follow comment
Showing
recognize_digits/cnn_mnist.py
已删除
100644 → 0
147.3 KB

| W: | H:
| W: | H:


248.3 KB
文件已移动

| W: | H:
| W: | H:


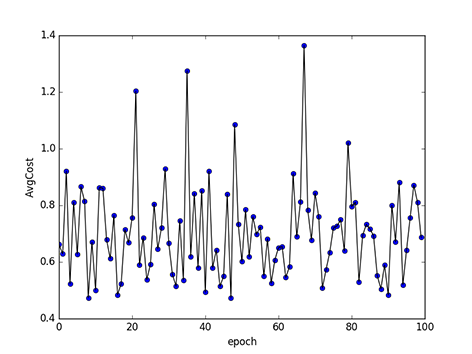
| W: | H:
| W: | H:
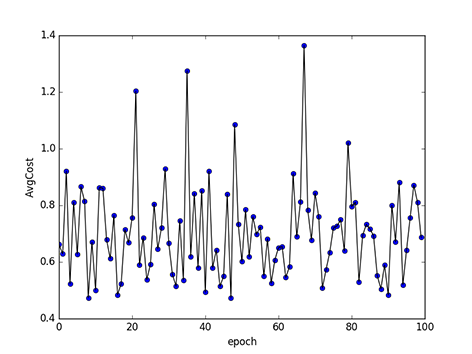
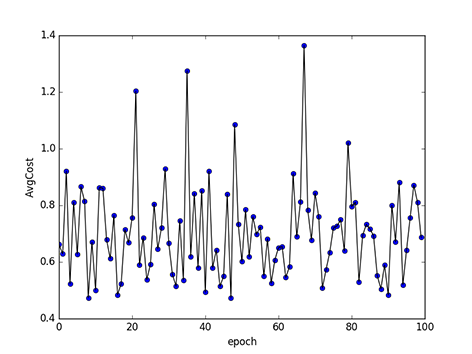
147.3 KB
49.4 KB | W: | H:
33.4 KB | W: | H:
248.3 KB
56.1 KB | W: | H:
46.5 KB | W: | H:
58.0 KB | W: | H:
41.7 KB | W: | H: