml_file.write(' ' + str(width) + ' \n')
+ xml_file.write(' ' + str(height) + ' \n')
+ xml_file.write(' 3 \n')
+ xml_file.write(' \n')
+ xml_file.write(' 0 \n')
+
+ for anno in label:
+ poly = anno['polys']
+ if anno['cls'] == 1:
+ gt_cls = 'redseal'
+ xmin = np.min(np.array(poly)[:, 0])
+ ymin = np.min(np.array(poly)[:, 1])
+ xmax = np.max(np.array(poly)[:, 0])
+ ymax = np.max(np.array(poly)[:, 1])
+ xmin,ymin,xmax,ymax= int(xmin),int(ymin),int(xmax),int(ymax)
+ xml_file.write(' \n')
+ xml_file.write(' '+str(gt_cls)+' \n')
+ xml_file.write(' Unspecified \n')
+ xml_file.write(' 0 \n')
+ xml_file.write(' 0 \n')
+ xml_file.write(' \n')
+ xml_file.write(' '+str(xmin)+' \n')
+ xml_file.write(' '+str(ymin)+' \n')
+ xml_file.write(' '+str(xmax)+' \n')
+ xml_file.write(' '+str(ymax)+' \n')
+ xml_file.write(' \n')
+ xml_file.write(' \n')
+ xml_file.write(' ')
+ xml_file.close()
+ print(f'{mode} xml save done!')
+
+
+gen_main_train_txt()
+gen_main_train_txt('valid')
+gen_xml_label('train')
+gen_xml_label('valid')
+
+```
+
+数据处理完成后,转换为VOC格式的印章检测数据存储在~/data/seal_VOC目录下,目录组织结构为:
+
+```
+├── Annotations/
+├── ImageSets/
+│ └── Main/
+│ ├── train.txt
+│ └── valid.txt
+├── JPEGImages/
+├── train.txt
+└── valid.txt
+└── label_list.txt
+```
+
+Annotations下为数据的标签,JPEGImages目录下为图像文件,label_list.txt为标注检测框类别标签文件。
+
+在接下来一节中,将介绍如何使用PaddleDetection工具库完成印章检测模型的训练。
+
+# 4. 印章检测实践
+
+在实际应用中,印章多是出现在合同,发票,公告等场景中,印章文字识别的任务需要排除图像中背景文字的影响,因此需要先检测出图像中的印章区域。
+
+
+借助PaddleDetection目标检测库可以很容易的实现印章检测任务,使用PaddleDetection训练印章检测任务流程如下:
+
+- 选择算法
+- 修改数据集配置路径
+- 启动训练
+
+
+**算法选择**
+
+PaddleDetection中有许多检测算法可以选择,考虑到每条数据中印章区域较为清晰,且考虑到性能需求。在本项目中,我们采用mobilenetv3为backbone的ppyolo算法完成印章检测任务,对应的配置文件是:configs/ppyolo/ppyolo_mbv3_large.yml
+
+
+
+**修改配置文件**
+
+配置文件中的默认数据路径是COCO,
+需要修改为印章检测的数据路径,主要修改如下:
+在配置文件'configs/ppyolo/ppyolo_mbv3_large.yml'末尾增加如下内容:
+```
+metric: VOC
+map_type: 11point
+num_classes: 2
+
+TrainDataset:
+ !VOCDataSet
+ dataset_dir: dataset/seal_VOC
+ anno_path: train.txt
+ label_list: label_list.txt
+ data_fields: ['image', 'gt_bbox', 'gt_class', 'difficult']
+
+EvalDataset:
+ !VOCDataSet
+ dataset_dir: dataset/seal_VOC
+ anno_path: test.txt
+ label_list: label_list.txt
+ data_fields: ['image', 'gt_bbox', 'gt_class', 'difficult']
+
+TestDataset:
+ !ImageFolder
+ anno_path: dataset/seal_VOC/label_list.txt
+```
+
+配置文件中设置的数据路径在PaddleDetection/dataset目录下,我们可以将处理后的印章检测训练数据移动到PaddleDetection/dataset目录下或者创建一个软连接。
+
+```
+!ln -s seal_VOC ./PaddleDetection/dataset/
+```
+
+另外图象中印章数量比较少,可以调整NMS后处理的检测框数量,即keep_top_k,nms_top_k 从100,1000,调整为10,100。在配置文件'configs/ppyolo/ppyolo_mbv3_large.yml'末尾增加如下内容完成后处理参数的调整
+```
+BBoxPostProcess:
+ decode:
+ name: YOLOBox
+ conf_thresh: 0.005
+ downsample_ratio: 32
+ clip_bbox: true
+ scale_x_y: 1.05
+ nms:
+ name: MultiClassNMS
+ keep_top_k: 10 # 修改前100
+ nms_threshold: 0.45
+ nms_top_k: 100 # 修改前1000
+ score_threshold: 0.005
+```
+
+
+修改完成后,需要在PaddleDetection中增加印章数据的处理代码,即在PaddleDetection/ppdet/data/source/目录下创建seal.py文件,文件中填充如下代码:
+```
+import os
+import numpy as np
+from ppdet.core.workspace import register, serializable
+from .dataset import DetDataset
+import cv2
+import json
+
+from ppdet.utils.logger import setup_logger
+logger = setup_logger(__name__)
+
+
+@register
+@serializable
+class SealDataSet(DetDataset):
+ """
+ Load dataset with COCO format.
+
+ Args:
+ dataset_dir (str): root directory for dataset.
+ image_dir (str): directory for images.
+ anno_path (str): coco annotation file path.
+ data_fields (list): key name of data dictionary, at least have 'image'.
+ sample_num (int): number of samples to load, -1 means all.
+ load_crowd (bool): whether to load crowded ground-truth.
+ False as default
+ allow_empty (bool): whether to load empty entry. False as default
+ empty_ratio (float): the ratio of empty record number to total
+ record's, if empty_ratio is out of [0. ,1.), do not sample the
+ records and use all the empty entries. 1. as default
+ """
+
+ def __init__(self,
+ dataset_dir=None,
+ image_dir=None,
+ anno_path=None,
+ data_fields=['image'],
+ sample_num=-1,
+ load_crowd=False,
+ allow_empty=False,
+ empty_ratio=1.):
+ super(SealDataSet, self).__init__(dataset_dir, image_dir, anno_path,
+ data_fields, sample_num)
+ self.load_image_only = False
+ self.load_semantic = False
+ self.load_crowd = load_crowd
+ self.allow_empty = allow_empty
+ self.empty_ratio = empty_ratio
+
+ def _sample_empty(self, records, num):
+ # if empty_ratio is out of [0. ,1.), do not sample the records
+ if self.empty_ratio < 0. or self.empty_ratio >= 1.:
+ return records
+ import random
+ sample_num = min(
+ int(num * self.empty_ratio / (1 - self.empty_ratio)), len(records))
+ records = random.sample(records, sample_num)
+ return records
+
+ def parse_dataset(self):
+ anno_path = os.path.join(self.dataset_dir, self.anno_path)
+ image_dir = os.path.join(self.dataset_dir, self.image_dir)
+
+ records = []
+ empty_records = []
+ ct = 0
+
+ assert anno_path.endswith('.txt'), \
+ 'invalid seal_gt file: ' + anno_path
+
+ all_datas = open(anno_path, 'r').readlines()
+
+ for idx, line in enumerate(all_datas):
+ im_path, label = line.strip().split('\t')
+ img_path = os.path.join(image_dir, im_path)
+ label = json.loads(label)
+ im_h, im_w, im_c = cv2.imread(img_path).shape
+
+ coco_rec = {
+ 'im_file': img_path,
+ 'im_id': np.array([idx]),
+ 'h': im_h,
+ 'w': im_w,
+ } if 'image' in self.data_fields else {}
+
+ if not self.load_image_only:
+ bboxes = []
+ for anno in label:
+ poly = anno['polys']
+ # poly to box
+ x1 = np.min(np.array(poly)[:, 0])
+ y1 = np.min(np.array(poly)[:, 1])
+ x2 = np.max(np.array(poly)[:, 0])
+ y2 = np.max(np.array(poly)[:, 1])
+ eps = 1e-5
+ if x2 - x1 > eps and y2 - y1 > eps:
+ clean_box = [
+ round(float(x), 3) for x in [x1, y1, x2, y2]
+ ]
+ anno = {'clean_box': clean_box, 'gt_cls':int(anno['cls'])}
+ bboxes.append(anno)
+ else:
+ logger.info("invalid box")
+
+ num_bbox = len(bboxes)
+ if num_bbox <= 0:
+ continue
+
+ gt_bbox = np.zeros((num_bbox, 4), dtype=np.float32)
+ gt_class = np.zeros((num_bbox, 1), dtype=np.int32)
+ is_crowd = np.zeros((num_bbox, 1), dtype=np.int32)
+ # gt_poly = [None] * num_bbox
+
+ for i, box in enumerate(bboxes):
+ gt_class[i][0] = box['gt_cls']
+ gt_bbox[i, :] = box['clean_box']
+ is_crowd[i][0] = 0
+
+ gt_rec = {
+ 'is_crowd': is_crowd,
+ 'gt_class': gt_class,
+ 'gt_bbox': gt_bbox,
+ # 'gt_poly': gt_poly,
+ }
+
+ for k, v in gt_rec.items():
+ if k in self.data_fields:
+ coco_rec[k] = v
+
+ records.append(coco_rec)
+ ct += 1
+ if self.sample_num > 0 and ct >= self.sample_num:
+ break
+ self.roidbs = records
+```
+
+**启动训练**
+
+启动单卡训练的命令为:
+```
+!python3 tools/train.py -c configs/ppyolo/ppyolo_mbv3_large.yml --eval
+
+# 分布式训练命令为:
+!python3 -m paddle.distributed.launch --gpus 0,1,2,3,4,5,6,7 tools/train.py -c configs/ppyolo/ppyolo_mbv3_large.yml --eval
+```
+
+训练完成后,日志中会打印模型的精度:
+
+```
+[07/05 11:42:09] ppdet.engine INFO: Eval iter: 0
+[07/05 11:42:14] ppdet.metrics.metrics INFO: Accumulating evaluatation results...
+[07/05 11:42:14] ppdet.metrics.metrics INFO: mAP(0.50, 11point) = 99.31%
+[07/05 11:42:14] ppdet.engine INFO: Total sample number: 112, averge FPS: 26.45840794253432
+[07/05 11:42:14] ppdet.engine INFO: Best test bbox ap is 0.996.
+```
+
+
+我们可以使用训练好的模型观察预测结果:
+```
+!python3 tools/infer.py -c configs/ppyolo/ppyolo_mbv3_large.yml -o weights=./output/ppyolo_mbv3_large/model_final.pdparams --img_dir=./test.jpg
+```
+预测结果如下:
+
+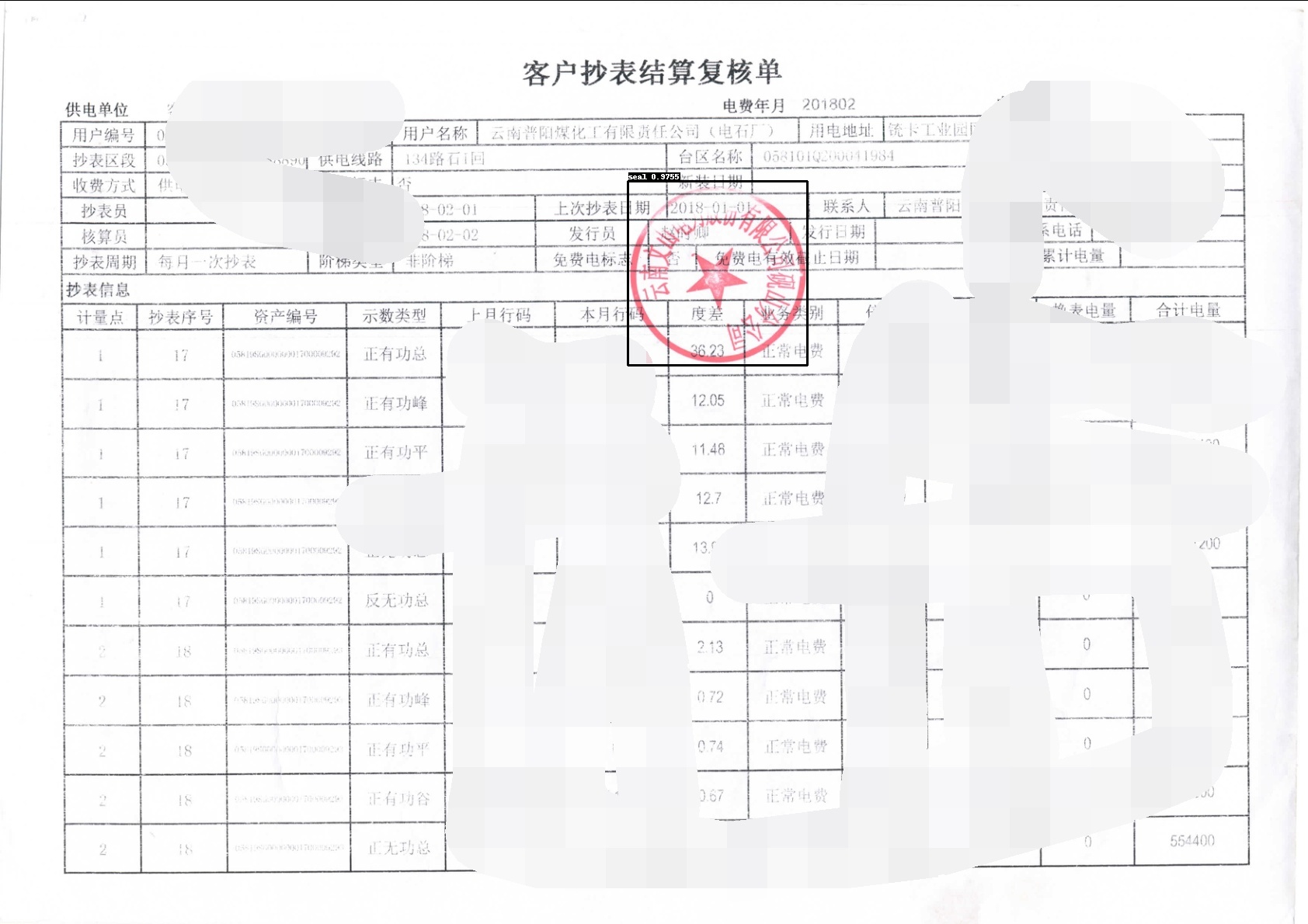
+
+# 5. 印章文字识别实践
+
+在使用ppyolo检测到印章区域后,接下来借助PaddleOCR里的文字识别能力,完成印章中文字的识别。
+
+PaddleOCR中的OCR算法包含文字检测算法,文字识别算法以及OCR端对端算法。
+
+文字检测算法负责检测到图像中的文字,再由文字识别模型识别出检测到的文字,进而实现OCR的任务。文字检测+文字识别串联完成OCR任务的架构称为两阶段的OCR算法。相对应的端对端的OCR方法可以用一个算法同时完成文字检测和识别的任务。
+
+
+| 文字检测 | 文字识别 | 端对端算法 |
+| -------- | -------- | -------- |
+| DB\DB++\EAST\SAST\PSENet | SVTR\CRNN\NRTN\Abinet\SAR\... | PGNet |
+
+
+本节中将分别介绍端对端的文字检测识别算法以及两阶段的文字检测识别算法在印章检测识别任务上的实践。
+
+
+## 5.1 端对端印章文字识别实践
+
+本节介绍使用PaddleOCR里的PGNet算法完成印章文字识别。
+
+PGNet属于端对端的文字检测识别算法,在PaddleOCR中的配置文件为:
+[PaddleOCR/configs/e2e/e2e_r50_vd_pg.yml](https://github.com/PaddlePaddle/PaddleOCR/blob/release/2.6/configs/e2e/e2e_r50_vd_pg.yml)
+
+使用PGNet完成文字检测识别任务的步骤为:
+- 修改配置文件
+- 启动训练
+
+PGNet默认配置文件的数据路径为totaltext数据集路径,本次训练中,需要修改为上一节数据处理后得到的标签文件和数据目录:
+
+训练数据配置修改后如下:
+```
+Train:
+ dataset:
+ name: PGDataSet
+ data_dir: ./train_data/seal_ppocr
+ label_file_list: [./train_data/seal_ppocr/seal_ppocr_img.txt]
+ ratio_list: [1.0]
+```
+测试数据集配置修改后如下:
+```
+Eval:
+ dataset:
+ name: PGDataSet
+ data_dir: ./train_data/seal_ppocr_test
+ label_file_list: [./train_data/seal_ppocr_test/seal_ppocr_img.txt]
+```
+
+启动训练的命令为:
+```
+!python3 tools/train.py -c configs/e2e/e2e_r50_vd_pg.yml
+```
+模型训练完成后,可以得到最终的精度为47.4%。数据量较少,以及数据质量较差会影响模型的训练精度,如果有更多的数据参与训练,精度将进一步提升。
+
+如需获取已训练模型,请扫文末的二维码填写问卷,加入PaddleOCR官方交流群获取全部OCR垂类模型下载链接、《动手学OCR》电子书等全套OCR学习资料🎁
+
+## 5.2 两阶段印章文字识别实践
+
+上一节介绍了使用PGNet实现印章识别任务的训练流程。本小节将介绍使用PaddleOCR里的文字检测和文字识别算法分别完成印章文字的检测和识别。
+
+### 5.2.1 印章文字检测
+
+PaddleOCR中包含丰富的文字检测算法,包含DB,DB++,EAST,SAST,PSENet等等。其中DB,DB++,PSENet均支持弯曲文字检测,本项目中,使用DB++作为印章弯曲文字检测算法。
+
+PaddleOCR中发布的db++文字检测算法模型是英文文本检测模型,因此需要重新训练模型。
+
+
+修改[DB++配置文件](DB++的默认配置文件位于[configs/det/det_r50_db++_icdar15.yml](https://github.com/PaddlePaddle/PaddleOCR/blob/release/2.6/configs/det/det_r50_db%2B%2B_icdar15.yml)
+中的数据路径:
+
+
+```
+Train:
+ dataset:
+ name: SimpleDataSet
+ data_dir: ./train_data/seal_ppocr
+ label_file_list: [./train_data/seal_ppocr/seal_ppocr_img.txt]
+ ratio_list: [1.0]
+```
+测试数据集配置修改后如下:
+```
+Eval:
+ dataset:
+ name: SimpleDataSet
+ data_dir: ./train_data/seal_ppocr_test
+ label_file_list: [./train_data/seal_ppocr_test/seal_ppocr_img.txt]
+```
+
+
+启动训练:
+```
+!python3 tools/train.py -c configs/det/det_r50_db++_icdar15.yml -o Global.epoch_num=100
+```
+
+考虑到数据较少,通过Global.epoch_num设置仅训练100个epoch。
+模型训练完成后,在测试集上预测的可视化效果如下:
+
+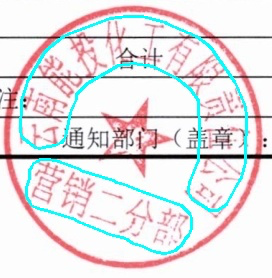
+
+
+如需获取已训练模型,请扫文末的二维码填写问卷,加入PaddleOCR官方交流群获取全部OCR垂类模型下载链接、《动手学OCR》电子书等全套OCR学习资料🎁
+
+
+### 5.2.2 印章文字识别
+
+上一节中完成了印章文字的检测模型训练,本节介绍印章文字识别模型的训练。识别模型采用SVTR算法,SVTR算法是IJCAI收录的文字识别算法,SVTR模型具备超轻量高精度的特点。
+
+在启动训练之前,需要准备印章文字识别需要的数据集,需要使用如下代码,将印章中的文字区域剪切出来构建训练集。
+
+```
+import cv2
+import numpy as np
+
+def get_rotate_crop_image(img, points):
+ '''
+ img_height, img_width = img.shape[0:2]
+ left = int(np.min(points[:, 0]))
+ right = int(np.max(points[:, 0]))
+ top = int(np.min(points[:, 1]))
+ bottom = int(np.max(points[:, 1]))
+ img_crop = img[top:bottom, left:right, :].copy()
+ points[:, 0] = points[:, 0] - left
+ points[:, 1] = points[:, 1] - top
+ '''
+ assert len(points) == 4, "shape of points must be 4*2"
+ img_crop_width = int(
+ max(
+ np.linalg.norm(points[0] - points[1]),
+ np.linalg.norm(points[2] - points[3])))
+ img_crop_height = int(
+ max(
+ np.linalg.norm(points[0] - points[3]),
+ np.linalg.norm(points[1] - points[2])))
+ pts_std = np.float32([[0, 0], [img_crop_width, 0],
+ [img_crop_width, img_crop_height],
+ [0, img_crop_height]])
+ M = cv2.getPerspectiveTransform(points, pts_std)
+ dst_img = cv2.warpPerspective(
+ img,
+ M, (img_crop_width, img_crop_height),
+ borderMode=cv2.BORDER_REPLICATE,
+ flags=cv2.INTER_CUBIC)
+ dst_img_height, dst_img_width = dst_img.shape[0:2]
+ if dst_img_height * 1.0 / dst_img_width >= 1.5:
+ dst_img = np.rot90(dst_img)
+ return dst_img
+
+
+def run(data_dir, label_file, save_dir):
+ datas = open(label_file, 'r').readlines()
+ for idx, line in enumerate(datas):
+ img_path, label = line.strip().split('\t')
+ img_path = os.path.join(data_dir, img_path)
+
+ label = json.loads(label)
+ src_im = cv2.imread(img_path)
+ if src_im is None:
+ continue
+
+ for anno in label:
+ seal_box = anno['seal_box']
+ txt_boxes = anno['polys']
+ crop_im = get_rotate_crop_image(src_im, text_boxes)
+
+ save_path = os.path.join(save_dir, f'{idx}.png')
+ if not os.path.exists(save_dir):
+ os.makedirs(save_dir)
+ # print(src_im.shape)
+ cv2.imwrite(save_path, crop_im)
+
+```
+
+
+数据处理完成后,即可配置训练的配置文件。SVTR配置文件选择[configs/rec/PP-OCRv3/ch_PP-OCRv3_rec.yml](https://github.com/PaddlePaddle/PaddleOCR/blob/release/2.6/configs/rec/PP-OCRv3/ch_PP-OCRv3_rec.yml)
+修改SVTR配置文件中的训练数据部分如下:
+
+```
+Train:
+ dataset:
+ name: SimpleDataSet
+ data_dir: ./train_data/seal_ppocr_crop/
+ label_file_list:
+ - ./train_data/seal_ppocr_crop/train_list.txt
+```
+
+修改预测部分配置文件:
+```
+Train:
+ dataset:
+ name: SimpleDataSet
+ data_dir: ./train_data/seal_ppocr_crop/
+ label_file_list:
+ - ./train_data/seal_ppocr_crop_test/train_list.txt
+```
+
+启动训练:
+
+```
+!python3 tools/train.py -c configs/rec/PP-OCRv3/ch_PP-OCRv3_rec.yml
+
+```
+
+训练完成后可以发现测试集指标达到了61%。
+由于数据较少,训练时会发现在训练集上的acc指标远大于测试集上的acc指标,即出现过拟合现象。通过补充数据和一些数据增强可以缓解这个问题。
+
+
+
+如需获取已训练模型,请扫下图二维码填写问卷,加入PaddleOCR官方交流群获取全部OCR垂类模型下载链接、《动手学OCR》电子书等全套OCR学习资料🎁
+
+
+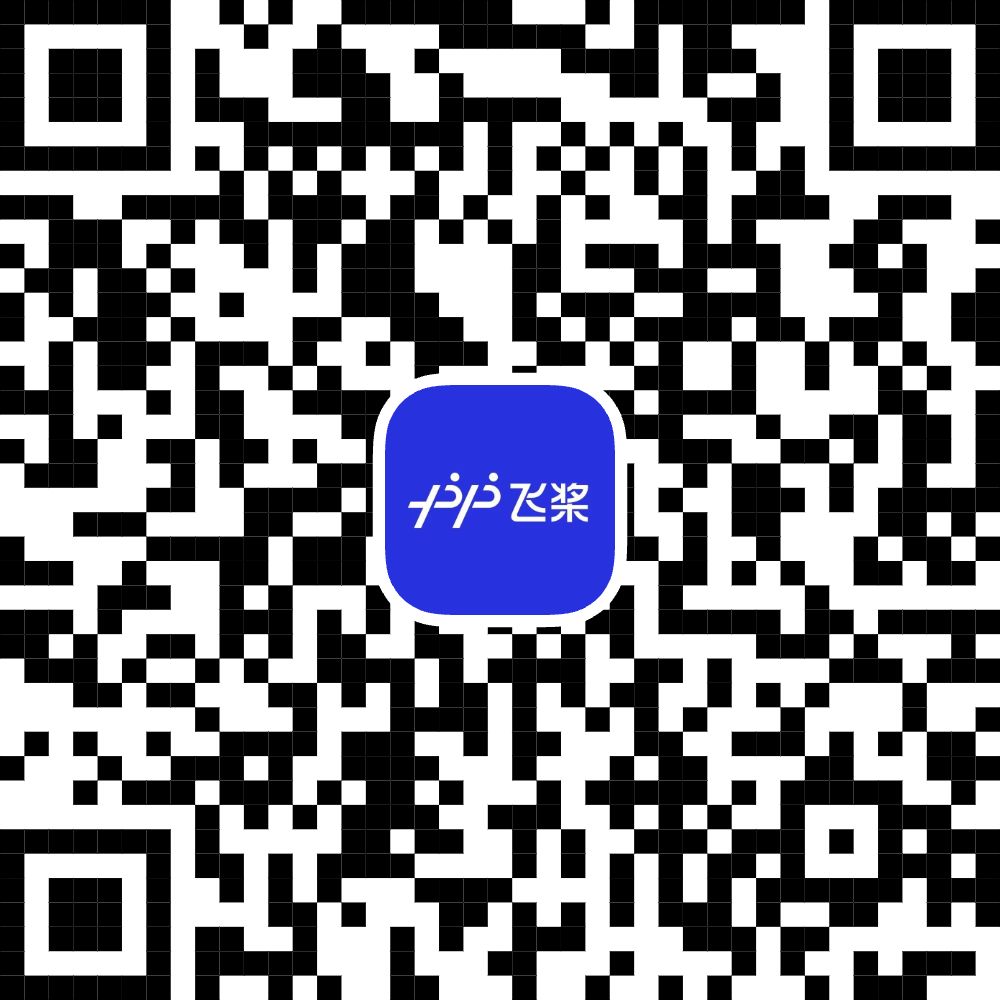
diff --git "a/applications/\345\277\253\351\200\237\346\236\204\345\273\272\345\215\241\350\257\201\347\261\273OCR.md" "b/applications/\345\277\253\351\200\237\346\236\204\345\273\272\345\215\241\350\257\201\347\261\273OCR.md"
new file mode 100644
index 0000000000000000000000000000000000000000..ab9ddf1bbd20e538f0b1e3d43e9a5af5383487b4
--- /dev/null
+++ "b/applications/\345\277\253\351\200\237\346\236\204\345\273\272\345\215\241\350\257\201\347\261\273OCR.md"
@@ -0,0 +1,782 @@
+# 快速构建卡证类OCR
+
+
+- [快速构建卡证类OCR](#快速构建卡证类ocr)
+ - [1. 金融行业卡证识别应用](#1-金融行业卡证识别应用)
+ - [1.1 金融行业中的OCR相关技术](#11-金融行业中的ocr相关技术)
+ - [1.2 金融行业中的卡证识别场景介绍](#12-金融行业中的卡证识别场景介绍)
+ - [1.3 OCR落地挑战](#13-ocr落地挑战)
+ - [2. 卡证识别技术解析](#2-卡证识别技术解析)
+ - [2.1 卡证分类模型](#21-卡证分类模型)
+ - [2.2 卡证识别模型](#22-卡证识别模型)
+ - [3. OCR技术拆解](#3-ocr技术拆解)
+ - [3.1技术流程](#31技术流程)
+ - [3.2 OCR技术拆解---卡证分类](#32-ocr技术拆解---卡证分类)
+ - [卡证分类:数据、模型准备](#卡证分类数据模型准备)
+ - [卡证分类---修改配置文件](#卡证分类---修改配置文件)
+ - [卡证分类---训练](#卡证分类---训练)
+ - [3.2 OCR技术拆解---卡证识别](#32-ocr技术拆解---卡证识别)
+ - [身份证识别:检测+分类](#身份证识别检测分类)
+ - [数据标注](#数据标注)
+ - [4 . 项目实践](#4--项目实践)
+ - [4.1 环境准备](#41-环境准备)
+ - [4.2 配置文件修改](#42-配置文件修改)
+ - [4.3 代码修改](#43-代码修改)
+ - [4.3.1 数据读取](#431-数据读取)
+ - [4.3.2 head修改](#432--head修改)
+ - [4.3.3 修改loss](#433-修改loss)
+ - [4.3.4 后处理](#434-后处理)
+ - [4.4. 模型启动](#44-模型启动)
+ - [5 总结](#5-总结)
+ - [References](#references)
+
+## 1. 金融行业卡证识别应用
+
+### 1.1 金融行业中的OCR相关技术
+
+* 《“十四五”数字经济发展规划》指出,2020年我国数字经济核心产业增加值占GDP比重达7.8%,随着数字经济迈向全面扩展,到2025年该比例将提升至10%。
+
+* 在过去数年的跨越发展与积累沉淀中,数字金融、金融科技已在对金融业的重塑与再造中充分印证了其自身价值。
+
+* 以智能为目标,提升金融数字化水平,实现业务流程自动化,降低人力成本。
+
+
+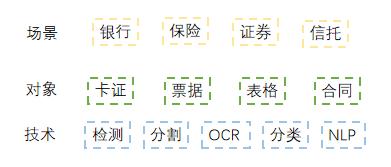
+
+
+
+### 1.2 金融行业中的卡证识别场景介绍
+
+应用场景:身份证、银行卡、营业执照、驾驶证等。
+
+应用难点:由于数据的采集来源多样,以及实际采集数据各种噪声:反光、褶皱、模糊、倾斜等各种问题干扰。
+
+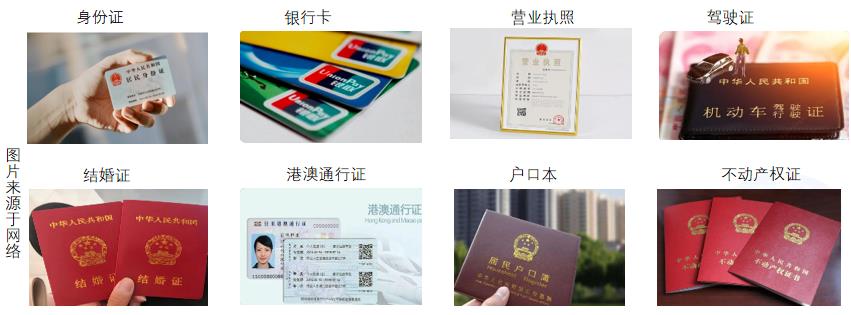
+
+
+
+### 1.3 OCR落地挑战
+
+
+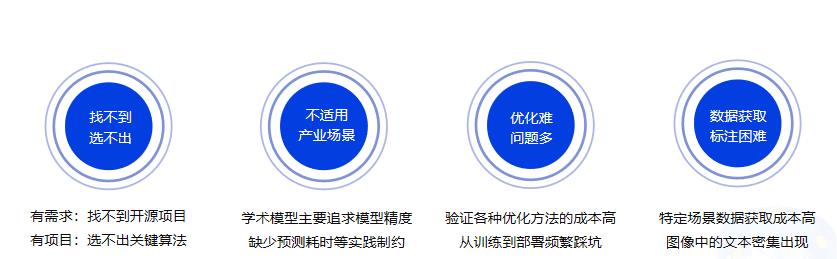
+
+
+
+
+
+## 2. 卡证识别技术解析
+
+
+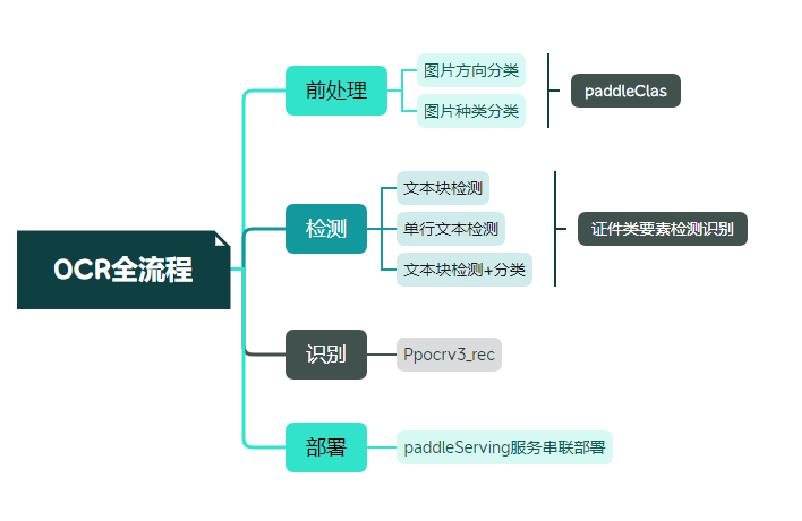
+
+
+### 2.1 卡证分类模型
+
+卡证分类:基于PPLCNet
+
+与其他轻量级模型相比在CPU环境下ImageNet数据集上的表现
+
+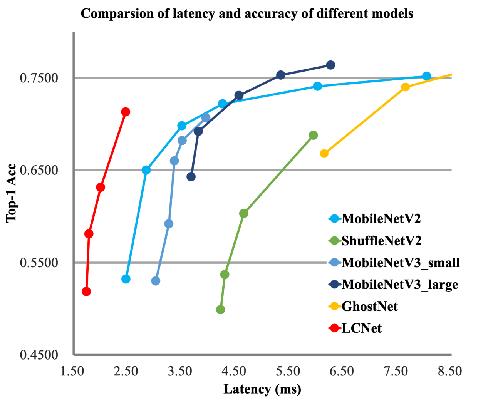
+
+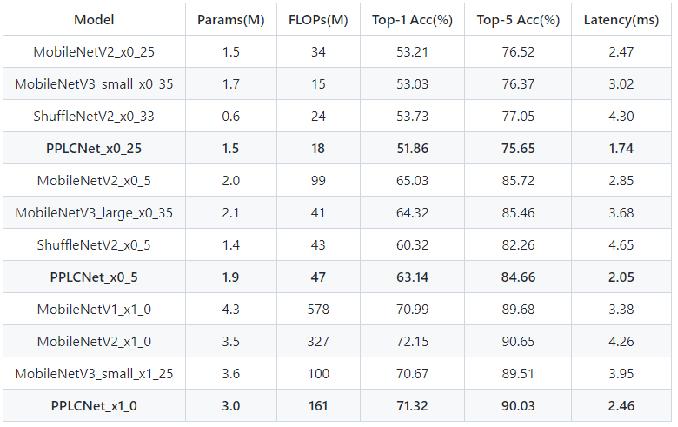
+
+
+
+* 模型来自模型库PaddleClas,它是一个图像识别和图像分类任务的工具集,助力使用者训练出更好的视觉模型和应用落地。
+
+
+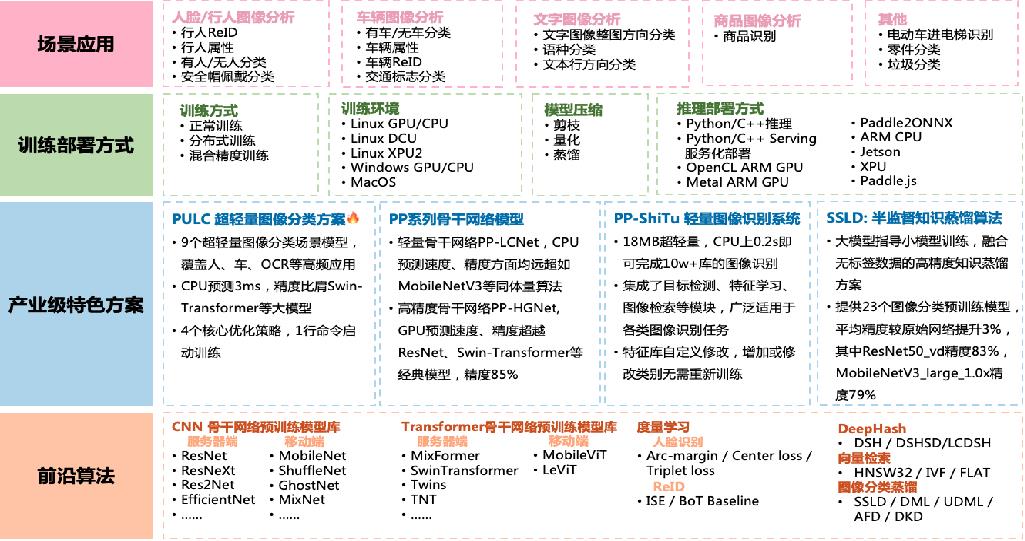
+
+
+
+### 2.2 卡证识别模型
+
+* 检测:DBNet 识别:SVRT
+
+
+
+
+* PPOCRv3在文本检测、识别进行了一系列改进优化,在保证精度的同时提升预测效率
+
+
+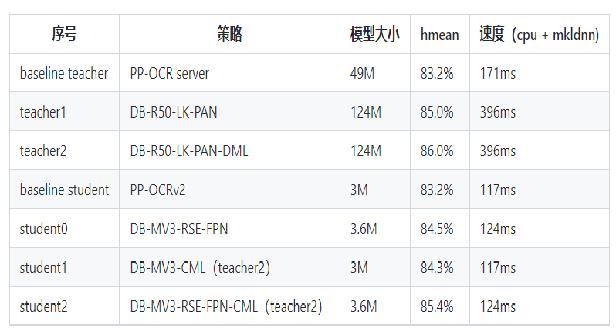
+
+
+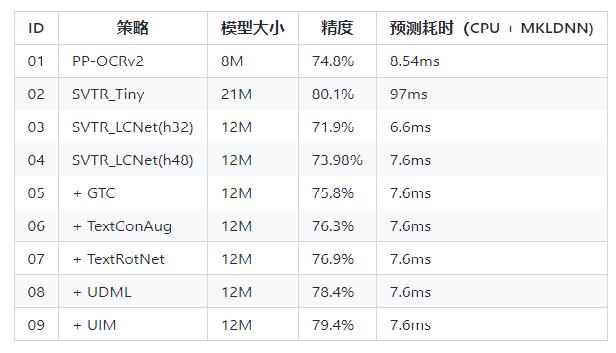
+
+
+## 3. OCR技术拆解
+
+### 3.1技术流程
+
+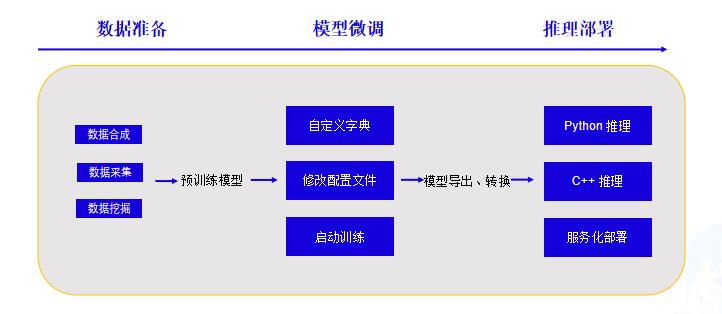
+
+
+### 3.2 OCR技术拆解---卡证分类
+
+#### 卡证分类:数据、模型准备
+
+
+A 使用爬虫获取无标注数据,将相同类别的放在同一文件夹下,文件名从0开始命名。具体格式如下图所示。
+
+ 注:卡证类数据,建议每个类别数据量在500张以上
+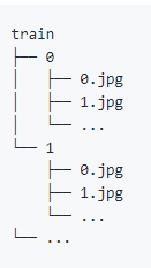
+
+
+B 一行命令生成标签文件
+
+```
+tree -r -i -f | grep -E "jpg|JPG|jpeg|JPEG|png|PNG|webp" | awk -F "/" '{print $0" "$2}' > train_list.txt
+```
+
+C [下载预训练模型 ](https://github.com/PaddlePaddle/PaddleClas/blob/release/2.4/docs/zh_CN/models/PP-LCNet.md)
+
+
+
+#### 卡证分类---修改配置文件
+
+
+配置文件主要修改三个部分:
+
+ 全局参数:预训练模型路径/训练轮次/图像尺寸
+
+ 模型结构:分类数
+
+ 数据处理:训练/评估数据路径
+
+
+ 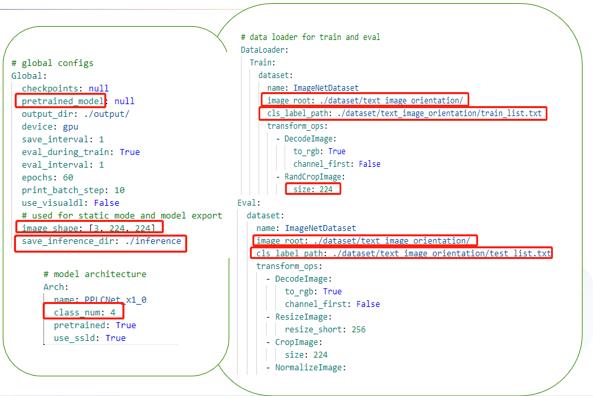
