diff --git a/.gitignore b/.gitignore
index 1a2dd675e961f1804fa58e2e2e49118536b84ce9..9eecb4f1056fc040d4c9579d593bee2cc4013837 100644
--- a/.gitignore
+++ b/.gitignore
@@ -21,3 +21,7 @@ output/
*.log
.clang-format
.clang_format.hook
+
+build/
+dist/
+paddleocr.egg-info/
\ No newline at end of file
diff --git a/MANIFEST.in b/MANIFEST.in
new file mode 100644
index 0000000000000000000000000000000000000000..388882df0c3701780dd6371bc91887356a7bca40
--- /dev/null
+++ b/MANIFEST.in
@@ -0,0 +1,8 @@
+include LICENSE.txt
+include README.md
+
+recursive-include ppocr/utils *.txt utility.py character.py check.py
+recursive-include ppocr/data/det *.py
+recursive-include ppocr/postprocess *.py
+recursive-include ppocr/postprocess/lanms *.*
+recursive-include tools/infer *.py
diff --git a/README.md b/README.md
index b49d18eecc5abf56d5251a6d1aa5baa5be1b1898..867e3cbcbffe1a7981409d26ef58793acea28522 100644
--- a/README.md
+++ b/README.md
@@ -1,209 +1,139 @@
-[English](README_en.md) | 简体中文
-
-## 简介
-PaddleOCR旨在打造一套丰富、领先、且实用的OCR工具库,助力使用者训练出更好的模型,并应用落地。
-
-**近期更新**
-- 2020.7.15 添加基于EasyEdge和Paddle-Lite的移动端DEMO,支持iOS和Android系统
-- 2020.7.15 完善预测部署,添加基于C++预测引擎推理、服务化部署和端侧部署方案,以及超轻量级中文OCR模型预测耗时Benchmark
-- 2020.7.15 整理OCR相关数据集、常用数据标注以及合成工具
-- 2020.7.9 添加支持空格的识别模型,识别效果,预测及训练方式请参考快速开始和文本识别训练相关文档
-- 2020.7.9 添加数据增强、学习率衰减策略,具体参考[配置文件](./doc/doc_ch/config.md)
-- [more](./doc/doc_ch/update.md)
-
-## 特性
-- 超轻量级中文OCR模型,总模型仅8.6M
- - 单模型支持中英文数字组合识别、竖排文本识别、长文本识别
- - 检测模型DB(4.1M)+识别模型CRNN(4.5M)
-- 实用通用中文OCR模型
-- 多种预测推理部署方案,包括服务部署和端侧部署
-- 多种文本检测训练算法,EAST、DB
-- 多种文本识别训练算法,Rosetta、CRNN、STAR-Net、RARE
-- 可运行于Linux、Windows、MacOS等多种系统
-
-## 快速体验
+English | [简体中文](README_ch.md)
+
+## Introduction
+PaddleOCR aims to create rich, leading, and practical OCR tools that help users train better models and apply them into practice.
+
+**Recent updates**
+- 2020.9.22 Update the PP-OCR technical article, https://arxiv.org/abs/2009.09941
+- 2020.9.19 Update the ultra lightweight compressed ppocr_mobile_slim series models, the overall model size is 3.5M (see [PP-OCR Pipline](#PP-OCR-Pipline)), suitable for mobile deployment. [Model Downloads](#Supported-Chinese-model-list)
+- 2020.9.17 Update the ultra lightweight ppocr_mobile series and general ppocr_server series Chinese and English ocr models, which are comparable to commercial effects. [Model Downloads](#Supported-Chinese-model-list)
+- 2020.8.24 Support the use of PaddleOCR through whl package installation,pelease refer [PaddleOCR Package](./doc/doc_en/whl_en.md)
+- 2020.8.21 Update the replay and PPT of the live lesson at Bilibili on August 18, lesson 2, easy to learn and use OCR tool spree. [Get Address](https://aistudio.baidu.com/aistudio/education/group/info/1519)
+- [more](./doc/doc_en/update_en.md)
+
+## Features
+- PPOCR series of high-quality pre-trained models, comparable to commercial effects
+ - Ultra lightweight ppocr_mobile series models: detection (2.6M) + direction classifier (0.9M) + recognition (4.6M) = 8.1M
+ - General ppocr_server series models: detection (47.2M) + direction classifier (0.9M) + recognition (107M) = 155.1M
+ - Ultra lightweight compression ppocr_mobile_slim series models: detection (1.4M) + direction classifier (0.5M) + recognition (1.6M) = 3.5M
+- Support Chinese, English, and digit recognition, vertical text recognition, and long text recognition
+- Support multi-language recognition: Korean, Japanese, German, French
+- Support user-defined training, provides rich predictive inference deployment solutions
+- Support PIP installation, easy to use
+- Support Linux, Windows, MacOS and other systems
+
+## Visualization
-
+
+
-上图是超轻量级中文OCR模型效果展示,更多效果图请见[效果展示页面](./doc/doc_ch/visualization.md)。
+The above pictures are the visualizations of the general ppocr_server model. For more effect pictures, please see [More visualizations](./doc/doc_en/visualization_en.md).
-- 超轻量级中文OCR在线体验地址:https://www.paddlepaddle.org.cn/hub/scene/ocr
-- 移动端DEMO体验(基于EasyEdge和Paddle-Lite, 支持iOS和Android系统):[安装包二维码获取地址](https://ai.baidu.com/easyedge/app/openSource?from=paddlelite)
+## Quick Experience
- Android手机也可以扫描下面二维码安装体验。
+You can also quickly experience the ultra-lightweight OCR : [Online Experience](https://www.paddlepaddle.org.cn/hub/scene/ocr)
-
-
-### 2.通用中文OCR效果展示 [more](./doc/doc_ch/visualization.md)
+- [**OCR Quick Start**](./doc/doc_en/quickstart_en.md)
+
+
+
+## PP-OCR 1.1 series model list(Update on Sep 17)
+
+| Model introduction | Model name | Recommended scene | Detection model | Direction classifier | Recognition model |
+| ------------------------------------------------------------ | ---------------------------- | ----------------- | ------------------------------------------------------------ | ------------------------------------------------------------ | ------------------------------------------------------------ |
+| Chinese and English ultra-lightweight OCR model (8.1M) | ch_ppocr_mobile_v1.1_xx | Mobile & server | [inference model](https://paddleocr.bj.bcebos.com/20-09-22/mobile/det/ch_ppocr_mobile_v1.1_det_infer.tar) / [pre-trained model](https://paddleocr.bj.bcebos.com/20-09-22/mobile/det/ch_ppocr_mobile_v1.1_det_train.tar) | [inference model](https://paddleocr.bj.bcebos.com/20-09-22/cls/ch_ppocr_mobile_v1.1_cls_infer.tar) / [pre-trained model](https://paddleocr.bj.bcebos.com/20-09-22/cls/ch_ppocr_mobile_v1.1_cls_train.tar) | [inference model](https://paddleocr.bj.bcebos.com/20-09-22/mobile/rec/ch_ppocr_mobile_v1.1_rec_infer.tar) / [pre-trained model](https://paddleocr.bj.bcebos.com/20-09-22/mobile/rec/ch_ppocr_mobile_v1.1_rec_pre.tar) |
+| Chinese and English general OCR model (155.1M) | ch_ppocr_server_v1.1_xx | Server | [inference model](https://paddleocr.bj.bcebos.com/20-09-22/server/det/ch_ppocr_server_v1.1_det_infer.tar) / [pre-trained model](https://paddleocr.bj.bcebos.com/20-09-22/server/det/ch_ppocr_server_v1.1_det_train.tar) | [inference model](https://paddleocr.bj.bcebos.com/20-09-22/cls/ch_ppocr_mobile_v1.1_cls_infer.tar) / [pre-trained model](https://paddleocr.bj.bcebos.com/20-09-22/cls/ch_ppocr_mobile_v1.1_cls_train.tar) | [inference model](https://paddleocr.bj.bcebos.com/20-09-22/server/rec/ch_ppocr_server_v1.1_rec_infer.tar) / [pre-trained model](https://paddleocr.bj.bcebos.com/20-09-22/server/rec/ch_ppocr_server_v1.1_rec_pre.tar) |
+| Chinese and English ultra-lightweight compressed OCR model (3.5M) | ch_ppocr_mobile_slim_v1.1_xx | Mobile | [inference model](https://paddleocr.bj.bcebos.com/20-09-22/mobile-slim/det/ch_ppocr_mobile_v1.1_det_prune_infer.tar) / [slim model](https://paddleocr.bj.bcebos.com/20-09-22/mobile-slim/det/ch_ppocr_mobile_v1.1_det_prune_opt.nb) | [inference model](https://paddleocr.bj.bcebos.com/20-09-22/cls/ch_ppocr_mobile_v1.1_cls_quant_infer.tar) / [slim model](https://paddleocr.bj.bcebos.com/20-09-22/cls/ch_ppocr_mobile_cls_quant_opt.nb) | [inference model](https://paddleocr.bj.bcebos.com/20-09-22/mobile-slim/rec/ch_ppocr_mobile_v1.1_rec_quant_infer.tar) / [slim model](https://paddleocr.bj.bcebos.com/20-09-22/mobile-slim/rec/ch_ppocr_mobile_v1.1_rec_quant_opt.nb) |
+
+For more model downloads (including multiple languages), please refer to [PP-OCR v1.1 series model downloads](./doc/doc_en/models_list_en.md)
+
+
+## Tutorials
+- [Installation](./doc/doc_en/installation_en.md)
+- [Quick Start](./doc/doc_en/quickstart_en.md)
+- [Code Structure](./doc/doc_en/tree_en.md)
+- Algorithm introduction
+ - [Text Detection Algorithm](./doc/doc_en/algorithm_overview_en.md)
+ - [Text Recognition Algorithm](./doc/doc_en/algorithm_overview_en.md)
+ - [PP-OCR Pipline](#PP-OCR-Pipline)
+- Model training/evaluation
+ - [Text Detection](./doc/doc_en/detection_en.md)
+ - [Text Recognition](./doc/doc_en/recognition_en.md)
+ - [Direction Classification](./doc/doc_en/angle_class_en.md)
+ - [Yml Configuration](./doc/doc_en/config_en.md)
+- Inference and Deployment
+ - [Quick inference based on pip](./doc/doc_en/whl_en.md)
+ - [Python Inference](./doc/doc_en/inference_en.md)
+ - [C++ Inference](./deploy/cpp_infer/readme_en.md)
+ - [Serving](./deploy/hubserving/readme_en.md)
+ - [Mobile](./deploy/lite/readme_en.md)
+ - [Model Quantization](./deploy/slim/quantization/README_en.md)
+ - [Model Compression](./deploy/slim/prune/README_en.md)
+ - [Benchmark](./doc/doc_en/benchmark_en.md)
+- Datasets
+ - [General OCR Datasets(Chinese/English)](./doc/doc_en/datasets_en.md)
+ - [HandWritten_OCR_Datasets(Chinese)](./doc/doc_en/handwritten_datasets_en.md)
+ - [Various OCR Datasets(multilingual)](./doc/doc_en/vertical_and_multilingual_datasets_en.md)
+ - [Data Annotation Tools](./doc/doc_en/data_annotation_en.md)
+ - [Data Synthesis Tools](./doc/doc_en/data_synthesis_en.md)
+- [Visualization](#Visualization)
+- [FAQ](./doc/doc_en/FAQ_en.md)
+- [Community](#Community)
+- [References](./doc/doc_en/reference_en.md)
+- [License](#LICENSE)
+- [Contribution](#CONTRIBUTION)
+
+
+
+## PP-OCR Pipline
-
+
-
-### 3.支持空格的中文OCR效果展示 [more](./doc/doc_ch/visualization.md)
+PP-OCR is a practical ultra-lightweight OCR system. It is mainly composed of three parts: DB text detection, detection frame correction and CRNN text recognition. The system adopts 19 effective strategies from 8 aspects including backbone network selection and adjustment, prediction head design, data augmentation, learning rate transformation strategy, regularization parameter selection, pre-training model use, and automatic model tailoring and quantization to optimize and slim down the models of each module. The final results are an ultra-lightweight Chinese and English OCR model with an overall size of 3.5M and a 2.8M English digital OCR model. For more details, please refer to the PP-OCR technical article (https://arxiv.org/abs/2009.09941).
+
+## Visualization [more](./doc/doc_en/visualization_en.md)
-
+
+
+
+
+
+
-
-## FAQ
-1. **转换attention识别模型时报错:KeyError: 'predict'**
-问题已解,请更新到最新代码。
-
-2. **关于推理速度**
-图片中的文字较多时,预测时间会增,可以使用--rec_batch_num设置更小预测batch num,默认值为30,可以改为10或其他数值。
-
-3. **服务部署与移动端部署**
-预计6月中下旬会先后发布基于Serving的服务部署方案和基于Paddle Lite的移动端部署方案,欢迎持续关注。
-
-4. **自研算法发布时间**
-自研算法SAST、SRN、End2End-PSL都将在7-8月陆续发布,敬请期待。
-
-[more](./doc/doc_ch/FAQ.md)
-
-
-## 欢迎加入PaddleOCR技术交流群
-请扫描下面二维码,完成问卷填写,获取加群二维码和OCR方向的炼丹秘籍
+
+## Community
+Scan the QR code below with your Wechat and completing the questionnaire, you can access to offical technical exchange group.
-
+
-
-## 许可证书
-本项目的发布受Apache 2.0 license许可认证。
-
-
-## 贡献代码
-我们非常欢迎你为PaddleOCR贡献代码,也十分感谢你的反馈。
-
-- 非常感谢 [Khanh Tran](https://github.com/xxxpsyduck) 贡献了英文文档。
-- 非常感谢 [zhangxin](https://github.com/ZhangXinNan)([Blog](https://blog.csdn.net/sdlypyzq)) 贡献新的可视化方式、添加.gitgnore、处理手动设置PYTHONPATH环境变量的问题
-- 非常感谢 [lyl120117](https://github.com/lyl120117) 贡献打印网络结构的代码
-- 非常感谢 [xiangyubo](https://github.com/xiangyubo) 贡献手写中文OCR数据集
+
+## License
+This project is released under Apache 2.0 license
+
+
+## Contribution
+We welcome all the contributions to PaddleOCR and appreciate for your feedback very much.
+
+- Many thanks to [Khanh Tran](https://github.com/xxxpsyduck) and [Karl Horky](https://github.com/karlhorky) for contributing and revising the English documentation.
+- Many thanks to [zhangxin](https://github.com/ZhangXinNan) for contributing the new visualize function、add .gitgnore and discard set PYTHONPATH manually.
+- Many thanks to [lyl120117](https://github.com/lyl120117) for contributing the code for printing the network structure.
+- Thanks [xiangyubo](https://github.com/xiangyubo) for contributing the handwritten Chinese OCR datasets.
+- Thanks [authorfu](https://github.com/authorfu) for contributing Android demo and [xiadeye](https://github.com/xiadeye) contributing iOS demo, respectively.
+- Thanks [BeyondYourself](https://github.com/BeyondYourself) for contributing many great suggestions and simplifying part of the code style.
+- Thanks [tangmq](https://gitee.com/tangmq) for contributing Dockerized deployment services to PaddleOCR and supporting the rapid release of callable Restful API services.
diff --git a/README_ch.md b/README_ch.md
new file mode 100644
index 0000000000000000000000000000000000000000..58b1acb982d2b880a949a4592026f6923a6bd343
--- /dev/null
+++ b/README_ch.md
@@ -0,0 +1,141 @@
+[English](README.md) | 简体中文
+
+## 简介
+PaddleOCR旨在打造一套丰富、领先、且实用的OCR工具库,助力使用者训练出更好的模型,并应用落地。
+
+**近期更新**
+- 2020.9.22 更新PP-OCR技术文章,https://arxiv.org/abs/2009.09941
+- 2020.9.19 更新超轻量压缩ppocr_mobile_slim系列模型,整体模型3.5M(详见[PP-OCR Pipline](#PP-OCR)),适合在移动端部署使用。[模型下载](#模型下载)
+- 2020.9.17 更新超轻量ppocr_mobile系列和通用ppocr_server系列中英文ocr模型,媲美商业效果。[模型下载](#模型下载)
+- 2020.8.26 更新OCR相关的84个常见问题及解答,具体参考[FAQ](./doc/doc_ch/FAQ.md)
+- 2020.8.24 支持通过whl包安装使用PaddleOCR,具体参考[Paddleocr Package使用说明](./doc/doc_ch/whl.md)
+- 2020.8.21 更新8月18日B站直播课回放和PPT,课节2,易学易用的OCR工具大礼包,[获取地址](https://aistudio.baidu.com/aistudio/education/group/info/1519)
+- [More](./doc/doc_ch/update.md)
+
+
+## 特性
+
+- PPOCR系列高质量预训练模型,准确的识别效果
+ - 超轻量ppocr_mobile移动端系列:检测(2.6M)+方向分类器(0.9M)+ 识别(4.6M)= 8.1M
+ - 通用ppocr_server系列:检测(47.2M)+方向分类器(0.9M)+ 识别(107M)= 155.1M
+ - 超轻量压缩ppocr_mobile_slim系列:检测(1.4M)+方向分类器(0.5M)+ 识别(1.6M)= 3.5M
+- 支持中英文数字组合识别、竖排文本识别、长文本识别
+- 支持多语言识别:韩语、日语、德语、法语
+- 支持用户自定义训练,提供丰富的预测推理部署方案
+- 支持PIP快速安装使用
+- 可运行于Linux、Windows、MacOS等多种系统
+
+## 效果展示
+
+
+
+
+## 许可证书
+本项目的发布受Apache 2.0 license许可认证。
+
+
+## 贡献代码
+我们非常欢迎你为PaddleOCR贡献代码,也十分感谢你的反馈。
+
+- 非常感谢 [Khanh Tran](https://github.com/xxxpsyduck) 和 [Karl Horky](https://github.com/karlhorky) 贡献修改英文文档
+- 非常感谢 [zhangxin](https://github.com/ZhangXinNan)([Blog](https://blog.csdn.net/sdlypyzq)) 贡献新的可视化方式、添加.gitgnore、处理手动设置PYTHONPATH环境变量的问题
+- 非常感谢 [lyl120117](https://github.com/lyl120117) 贡献打印网络结构的代码
+- 非常感谢 [xiangyubo](https://github.com/xiangyubo) 贡献手写中文OCR数据集
+- 非常感谢 [authorfu](https://github.com/authorfu) 贡献Android和[xiadeye](https://github.com/xiadeye) 贡献IOS的demo代码
+- 非常感谢 [BeyondYourself](https://github.com/BeyondYourself) 给PaddleOCR提了很多非常棒的建议,并简化了PaddleOCR的部分代码风格。
+- 非常感谢 [tangmq](https://gitee.com/tangmq) 给PaddleOCR增加Docker化部署服务,支持快速发布可调用的Restful API服务。
diff --git a/README_en.md b/README_en.md
deleted file mode 100644
index 38bda392072087f08ecca69bb2d16493e9bd2ffd..0000000000000000000000000000000000000000
--- a/README_en.md
+++ /dev/null
@@ -1,302 +0,0 @@
-English | [简体中文](README.md)
-
-## INTRODUCTION
-PaddleOCR aims to create a rich, leading, and practical OCR tools that help users train better models and apply them into practice.
-
-**Recent updates**、
-- 2020.7.9 Add recognition model to support space, [recognition result](#space Chinese OCR results). For more information: [Recognition](./doc/doc_ch/recognition.md) and [quickstart](./doc/doc_ch/quickstart.md)
-- 2020.7.9 Add data auguments and learning rate decay strategies,please read [config](./doc/doc_en/config_en.md)
-- 2020.6.8 Add [dataset](./doc/doc_en/datasets_en.md) and keep updating
-- 2020.6.5 Support exporting `attention` model to `inference_model`
-- 2020.6.5 Support separate prediction and recognition, output result score
-- [more](./doc/doc_en/update_en.md)
-
-## FEATURES
-- Lightweight Chinese OCR model, total model size is only 8.6M
- - Single model supports Chinese and English numbers combination recognition, vertical text recognition, long text recognition
- - Detection model DB (4.1M) + recognition model CRNN (4.5M)
-- Various text detection algorithms: EAST, DB
-- Various text recognition algorithms: Rosetta, CRNN, STAR-Net, RARE
-
-
-### Supported Chinese models list:
-
-|Model Name|Description |Detection Model link|Recognition Model link| Support for space Recognition Model link|
-|-|-|-|-|-|
-|chinese_db_crnn_mobile|lightweight Chinese OCR model|[inference model](https://paddleocr.bj.bcebos.com/ch_models/ch_det_mv3_db_infer.tar) / [pre-trained model](https://paddleocr.bj.bcebos.com/ch_models/ch_det_mv3_db.tar)|[inference model](https://paddleocr.bj.bcebos.com/ch_models/ch_rec_mv3_crnn_infer.tar) / [pre-trained model](https://paddleocr.bj.bcebos.com/ch_models/ch_rec_mv3_crnn.tar)|[inference model](https://paddleocr.bj.bcebos.com/ch_models/ch_rec_mv3_crnn_enhance_infer.tar) / [pre-train model](https://paddleocr.bj.bcebos.com/ch_models/ch_rec_mv3_crnn_enhance.tar)
-|chinese_db_crnn_server|General Chinese OCR model|[inference model](https://paddleocr.bj.bcebos.com/ch_models/ch_det_r50_vd_db_infer.tar) / [pre-trained model](https://paddleocr.bj.bcebos.com/ch_models/ch_det_r50_vd_db.tar)|[inference model](https://paddleocr.bj.bcebos.com/ch_models/ch_rec_r34_vd_crnn_infer.tar) / [pre-trained model](https://paddleocr.bj.bcebos.com/ch_models/ch_rec_r34_vd_crnn.tar)|[inference model](https://paddleocr.bj.bcebos.com/ch_models/ch_rec_r34_vd_crnn_enhance_infer.tar) / [pre-train model](https://paddleocr.bj.bcebos.com/ch_models/ch_rec_r34_vd_crnn_enhance.tar)
-
-
-For testing our Chinese OCR online:https://www.paddlepaddle.org.cn/hub/scene/ocr
-
-**You can also quickly experience the lightweight Chinese OCR and General Chinese OCR models as follows:**
-
-## **LIGHTWEIGHT CHINESE OCR AND GENERAL CHINESE OCR INFERENCE**
-
-
-
-The picture above is the result of our lightweight Chinese OCR model. For more testing results, please see the end of the article [lightweight Chinese OCR results](#lightweight-Chinese-OCR-results) , [General Chinese OCR results](#General-Chinese-OCR-results) and [Support for space Recognition Model](#Space-Chinese-OCR-results).
-
-#### 1. ENVIRONMENT CONFIGURATION
-
-Please see [Quick installation](./doc/doc_en/installation_en.md)
-
-#### 2. DOWNLOAD INFERENCE MODELS
-
-#### (1) Download lightweight Chinese OCR models
-*If wget is not installed in the windows system, you can copy the link to the browser to download the model. After model downloaded, unzip it and place it in the corresponding directory*
-
-Copy the detection and recognition 'inference model' address in [Chinese model List](#Supported-Chinese-model-list), download and unpack:
-
-```
-mkdir inference && cd inference
-# Download the detection part of the Chinese OCR and decompress it
-wget {url/of/detection/inference_model} && tar xf {name/of/detection/inference_model/package}
-# Download the recognition part of the Chinese OCR and decompress it
-wget {url/of/recognition/inference_model} && tar xf {name/of/recognition/inference_model/package}
-cd ..
-```
-
-Take lightweight Chinese OCR model as an example:
-
-```
-mkdir inference && cd inference
-# Download the detection part of the lightweight Chinese OCR and decompress it
-wget https://paddleocr.bj.bcebos.com/ch_models/ch_det_mv3_db_infer.tar && tar xf ch_det_mv3_db_infer.tar
-# Download the recognition part of the lightweight Chinese OCR and decompress it
-wget https://paddleocr.bj.bcebos.com/ch_models/ch_rec_mv3_crnn_infer.tar && tar xf ch_rec_mv3_crnn_infer.tar
-# Download the space-recognized part of the lightweight Chinese OCR and decompress it
-wget https://paddleocr.bj.bcebos.com/ch_models/ch_rec_mv3_crnn_enhance_infer.tar && tar xf ch_rec_mv3_crnn_enhance_infer.tar
-
-cd ..
-```
-
-After the decompression is completed, the file structure should be as follows:
-
-```
-|-inference
- |-ch_rec_mv3_crnn
- |- model
- |- params
- |-ch_det_mv3_db
- |- model
- |- params
- ...
-```
-
-#### 3. SINGLE IMAGE AND BATCH PREDICTION
-
-The following code implements text detection and recognition inference tandemly. When performing prediction, you need to specify the path of a single image or image folder through the parameter `image_dir`, the parameter `det_model_dir` specifies the path to detection model, and the parameter `rec_model_dir` specifies the path to the recognition model. The visual prediction results are saved to the `./inference_results` folder by default.
-
-```bash
-
-# Prediction on a single image by specifying image path to image_dir
-python3 tools/infer/predict_system.py --image_dir="./doc/imgs/11.jpg" --det_model_dir="./inference/ch_det_mv3_db/" --rec_model_dir="./inference/ch_rec_mv3_crnn/"
-
-# Prediction on a batch of images by specifying image folder path to image_dir
-python3 tools/infer/predict_system.py --image_dir="./doc/imgs/" --det_model_dir="./inference/ch_det_mv3_db/" --rec_model_dir="./inference/ch_rec_mv3_crnn/"
-
-# If you want to use CPU for prediction, you need to set the use_gpu parameter to False
-python3 tools/infer/predict_system.py --image_dir="./doc/imgs/11.jpg" --det_model_dir="./inference/ch_det_mv3_db/" --rec_model_dir="./inference/ch_rec_mv3_crnn/" --use_gpu=False
-```
-
-To run inference of the Generic Chinese OCR model, follow these steps above to download the corresponding models and update the relevant parameters. Examples are as follows:
-```
-# Prediction on a single image by specifying image path to image_dir
-python3 tools/infer/predict_system.py --image_dir="./doc/imgs/11.jpg" --det_model_dir="./inference/ch_det_r50_vd_db/" --rec_model_dir="./inference/ch_rec_r34_vd_crnn/"
-```
-
-To run inference of the space-Generic Chinese OCR model, follow these steps above to download the corresponding models and update the relevant parameters. Examples are as follows:
-
-```
-# Prediction on a single image by specifying image path to image_dir
-python3 tools/infer/predict_system.py --image_dir="./doc/imgs_en/img_12.jpg" --det_model_dir="./inference/ch_det_r50_vd_db/" --rec_model_dir="./inference/ch_rec_r34_vd_crnn_enhance/"
-```
-
-For more text detection and recognition models, please refer to the document [Inference](./doc/doc_en/inference_en.md)
-
-## DOCUMENTATION
-- [Quick installation](./doc/doc_en/installation_en.md)
-- [Text detection model training/evaluation/prediction](./doc/doc_en/detection_en.md)
-- [Text recognition model training/evaluation/prediction](./doc/doc_en/recognition_en.md)
-- [Inference](./doc/doc_en/inference_en.md)
-- [Introduction of yml file](./doc/doc_en/config_en.md)
-- [Dataset](./doc/doc_en/datasets_en.md)
-- [FAQ]((#FAQ)
-
-## TEXT DETECTION ALGORITHM
-
-PaddleOCR open source text detection algorithms list:
-- [x] EAST([paper](https://arxiv.org/abs/1704.03155))
-- [x] DB([paper](https://arxiv.org/abs/1911.08947))
-- [ ] SAST([paper](https://arxiv.org/abs/1908.05498))(Baidu Self-Research, comming soon)
-
-On the ICDAR2015 dataset, the text detection result is as follows:
-
-|Model|Backbone|precision|recall|Hmean|Download link|
-|-|-|-|-|-|-|
-|EAST|ResNet50_vd|88.18%|85.51%|86.82%|[Download link](https://paddleocr.bj.bcebos.com/det_r50_vd_east.tar)|
-|EAST|MobileNetV3|81.67%|79.83%|80.74%|[Download link](https://paddleocr.bj.bcebos.com/det_mv3_east.tar)|
-|DB|ResNet50_vd|83.79%|80.65%|82.19%|[Download link](https://paddleocr.bj.bcebos.com/det_r50_vd_db.tar)|
-|DB|MobileNetV3|75.92%|73.18%|74.53%|[Download link](https://paddleocr.bj.bcebos.com/det_mv3_db.tar)|
-
-For use of [LSVT](https://github.com/PaddlePaddle/PaddleOCR/blob/develop/doc/doc_en/datasets_en.md#1-icdar2019-lsvt) street view dataset with a total of 3w training data,the related configuration and pre-trained models for Chinese detection task are as follows:
-|Model|Backbone|Configuration file|Pre-trained model|
-|-|-|-|-|
-|lightweight Chinese model|MobileNetV3|det_mv3_db.yml|[Download link](https://paddleocr.bj.bcebos.com/ch_models/ch_det_mv3_db.tar)|
-|General Chinese OCR model|ResNet50_vd|det_r50_vd_db.yml|[Download link](https://paddleocr.bj.bcebos.com/ch_models/ch_det_r50_vd_db.tar)|
-
-* Note: For the training and evaluation of the above DB model, post-processing parameters box_thresh=0.6 and unclip_ratio=1.5 need to be set. If using different datasets and different models for training, these two parameters can be adjusted for better result.
-
-For the training guide and use of PaddleOCR text detection algorithms, please refer to the document [Text detection model training/evaluation/prediction](./doc/doc_en/detection_en.md)
-
-## TEXT RECOGNITION ALGORITHM
-
-PaddleOCR open-source text recognition algorithms list:
-- [x] CRNN([paper](https://arxiv.org/abs/1507.05717))
-- [x] Rosetta([paper](https://arxiv.org/abs/1910.05085))
-- [x] STAR-Net([paper](http://www.bmva.org/bmvc/2016/papers/paper043/index.html))
-- [x] RARE([paper](https://arxiv.org/abs/1603.03915v1))
-- [ ] SRN([paper](https://arxiv.org/abs/2003.12294))(Baidu Self-Research, comming soon)
-
-Refer to [DTRB](https://arxiv.org/abs/1904.01906), the training and evaluation result of these above text recognition (using MJSynth and SynthText for training, evaluate on IIIT, SVT, IC03, IC13, IC15, SVTP, CUTE) is as follow:
-
-|Model|Backbone|Avg Accuracy|Module combination|Download link|
-|-|-|-|-|-|
-|Rosetta|Resnet34_vd|80.24%|rec_r34_vd_none_none_ctc|[Download link](https://paddleocr.bj.bcebos.com/rec_r34_vd_none_none_ctc.tar)|
-|Rosetta|MobileNetV3|78.16%|rec_mv3_none_none_ctc|[Download link](https://paddleocr.bj.bcebos.com/rec_mv3_none_none_ctc.tar)|
-|CRNN|Resnet34_vd|82.20%|rec_r34_vd_none_bilstm_ctc|[Download link](https://paddleocr.bj.bcebos.com/rec_r34_vd_none_bilstm_ctc.tar)|
-|CRNN|MobileNetV3|79.37%|rec_mv3_none_bilstm_ctc|[Download link](https://paddleocr.bj.bcebos.com/rec_mv3_none_bilstm_ctc.tar)|
-|STAR-Net|Resnet34_vd|83.93%|rec_r34_vd_tps_bilstm_ctc|[Download link](https://paddleocr.bj.bcebos.com/rec_r34_vd_tps_bilstm_ctc.tar)|
-|STAR-Net|MobileNetV3|81.56%|rec_mv3_tps_bilstm_ctc|[Download link](https://paddleocr.bj.bcebos.com/rec_mv3_tps_bilstm_ctc.tar)|
-|RARE|Resnet34_vd|84.90%|rec_r34_vd_tps_bilstm_attn|[Download link](https://paddleocr.bj.bcebos.com/rec_r34_vd_tps_bilstm_attn.tar)|
-|RARE|MobileNetV3|83.32%|rec_mv3_tps_bilstm_attn|[Download link](https://paddleocr.bj.bcebos.com/rec_mv3_tps_bilstm_attn.tar)|
-
-We use [LSVT](https://github.com/PaddlePaddle/PaddleOCR/blob/develop/doc/doc_en/datasets_en.md#1-icdar2019-lsvt) dataset and cropout 30w traning data from original photos by using position groundtruth and make some calibration needed. In addition, based on the LSVT corpus, 500w synthetic data is generated to train the Chinese model. The related configuration and pre-trained models are as follows:
-|Model|Backbone|Configuration file|Pre-trained model|
-|-|-|-|-|
-|lightweight Chinese model|MobileNetV3|rec_chinese_lite_train.yml|[Download link](https://paddleocr.bj.bcebos.com/ch_models/ch_rec_mv3_crnn.tar)|[inference model](https://paddleocr.bj.bcebos.com/ch_models/ch_rec_mv3_crnn_enhance_infer.tar) & [pre-trained model](https://paddleocr.bj.bcebos.com/ch_models/ch_rec_mv3_crnn_enhance.tar)|
-|General Chinese OCR model|Resnet34_vd|rec_chinese_common_train.yml|[Download link](https://paddleocr.bj.bcebos.com/ch_models/ch_rec_r34_vd_crnn.tar)|[inference model](https://paddleocr.bj.bcebos.com/ch_models/ch_rec_r34_vd_crnn_enhance_infer.tar) & [pre-trained model](https://paddleocr.bj.bcebos.com/ch_models/ch_rec_r34_vd_crnn_enhance.tar)|
-
-Please refer to the document for training guide and use of PaddleOCR text recognition algorithms [Text recognition model training/evaluation/prediction](./doc/doc_en/recognition_en.md)
-
-## END-TO-END OCR ALGORITHM
-- [ ] [End2End-PSL](https://arxiv.org/abs/1909.07808)(Baidu Self-Research, comming soon)
-
-
-## LIGHTWEIGHT CHINESE OCR RESULTS
-
-
-
-
-
-
-
-
-
-
-## General Chinese OCR results
-
-
-
-
-
-
-## space Chinese OCR results
-
-### LIGHTWEIGHT CHINESE OCR RESULTS
-
-
-
-### General Chinese OCR results
-
-
-
-## FAQ
-1. Error when using attention-based recognition model: KeyError: 'predict'
-
- The inference of recognition model based on attention loss is still being debugged. For Chinese text recognition, it is recommended to choose the recognition model based on CTC loss first. In practice, it is also found that the recognition model based on attention loss is not as effective as the one based on CTC loss.
-
-2. About inference speed
-
- When there are a lot of texts in the picture, the prediction time will increase. You can use `--rec_batch_num` to set a smaller prediction batch size. The default value is 30, which can be changed to 10 or other values.
-
-3. Service deployment and mobile deployment
-
- It is expected that the service deployment based on Serving and the mobile deployment based on Paddle Lite will be released successively in mid-to-late June. Stay tuned for more updates.
-
-4. Release time of self-developed algorithm
-
- Baidu Self-developed algorithms such as SAST, SRN and end2end PSL will be released in June or July. Please be patient.
-
-[more](./doc/doc_en/FAQ_en.md)
-
-## WELCOME TO THE PaddleOCR TECHNICAL EXCHANGE GROUP
-WeChat: paddlehelp, note OCR, our assistant will get you into the group~
-
-
-
-## REFERENCES
-```
-1. EAST:
-@inproceedings{zhou2017east,
- title={EAST: an efficient and accurate scene text detector},
- author={Zhou, Xinyu and Yao, Cong and Wen, He and Wang, Yuzhi and Zhou, Shuchang and He, Weiran and Liang, Jiajun},
- booktitle={Proceedings of the IEEE conference on Computer Vision and Pattern Recognition},
- pages={5551--5560},
- year={2017}
-}
-
-2. DB:
-@article{liao2019real,
- title={Real-time Scene Text Detection with Differentiable Binarization},
- author={Liao, Minghui and Wan, Zhaoyi and Yao, Cong and Chen, Kai and Bai, Xiang},
- journal={arXiv preprint arXiv:1911.08947},
- year={2019}
-}
-
-3. DTRB:
-@inproceedings{baek2019wrong,
- title={What is wrong with scene text recognition model comparisons? dataset and model analysis},
- author={Baek, Jeonghun and Kim, Geewook and Lee, Junyeop and Park, Sungrae and Han, Dongyoon and Yun, Sangdoo and Oh, Seong Joon and Lee, Hwalsuk},
- booktitle={Proceedings of the IEEE International Conference on Computer Vision},
- pages={4715--4723},
- year={2019}
-}
-
-4. SAST:
-@inproceedings{wang2019single,
- title={A Single-Shot Arbitrarily-Shaped Text Detector based on Context Attended Multi-Task Learning},
- author={Wang, Pengfei and Zhang, Chengquan and Qi, Fei and Huang, Zuming and En, Mengyi and Han, Junyu and Liu, Jingtuo and Ding, Errui and Shi, Guangming},
- booktitle={Proceedings of the 27th ACM International Conference on Multimedia},
- pages={1277--1285},
- year={2019}
-}
-
-5. SRN:
-@article{yu2020towards,
- title={Towards Accurate Scene Text Recognition with Semantic Reasoning Networks},
- author={Yu, Deli and Li, Xuan and Zhang, Chengquan and Han, Junyu and Liu, Jingtuo and Ding, Errui},
- journal={arXiv preprint arXiv:2003.12294},
- year={2020}
-}
-
-6. end2end-psl:
-@inproceedings{sun2019chinese,
- title={Chinese Street View Text: Large-scale Chinese Text Reading with Partially Supervised Learning},
- author={Sun, Yipeng and Liu, Jiaming and Liu, Wei and Han, Junyu and Ding, Errui and Liu, Jingtuo},
- booktitle={Proceedings of the IEEE International Conference on Computer Vision},
- pages={9086--9095},
- year={2019}
-}
-```
-
-## LICENSE
-This project is released under Apache 2.0 license
-
-## CONTRIBUTION
-We welcome all the contributions to PaddleOCR and appreciate for your feedback very much.
-
-- Many thanks to [Khanh Tran](https://github.com/xxxpsyduck) for contributing the English documentation.
-- Many thanks to [zhangxin](https://github.com/ZhangXinNan) for contributing the new visualize function、add .gitgnore and discard set PYTHONPATH manually.
-- Many thanks to [lyl120117](https://github.com/lyl120117) for contributing the code for printing the network structure.
diff --git a/__init__.py b/__init__.py
new file mode 100644
index 0000000000000000000000000000000000000000..7d94f66be072067172d56da13d8bb27d9aeac431
--- /dev/null
+++ b/__init__.py
@@ -0,0 +1,17 @@
+# Copyright (c) 2020 PaddlePaddle Authors. All Rights Reserved.
+#
+# Licensed under the Apache License, Version 2.0 (the "License");
+# you may not use this file except in compliance with the License.
+# You may obtain a copy of the License at
+#
+# http://www.apache.org/licenses/LICENSE-2.0
+#
+# Unless required by applicable law or agreed to in writing, software
+# distributed under the License is distributed on an "AS IS" BASIS,
+# WITHOUT WARRANTIES OR CONDITIONS OF ANY KIND, either express or implied.
+# See the License for the specific language governing permissions and
+# limitations under the License.
+
+__all__ = ['PaddleOCR', 'draw_ocr']
+from .paddleocr import PaddleOCR
+from .tools.infer.utility import draw_ocr
diff --git a/configs/cls/cls_mv3.yml b/configs/cls/cls_mv3.yml
new file mode 100755
index 0000000000000000000000000000000000000000..57afab507c03c2a32f1665f908170de05d91143a
--- /dev/null
+++ b/configs/cls/cls_mv3.yml
@@ -0,0 +1,44 @@
+Global:
+ algorithm: CLS
+ use_gpu: False
+ epoch_num: 100
+ log_smooth_window: 20
+ print_batch_step: 100
+ save_model_dir: output/cls_mv3
+ save_epoch_step: 3
+ eval_batch_step: 500
+ train_batch_size_per_card: 512
+ test_batch_size_per_card: 512
+ image_shape: [3, 48, 192]
+ label_list: ['0','180']
+ distort: True
+ reader_yml: ./configs/cls/cls_reader.yml
+ pretrain_weights:
+ checkpoints:
+ save_inference_dir:
+ infer_img:
+
+Architecture:
+ function: ppocr.modeling.architectures.cls_model,ClsModel
+
+Backbone:
+ function: ppocr.modeling.backbones.rec_mobilenet_v3,MobileNetV3
+ scale: 0.35
+ model_name: small
+
+Head:
+ function: ppocr.modeling.heads.cls_head,ClsHead
+ class_dim: 2
+
+Loss:
+ function: ppocr.modeling.losses.cls_loss,ClsLoss
+
+Optimizer:
+ function: ppocr.optimizer,AdamDecay
+ base_lr: 0.001
+ beta1: 0.9
+ beta2: 0.999
+ decay:
+ function: cosine_decay
+ step_each_epoch: 1169
+ total_epoch: 100
\ No newline at end of file
diff --git a/configs/cls/cls_reader.yml b/configs/cls/cls_reader.yml
new file mode 100755
index 0000000000000000000000000000000000000000..2b1d4c4e75217998f2c489bcd3bfbbb8b8b7f415
--- /dev/null
+++ b/configs/cls/cls_reader.yml
@@ -0,0 +1,13 @@
+TrainReader:
+ reader_function: ppocr.data.cls.dataset_traversal,SimpleReader
+ num_workers: 8
+ img_set_dir: ./train_data/cls
+ label_file_path: ./train_data/cls/train.txt
+
+EvalReader:
+ reader_function: ppocr.data.cls.dataset_traversal,SimpleReader
+ img_set_dir: ./train_data/cls
+ label_file_path: ./train_data/cls/test.txt
+
+TestReader:
+ reader_function: ppocr.data.cls.dataset_traversal,SimpleReader
diff --git a/configs/det/det_mv3_db.yml b/configs/det/det_mv3_db.yml
index caa7bd4fa09752cff8b4d596e80b5729cce175bf..91a8e86f8bba440df83c1d9f7da0e6523d5907bb 100755
--- a/configs/det/det_mv3_db.yml
+++ b/configs/det/det_mv3_db.yml
@@ -49,6 +49,6 @@ Optimizer:
PostProcess:
function: ppocr.postprocess.db_postprocess,DBPostProcess
thresh: 0.3
- box_thresh: 0.7
+ box_thresh: 0.6
max_candidates: 1000
- unclip_ratio: 2.0
+ unclip_ratio: 1.5
diff --git a/configs/det/det_mv3_db_v1.1.yml b/configs/det/det_mv3_db_v1.1.yml
new file mode 100755
index 0000000000000000000000000000000000000000..afc11aa01dc329d095abac6d61a48cf604ee2aa2
--- /dev/null
+++ b/configs/det/det_mv3_db_v1.1.yml
@@ -0,0 +1,59 @@
+Global:
+ algorithm: DB
+ use_gpu: true
+ epoch_num: 1200
+ log_smooth_window: 20
+ print_batch_step: 2
+ save_model_dir: ./output/det_db/
+ save_epoch_step: 200
+ # evaluation is run every 5000 iterations after the 4000th iteration
+ eval_batch_step: [4000, 5000]
+ train_batch_size_per_card: 16
+ test_batch_size_per_card: 16
+ image_shape: [3, 640, 640]
+ reader_yml: ./configs/det/det_db_icdar15_reader.yml
+ pretrain_weights: ./pretrain_models/MobileNetV3_large_x0_5_pretrained/
+ checkpoints:
+ save_res_path: ./output/det_db/predicts_db.txt
+ save_inference_dir:
+
+Architecture:
+ function: ppocr.modeling.architectures.det_model,DetModel
+
+Backbone:
+ function: ppocr.modeling.backbones.det_mobilenet_v3,MobileNetV3
+ scale: 0.5
+ model_name: large
+ disable_se: true
+
+Head:
+ function: ppocr.modeling.heads.det_db_head,DBHead
+ model_name: large
+ k: 50
+ inner_channels: 96
+ out_channels: 2
+
+Loss:
+ function: ppocr.modeling.losses.det_db_loss,DBLoss
+ balance_loss: true
+ main_loss_type: DiceLoss
+ alpha: 5
+ beta: 10
+ ohem_ratio: 3
+
+Optimizer:
+ function: ppocr.optimizer,AdamDecay
+ base_lr: 0.001
+ beta1: 0.9
+ beta2: 0.999
+ decay:
+ function: cosine_decay_warmup
+ step_each_epoch: 16
+ total_epoch: 1200
+
+PostProcess:
+ function: ppocr.postprocess.db_postprocess,DBPostProcess
+ thresh: 0.3
+ box_thresh: 0.6
+ max_candidates: 1000
+ unclip_ratio: 1.5
diff --git a/configs/det/det_r18_vd_db_v1.1.yml b/configs/det/det_r18_vd_db_v1.1.yml
new file mode 100755
index 0000000000000000000000000000000000000000..aa6dc0ee01c7e218ac6b3815c7ebacf886507e14
--- /dev/null
+++ b/configs/det/det_r18_vd_db_v1.1.yml
@@ -0,0 +1,57 @@
+Global:
+ algorithm: DB
+ use_gpu: true
+ epoch_num: 1200
+ log_smooth_window: 20
+ print_batch_step: 2
+ save_model_dir: ./output/det_r_18_vd_db/
+ save_epoch_step: 200
+ eval_batch_step: [3000, 2000]
+ train_batch_size_per_card: 8
+ test_batch_size_per_card: 1
+ image_shape: [3, 640, 640]
+ reader_yml: ./configs/det/det_db_icdar15_reader.yml
+ pretrain_weights: ./pretrain_models/ResNet18_vd_pretrained/
+ save_res_path: ./output/det_r18_vd_db/predicts_db.txt
+ checkpoints:
+ save_inference_dir:
+
+Architecture:
+ function: ppocr.modeling.architectures.det_model,DetModel
+
+Backbone:
+ function: ppocr.modeling.backbones.det_resnet_vd,ResNet
+ layers: 18
+
+Head:
+ function: ppocr.modeling.heads.det_db_head,DBHead
+ model_name: large
+ k: 50
+ inner_channels: 256
+ out_channels: 2
+
+Loss:
+ function: ppocr.modeling.losses.det_db_loss,DBLoss
+ balance_loss: true
+ main_loss_type: DiceLoss
+ alpha: 5
+ beta: 10
+ ohem_ratio: 3
+
+Optimizer:
+ function: ppocr.optimizer,AdamDecay
+ base_lr: 0.001
+ beta1: 0.9
+ beta2: 0.999
+ decay:
+ function: cosine_decay_warmup
+ step_each_epoch: 32
+ total_epoch: 1200
+
+PostProcess:
+ function: ppocr.postprocess.db_postprocess,DBPostProcess
+ thresh: 0.3
+ box_thresh: 0.6
+ max_candidates: 1000
+ unclip_ratio: 1.5
+
diff --git a/configs/det/det_r50_vd_sast_icdar15.yml b/configs/det/det_r50_vd_sast_icdar15.yml
new file mode 100644
index 0000000000000000000000000000000000000000..f1ecd61dc8ccb14fde98c2fc55cb2c9e630b5c44
--- /dev/null
+++ b/configs/det/det_r50_vd_sast_icdar15.yml
@@ -0,0 +1,50 @@
+Global:
+ algorithm: SAST
+ use_gpu: true
+ epoch_num: 2000
+ log_smooth_window: 20
+ print_batch_step: 2
+ save_model_dir: ./output/det_sast/
+ save_epoch_step: 20
+ eval_batch_step: 5000
+ train_batch_size_per_card: 8
+ test_batch_size_per_card: 8
+ image_shape: [3, 512, 512]
+ reader_yml: ./configs/det/det_sast_icdar15_reader.yml
+ pretrain_weights: ./pretrain_models/ResNet50_vd_ssld_pretrained/
+ save_res_path: ./output/det_sast/predicts_sast.txt
+ checkpoints:
+ save_inference_dir:
+
+Architecture:
+ function: ppocr.modeling.architectures.det_model,DetModel
+
+Backbone:
+ function: ppocr.modeling.backbones.det_resnet_vd_sast,ResNet
+ layers: 50
+
+Head:
+ function: ppocr.modeling.heads.det_sast_head,SASTHead
+ model_name: large
+ only_fpn_up: False
+# with_cab: False
+ with_cab: True
+
+Loss:
+ function: ppocr.modeling.losses.det_sast_loss,SASTLoss
+
+Optimizer:
+ function: ppocr.optimizer,RMSProp
+ base_lr: 0.001
+ decay:
+ function: piecewise_decay
+ boundaries: [30000, 50000, 80000, 100000, 150000]
+ decay_rate: 0.3
+
+PostProcess:
+ function: ppocr.postprocess.sast_postprocess,SASTPostProcess
+ score_thresh: 0.5
+ sample_pts_num: 2
+ nms_thresh: 0.2
+ expand_scale: 1.0
+ shrink_ratio_of_width: 0.3
\ No newline at end of file
diff --git a/configs/det/det_r50_vd_sast_totaltext.yml b/configs/det/det_r50_vd_sast_totaltext.yml
new file mode 100644
index 0000000000000000000000000000000000000000..ec42ce6d4bafd0c5d4360a255f35d07e83f90787
--- /dev/null
+++ b/configs/det/det_r50_vd_sast_totaltext.yml
@@ -0,0 +1,50 @@
+Global:
+ algorithm: SAST
+ use_gpu: true
+ epoch_num: 2000
+ log_smooth_window: 20
+ print_batch_step: 2
+ save_model_dir: ./output/det_sast/
+ save_epoch_step: 20
+ eval_batch_step: 5000
+ train_batch_size_per_card: 8
+ test_batch_size_per_card: 1
+ image_shape: [3, 512, 512]
+ reader_yml: ./configs/det/det_sast_totaltext_reader.yml
+ pretrain_weights: ./pretrain_models/ResNet50_vd_ssld_pretrained/
+ save_res_path: ./output/det_sast/predicts_sast.txt
+ checkpoints:
+ save_inference_dir:
+
+Architecture:
+ function: ppocr.modeling.architectures.det_model,DetModel
+
+Backbone:
+ function: ppocr.modeling.backbones.det_resnet_vd_sast,ResNet
+ layers: 50
+
+Head:
+ function: ppocr.modeling.heads.det_sast_head,SASTHead
+ model_name: large
+ only_fpn_up: False
+ # with_cab: False
+ with_cab: True
+
+Loss:
+ function: ppocr.modeling.losses.det_sast_loss,SASTLoss
+
+Optimizer:
+ function: ppocr.optimizer,RMSProp
+ base_lr: 0.001
+ decay:
+ function: piecewise_decay
+ boundaries: [30000, 50000, 80000, 100000, 150000]
+ decay_rate: 0.3
+
+PostProcess:
+ function: ppocr.postprocess.sast_postprocess,SASTPostProcess
+ score_thresh: 0.5
+ sample_pts_num: 6
+ nms_thresh: 0.2
+ expand_scale: 1.2
+ shrink_ratio_of_width: 0.2
\ No newline at end of file
diff --git a/configs/det/det_sast_icdar15_reader.yml b/configs/det/det_sast_icdar15_reader.yml
new file mode 100644
index 0000000000000000000000000000000000000000..ee45a85da7452e2069b0d7467b1ccfc44dd656b7
--- /dev/null
+++ b/configs/det/det_sast_icdar15_reader.yml
@@ -0,0 +1,24 @@
+TrainReader:
+ reader_function: ppocr.data.det.dataset_traversal,TrainReader
+ process_function: ppocr.data.det.sast_process,SASTProcessTrain
+ num_workers: 8
+ img_set_dir: ./train_data/
+ label_file_path: [./train_data/icdar2013/train_label_json.txt, ./train_data/icdar2015/train_label_json.txt, ./train_data/icdar17_mlt_latin/train_label_json.txt, ./train_data/coco_text_icdar_4pts/train_label_json.txt]
+ data_ratio_list: [0.1, 0.45, 0.3, 0.15]
+ min_crop_side_ratio: 0.3
+ min_crop_size: 24
+ min_text_size: 4
+ max_text_size: 512
+
+EvalReader:
+ reader_function: ppocr.data.det.dataset_traversal,EvalTestReader
+ process_function: ppocr.data.det.sast_process,SASTProcessTest
+ img_set_dir: ./train_data/icdar2015/text_localization/
+ label_file_path: ./train_data/icdar2015/text_localization/test_icdar2015_label.txt
+ max_side_len: 1536
+
+TestReader:
+ reader_function: ppocr.data.det.dataset_traversal,EvalTestReader
+ process_function: ppocr.data.det.sast_process,SASTProcessTest
+ infer_img: ./train_data/icdar2015/text_localization/ch4_test_images/img_11.jpg
+ max_side_len: 1536
diff --git a/configs/det/det_sast_totaltext_reader.yml b/configs/det/det_sast_totaltext_reader.yml
new file mode 100644
index 0000000000000000000000000000000000000000..92503d9f0e2b57f0d22b15591c5400185daf2afa
--- /dev/null
+++ b/configs/det/det_sast_totaltext_reader.yml
@@ -0,0 +1,24 @@
+TrainReader:
+ reader_function: ppocr.data.det.dataset_traversal,TrainReader
+ process_function: ppocr.data.det.sast_process,SASTProcessTrain
+ num_workers: 8
+ img_set_dir: ./train_data/
+ label_file_path: [./train_data/art_latin_icdar_14pt/train_no_tt_test/train_label_json.txt, ./train_data/total_text_icdar_14pt/train_label_json.txt]
+ data_ratio_list: [0.5, 0.5]
+ min_crop_side_ratio: 0.3
+ min_crop_size: 24
+ min_text_size: 4
+ max_text_size: 512
+
+EvalReader:
+ reader_function: ppocr.data.det.dataset_traversal,EvalTestReader
+ process_function: ppocr.data.det.sast_process,SASTProcessTest
+ img_set_dir: ./train_data/
+ label_file_path: ./train_data/total_text_icdar_14pt/test_label_json.txt
+ max_side_len: 768
+
+TestReader:
+ reader_function: ppocr.data.det.dataset_traversal,EvalTestReader
+ process_function: ppocr.data.det.sast_process,SASTProcessTest
+ infer_img: ./train_data/afs/total_text/Images/Test/img623.jpg
+ max_side_len: 768
diff --git a/configs/rec/ch_ppocr_v1.1/rec_chinese_common_train_v1.1.yml b/configs/rec/ch_ppocr_v1.1/rec_chinese_common_train_v1.1.yml
new file mode 100644
index 0000000000000000000000000000000000000000..8a84c635d32cce44daf405a8d48bcb0547b13acd
--- /dev/null
+++ b/configs/rec/ch_ppocr_v1.1/rec_chinese_common_train_v1.1.yml
@@ -0,0 +1,52 @@
+Global:
+ algorithm: CRNN
+ use_gpu: true
+ epoch_num: 500
+ log_smooth_window: 20
+ print_batch_step: 10
+ save_model_dir: ./output/rec_CRNN
+ save_epoch_step: 3
+ eval_batch_step: 2000
+ train_batch_size_per_card: 128
+ test_batch_size_per_card: 128
+ image_shape: [3, 32, 320]
+ max_text_length: 25
+ character_type: ch
+ character_dict_path: ./ppocr/utils/ppocr_keys_v1.txt
+ loss_type: ctc
+ distort: true
+ use_space_char: true
+ reader_yml: ./configs/rec/rec_chinese_reader.yml
+ pretrain_weights:
+ checkpoints:
+ save_inference_dir:
+ infer_img:
+
+Architecture:
+ function: ppocr.modeling.architectures.rec_model,RecModel
+
+Backbone:
+ function: ppocr.modeling.backbones.rec_resnet_vd,ResNet
+ layers: 34
+
+Head:
+ function: ppocr.modeling.heads.rec_ctc_head,CTCPredict
+ encoder_type: rnn
+ fc_decay: 0.00004
+ SeqRNN:
+ hidden_size: 256
+
+Loss:
+ function: ppocr.modeling.losses.rec_ctc_loss,CTCLoss
+
+Optimizer:
+ function: ppocr.optimizer,AdamDecay
+ base_lr: 0.0005
+ l2_decay: 0.00004
+ beta1: 0.9
+ beta2: 0.999
+ decay:
+ function: cosine_decay_warmup
+ step_each_epoch: 254
+ total_epoch: 500
+ warmup_minibatch: 1000
diff --git a/configs/rec/ch_ppocr_v1.1/rec_chinese_lite_train_v1.1.yml b/configs/rec/ch_ppocr_v1.1/rec_chinese_lite_train_v1.1.yml
new file mode 100755
index 0000000000000000000000000000000000000000..89333f89ad9a6af4dd744daa8972ce35f805113a
--- /dev/null
+++ b/configs/rec/ch_ppocr_v1.1/rec_chinese_lite_train_v1.1.yml
@@ -0,0 +1,54 @@
+Global:
+ algorithm: CRNN
+ use_gpu: true
+ epoch_num: 500
+ log_smooth_window: 20
+ print_batch_step: 10
+ save_model_dir: ./output/rec_CRNN
+ save_epoch_step: 3
+ eval_batch_step: 2000
+ train_batch_size_per_card: 256
+ test_batch_size_per_card: 256
+ image_shape: [3, 32, 320]
+ max_text_length: 25
+ character_type: ch
+ character_dict_path: ./ppocr/utils/ppocr_keys_v1.txt
+ loss_type: ctc
+ distort: true
+ use_space_char: true
+ reader_yml: ./configs/rec/rec_chinese_reader.yml
+ pretrain_weights:
+ checkpoints:
+ save_inference_dir:
+ infer_img:
+
+Architecture:
+ function: ppocr.modeling.architectures.rec_model,RecModel
+
+Backbone:
+ function: ppocr.modeling.backbones.rec_mobilenet_v3,MobileNetV3
+ scale: 0.5
+ model_name: small
+ small_stride: [1, 2, 2, 2]
+
+Head:
+ function: ppocr.modeling.heads.rec_ctc_head,CTCPredict
+ encoder_type: rnn
+ fc_decay: 0.00001
+ SeqRNN:
+ hidden_size: 48
+
+Loss:
+ function: ppocr.modeling.losses.rec_ctc_loss,CTCLoss
+
+Optimizer:
+ function: ppocr.optimizer,AdamDecay
+ base_lr: 0.0005
+ l2_decay: 0.00001
+ beta1: 0.9
+ beta2: 0.999
+ decay:
+ function: cosine_decay_warmup
+ step_each_epoch: 254
+ total_epoch: 500
+ warmup_minibatch: 1000
diff --git a/configs/rec/multi_languages/rec_en_lite_train.yml b/configs/rec/multi_languages/rec_en_lite_train.yml
new file mode 100644
index 0000000000000000000000000000000000000000..128424b4d3a5631f8237f6cd596c901990ff2277
--- /dev/null
+++ b/configs/rec/multi_languages/rec_en_lite_train.yml
@@ -0,0 +1,53 @@
+Global:
+ algorithm: CRNN
+ use_gpu: true
+ epoch_num: 500
+ log_smooth_window: 20
+ print_batch_step: 10
+ save_model_dir: ./output/en_number
+ save_epoch_step: 3
+ eval_batch_step: 2000
+ train_batch_size_per_card: 256
+ test_batch_size_per_card: 256
+ image_shape: [3, 32, 320]
+ max_text_length: 30
+ character_type: ch
+ character_dict_path: ./ppocr/utils/ic15_dict.txt
+ loss_type: ctc
+ distort: false
+ use_space_char: false
+ reader_yml: ./configs/rec/multi_languages/rec_en_reader.yml
+ pretrain_weights:
+ checkpoints:
+ save_inference_dir:
+ infer_img:
+
+Architecture:
+ function: ppocr.modeling.architectures.rec_model,RecModel
+
+Backbone:
+ function: ppocr.modeling.backbones.rec_mobilenet_v3,MobileNetV3
+ scale: 0.5
+ model_name: small
+ small_stride: [1, 2, 2, 2]
+
+Head:
+ function: ppocr.modeling.heads.rec_ctc_head,CTCPredict
+ encoder_type: rnn
+ SeqRNN:
+ hidden_size: 48
+
+Loss:
+ function: ppocr.modeling.losses.rec_ctc_loss,CTCLoss
+
+Optimizer:
+ function: ppocr.optimizer,AdamDecay
+ l2_decay: 0.00001
+ base_lr: 0.001
+ beta1: 0.9
+ beta2: 0.999
+ decay:
+ function: cosine_decay_warmup
+ warmup_minibatch: 1000
+ step_each_epoch: 6530
+ total_epoch: 500
diff --git a/configs/rec/multi_languages/rec_en_reader.yml b/configs/rec/multi_languages/rec_en_reader.yml
new file mode 100755
index 0000000000000000000000000000000000000000..558e2c9b653642f919b5a1e15211b934dc39ad13
--- /dev/null
+++ b/configs/rec/multi_languages/rec_en_reader.yml
@@ -0,0 +1,13 @@
+TrainReader:
+ reader_function: ppocr.data.rec.dataset_traversal,SimpleReader
+ num_workers: 8
+ img_set_dir: ./train_data
+ label_file_path: ./train_data/en_train.txt
+
+EvalReader:
+ reader_function: ppocr.data.rec.dataset_traversal,SimpleReader
+ img_set_dir: ./train_data
+ label_file_path: ./train_data/en_eval.txt
+
+TestReader:
+ reader_function: ppocr.data.rec.dataset_traversal,SimpleReader
diff --git a/configs/rec/multi_languages/rec_french_lite_train.yml b/configs/rec/multi_languages/rec_french_lite_train.yml
new file mode 100755
index 0000000000000000000000000000000000000000..2cf54c427eb6a7c64f4b54b021c44013a1dc1d6a
--- /dev/null
+++ b/configs/rec/multi_languages/rec_french_lite_train.yml
@@ -0,0 +1,52 @@
+Global:
+ algorithm: CRNN
+ use_gpu: true
+ epoch_num: 500
+ log_smooth_window: 20
+ print_batch_step: 10
+ save_model_dir: ./output/rec_french
+ save_epoch_step: 1
+ eval_batch_step: 2000
+ train_batch_size_per_card: 256
+ test_batch_size_per_card: 256
+ image_shape: [3, 32, 320]
+ max_text_length: 25
+ character_type: french
+ character_dict_path: ./ppocr/utils/french_dict.txt
+ loss_type: ctc
+ distort: true
+ use_space_char: false
+ reader_yml: ./configs/rec/multi_languages/rec_french_reader.yml
+ pretrain_weights:
+ checkpoints:
+ save_inference_dir:
+ infer_img:
+
+Architecture:
+ function: ppocr.modeling.architectures.rec_model,RecModel
+
+Backbone:
+ function: ppocr.modeling.backbones.rec_mobilenet_v3,MobileNetV3
+ scale: 0.5
+ model_name: small
+ small_stride: [1, 2, 2, 2]
+
+Head:
+ function: ppocr.modeling.heads.rec_ctc_head,CTCPredict
+ encoder_type: rnn
+ SeqRNN:
+ hidden_size: 48
+
+Loss:
+ function: ppocr.modeling.losses.rec_ctc_loss,CTCLoss
+
+Optimizer:
+ function: ppocr.optimizer,AdamDecay
+ l2_decay: 0.00001
+ base_lr: 0.001
+ beta1: 0.9
+ beta2: 0.999
+ decay:
+ function: cosine_decay
+ step_each_epoch: 254
+ total_epoch: 500
diff --git a/configs/rec/multi_languages/rec_french_reader.yml b/configs/rec/multi_languages/rec_french_reader.yml
new file mode 100755
index 0000000000000000000000000000000000000000..e456de1dc8800822cc9af496e825c45cdbebe081
--- /dev/null
+++ b/configs/rec/multi_languages/rec_french_reader.yml
@@ -0,0 +1,13 @@
+TrainReader:
+ reader_function: ppocr.data.rec.dataset_traversal,SimpleReader
+ num_workers: 8
+ img_set_dir: ./train_data
+ label_file_path: ./train_data/french_train.txt
+
+EvalReader:
+ reader_function: ppocr.data.rec.dataset_traversal,SimpleReader
+ img_set_dir: ./train_data
+ label_file_path: ./train_data/french_eval.txt
+
+TestReader:
+ reader_function: ppocr.data.rec.dataset_traversal,SimpleReader
diff --git a/configs/rec/multi_languages/rec_ger_lite_train.yml b/configs/rec/multi_languages/rec_ger_lite_train.yml
new file mode 100755
index 0000000000000000000000000000000000000000..beb1755b105fea9cbade9f35ceac15d380651f37
--- /dev/null
+++ b/configs/rec/multi_languages/rec_ger_lite_train.yml
@@ -0,0 +1,52 @@
+Global:
+ algorithm: CRNN
+ use_gpu: true
+ epoch_num: 500
+ log_smooth_window: 20
+ print_batch_step: 10
+ save_model_dir: ./output/rec_german
+ save_epoch_step: 1
+ eval_batch_step: 2000
+ train_batch_size_per_card: 256
+ test_batch_size_per_card: 256
+ image_shape: [3, 32, 320]
+ max_text_length: 25
+ character_type: german
+ character_dict_path: ./ppocr/utils/german_dict.txt
+ loss_type: ctc
+ distort: true
+ use_space_char: false
+ reader_yml: ./configs/rec/multi_languages/rec_ger_reader.yml
+ pretrain_weights:
+ checkpoints:
+ save_inference_dir:
+ infer_img:
+
+Architecture:
+ function: ppocr.modeling.architectures.rec_model,RecModel
+
+Backbone:
+ function: ppocr.modeling.backbones.rec_mobilenet_v3,MobileNetV3
+ scale: 0.5
+ model_name: small
+ small_stride: [1, 2, 2, 2]
+
+Head:
+ function: ppocr.modeling.heads.rec_ctc_head,CTCPredict
+ encoder_type: rnn
+ SeqRNN:
+ hidden_size: 48
+
+Loss:
+ function: ppocr.modeling.losses.rec_ctc_loss,CTCLoss
+
+Optimizer:
+ function: ppocr.optimizer,AdamDecay
+ l2_decay: 0.00001
+ base_lr: 0.001
+ beta1: 0.9
+ beta2: 0.999
+ decay:
+ function: cosine_decay
+ step_each_epoch: 254
+ total_epoch: 500
diff --git a/configs/rec/multi_languages/rec_ger_reader.yml b/configs/rec/multi_languages/rec_ger_reader.yml
new file mode 100755
index 0000000000000000000000000000000000000000..edd78d4f115dc7e1376556ee0c93f655ac891e47
--- /dev/null
+++ b/configs/rec/multi_languages/rec_ger_reader.yml
@@ -0,0 +1,13 @@
+TrainReader:
+ reader_function: ppocr.data.rec.dataset_traversal,SimpleReader
+ num_workers: 8
+ img_set_dir: ./train_data
+ label_file_path: ./train_data/de_train.txt
+
+EvalReader:
+ reader_function: ppocr.data.rec.dataset_traversal,SimpleReader
+ img_set_dir: ./train_data
+ label_file_path: ./train_data/de_eval.txt
+
+TestReader:
+ reader_function: ppocr.data.rec.dataset_traversal,SimpleReader
diff --git a/configs/rec/multi_languages/rec_japan_lite_train.yml b/configs/rec/multi_languages/rec_japan_lite_train.yml
new file mode 100755
index 0000000000000000000000000000000000000000..fbbab33eadd2901d9eac93f49e737e92d9441270
--- /dev/null
+++ b/configs/rec/multi_languages/rec_japan_lite_train.yml
@@ -0,0 +1,52 @@
+Global:
+ algorithm: CRNN
+ use_gpu: true
+ epoch_num: 500
+ log_smooth_window: 20
+ print_batch_step: 10
+ save_model_dir: ./output/rec_japan
+ save_epoch_step: 1
+ eval_batch_step: 2000
+ train_batch_size_per_card: 256
+ test_batch_size_per_card: 256
+ image_shape: [3, 32, 320]
+ max_text_length: 25
+ character_type: japan
+ character_dict_path: ./ppocr/utils/japan_dict.txt
+ loss_type: ctc
+ distort: true
+ use_space_char: false
+ reader_yml: ./configs/rec/multi_languages/rec_japan_reader.yml
+ pretrain_weights:
+ checkpoints:
+ save_inference_dir:
+ infer_img:
+
+Architecture:
+ function: ppocr.modeling.architectures.rec_model,RecModel
+
+Backbone:
+ function: ppocr.modeling.backbones.rec_mobilenet_v3,MobileNetV3
+ scale: 0.5
+ model_name: small
+ small_stride: [1, 2, 2, 2]
+
+Head:
+ function: ppocr.modeling.heads.rec_ctc_head,CTCPredict
+ encoder_type: rnn
+ SeqRNN:
+ hidden_size: 48
+
+Loss:
+ function: ppocr.modeling.losses.rec_ctc_loss,CTCLoss
+
+Optimizer:
+ function: ppocr.optimizer,AdamDecay
+ l2_decay: 0.00001
+ base_lr: 0.001
+ beta1: 0.9
+ beta2: 0.999
+ decay:
+ function: cosine_decay
+ step_each_epoch: 254
+ total_epoch: 500
diff --git a/configs/rec/multi_languages/rec_japan_reader.yml b/configs/rec/multi_languages/rec_japan_reader.yml
new file mode 100755
index 0000000000000000000000000000000000000000..348590920a131843a6ab7d8c76498a486d4ed709
--- /dev/null
+++ b/configs/rec/multi_languages/rec_japan_reader.yml
@@ -0,0 +1,13 @@
+TrainReader:
+ reader_function: ppocr.data.rec.dataset_traversal,SimpleReader
+ num_workers: 8
+ img_set_dir: ./train_data
+ label_file_path: ./train_data/japan_train.txt
+
+EvalReader:
+ reader_function: ppocr.data.rec.dataset_traversal,SimpleReader
+ img_set_dir: ./train_data
+ label_file_path: ./train_data/japan_eval.txt
+
+TestReader:
+ reader_function: ppocr.data.rec.dataset_traversal,SimpleReader
diff --git a/configs/rec/multi_languages/rec_korean_lite_train.yml b/configs/rec/multi_languages/rec_korean_lite_train.yml
new file mode 100755
index 0000000000000000000000000000000000000000..29cc08aaefb017c690551e030a57e85ebb21e2dd
--- /dev/null
+++ b/configs/rec/multi_languages/rec_korean_lite_train.yml
@@ -0,0 +1,52 @@
+Global:
+ algorithm: CRNN
+ use_gpu: true
+ epoch_num: 500
+ log_smooth_window: 20
+ print_batch_step: 10
+ save_model_dir: ./output/rec_korean
+ save_epoch_step: 1
+ eval_batch_step: 2000
+ train_batch_size_per_card: 256
+ test_batch_size_per_card: 256
+ image_shape: [3, 32, 320]
+ max_text_length: 25
+ character_type: korean
+ character_dict_path: ./ppocr/utils/korean_dict.txt
+ loss_type: ctc
+ distort: true
+ use_space_char: false
+ reader_yml: ./configs/rec/multi_languages/rec_korean_reader.yml
+ pretrain_weights:
+ checkpoints:
+ save_inference_dir:
+ infer_img:
+
+Architecture:
+ function: ppocr.modeling.architectures.rec_model,RecModel
+
+Backbone:
+ function: ppocr.modeling.backbones.rec_mobilenet_v3,MobileNetV3
+ scale: 0.5
+ model_name: small
+ small_stride: [1, 2, 2, 2]
+
+Head:
+ function: ppocr.modeling.heads.rec_ctc_head,CTCPredict
+ encoder_type: rnn
+ SeqRNN:
+ hidden_size: 48
+
+Loss:
+ function: ppocr.modeling.losses.rec_ctc_loss,CTCLoss
+
+Optimizer:
+ function: ppocr.optimizer,AdamDecay
+ l2_decay: 0.00001
+ base_lr: 0.001
+ beta1: 0.9
+ beta2: 0.999
+ decay:
+ function: cosine_decay
+ step_each_epoch: 254
+ total_epoch: 500
diff --git a/configs/rec/multi_languages/rec_korean_reader.yml b/configs/rec/multi_languages/rec_korean_reader.yml
new file mode 100755
index 0000000000000000000000000000000000000000..58ebf6cf8d340a06c0b3e2883be8839112980123
--- /dev/null
+++ b/configs/rec/multi_languages/rec_korean_reader.yml
@@ -0,0 +1,13 @@
+TrainReader:
+ reader_function: ppocr.data.rec.dataset_traversal,SimpleReader
+ num_workers: 8
+ img_set_dir: ./train_data
+ label_file_path: ./train_data/korean_train.txt
+
+EvalReader:
+ reader_function: ppocr.data.rec.dataset_traversal,SimpleReader
+ img_set_dir: ./train_data
+ label_file_path: ./train_data/korean_eval.txt
+
+TestReader:
+ reader_function: ppocr.data.rec.dataset_traversal,SimpleReader
diff --git a/configs/rec/rec_r50fpn_vd_none_srn.yml b/configs/rec/rec_r50fpn_vd_none_srn.yml
new file mode 100755
index 0000000000000000000000000000000000000000..30709e479f8da56b6bd7fe9ebf817a27bff9cc38
--- /dev/null
+++ b/configs/rec/rec_r50fpn_vd_none_srn.yml
@@ -0,0 +1,49 @@
+Global:
+ algorithm: SRN
+ use_gpu: true
+ epoch_num: 72
+ log_smooth_window: 20
+ print_batch_step: 10
+ save_model_dir: output/rec_pvam_withrotate
+ save_epoch_step: 1
+ eval_batch_step: 8000
+ train_batch_size_per_card: 64
+ test_batch_size_per_card: 1
+ image_shape: [1, 64, 256]
+ max_text_length: 25
+ character_type: en
+ loss_type: srn
+ num_heads: 8
+ average_window: 0.15
+ max_average_window: 15625
+ min_average_window: 10000
+ reader_yml: ./configs/rec/rec_benchmark_reader.yml
+ pretrain_weights:
+ checkpoints:
+ save_inference_dir:
+ infer_img:
+
+Architecture:
+ function: ppocr.modeling.architectures.rec_model,RecModel
+
+Backbone:
+ function: ppocr.modeling.backbones.rec_resnet_fpn,ResNet
+ layers: 50
+
+Head:
+ function: ppocr.modeling.heads.rec_srn_all_head,SRNPredict
+ encoder_type: rnn
+ num_encoder_TUs: 2
+ num_decoder_TUs: 4
+ hidden_dims: 512
+ SeqRNN:
+ hidden_size: 256
+
+Loss:
+ function: ppocr.modeling.losses.rec_srn_loss,SRNLoss
+
+Optimizer:
+ function: ppocr.optimizer,AdamDecay
+ base_lr: 0.0001
+ beta1: 0.9
+ beta2: 0.999
diff --git a/deploy/android_demo/README.md b/deploy/android_demo/README.md
index 4d85dee99ab3616594b4ff3a17acb97a6267b12d..e35e757914aa355c97293662652b1e02676e32eb 100644
--- a/deploy/android_demo/README.md
+++ b/deploy/android_demo/README.md
@@ -1,6 +1,6 @@
# 如何快速测试
### 1. 安装最新版本的Android Studio
-可以从https://developer.android.com/studio下载。本Demo使用是4.0版本Android Studio编写。
+可以从https://developer.android.com/studio 下载。本Demo使用是4.0版本Android Studio编写。
### 2. 按照NDK 20 以上版本
Demo测试的时候使用的是NDK 20b版本,20版本以上均可以支持编译成功。
diff --git a/deploy/android_demo/app/build.gradle b/deploy/android_demo/app/build.gradle
index adf3968b40960b50bc62a7ba669ce28346afa362..5ecb11692c2a66f941dc41425761519607bad39e 100644
--- a/deploy/android_demo/app/build.gradle
+++ b/deploy/android_demo/app/build.gradle
@@ -3,11 +3,11 @@ import java.security.MessageDigest
apply plugin: 'com.android.application'
android {
- compileSdkVersion 28
+ compileSdkVersion 29
defaultConfig {
applicationId "com.baidu.paddle.lite.demo.ocr"
- minSdkVersion 15
- targetSdkVersion 28
+ minSdkVersion 23
+ targetSdkVersion 29
versionCode 1
versionName "1.0"
testInstrumentationRunner "android.support.test.runner.AndroidJUnitRunner"
@@ -39,9 +39,8 @@ android {
dependencies {
implementation fileTree(include: ['*.jar'], dir: 'libs')
- implementation 'com.android.support:appcompat-v7:28.0.0'
- implementation 'com.android.support.constraint:constraint-layout:1.1.3'
- implementation 'com.android.support:design:28.0.0'
+ implementation 'androidx.appcompat:appcompat:1.1.0'
+ implementation 'androidx.constraintlayout:constraintlayout:1.1.3'
testImplementation 'junit:junit:4.12'
androidTestImplementation 'com.android.support.test:runner:1.0.2'
androidTestImplementation 'com.android.support.test.espresso:espresso-core:3.0.2'
diff --git a/deploy/android_demo/app/src/main/AndroidManifest.xml b/deploy/android_demo/app/src/main/AndroidManifest.xml
index ff1900d637a827998c4da52b9a2dda51b8ae89c8..54482b1dcc9de66021d0109e5683302c8445ba6a 100644
--- a/deploy/android_demo/app/src/main/AndroidManifest.xml
+++ b/deploy/android_demo/app/src/main/AndroidManifest.xml
@@ -14,10 +14,10 @@
android:roundIcon="@mipmap/ic_launcher_round"
android:supportsRtl="true"
android:theme="@style/AppTheme">
+
-
@@ -25,6 +25,15 @@
android:name="com.baidu.paddle.lite.demo.ocr.SettingsActivity"
android:label="Settings">
+
+
+
\ No newline at end of file
diff --git a/deploy/android_demo/app/src/main/assets/images/180.jpg b/deploy/android_demo/app/src/main/assets/images/180.jpg
new file mode 100644
index 0000000000000000000000000000000000000000..84cf4c79ef14769d01b0b0e9667387bd16b3e6e7
Binary files /dev/null and b/deploy/android_demo/app/src/main/assets/images/180.jpg differ
diff --git a/deploy/android_demo/app/src/main/assets/images/270.jpg b/deploy/android_demo/app/src/main/assets/images/270.jpg
new file mode 100644
index 0000000000000000000000000000000000000000..568739043b7779425b0abeb4459dbb485caed847
Binary files /dev/null and b/deploy/android_demo/app/src/main/assets/images/270.jpg differ
diff --git a/deploy/android_demo/app/src/main/assets/images/90.jpg b/deploy/android_demo/app/src/main/assets/images/90.jpg
new file mode 100644
index 0000000000000000000000000000000000000000..49e949aa9cc14e3afc507c5806c87d9894c2dcb9
Binary files /dev/null and b/deploy/android_demo/app/src/main/assets/images/90.jpg differ
diff --git a/deploy/android_demo/app/src/main/cpp/native.cpp b/deploy/android_demo/app/src/main/cpp/native.cpp
index 33233e5372e307a892786c6bea779691e1f6781a..963c5246d5b7b50720f92705d288526ae2cc6a73 100644
--- a/deploy/android_demo/app/src/main/cpp/native.cpp
+++ b/deploy/android_demo/app/src/main/cpp/native.cpp
@@ -4,112 +4,111 @@
#include "native.h"
#include "ocr_ppredictor.h"
-#include
#include
#include
+#include
static paddle::lite_api::PowerMode str_to_cpu_mode(const std::string &cpu_mode);
-extern "C"
-JNIEXPORT jlong JNICALL
-Java_com_baidu_paddle_lite_demo_ocr_OCRPredictorNative_init(JNIEnv *env, jobject thiz,
- jstring j_det_model_path,
- jstring j_rec_model_path,
- jint j_thread_num,
- jstring j_cpu_mode) {
- std::string det_model_path = jstring_to_cpp_string(env, j_det_model_path);
- std::string rec_model_path = jstring_to_cpp_string(env, j_rec_model_path);
- int thread_num = j_thread_num;
- std::string cpu_mode = jstring_to_cpp_string(env, j_cpu_mode);
- ppredictor::OCR_Config conf;
- conf.thread_num = thread_num;
- conf.mode = str_to_cpu_mode(cpu_mode);
- ppredictor::OCR_PPredictor *orc_predictor = new ppredictor::OCR_PPredictor{conf};
- orc_predictor->init_from_file(det_model_path, rec_model_path);
- return reinterpret_cast(orc_predictor);
+extern "C" JNIEXPORT jlong JNICALL
+Java_com_baidu_paddle_lite_demo_ocr_OCRPredictorNative_init(
+ JNIEnv *env, jobject thiz, jstring j_det_model_path,
+ jstring j_rec_model_path, jstring j_cls_model_path, jint j_thread_num,
+ jstring j_cpu_mode) {
+ std::string det_model_path = jstring_to_cpp_string(env, j_det_model_path);
+ std::string rec_model_path = jstring_to_cpp_string(env, j_rec_model_path);
+ std::string cls_model_path = jstring_to_cpp_string(env, j_cls_model_path);
+ int thread_num = j_thread_num;
+ std::string cpu_mode = jstring_to_cpp_string(env, j_cpu_mode);
+ ppredictor::OCR_Config conf;
+ conf.thread_num = thread_num;
+ conf.mode = str_to_cpu_mode(cpu_mode);
+ ppredictor::OCR_PPredictor *orc_predictor =
+ new ppredictor::OCR_PPredictor{conf};
+ orc_predictor->init_from_file(det_model_path, rec_model_path, cls_model_path);
+ return reinterpret_cast(orc_predictor);
}
/**
- * "LITE_POWER_HIGH" 转为 paddle::lite_api::LITE_POWER_HIGH
+ * "LITE_POWER_HIGH" convert to paddle::lite_api::LITE_POWER_HIGH
* @param cpu_mode
* @return
*/
-static paddle::lite_api::PowerMode str_to_cpu_mode(const std::string &cpu_mode) {
- static std::map cpu_mode_map{
- {"LITE_POWER_HIGH", paddle::lite_api::LITE_POWER_HIGH},
- {"LITE_POWER_LOW", paddle::lite_api::LITE_POWER_HIGH},
- {"LITE_POWER_FULL", paddle::lite_api::LITE_POWER_FULL},
- {"LITE_POWER_NO_BIND", paddle::lite_api::LITE_POWER_NO_BIND},
- {"LITE_POWER_RAND_HIGH", paddle::lite_api::LITE_POWER_RAND_HIGH},
- {"LITE_POWER_RAND_LOW", paddle::lite_api::LITE_POWER_RAND_LOW}
- };
- std::string upper_key;
- std::transform(cpu_mode.cbegin(), cpu_mode.cend(), upper_key.begin(), ::toupper);
- auto index = cpu_mode_map.find(upper_key);