+
+#### 卡证分类---训练
+
+
+指定配置文件启动训练:
+
+```
+!python /home/aistudio/work/PaddleClas/tools/train.py -c /home/aistudio/work/PaddleClas/ppcls/configs/PULC/text_image_orientation/PPLCNet_x1_0.yaml
+```
+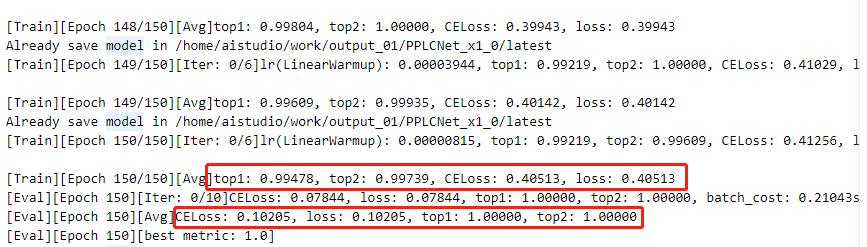
+
+ 注:日志中显示了训练结果和评估结果(训练时可以设置固定轮数评估一次)
+
+
+### 3.2 OCR技术拆解---卡证识别
+
+卡证识别(以身份证检测为例)
+存在的困难及问题:
+
+ * 在自然场景下,由于各种拍摄设备以及光线、角度不同等影响导致实际得到的证件影像千差万别。
+
+ * 如何快速提取需要的关键信息
+
+ * 多行的文本信息,检测结果如何正确拼接
+
+ 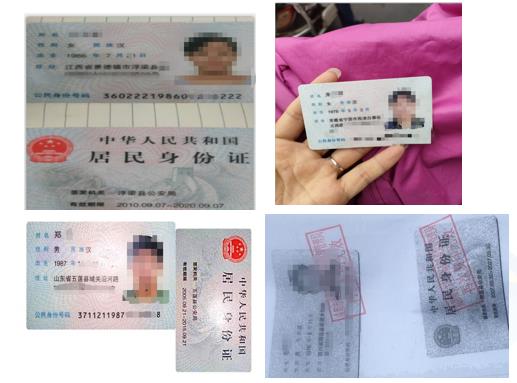
+
+
+
+* OCR技术拆解---OCR工具库
+
+ PaddleOCR是一个丰富、领先且实用的OCR工具库,助力开发者训练出更好的模型并应用落地
+
+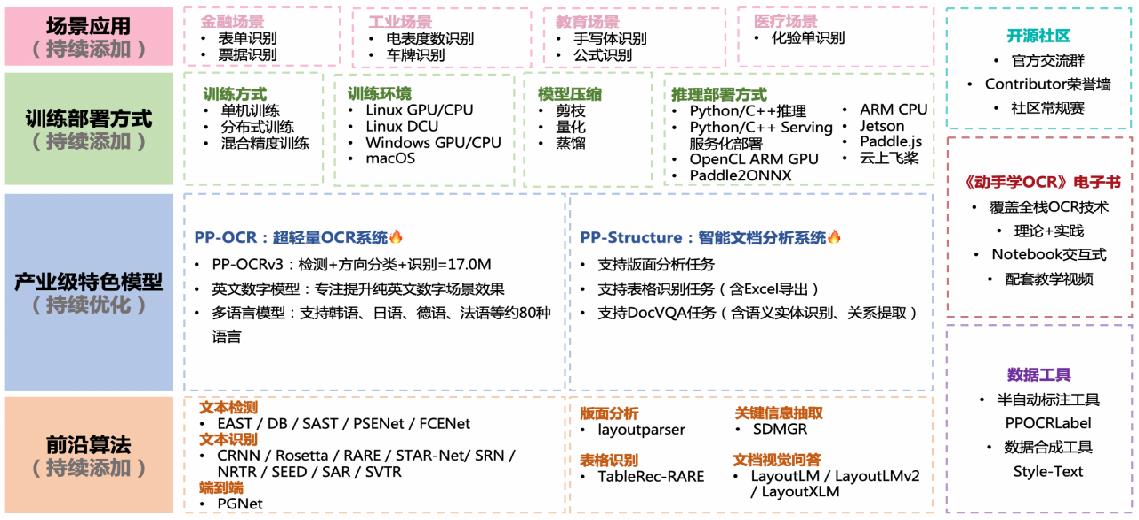
+
+
+身份证识别:用现有的方法识别
+
+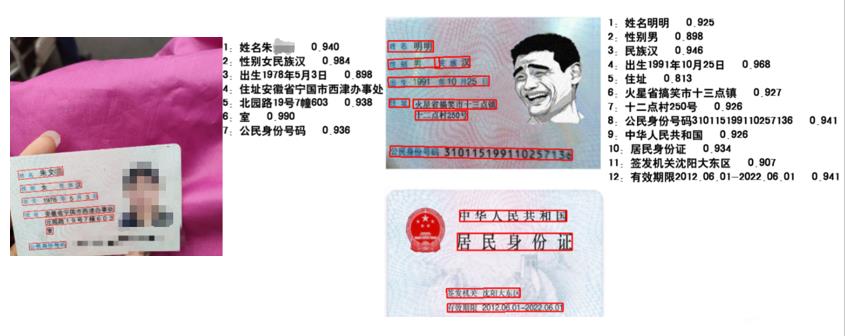
+
+
+
+
+#### 身份证识别:检测+分类
+
+> 方法:基于现有的dbnet检测模型,加入分类方法。检测同时进行分类,从一定程度上优化识别流程
+
+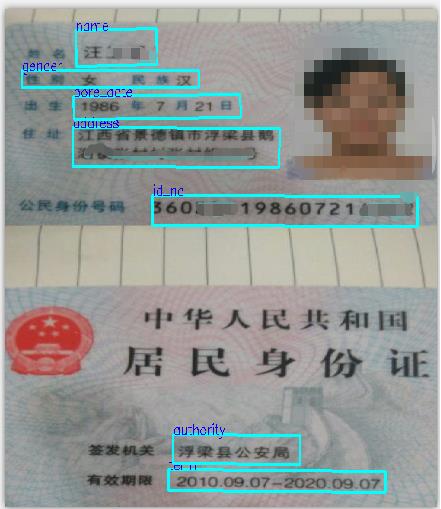
+
+
+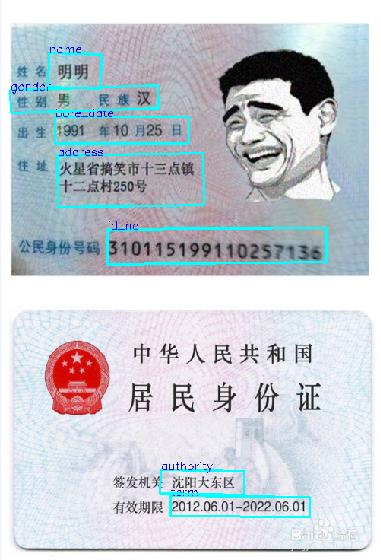
+
+#### 数据标注
+
+使用PaddleOCRLable进行快速标注
+
+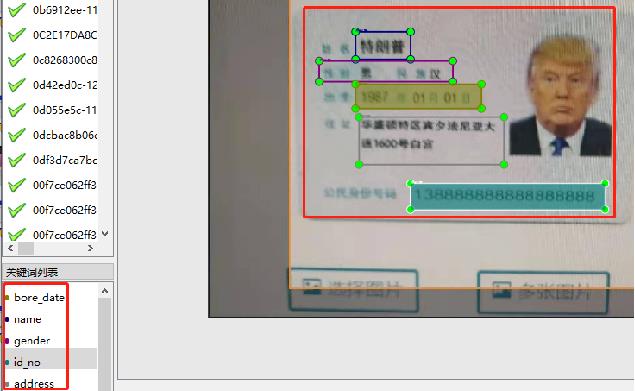
+
+
+* 修改PPOCRLabel.py,将下图中的kie参数设置为True
+
+
+
+
+
+* 数据标注踩坑分享
+
+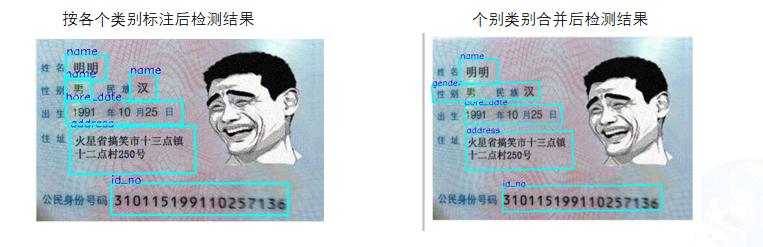
+
+ 注:两者只有标注有差别,训练参数数据集都相同
+
+## 4 . 项目实践
+
+AIStudio项目链接:[快速构建卡证类OCR](https://aistudio.baidu.com/aistudio/projectdetail/4459116)
+
+### 4.1 环境准备
+
+1)拉取[paddleocr](https://github.com/PaddlePaddle/PaddleOCR)项目,如果从github上拉取速度慢可以选择从gitee上获取。
+```
+!git clone https://github.com/PaddlePaddle/PaddleOCR.git -b release/2.6 /home/aistudio/work/
+```
+
+2)获取并解压预训练模型,如果要使用其他模型可以从模型库里自主选择合适模型。
+```
+!wget -P work/pre_trained/ https://paddleocr.bj.bcebos.com/PP-OCRv3/chinese/ch_PP-OCRv3_det_distill_train.tar
+!tar -vxf /home/aistudio/work/pre_trained/ch_PP-OCRv3_det_distill_train.tar -C /home/aistudio/work/pre_trained
+```
+3) 安装必要依赖
+```
+!pip install -r /home/aistudio/work/requirements.txt
+```
+
+### 4.2 配置文件修改
+
+修改配置文件 *work/configs/det/detmv3db.yml*
+
+具体修改说明如下:
+
+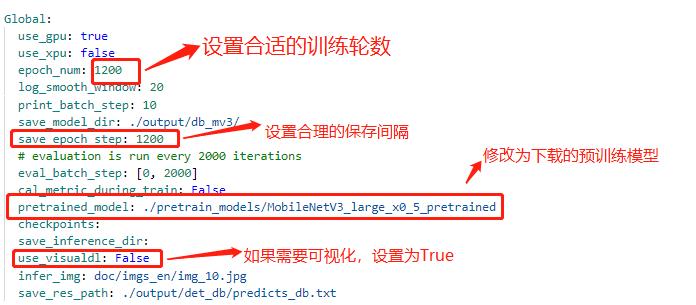
+
+ 注:在上述的配置文件的Global变量中需要添加以下两个参数:
+
+ label_list 为标签表
+ num_classes 为分类数
+ 上述两个参数根据实际的情况配置即可
+
+
+
+
+其中lable_list内容如下例所示,***建议第一个参数设置为 background,不要设置为实际要提取的关键信息种类***:
+
+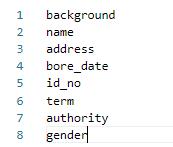
+
+配置文件中的其他设置说明
+
+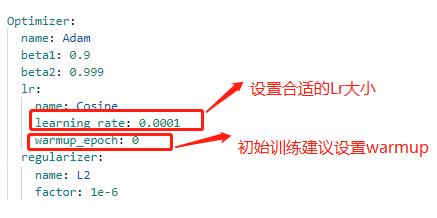
+
+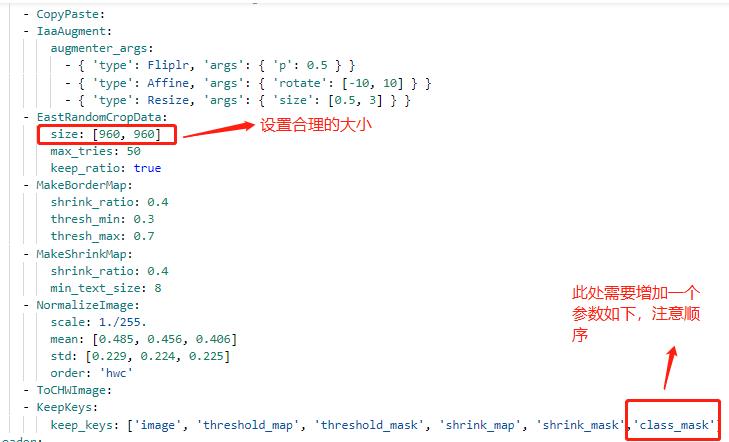
+
+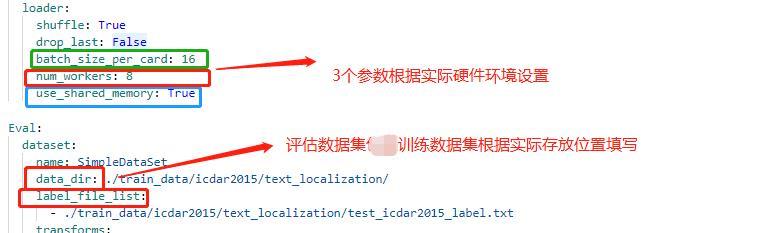
+
+
+
+
+### 4.3 代码修改
+
+
+#### 4.3.1 数据读取
+
+
+
+* 修改 PaddleOCR/ppocr/data/imaug/label_ops.py中的DetLabelEncode
+
+
+```python
+class DetLabelEncode(object):
+
+ # 修改检测标签的编码处,新增了参数分类数:num_classes,重写初始化方法,以及分类标签的读取
+
+ def __init__(self, label_list, num_classes=8, **kwargs):
+ self.num_classes = num_classes
+ self.label_list = []
+ if label_list:
+ if isinstance(label_list, str):
+ with open(label_list, 'r+', encoding='utf-8') as f:
+ for line in f.readlines():
+ self.label_list.append(line.replace("\n", ""))
+ else:
+ self.label_list = label_list
+ else:
+ assert ' please check label_list whether it is none or config is right'
+
+ if num_classes != len(self.label_list): # 校验分类数和标签的一致性
+ assert 'label_list length is not equal to the num_classes'
+
+ def __call__(self, data):
+ label = data['label']
+ label = json.loads(label)
+ nBox = len(label)
+ boxes, txts, txt_tags, classes = [], [], [], []
+ for bno in range(0, nBox):
+ box = label[bno]['points']
+ txt = label[bno]['key_cls'] # 此处将kie中的参数作为分类读取
+ boxes.append(box)
+ txts.append(txt)
+
+ if txt in ['*', '###']:
+ txt_tags.append(True)
+ if self.num_classes > 1:
+ classes.append(-2)
+ else:
+ txt_tags.append(False)
+ if self.num_classes > 1: # 将KIE内容的key标签作为分类标签使用
+ classes.append(int(self.label_list.index(txt)))
+
+ if len(boxes) == 0:
+
+ return None
+ boxes = self.expand_points_num(boxes)
+ boxes = np.array(boxes, dtype=np.float32)
+ txt_tags = np.array(txt_tags, dtype=np.bool)
+ classes = classes
+ data['polys'] = boxes
+ data['texts'] = txts
+ data['ignore_tags'] = txt_tags
+ if self.num_classes > 1:
+ data['classes'] = classes
+ return data
+```
+
+* 修改 PaddleOCR/ppocr/data/imaug/make_shrink_map.py中的MakeShrinkMap类。这里需要注意的是,如果我们设置的label_list中的第一个参数为要检测的信息那么会得到如下的mask,
+
+举例说明:
+这是检测的mask图,图中有四个mask那么实际对应的分类应该是4类
+
+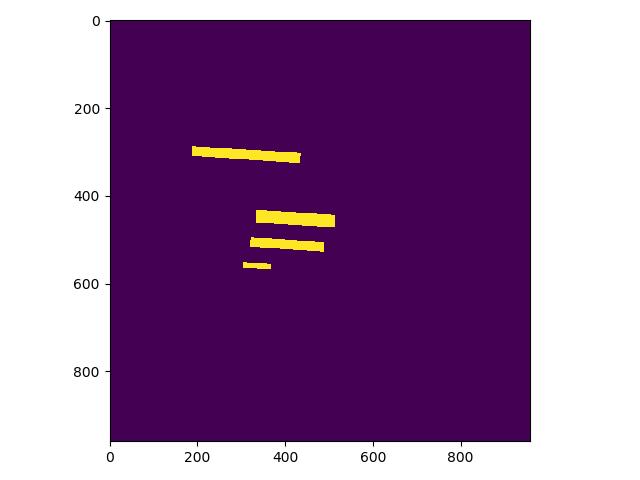
+
+
+
+label_list中第一个为关键分类,则得到的分类Mask实际如下,与上图相比,少了一个box:
+
+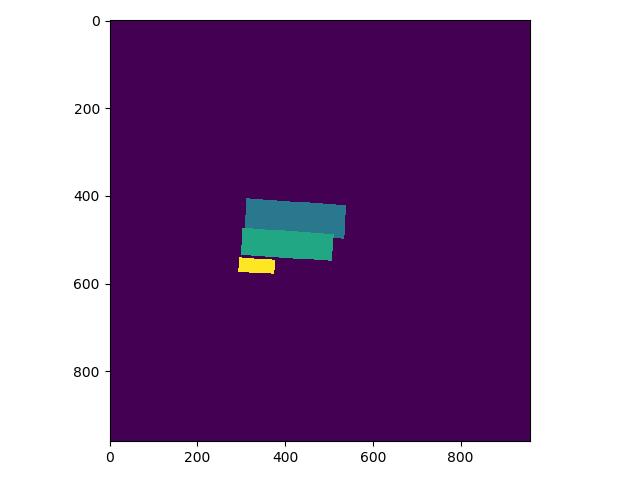
+
+
+
+```python
+class MakeShrinkMap(object):
+ r'''
+ Making binary mask from detection data with ICDAR format.
+ Typically following the process of class `MakeICDARData`.
+ '''
+
+ def __init__(self, min_text_size=8, shrink_ratio=0.4, num_classes=8, **kwargs):
+ self.min_text_size = min_text_size
+ self.shrink_ratio = shrink_ratio
+ self.num_classes = num_classes # 添加了分类
+
+ def __call__(self, data):
+ image = data['image']
+ text_polys = data['polys']
+ ignore_tags = data['ignore_tags']
+ if self.num_classes > 1:
+ classes = data['classes']
+
+ h, w = image.shape[:2]
+ text_polys, ignore_tags = self.validate_polygons(text_polys,
+ ignore_tags, h, w)
+ gt = np.zeros((h, w), dtype=np.float32)
+ mask = np.ones((h, w), dtype=np.float32)
+ gt_class = np.zeros((h, w), dtype=np.float32) # 新增分类
+ for i in range(len(text_polys)):
+ polygon = text_polys[i]
+ height = max(polygon[:, 1]) - min(polygon[:, 1])
+ width = max(polygon[:, 0]) - min(polygon[:, 0])
+ if ignore_tags[i] or min(height, width) < self.min_text_size:
+ cv2.fillPoly(mask,
+ polygon.astype(np.int32)[np.newaxis, :, :], 0)
+ ignore_tags[i] = True
+ else:
+ polygon_shape = Polygon(polygon)
+ subject = [tuple(l) for l in polygon]
+ padding = pyclipper.PyclipperOffset()
+ padding.AddPath(subject, pyclipper.JT_ROUND,
+ pyclipper.ET_CLOSEDPOLYGON)
+ shrinked = []
+
+ # Increase the shrink ratio every time we get multiple polygon returned back
+ possible_ratios = np.arange(self.shrink_ratio, 1,
+ self.shrink_ratio)
+ np.append(possible_ratios, 1)
+ for ratio in possible_ratios:
+ distance = polygon_shape.area * (
+ 1 - np.power(ratio, 2)) / polygon_shape.length
+ shrinked = padding.Execute(-distance)
+ if len(shrinked) == 1:
+ break
+
+ if shrinked == []:
+ cv2.fillPoly(mask,
+ polygon.astype(np.int32)[np.newaxis, :, :], 0)
+ ignore_tags[i] = True
+ continue
+
+ for each_shirnk in shrinked:
+ shirnk = np.array(each_shirnk).reshape(-1, 2)
+ cv2.fillPoly(gt, [shirnk.astype(np.int32)], 1)
+ if self.num_classes > 1: # 绘制分类的mask
+ cv2.fillPoly(gt_class, polygon.astype(np.int32)[np.newaxis, :, :], classes[i])
+
+
+ data['shrink_map'] = gt
+
+ if self.num_classes > 1:
+ data['class_mask'] = gt_class
+
+ data['shrink_mask'] = mask
+ return data
+```
+
+由于在训练数据中会对数据进行resize设置,yml中的操作为:EastRandomCropData,所以需要修改PaddleOCR/ppocr/data/imaug/random_crop_data.py中的EastRandomCropData
+
+
+```python
+class EastRandomCropData(object):
+ def __init__(self,
+ size=(640, 640),
+ max_tries=10,
+ min_crop_side_ratio=0.1,
+ keep_ratio=True,
+ num_classes=8,
+ **kwargs):
+ self.size = size
+ self.max_tries = max_tries
+ self.min_crop_side_ratio = min_crop_side_ratio
+ self.keep_ratio = keep_ratio
+ self.num_classes = num_classes
+
+ def __call__(self, data):
+ img = data['image']
+ text_polys = data['polys']
+ ignore_tags = data['ignore_tags']
+ texts = data['texts']
+ if self.num_classes > 1:
+ classes = data['classes']
+ all_care_polys = [
+ text_polys[i] for i, tag in enumerate(ignore_tags) if not tag
+ ]
+ # 计算crop区域
+ crop_x, crop_y, crop_w, crop_h = crop_area(
+ img, all_care_polys, self.min_crop_side_ratio, self.max_tries)
+ # crop 图片 保持比例填充
+ scale_w = self.size[0] / crop_w
+ scale_h = self.size[1] / crop_h
+ scale = min(scale_w, scale_h)
+ h = int(crop_h * scale)
+ w = int(crop_w * scale)
+ if self.keep_ratio:
+ padimg = np.zeros((self.size[1], self.size[0], img.shape[2]),
+ img.dtype)
+ padimg[:h, :w] = cv2.resize(
+ img[crop_y:crop_y + crop_h, crop_x:crop_x + crop_w], (w, h))
+ img = padimg
+ else:
+ img = cv2.resize(
+ img[crop_y:crop_y + crop_h, crop_x:crop_x + crop_w],
+ tuple(self.size))
+ # crop 文本框
+ text_polys_crop = []
+ ignore_tags_crop = []
+ texts_crop = []
+ classes_crop = []
+ for poly, text, tag,class_index in zip(text_polys, texts, ignore_tags,classes):
+ poly = ((poly - (crop_x, crop_y)) * scale).tolist()
+ if not is_poly_outside_rect(poly, 0, 0, w, h):
+ text_polys_crop.append(poly)
+ ignore_tags_crop.append(tag)
+ texts_crop.append(text)
+ if self.num_classes > 1:
+ classes_crop.append(class_index)
+ data['image'] = img
+ data['polys'] = np.array(text_polys_crop)
+ data['ignore_tags'] = ignore_tags_crop
+ data['texts'] = texts_crop
+ if self.num_classes > 1:
+ data['classes'] = classes_crop
+ return data
+```
+
+#### 4.3.2 head修改
+
+
+
+主要修改 ppocr/modeling/heads/det_db_head.py,将Head类中的最后一层的输出修改为实际的分类数,同时在DBHead中新增分类的head。
+
+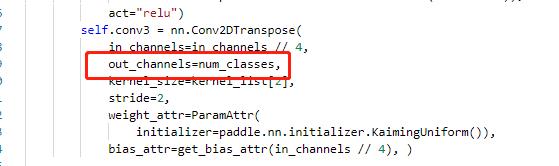
+
+
+
+#### 4.3.3 修改loss
+
+
+修改PaddleOCR/ppocr/losses/det_db_loss.py中的DBLoss类,分类采用交叉熵损失函数进行计算。
+
+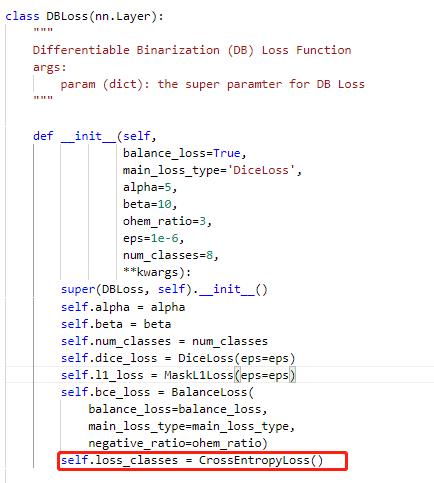
+
+
+#### 4.3.4 后处理
+
+
+
+由于涉及到eval以及后续推理能否正常使用,我们需要修改后处理的相关代码,修改位置 PaddleOCR/ppocr/postprocess/db_postprocess.py中的DBPostProcess类
+
+
+```python
+class DBPostProcess(object):
+ """
+ The post process for Differentiable Binarization (DB).
+ """
+
+ def __init__(self,
+ thresh=0.3,
+ box_thresh=0.7,
+ max_candidates=1000,
+ unclip_ratio=2.0,
+ use_dilation=False,
+ score_mode="fast",
+ **kwargs):
+ self.thresh = thresh
+ self.box_thresh = box_thresh
+ self.max_candidates = max_candidates
+ self.unclip_ratio = unclip_ratio
+ self.min_size = 3
+ self.score_mode = score_mode
+ assert score_mode in [
+ "slow", "fast"
+ ], "Score mode must be in [slow, fast] but got: {}".format(score_mode)
+
+ self.dilation_kernel = None if not use_dilation else np.array(
+ [[1, 1], [1, 1]])
+
+ def boxes_from_bitmap(self, pred, _bitmap, classes, dest_width, dest_height):
+ """
+ _bitmap: single map with shape (1, H, W),
+ whose values are binarized as {0, 1}
+ """
+
+ bitmap = _bitmap
+ height, width = bitmap.shape
+
+ outs = cv2.findContours((bitmap * 255).astype(np.uint8), cv2.RETR_LIST,
+ cv2.CHAIN_APPROX_SIMPLE)
+ if len(outs) == 3:
+ img, contours, _ = outs[0], outs[1], outs[2]
+ elif len(outs) == 2:
+ contours, _ = outs[0], outs[1]
+
+ num_contours = min(len(contours), self.max_candidates)
+
+ boxes = []
+ scores = []
+ class_indexes = []
+ class_scores = []
+ for index in range(num_contours):
+ contour = contours[index]
+ points, sside = self.get_mini_boxes(contour)
+ if sside < self.min_size:
+ continue
+ points = np.array(points)
+ if self.score_mode == "fast":
+ score, class_index, class_score = self.box_score_fast(pred, points.reshape(-1, 2), classes)
+ else:
+ score, class_index, class_score = self.box_score_slow(pred, contour, classes)
+ if self.box_thresh > score:
+ continue
+
+ box = self.unclip(points).reshape(-1, 1, 2)
+ box, sside = self.get_mini_boxes(box)
+ if sside < self.min_size + 2:
+ continue
+ box = np.array(box)
+
+ box[:, 0] = np.clip(
+ np.round(box[:, 0] / width * dest_width), 0, dest_width)
+ box[:, 1] = np.clip(
+ np.round(box[:, 1] / height * dest_height), 0, dest_height)
+
+ boxes.append(box.astype(np.int16))
+ scores.append(score)
+
+ class_indexes.append(class_index)
+ class_scores.append(class_score)
+
+ if classes is None:
+ return np.array(boxes, dtype=np.int16), scores
+ else:
+ return np.array(boxes, dtype=np.int16), scores, class_indexes, class_scores
+
+ def unclip(self, box):
+ unclip_ratio = self.unclip_ratio
+ poly = Polygon(box)
+ distance = poly.area * unclip_ratio / poly.length
+ offset = pyclipper.PyclipperOffset()
+ offset.AddPath(box, pyclipper.JT_ROUND, pyclipper.ET_CLOSEDPOLYGON)
+ expanded = np.array(offset.Execute(distance))
+ return expanded
+
+ def get_mini_boxes(self, contour):
+ bounding_box = cv2.minAreaRect(contour)
+ points = sorted(list(cv2.boxPoints(bounding_box)), key=lambda x: x[0])
+
+ index_1, index_2, index_3, index_4 = 0, 1, 2, 3
+ if points[1][1] > points[0][1]:
+ index_1 = 0
+ index_4 = 1
+ else:
+ index_1 = 1
+ index_4 = 0
+ if points[3][1] > points[2][1]:
+ index_2 = 2
+ index_3 = 3
+ else:
+ index_2 = 3
+ index_3 = 2
+
+ box = [
+ points[index_1], points[index_2], points[index_3], points[index_4]
+ ]
+ return box, min(bounding_box[1])
+
+ def box_score_fast(self, bitmap, _box, classes):
+ '''
+ box_score_fast: use bbox mean score as the mean score
+ '''
+ h, w = bitmap.shape[:2]
+ box = _box.copy()
+ xmin = np.clip(np.floor(box[:, 0].min()).astype(np.int), 0, w - 1)
+ xmax = np.clip(np.ceil(box[:, 0].max()).astype(np.int), 0, w - 1)
+ ymin = np.clip(np.floor(box[:, 1].min()).astype(np.int), 0, h - 1)
+ ymax = np.clip(np.ceil(box[:, 1].max()).astype(np.int), 0, h - 1)
+
+ mask = np.zeros((ymax - ymin + 1, xmax - xmin + 1), dtype=np.uint8)
+ box[:, 0] = box[:, 0] - xmin
+ box[:, 1] = box[:, 1] - ymin
+ cv2.fillPoly(mask, box.reshape(1, -1, 2).astype(np.int32), 1)
+
+ if classes is None:
+ return cv2.mean(bitmap[ymin:ymax + 1, xmin:xmax + 1], mask)[0], None, None
+ else:
+ k = 999
+ class_mask = np.full((ymax - ymin + 1, xmax - xmin + 1), k, dtype=np.int32)
+
+ cv2.fillPoly(class_mask, box.reshape(1, -1, 2).astype(np.int32), 0)
+ classes = classes[ymin:ymax + 1, xmin:xmax + 1]
+
+ new_classes = classes + class_mask
+ a = new_classes.reshape(-1)
+ b = np.where(a >= k)
+ classes = np.delete(a, b[0].tolist())
+
+ class_index = np.argmax(np.bincount(classes))
+ class_score = np.sum(classes == class_index) / len(classes)
+
+ return cv2.mean(bitmap[ymin:ymax + 1, xmin:xmax + 1], mask)[0], class_index, class_score
+
+ def box_score_slow(self, bitmap, contour, classes):
+ """
+ box_score_slow: use polyon mean score as the mean score
+ """
+ h, w = bitmap.shape[:2]
+ contour = contour.copy()
+ contour = np.reshape(contour, (-1, 2))
+
+ xmin = np.clip(np.min(contour[:, 0]), 0, w - 1)
+ xmax = np.clip(np.max(contour[:, 0]), 0, w - 1)
+ ymin = np.clip(np.min(contour[:, 1]), 0, h - 1)
+ ymax = np.clip(np.max(contour[:, 1]), 0, h - 1)
+
+ mask = np.zeros((ymax - ymin + 1, xmax - xmin + 1), dtype=np.uint8)
+
+ contour[:, 0] = contour[:, 0] - xmin
+ contour[:, 1] = contour[:, 1] - ymin
+
+ cv2.fillPoly(mask, contour.reshape(1, -1, 2).astype(np.int32), 1)
+
+ if classes is None:
+ return cv2.mean(bitmap[ymin:ymax + 1, xmin:xmax + 1], mask)[0], None, None
+ else:
+ k = 999
+ class_mask = np.full((ymax - ymin + 1, xmax - xmin + 1), k, dtype=np.int32)
+
+ cv2.fillPoly(class_mask, contour.reshape(1, -1, 2).astype(np.int32), 0)
+ classes = classes[ymin:ymax + 1, xmin:xmax + 1]
+
+ new_classes = classes + class_mask
+ a = new_classes.reshape(-1)
+ b = np.where(a >= k)
+ classes = np.delete(a, b[0].tolist())
+
+ class_index = np.argmax(np.bincount(classes))
+ class_score = np.sum(classes == class_index) / len(classes)
+
+ return cv2.mean(bitmap[ymin:ymax + 1, xmin:xmax + 1], mask)[0], class_index, class_score
+
+ def __call__(self, outs_dict, shape_list):
+ pred = outs_dict['maps']
+ if isinstance(pred, paddle.Tensor):
+ pred = pred.numpy()
+ pred = pred[:, 0, :, :]
+ segmentation = pred > self.thresh
+
+ if "classes" in outs_dict:
+ classes = outs_dict['classes']
+ if isinstance(classes, paddle.Tensor):
+ classes = classes.numpy()
+ classes = classes[:, 0, :, :]
+
+ else:
+ classes = None
+
+ boxes_batch = []
+ for batch_index in range(pred.shape[0]):
+ src_h, src_w, ratio_h, ratio_w = shape_list[batch_index]
+ if self.dilation_kernel is not None:
+ mask = cv2.dilate(
+ np.array(segmentation[batch_index]).astype(np.uint8),
+ self.dilation_kernel)
+ else:
+ mask = segmentation[batch_index]
+
+ if classes is None:
+ boxes, scores = self.boxes_from_bitmap(pred[batch_index], mask, None,
+ src_w, src_h)
+ boxes_batch.append({'points': boxes})
+ else:
+ boxes, scores, class_indexes, class_scores = self.boxes_from_bitmap(pred[batch_index], mask,
+ classes[batch_index],
+ src_w, src_h)
+ boxes_batch.append({'points': boxes, "classes": class_indexes, "class_scores": class_scores})
+
+ return boxes_batch
+```
+
+### 4.4. 模型启动
+
+在完成上述步骤后我们就可以正常启动训练
+
+```
+!python /home/aistudio/work/PaddleOCR/tools/train.py -c /home/aistudio/work/PaddleOCR/configs/det/det_mv3_db.yml
+```
+
+其他命令:
+```
+!python /home/aistudio/work/PaddleOCR/tools/eval.py -c /home/aistudio/work/PaddleOCR/configs/det/det_mv3_db.yml
+!python /home/aistudio/work/PaddleOCR/tools/infer_det.py -c /home/aistudio/work/PaddleOCR/configs/det/det_mv3_db.yml
+```
+模型推理
+```
+!python /home/aistudio/work/PaddleOCR/tools/infer/predict_det.py --image_dir="/home/aistudio/work/test_img/" --det_model_dir="/home/aistudio/work/PaddleOCR/output/infer"
+```
+
+## 5 总结
+
+1. 分类+检测在一定程度上能够缩短用时,具体的模型选取要根据业务场景恰当选择。
+2. 数据标注需要多次进行测试调整标注方法,一般进行检测模型微调,需要标注至少上百张。
+3. 设置合理的batch_size以及resize大小,同时注意lr设置。
+
+
+## References
+
+1 https://github.com/PaddlePaddle/PaddleOCR
+
+2 https://github.com/PaddlePaddle/PaddleClas
+
+3 https://blog.csdn.net/YY007H/article/details/124491217
diff --git "a/applications/\346\211\253\346\217\217\345\220\210\345\220\214\345\205\263\351\224\256\344\277\241\346\201\257\346\217\220\345\217\226.md" "b/applications/\346\211\253\346\217\217\345\220\210\345\220\214\345\205\263\351\224\256\344\277\241\346\201\257\346\217\220\345\217\226.md"
new file mode 100644
index 0000000000000000000000000000000000000000..26c64a34c14e621b4bc15df67c66141024bf7cc4
--- /dev/null
+++ "b/applications/\346\211\253\346\217\217\345\220\210\345\220\214\345\205\263\351\224\256\344\277\241\346\201\257\346\217\220\345\217\226.md"
@@ -0,0 +1,284 @@
+# 金融智能核验:扫描合同关键信息抽取
+
+本案例将使用OCR技术和通用信息抽取技术,实现合同关键信息审核和比对。通过本章的学习,你可以快速掌握:
+
+1. 使用PaddleOCR提取扫描文本内容
+2. 使用PaddleNLP抽取自定义信息
+
+点击进入 [AI Studio 项目](https://aistudio.baidu.com/aistudio/projectdetail/4545772)
+
+## 1. 项目背景
+合同审核广泛应用于大中型企业、上市公司、证券、基金公司中,是规避风险的重要任务。
+- 合同内容对比:合同审核场景中,快速找出不同版本合同修改区域、版本差异;如合同盖章归档场景中有效识别实际签署的纸质合同、电子版合同差异。
+
+- 合规性检查:法务人员进行合同审核,如合同完备性检查、大小写金额检查、签约主体一致性检查、双方权利和义务对等性分析等。
+
+- 风险点识别:通过合同审核可识别事实倾向型风险点和数值计算型风险点等,例如交付地点约定不明、合同总价款不一致、重要条款缺失等风险点。
+
+
+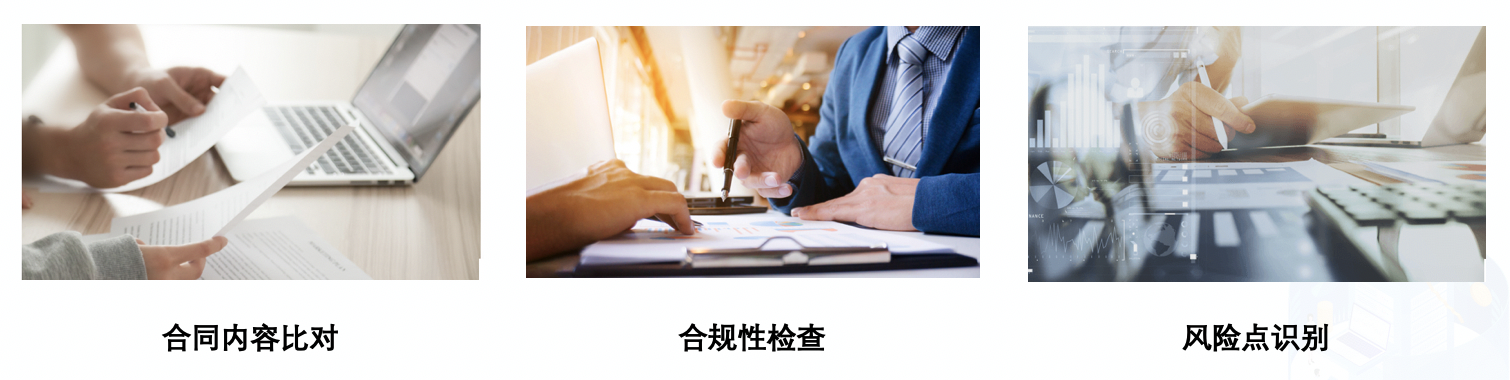
+
+传统业务中大多使用人工进行纸质版合同审核,存在成本高,工作量大,效率低的问题,且一旦出错将造成巨额损失。
+
+
+本项目针对以上场景,使用PaddleOCR+PaddleNLP快速提取文本内容,经过少量数据微调即可准确抽取关键信息,**高效完成合同内容对比、合规性检查、风险点识别等任务,提高效率,降低风险**。
+
+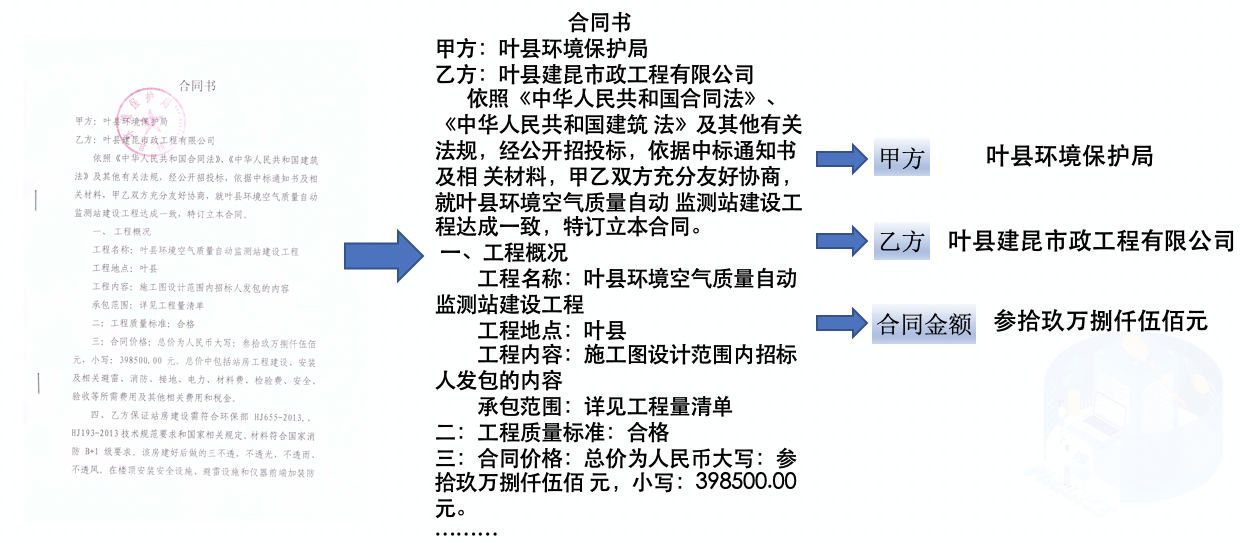
+
+
+## 2. 解决方案
+
+### 2.1 扫描合同文本内容提取
+
+使用PaddleOCR开源的模型可以快速完成扫描文档的文本内容提取,在清晰文档上识别准确率可达到95%+。下面来快速体验一下:
+
+#### 2.1.1 环境准备
+
+[PaddleOCR](https://github.com/PaddlePaddle/PaddleOCR)提供了适用于通用场景的高精轻量模型,提供数据预处理-模型推理-后处理全流程,支持pip安装:
+
+```
+python -m pip install paddleocr
+```
+
+#### 2.1.2 效果测试
+
+使用一张合同图片作为测试样本,感受ppocrv3模型效果:
+
+
+
+使用中文检测+识别模型提取文本,实例化PaddleOCR类:
+
+```
+from paddleocr import PaddleOCR, draw_ocr
+
+# paddleocr目前支持中英文、英文、法语、德语、韩语、日语等80个语种,可以通过修改lang参数进行切换
+ocr = PaddleOCR(use_angle_cls=False, lang="ch") # need to run only once to download and load model into memory
+```
+
+一行命令启动预测,预测结果包括`检测框`和`文本识别内容`:
+
+```
+img_path = "./test_img/hetong2.jpg"
+result = ocr.ocr(img_path, cls=False)
+for line in result:
+ print(line)
+
+# 可视化结果
+from PIL import Image
+
+image = Image.open(img_path).convert('RGB')
+boxes = [line[0] for line in result]
+txts = [line[1][0] for line in result]
+scores = [line[1][1] for line in result]
+im_show = draw_ocr(image, boxes, txts, scores, font_path='./simfang.ttf')
+im_show = Image.fromarray(im_show)
+im_show.show()
+```
+
+#### 2.1.3 图片预处理
+
+通过上图可视化结果可以看到,印章部分造成的文本遮盖,影响了文本识别结果,因此可以考虑通道提取,去除图片中的红色印章:
+
+```
+import cv2
+import numpy as np
+import matplotlib.pyplot as plt
+
+#读入图像,三通道
+image=cv2.imread("./test_img/hetong2.jpg",cv2.IMREAD_COLOR) #timg.jpeg
+
+#获得三个通道
+Bch,Gch,Rch=cv2.split(image)
+
+#保存三通道图片
+cv2.imwrite('blue_channel.jpg',Bch)
+cv2.imwrite('green_channel.jpg',Gch)
+cv2.imwrite('red_channel.jpg',Rch)
+```
+#### 2.1.4 合同文本信息提取
+
+经过2.1.3的预处理后,合同照片的红色通道被分离,获得了一张相对更干净的图片,此时可以再次使用ppocr模型提取文本内容:
+
+```
+import numpy as np
+import cv2
+
+
+img_path = './red_channel.jpg'
+result = ocr.ocr(img_path, cls=False)
+
+# 可视化结果
+from PIL import Image
+
+image = Image.open(img_path).convert('RGB')
+boxes = [line[0] for line in result]
+txts = [line[1][0] for line in result]
+scores = [line[1][1] for line in result]
+im_show = draw_ocr(image, boxes, txts, scores, font_path='./simfang.ttf')
+im_show = Image.fromarray(im_show)
+vis = np.array(im_show)
+im_show.show()
+```
+
+忽略检测框内容,提取完整的合同文本:
+
+```
+txts = [line[1][0] for line in result]
+all_context = "\n".join(txts)
+print(all_context)
+```
+
+通过以上环节就完成了扫描合同关键信息抽取的第一步:文本内容提取,接下来可以基于识别出的文本内容抽取关键信息
+
+### 2.2 合同关键信息抽取
+
+#### 2.2.1 环境准备
+
+安装PaddleNLP
+
+
+```
+pip install --upgrade pip
+pip install --upgrade paddlenlp
+```
+
+#### 2.2.2 合同关键信息抽取
+
+PaddleNLP 使用 Taskflow 统一管理多场景任务的预测功能,其中`information_extraction` 通过大量的有标签样本进行训练,在通用的场景中一般可以直接使用,只需更换关键字即可。例如在合同信息抽取中,我们重新定义抽取关键字:
+
+甲方、乙方、币种、金额、付款方式
+
+
+将使用OCR提取好的文本作为输入,使用三行命令可以对上文中提取到的合同文本进行关键信息抽取:
+
+```
+from paddlenlp import Taskflow
+schema = ["甲方","乙方","总价"]
+ie = Taskflow('information_extraction', schema=schema)
+ie.set_schema(schema)
+ie(all_context)
+```
+
+可以看到UIE模型可以准确的提取出关键信息,用于后续的信息比对或审核。
+
+## 3.效果优化
+
+### 3.1 文本识别后处理调优
+
+实际图片采集过程中,可能出现部分图片弯曲等问题,导致使用默认参数识别文本时存在漏检,影响关键信息获取。
+
+例如下图:
+
+
+
+
+直接进行预测:
+
+```
+img_path = "./test_img/hetong3.jpg"
+# 预测结果
+result = ocr.ocr(img_path, cls=False)
+# 可视化结果
+from PIL import Image
+
+image = Image.open(img_path).convert('RGB')
+boxes = [line[0] for line in result]
+txts = [line[1][0] for line in result]
+scores = [line[1][1] for line in result]
+im_show = draw_ocr(image, boxes, txts, scores, font_path='./simfang.ttf')
+im_show = Image.fromarray(im_show)
+im_show.show()
+```
+
+可视化结果可以看到,弯曲图片存在漏检,一般来说可以通过调整后处理参数解决,无需重新训练模型。漏检问题往往是因为检测模型获得的分割图太小,生成框的得分过低被过滤掉了,通常有两种方式调整参数:
+- 开启`use_dilatiion=True` 膨胀分割区域
+- 调小`det_db_box_thresh`阈值
+
+```
+# 重新实例化 PaddleOCR
+ocr = PaddleOCR(use_angle_cls=False, lang="ch", det_db_box_thresh=0.3, use_dilation=True)
+
+# 预测并可视化
+img_path = "./test_img/hetong3.jpg"
+# 预测结果
+result = ocr.ocr(img_path, cls=False)
+# 可视化结果
+image = Image.open(img_path).convert('RGB')
+boxes = [line[0] for line in result]
+txts = [line[1][0] for line in result]
+scores = [line[1][1] for line in result]
+im_show = draw_ocr(image, boxes, txts, scores, font_path='./simfang.ttf')
+im_show = Image.fromarray(im_show)
+im_show.show()
+```
+
+可以看到漏检问题被很好的解决,提取完整的文本内容:
+
+```
+txts = [line[1][0] for line in result]
+context = "\n".join(txts)
+print(context)
+```
+
+### 3.2 关键信息提取调优
+
+UIE通过大量有标签样本进行训练,得到了一个开箱即用的高精模型。 然而针对不同场景,可能会出现部分实体无法被抽取的情况。通常来说有以下几个方法进行效果调优:
+
+
+- 修改 schema
+- 添加正则方法
+- 标注小样本微调模型
+
+**修改schema**
+
+Prompt和原文描述越像,抽取效果越好,例如
+```
+三:合同价格:总价为人民币大写:参拾玖万捌仟伍佰
+元,小写:398500.00元。总价中包括站房工程建设、安装
+及相关避雷、消防、接地、电力、材料费、检验费、安全、
+验收等所需费用及其他相关费用和税金。
+```
+schema = ["总金额"] 时无法准确抽取,与原文描述差异较大。 修改 schema = ["总价"] 再次尝试:
+
+```
+from paddlenlp import Taskflow
+# schema = ["总金额"]
+schema = ["总价"]
+ie = Taskflow('information_extraction', schema=schema)
+ie.set_schema(schema)
+ie(all_context)
+```
+
+
+**模型微调**
+
+UIE的建模方式主要是通过 `Prompt` 方式来建模, `Prompt` 在小样本上进行微调效果非常有效。详细的数据标注+模型微调步骤可以参考项目:
+
+[PaddleNLP信息抽取技术重磅升级!](https://aistudio.baidu.com/aistudio/projectdetail/3914778?channelType=0&channel=0)
+
+[工单信息抽取](https://aistudio.baidu.com/aistudio/projectdetail/3914778?contributionType=1)
+
+[快递单信息抽取](https://aistudio.baidu.com/aistudio/projectdetail/4038499?contributionType=1)
+
+
+## 总结
+
+扫描合同的关键信息提取可以使用 PaddleOCR + PaddleNLP 组合实现,两个工具均有以下优势:
+
+* 使用简单:whl包一键安装,3行命令调用
+* 效果领先:优秀的模型效果可覆盖几乎全部的应用场景
+* 调优成本低:OCR模型可通过后处理参数的调整适配略有偏差的扫描文本, UIE模型可以通过极少的标注样本微调,成本很低。
+
+## 作业
+
+尝试自己解析出 `test_img/homework.png` 扫描合同中的 [甲方、乙方] 关键词:
+
+
+
+
+
+
+
+更多场景下的垂类模型获取,请扫下图二维码填写问卷,加入PaddleOCR官方交流群获取模型下载链接、《动手学OCR》电子书等全套OCR学习资料🎁
+
+
diff --git a/deploy/paddle2onnx/readme.md b/deploy/paddle2onnx/readme.md
index 8e821892142d65caddd6fa3bd8ff24a372fe9a5d..bac29784413d51eb8f7c10ed1b78187454e038d7 100644
--- a/deploy/paddle2onnx/readme.md
+++ b/deploy/paddle2onnx/readme.md
@@ -1,63 +1,64 @@
-# Paddle2ONNX模型转化与预测
+# Paddle2ONNX model transformation and prediction
-本章节介绍 PaddleOCR 模型如何转化为 ONNX 模型,并基于 ONNXRuntime 引擎预测。
+This chapter describes how the PaddleOCR model is converted into an ONNX model and predicted based on the ONNXRuntime engine.
-## 1. 环境准备
+## 1. Environment preparation
-需要准备 PaddleOCR、Paddle2ONNX 模型转化环境,和 ONNXRuntime 预测环境
+Need to prepare PaddleOCR, Paddle2ONNX model conversion environment, and ONNXRuntime prediction environment
### PaddleOCR
-克隆PaddleOCR的仓库,使用release/2.4分支,并进行安装,由于PaddleOCR仓库比较大,git clone速度比较慢,所以本教程已下载
+Clone the PaddleOCR repository, use the release/2.6 branch, and install it.
```
-git clone -b release/2.4 https://github.com/PaddlePaddle/PaddleOCR.git
+git clone -b release/2.6 https://github.com/PaddlePaddle/PaddleOCR.git
cd PaddleOCR && python3.7 setup.py install
```
### Paddle2ONNX
-Paddle2ONNX 支持将 PaddlePaddle 模型格式转化到 ONNX 模型格式,算子目前稳定支持导出 ONNX Opset 9~11,部分Paddle算子支持更低的ONNX Opset转换。
-更多细节可参考 [Paddle2ONNX](https://github.com/PaddlePaddle/Paddle2ONNX/blob/develop/README_zh.md)
+Paddle2ONNX supports converting the PaddlePaddle model format to the ONNX model format. The operator currently supports exporting ONNX Opset 9~11 stably, and some Paddle operators support lower ONNX Opset conversion.
+For more details, please refer to [Paddle2ONNX](https://github.com/PaddlePaddle/Paddle2ONNX/blob/develop/README_en.md)
-- 安装 Paddle2ONNX
+
+- install Paddle2ONNX
```
python3.7 -m pip install paddle2onnx
```
-- 安装 ONNXRuntime
+- install ONNXRuntime
```
-# 建议安装 1.9.0 版本,可根据环境更换版本号
+# It is recommended to install version 1.9.0, and the version number can be changed according to the environment
python3.7 -m pip install onnxruntime==1.9.0
```
-## 2. 模型转换
+## 2. Model conversion
-- Paddle 模型下载
+- Paddle model download
-有两种方式获取Paddle静态图模型:在 [model_list](../../doc/doc_ch/models_list.md) 中下载PaddleOCR提供的预测模型;
-参考[模型导出说明](../../doc/doc_ch/inference.md#训练模型转inference模型)把训练好的权重转为 inference_model。
+There are two ways to obtain the Paddle model: Download the prediction model provided by PaddleOCR in [model_list](../../doc/doc_en/models_list_en.md);
+Refer to [Model Export Instructions](../../doc/doc_en/inference_en.md#1-convert-training-model-to-inference-model) to convert the trained weights to inference_model.
-以 ppocr 中文检测、识别、分类模型为例:
+Take the PP-OCRv3 detection, recognition, and classification model as an example:
```
-wget -nc -P ./inference https://paddleocr.bj.bcebos.com/PP-OCRv2/chinese/ch_PP-OCRv2_det_infer.tar
-cd ./inference && tar xf ch_PP-OCRv2_det_infer.tar && cd ..
+wget -nc -P ./inference https://paddleocr.bj.bcebos.com/PP-OCRv3/english/en_PP-OCRv3_det_infer.tar
+cd ./inference && tar xf en_PP-OCRv3_det_infer.tar && cd ..
-wget -nc -P ./inference https://paddleocr.bj.bcebos.com/PP-OCRv2/chinese/ch_PP-OCRv2_rec_infer.tar
-cd ./inference && tar xf ch_PP-OCRv2_rec_infer.tar && cd ..
+wget -nc -P ./inference https://paddleocr.bj.bcebos.com/PP-OCRv3/english/en_PP-OCRv3_rec_infer.tar
+cd ./inference && tar xf en_PP-OCRv3_rec_infer.tar && cd ..
wget -nc -P ./inference https://paddleocr.bj.bcebos.com/dygraph_v2.0/ch/ch_ppocr_mobile_v2.0_cls_infer.tar
cd ./inference && tar xf ch_ppocr_mobile_v2.0_cls_infer.tar && cd ..
```
-- 模型转换
+- convert model
-使用 Paddle2ONNX 将Paddle静态图模型转换为ONNX模型格式:
+Convert Paddle inference model to ONNX model format using Paddle2ONNX:
```
-paddle2onnx --model_dir ./inference/ch_PP-OCRv2_det_infer \
+paddle2onnx --model_dir ./inference/en_PP-OCRv3_det_infer \
--model_filename inference.pdmodel \
--params_filename inference.pdiparams \
--save_file ./inference/det_onnx/model.onnx \
@@ -65,7 +66,7 @@ paddle2onnx --model_dir ./inference/ch_PP-OCRv2_det_infer \
--input_shape_dict="{'x':[-1,3,-1,-1]}" \
--enable_onnx_checker True
-paddle2onnx --model_dir ./inference/ch_PP-OCRv2_rec_infer \
+paddle2onnx --model_dir ./inference/en_PP-OCRv3_rec_infer \
--model_filename inference.pdmodel \
--params_filename inference.pdiparams \
--save_file ./inference/rec_onnx/model.onnx \
@@ -81,136 +82,89 @@ paddle2onnx --model_dir ./inference/ch_ppocr_mobile_v2.0_cls_infer \
--input_shape_dict="{'x':[-1,3,-1,-1]}" \
--enable_onnx_checker True
```
+After execution, the ONNX model will be saved in `./inference/det_onnx/`, `./inference/rec_onnx/`, `./inference/cls_onnx/` paths respectively
-执行完毕后,ONNX 模型会被分别保存在 `./inference/det_onnx/`,`./inference/rec_onnx/`,`./inference/cls_onnx/`路径下
-
-* 注意:对于OCR模型,转化过程中必须采用动态shape的形式,即加入选项--input_shape_dict="{'x': [-1, 3, -1, -1]}",否则预测结果可能与直接使用Paddle预测有细微不同。
- 另外,以下几个模型暂不支持转换为 ONNX 模型:
- NRTR、SAR、RARE、SRN
+* Note: For the OCR model, the conversion process must be in the form of dynamic shape, that is, add the option --input_shape_dict="{'x': [-1, 3, -1, -1]}", otherwise the prediction result may be the same as Predicting directly with Paddle is slightly different.
+ In addition, the following models do not currently support conversion to ONNX models:
+ NRTR, SAR, RARE, SRN
-## 3. 推理预测
+## 3. prediction
-以中文OCR模型为例,使用 ONNXRuntime 预测可执行如下命令:
+Take the English OCR model as an example, use **ONNXRuntime** to predict and execute the following commands:
```
python3.7 tools/infer/predict_system.py --use_gpu=False --use_onnx=True \
--det_model_dir=./inference/det_onnx/model.onnx \
--rec_model_dir=./inference/rec_onnx/model.onnx \
--cls_model_dir=./inference/cls_onnx/model.onnx \
---image_dir=./deploy/lite/imgs/lite_demo.png
+--image_dir=doc/imgs_en/img_12.jpg \
+--rec_char_dict_path=ppocr/utils/en_dict.txt
```
-以中文OCR模型为例,使用 Paddle Inference 预测可执行如下命令:
+Taking the English OCR model as an example, use **Paddle Inference** to predict and execute the following commands:
```
python3.7 tools/infer/predict_system.py --use_gpu=False \
--cls_model_dir=./inference/ch_ppocr_mobile_v2.0_cls_infer \
---rec_model_dir=./inference/ch_PP-OCRv2_rec_infer \
---det_model_dir=./inference/ch_PP-OCRv2_det_infer \
---image_dir=./deploy/lite/imgs/lite_demo.png
+--rec_model_dir=./inference/en_PP-OCRv3_rec_infer \
+--det_model_dir=./inference/en_PP-OCRv3_det_infer \
+--image_dir=doc/imgs_en/img_12.jpg \
+--rec_char_dict_path=ppocr/utils/en_dict.txt
```
-执行命令后在终端会打印出预测的识别信息,并在 `./inference_results/` 下保存可视化结果。
+After executing the command, the predicted identification information will be printed out in the terminal, and the visualization results will be saved under `./inference_results/`.
-ONNXRuntime 执行效果:
+ONNXRuntime result:
-
+
-Paddle Inference 执行效果:
+Paddle Inference result:
-
+
-使用 ONNXRuntime 预测,终端输出:
-```
-[2022/02/22 17:48:27] root DEBUG: dt_boxes num : 38, elapse : 0.043187856674194336
-[2022/02/22 17:48:27] root DEBUG: rec_res num : 38, elapse : 0.592170000076294
-[2022/02/22 17:48:27] root DEBUG: 0 Predict time of ./deploy/lite/imgs/lite_demo.png: 0.642s
-[2022/02/22 17:48:27] root DEBUG: The, 0.984
-[2022/02/22 17:48:27] root DEBUG: visualized, 0.882
-[2022/02/22 17:48:27] root DEBUG: etect18片, 0.720
-[2022/02/22 17:48:27] root DEBUG: image saved in./vis.jpg, 0.947
-[2022/02/22 17:48:27] root DEBUG: 纯臻营养护发素0.993604, 0.996
-[2022/02/22 17:48:27] root DEBUG: 产品信息/参数, 0.922
-[2022/02/22 17:48:27] root DEBUG: 0.992728, 0.914
-[2022/02/22 17:48:27] root DEBUG: (45元/每公斤,100公斤起订), 0.926
-[2022/02/22 17:48:27] root DEBUG: 0.97417, 0.977
-[2022/02/22 17:48:27] root DEBUG: 每瓶22元,1000瓶起订)0.993976, 0.962
-[2022/02/22 17:48:27] root DEBUG: 【品牌】:代加工方式/0EMODM, 0.945
-[2022/02/22 17:48:27] root DEBUG: 0.985133, 0.980
-[2022/02/22 17:48:27] root DEBUG: 【品名】:纯臻营养护发素, 0.921
-[2022/02/22 17:48:27] root DEBUG: 0.995007, 0.883
-[2022/02/22 17:48:27] root DEBUG: 【产品编号】:YM-X-30110.96899, 0.955
-[2022/02/22 17:48:27] root DEBUG: 【净含量】:220ml, 0.943
-[2022/02/22 17:48:27] root DEBUG: Q.996577, 0.932
-[2022/02/22 17:48:27] root DEBUG: 【适用人群】:适合所有肤质, 0.913
-[2022/02/22 17:48:27] root DEBUG: 0.995842, 0.969
-[2022/02/22 17:48:27] root DEBUG: 【主要成分】:鲸蜡硬脂醇、燕麦B-葡聚, 0.883
-[2022/02/22 17:48:27] root DEBUG: 0.961928, 0.964
-[2022/02/22 17:48:27] root DEBUG: 10, 0.812
-[2022/02/22 17:48:27] root DEBUG: 糖、椰油酰胺丙基甜菜碱、泛醒, 0.866
-[2022/02/22 17:48:27] root DEBUG: 0.925898, 0.943
-[2022/02/22 17:48:27] root DEBUG: (成品包材), 0.974
-[2022/02/22 17:48:27] root DEBUG: 0.972573, 0.961
-[2022/02/22 17:48:27] root DEBUG: 【主要功能】:可紧致头发磷层,从而达到, 0.936
-[2022/02/22 17:48:27] root DEBUG: 0.994448, 0.952
-[2022/02/22 17:48:27] root DEBUG: 13, 0.998
-[2022/02/22 17:48:27] root DEBUG: 即时持久改善头发光泽的效果,给干燥的头, 0.994
-[2022/02/22 17:48:27] root DEBUG: 0.990198, 0.975
-[2022/02/22 17:48:27] root DEBUG: 14, 0.977
-[2022/02/22 17:48:27] root DEBUG: 发足够的滋养, 0.991
-[2022/02/22 17:48:27] root DEBUG: 0.997668, 0.918
-[2022/02/22 17:48:27] root DEBUG: 花费了0.457335秒, 0.901
-[2022/02/22 17:48:27] root DEBUG: The visualized image saved in ./inference_results/lite_demo.png
-[2022/02/22 17:48:27] root INFO: The predict total time is 0.7003889083862305
-```
-
-使用 Paddle Inference 预测,终端输出:
-
-```
-[2022/02/22 17:47:25] root DEBUG: dt_boxes num : 38, elapse : 0.11791276931762695
-[2022/02/22 17:47:27] root DEBUG: rec_res num : 38, elapse : 2.6206860542297363
-[2022/02/22 17:47:27] root DEBUG: 0 Predict time of ./deploy/lite/imgs/lite_demo.png: 2.746s
-[2022/02/22 17:47:27] root DEBUG: The, 0.984
-[2022/02/22 17:47:27] root DEBUG: visualized, 0.882
-[2022/02/22 17:47:27] root DEBUG: etect18片, 0.720
-[2022/02/22 17:47:27] root DEBUG: image saved in./vis.jpg, 0.947
-[2022/02/22 17:47:27] root DEBUG: 纯臻营养护发素0.993604, 0.996
-[2022/02/22 17:47:27] root DEBUG: 产品信息/参数, 0.922
-[2022/02/22 17:47:27] root DEBUG: 0.992728, 0.914
-[2022/02/22 17:47:27] root DEBUG: (45元/每公斤,100公斤起订), 0.926
-[2022/02/22 17:47:27] root DEBUG: 0.97417, 0.977
-[2022/02/22 17:47:27] root DEBUG: 每瓶22元,1000瓶起订)0.993976, 0.962
-[2022/02/22 17:47:27] root DEBUG: 【品牌】:代加工方式/0EMODM, 0.945
-[2022/02/22 17:47:27] root DEBUG: 0.985133, 0.980
-[2022/02/22 17:47:27] root DEBUG: 【品名】:纯臻营养护发素, 0.921
-[2022/02/22 17:47:27] root DEBUG: 0.995007, 0.883
-[2022/02/22 17:47:27] root DEBUG: 【产品编号】:YM-X-30110.96899, 0.955
-[2022/02/22 17:47:27] root DEBUG: 【净含量】:220ml, 0.943
-[2022/02/22 17:47:27] root DEBUG: Q.996577, 0.932
-[2022/02/22 17:47:27] root DEBUG: 【适用人群】:适合所有肤质, 0.913
-[2022/02/22 17:47:27] root DEBUG: 0.995842, 0.969
-[2022/02/22 17:47:27] root DEBUG: 【主要成分】:鲸蜡硬脂醇、燕麦B-葡聚, 0.883
-[2022/02/22 17:47:27] root DEBUG: 0.961928, 0.964
-[2022/02/22 17:47:27] root DEBUG: 10, 0.812
-[2022/02/22 17:47:27] root DEBUG: 糖、椰油酰胺丙基甜菜碱、泛醒, 0.866
-[2022/02/22 17:47:27] root DEBUG: 0.925898, 0.943
-[2022/02/22 17:47:27] root DEBUG: (成品包材), 0.974
-[2022/02/22 17:47:27] root DEBUG: 0.972573, 0.961
-[2022/02/22 17:47:27] root DEBUG: 【主要功能】:可紧致头发磷层,从而达到, 0.936
-[2022/02/22 17:47:27] root DEBUG: 0.994448, 0.952
-[2022/02/22 17:47:27] root DEBUG: 13, 0.998
-[2022/02/22 17:47:27] root DEBUG: 即时持久改善头发光泽的效果,给干燥的头, 0.994
-[2022/02/22 17:47:27] root DEBUG: 0.990198, 0.975
-[2022/02/22 17:47:27] root DEBUG: 14, 0.977
-[2022/02/22 17:47:27] root DEBUG: 发足够的滋养, 0.991
-[2022/02/22 17:47:27] root DEBUG: 0.997668, 0.918
-[2022/02/22 17:47:27] root DEBUG: 花费了0.457335秒, 0.901
-[2022/02/22 17:47:27] root DEBUG: The visualized image saved in ./inference_results/lite_demo.png
-[2022/02/22 17:47:27] root INFO: The predict total time is 2.8338775634765625
+Using ONNXRuntime to predict, terminal output:
+```
+[2022/10/10 12:06:28] ppocr DEBUG: dt_boxes num : 11, elapse : 0.3568880558013916
+[2022/10/10 12:06:31] ppocr DEBUG: rec_res num : 11, elapse : 2.6445000171661377
+[2022/10/10 12:06:31] ppocr DEBUG: 0 Predict time of doc/imgs_en/img_12.jpg: 3.021s
+[2022/10/10 12:06:31] ppocr DEBUG: ACKNOWLEDGEMENTS, 0.997
+[2022/10/10 12:06:31] ppocr DEBUG: We would like to thank all the designers and, 0.976
+[2022/10/10 12:06:31] ppocr DEBUG: contributors who have been involved in the, 0.979
+[2022/10/10 12:06:31] ppocr DEBUG: production of this book; their contributions, 0.989
+[2022/10/10 12:06:31] ppocr DEBUG: have been indispensable to its creation. We, 0.956
+[2022/10/10 12:06:31] ppocr DEBUG: would also like to express our gratitude to all, 0.991
+[2022/10/10 12:06:31] ppocr DEBUG: the producers for their invaluable opinions, 0.978
+[2022/10/10 12:06:31] ppocr DEBUG: and assistance throughout this project. And to, 0.988
+[2022/10/10 12:06:31] ppocr DEBUG: the many others whose names are not credited, 0.958
+[2022/10/10 12:06:31] ppocr DEBUG: but have made specific input in this book, we, 0.970
+[2022/10/10 12:06:31] ppocr DEBUG: thank you for your continuous support., 0.998
+[2022/10/10 12:06:31] ppocr DEBUG: The visualized image saved in ./inference_results/img_12.jpg
+[2022/10/10 12:06:31] ppocr INFO: The predict total time is 3.2482550144195557
+```
+
+Using Paddle Inference to predict, terminal output:
+
+```
+[2022/10/10 12:06:28] ppocr DEBUG: dt_boxes num : 11, elapse : 0.3568880558013916
+[2022/10/10 12:06:31] ppocr DEBUG: rec_res num : 11, elapse : 2.6445000171661377
+[2022/10/10 12:06:31] ppocr DEBUG: 0 Predict time of doc/imgs_en/img_12.jpg: 3.021s
+[2022/10/10 12:06:31] ppocr DEBUG: ACKNOWLEDGEMENTS, 0.997
+[2022/10/10 12:06:31] ppocr DEBUG: We would like to thank all the designers and, 0.976
+[2022/10/10 12:06:31] ppocr DEBUG: contributors who have been involved in the, 0.979
+[2022/10/10 12:06:31] ppocr DEBUG: production of this book; their contributions, 0.989
+[2022/10/10 12:06:31] ppocr DEBUG: have been indispensable to its creation. We, 0.956
+[2022/10/10 12:06:31] ppocr DEBUG: would also like to express our gratitude to all, 0.991
+[2022/10/10 12:06:31] ppocr DEBUG: the producers for their invaluable opinions, 0.978
+[2022/10/10 12:06:31] ppocr DEBUG: and assistance throughout this project. And to, 0.988
+[2022/10/10 12:06:31] ppocr DEBUG: the many others whose names are not credited, 0.958
+[2022/10/10 12:06:31] ppocr DEBUG: but have made specific input in this book, we, 0.970
+[2022/10/10 12:06:31] ppocr DEBUG: thank you for your continuous support., 0.998
+[2022/10/10 12:06:31] ppocr DEBUG: The visualized image saved in ./inference_results/img_12.jpg
+[2022/10/10 12:06:31] ppocr INFO: The predict total time is 3.2482550144195557
```
diff --git a/doc/doc_en/inference_ppocr_en.md b/doc/doc_en/inference_ppocr_en.md
index 4c9db51e1d23e5ac05cfcb3ec43748df75c0b36c..42bce8116d5a5edec854d08d0ddeaa43792137df 100755
--- a/doc/doc_en/inference_ppocr_en.md
+++ b/doc/doc_en/inference_ppocr_en.md
@@ -84,9 +84,9 @@ For English recognition model inference, you can execute the following commands,
```
# download en model:
-wget https://paddleocr.bj.bcebos.com/PP-OCRv3/english/en_PP-OCRv3_det_infer.tar
-tar xf en_PP-OCRv3_det_infer.tar
-python3 tools/infer/predict_rec.py --image_dir="./doc/imgs_words/en/word_1.png" --rec_model_dir="./en_PP-OCRv3_det_infer/" --rec_char_dict_path="ppocr/utils/en_dict.txt"
+wget https://paddleocr.bj.bcebos.com/PP-OCRv3/english/en_PP-OCRv3_rec_infer.tar
+tar xf en_PP-OCRv3_rec_infer.tar
+python3 tools/infer/predict_rec.py --image_dir="./doc/imgs_words/en/word_1.png" --rec_model_dir="./en_PP-OCRv3_rec_infer/" --rec_char_dict_path="ppocr/utils/en_dict.txt"
```

diff --git a/ppocr/data/imaug/random_crop_data.py b/ppocr/data/imaug/random_crop_data.py
index 64aa110de4e3df950ce21e6d657877081b0fdd13..d634ac6f33ca26f89e4917847ca4ed96f22e5a65 100644
--- a/ppocr/data/imaug/random_crop_data.py
+++ b/ppocr/data/imaug/random_crop_data.py
@@ -52,6 +52,7 @@ def split_regions(axis):
region = axis[min_axis:i]
min_axis = i
regions.append(region)
+ regions.append(axis[min_axis:]) # 添加的一行
return regions
diff --git a/ppstructure/table/README.md b/ppstructure/table/README.md
index 08635516ba8301e6f98f175e5eba8c0a97b1708e..1d082f3878c56e42d175d13c75e1fe17916e7781 100644
--- a/ppstructure/table/README.md
+++ b/ppstructure/table/README.md
@@ -114,7 +114,7 @@ python3 table/eval_table.py \
--det_model_dir=path/to/det_model_dir \
--rec_model_dir=path/to/rec_model_dir \
--table_model_dir=path/to/table_model_dir \
- --image_dir=../doc/table/1.png \
+ --image_dir=docs/table/table.jpg \
--rec_char_dict_path=../ppocr/utils/dict/table_dict.txt \
--table_char_dict_path=../ppocr/utils/dict/table_structure_dict.txt \
--det_limit_side_len=736 \
@@ -145,6 +145,7 @@ python3 table/eval_table.py \
--table_char_dict_path=../ppocr/utils/dict/table_structure_dict.txt \
--det_limit_side_len=736 \
--det_limit_type=min \
+ --rec_image_shape=3,32,320 \
--gt_path=path/to/gt.txt
```
diff --git a/ppstructure/table/README_ch.md b/ppstructure/table/README_ch.md
index 1ef126261d9ce832cd1919a1b3991f341add998c..feccb70adfe20fa8c1cd06f33a10ee6fa043e69e 100644
--- a/ppstructure/table/README_ch.md
+++ b/ppstructure/table/README_ch.md
@@ -118,7 +118,7 @@ python3 table/eval_table.py \
--det_model_dir=path/to/det_model_dir \
--rec_model_dir=path/to/rec_model_dir \
--table_model_dir=path/to/table_model_dir \
- --image_dir=../doc/table/1.png \
+ --image_dir=docs/table/table.jpg \
--rec_char_dict_path=../ppocr/utils/dict/table_dict.txt \
--table_char_dict_path=../ppocr/utils/dict/table_structure_dict.txt \
--det_limit_side_len=736 \
@@ -149,6 +149,7 @@ python3 table/eval_table.py \
--table_char_dict_path=../ppocr/utils/dict/table_structure_dict.txt \
--det_limit_side_len=736 \
--det_limit_type=min \
+ --rec_image_shape=3,32,320 \
--gt_path=path/to/gt.txt
```
diff --git a/tools/infer/predict_system.py b/tools/infer/predict_system.py
index e0f2c41fa2aba23491efee920afbd76db1ec84e0..f12fd626c804bacd2607381f6bbd6d2179e20032 100755
--- a/tools/infer/predict_system.py
+++ b/tools/infer/predict_system.py
@@ -116,7 +116,10 @@ def sorted_boxes(dt_boxes):
sorted boxes(array) with shape [4, 2]
"""
num_boxes = dt_boxes.shape[0]
- sorted_boxes = sorted(dt_boxes, key=lambda x: (x[0][1], x[0][0]))
+ if abs(num_boxes - 2) < 1e-4:
+ sorted_boxes = sorted(dt_boxes, key=lambda x: (x[1], x[0]))
+ else:
+ sorted_boxes = sorted(dt_boxes, key=lambda x: (x[0][1], x[0][0]))
_boxes = list(sorted_boxes)
for i in range(num_boxes - 1):
diff --git a/tools/infer/utility.py b/tools/infer/utility.py
index e6adad3dd8c2d57775ab5f7fa489dca98d22eb3d..a736c358e5e6a283bbb9d1dd5851afaf0c4e0c47 100644
--- a/tools/infer/utility.py
+++ b/tools/infer/utility.py
@@ -225,7 +225,8 @@ def create_predictor(args, mode, logger):
use_calib_mode=False)
# collect shape
- trt_shape_f = os.path.join(model_dir, f"{mode}_trt_dynamic_shape.txt")
+ trt_shape_f = os.path.join(model_dir,
+ f"{mode}_trt_dynamic_shape.txt")
if not os.path.exists(trt_shape_f):
config.collect_shape_range_info(trt_shape_f)