- if (index == cpu_mode_map.end()) {
- LOGE("cpu_mode not found %s", upper_key.c_str());
- return paddle::lite_api::LITE_POWER_HIGH;
- } else {
- return index->second;
- }
-
+static paddle::lite_api::PowerMode
+str_to_cpu_mode(const std::string &cpu_mode) {
+ static std::map cpu_mode_map{
+ {"LITE_POWER_HIGH", paddle::lite_api::LITE_POWER_HIGH},
+ {"LITE_POWER_LOW", paddle::lite_api::LITE_POWER_HIGH},
+ {"LITE_POWER_FULL", paddle::lite_api::LITE_POWER_FULL},
+ {"LITE_POWER_NO_BIND", paddle::lite_api::LITE_POWER_NO_BIND},
+ {"LITE_POWER_RAND_HIGH", paddle::lite_api::LITE_POWER_RAND_HIGH},
+ {"LITE_POWER_RAND_LOW", paddle::lite_api::LITE_POWER_RAND_LOW}};
+ std::string upper_key;
+ std::transform(cpu_mode.cbegin(), cpu_mode.cend(), upper_key.begin(),
+ ::toupper);
+ auto index = cpu_mode_map.find(upper_key);
+ if (index == cpu_mode_map.end()) {
+ LOGE("cpu_mode not found %s", upper_key.c_str());
+ return paddle::lite_api::LITE_POWER_HIGH;
+ } else {
+ return index->second;
+ }
}
-extern "C"
-JNIEXPORT jfloatArray JNICALL
-Java_com_baidu_paddle_lite_demo_ocr_OCRPredictorNative_forward(JNIEnv *env, jobject thiz,
- jlong java_pointer, jfloatArray buf,
- jfloatArray ddims,
- jobject original_image) {
- LOGI("begin to run native forward");
- if (java_pointer == 0) {
- LOGE("JAVA pointer is NULL");
- return cpp_array_to_jfloatarray(env, nullptr, 0);
- }
- cv::Mat origin = bitmap_to_cv_mat(env, original_image);
- if (origin.size == 0) {
- LOGE("origin bitmap cannot convert to CV Mat");
- return cpp_array_to_jfloatarray(env, nullptr, 0);
- }
- ppredictor::OCR_PPredictor *ppredictor = (ppredictor::OCR_PPredictor *) java_pointer;
- std::vector dims_float_arr = jfloatarray_to_float_vector(env, ddims);
- std::vector dims_arr;
- dims_arr.resize(dims_float_arr.size());
- std::copy(dims_float_arr.cbegin(), dims_float_arr.cend(), dims_arr.begin());
+extern "C" JNIEXPORT jfloatArray JNICALL
+Java_com_baidu_paddle_lite_demo_ocr_OCRPredictorNative_forward(
+ JNIEnv *env, jobject thiz, jlong java_pointer, jfloatArray buf,
+ jfloatArray ddims, jobject original_image) {
+ LOGI("begin to run native forward");
+ if (java_pointer == 0) {
+ LOGE("JAVA pointer is NULL");
+ return cpp_array_to_jfloatarray(env, nullptr, 0);
+ }
+ cv::Mat origin = bitmap_to_cv_mat(env, original_image);
+ if (origin.size == 0) {
+ LOGE("origin bitmap cannot convert to CV Mat");
+ return cpp_array_to_jfloatarray(env, nullptr, 0);
+ }
+ ppredictor::OCR_PPredictor *ppredictor =
+ (ppredictor::OCR_PPredictor *)java_pointer;
+ std::vector dims_float_arr = jfloatarray_to_float_vector(env, ddims);
+ std::vector dims_arr;
+ dims_arr.resize(dims_float_arr.size());
+ std::copy(dims_float_arr.cbegin(), dims_float_arr.cend(), dims_arr.begin());
- // 这里值有点大,就不调用jfloatarray_to_float_vector了
- int64_t buf_len = (int64_t) env->GetArrayLength(buf);
- jfloat *buf_data = env->GetFloatArrayElements(buf, JNI_FALSE);
- float *data = (jfloat *) buf_data;
- std::vector results = ppredictor->infer_ocr(dims_arr, data,
- buf_len,
- NET_OCR, origin);
- LOGI("infer_ocr finished with boxes %ld", results.size());
- // 这里将std::vector 序列化成 float数组,传输到java层再反序列化
- std::vector float_arr;
- for (const ppredictor::OCRPredictResult &r :results) {
- float_arr.push_back(r.points.size());
- float_arr.push_back(r.word_index.size());
- float_arr.push_back(r.score);
- for (const std::vector &point : r.points) {
- float_arr.push_back(point.at(0));
- float_arr.push_back(point.at(1));
- }
- for (int index: r.word_index) {
- float_arr.push_back(index);
- }
+ // 这里值有点大,就不调用jfloatarray_to_float_vector了
+ int64_t buf_len = (int64_t)env->GetArrayLength(buf);
+ jfloat *buf_data = env->GetFloatArrayElements(buf, JNI_FALSE);
+ float *data = (jfloat *)buf_data;
+ std::vector results =
+ ppredictor->infer_ocr(dims_arr, data, buf_len, NET_OCR, origin);
+ LOGI("infer_ocr finished with boxes %ld", results.size());
+ // 这里将std::vector 序列化成
+ // float数组,传输到java层再反序列化
+ std::vector float_arr;
+ for (const ppredictor::OCRPredictResult &r : results) {
+ float_arr.push_back(r.points.size());
+ float_arr.push_back(r.word_index.size());
+ float_arr.push_back(r.score);
+ for (const std::vector &point : r.points) {
+ float_arr.push_back(point.at(0));
+ float_arr.push_back(point.at(1));
}
- return cpp_array_to_jfloatarray(env, float_arr.data(), float_arr.size());
+ for (int index : r.word_index) {
+ float_arr.push_back(index);
+ }
+ }
+ return cpp_array_to_jfloatarray(env, float_arr.data(), float_arr.size());
}
-extern "C"
-JNIEXPORT void JNICALL
-Java_com_baidu_paddle_lite_demo_ocr_OCRPredictorNative_release(JNIEnv *env, jobject thiz,
- jlong java_pointer){
- if (java_pointer == 0) {
- LOGE("JAVA pointer is NULL");
- return;
- }
- ppredictor::OCR_PPredictor *ppredictor = (ppredictor::OCR_PPredictor *) java_pointer;
- delete ppredictor;
+extern "C" JNIEXPORT void JNICALL
+Java_com_baidu_paddle_lite_demo_ocr_OCRPredictorNative_release(
+ JNIEnv *env, jobject thiz, jlong java_pointer) {
+ if (java_pointer == 0) {
+ LOGE("JAVA pointer is NULL");
+ return;
+ }
+ ppredictor::OCR_PPredictor *ppredictor =
+ (ppredictor::OCR_PPredictor *)java_pointer;
+ delete ppredictor;
}
\ No newline at end of file
diff --git a/deploy/android_demo/app/src/main/cpp/ocr_cls_process.cpp b/deploy/android_demo/app/src/main/cpp/ocr_cls_process.cpp
new file mode 100644
index 0000000000000000000000000000000000000000..d720066667b60ee87bc1a1227ad720074254074e
--- /dev/null
+++ b/deploy/android_demo/app/src/main/cpp/ocr_cls_process.cpp
@@ -0,0 +1,46 @@
+// Copyright (c) 2020 PaddlePaddle Authors. All Rights Reserved.
+//
+// Licensed under the Apache License, Version 2.0 (the "License");
+// you may not use this file except in compliance with the License.
+// You may obtain a copy of the License at
+//
+// http://www.apache.org/licenses/LICENSE-2.0
+//
+// Unless required by applicable law or agreed to in writing, software
+// distributed under the License is distributed on an "AS IS" BASIS,
+// WITHOUT WARRANTIES OR CONDITIONS OF ANY KIND, either express or implied.
+// See the License for the specific language governing permissions and
+// limitations under the License.
+
+#include "ocr_cls_process.h"
+#include
+#include
+#include
+#include
+#include
+#include
+
+const std::vector CLS_IMAGE_SHAPE = {3, 32, 100};
+
+cv::Mat cls_resize_img(const cv::Mat &img) {
+ int imgC = CLS_IMAGE_SHAPE[0];
+ int imgW = CLS_IMAGE_SHAPE[2];
+ int imgH = CLS_IMAGE_SHAPE[1];
+
+ float ratio = float(img.cols) / float(img.rows);
+ int resize_w = 0;
+ if (ceilf(imgH * ratio) > imgW)
+ resize_w = imgW;
+ else
+ resize_w = int(ceilf(imgH * ratio));
+
+ cv::Mat resize_img;
+ cv::resize(img, resize_img, cv::Size(resize_w, imgH), 0.f, 0.f,
+ cv::INTER_CUBIC);
+
+ if (resize_w < imgW) {
+ cv::copyMakeBorder(resize_img, resize_img, 0, 0, 0, int(imgW - resize_w),
+ cv::BORDER_CONSTANT, {0, 0, 0});
+ }
+ return resize_img;
+}
\ No newline at end of file
diff --git a/deploy/android_demo/app/src/main/cpp/ocr_cls_process.h b/deploy/android_demo/app/src/main/cpp/ocr_cls_process.h
new file mode 100644
index 0000000000000000000000000000000000000000..1c30ee1071e647ce1ab7050ac0641d0eff7c62ad
--- /dev/null
+++ b/deploy/android_demo/app/src/main/cpp/ocr_cls_process.h
@@ -0,0 +1,23 @@
+// Copyright (c) 2020 PaddlePaddle Authors. All Rights Reserved.
+//
+// Licensed under the Apache License, Version 2.0 (the "License");
+// you may not use this file except in compliance with the License.
+// You may obtain a copy of the License at
+//
+// http://www.apache.org/licenses/LICENSE-2.0
+//
+// Unless required by applicable law or agreed to in writing, software
+// distributed under the License is distributed on an "AS IS" BASIS,
+// WITHOUT WARRANTIES OR CONDITIONS OF ANY KIND, either express or implied.
+// See the License for the specific language governing permissions and
+// limitations under the License.
+
+#pragma once
+
+#include "common.h"
+#include
+#include
+
+extern const std::vector CLS_IMAGE_SHAPE;
+
+cv::Mat cls_resize_img(const cv::Mat &img);
\ No newline at end of file
diff --git a/deploy/android_demo/app/src/main/cpp/ocr_ppredictor.cpp b/deploy/android_demo/app/src/main/cpp/ocr_ppredictor.cpp
index 6548157b7ecac09ca3802ee6e226d555bfcd9099..f0d855e83f010ef762cb4b01086e41a0f64fb4cb 100644
--- a/deploy/android_demo/app/src/main/cpp/ocr_ppredictor.cpp
+++ b/deploy/android_demo/app/src/main/cpp/ocr_ppredictor.cpp
@@ -3,184 +3,237 @@
//
#include "ocr_ppredictor.h"
-#include "preprocess.h"
#include "common.h"
-#include "ocr_db_post_process.h"
+#include "ocr_cls_process.h"
#include "ocr_crnn_process.h"
+#include "ocr_db_post_process.h"
+#include "preprocess.h"
namespace ppredictor {
-OCR_PPredictor::OCR_PPredictor(const OCR_Config &config) : _config(config) {
+OCR_PPredictor::OCR_PPredictor(const OCR_Config &config) : _config(config) {}
-}
+int OCR_PPredictor::init(const std::string &det_model_content,
+ const std::string &rec_model_content,
+ const std::string &cls_model_content) {
+ _det_predictor = std::unique_ptr(
+ new PPredictor{_config.thread_num, NET_OCR, _config.mode});
+ _det_predictor->init_nb(det_model_content);
-int
-OCR_PPredictor::init(const std::string &det_model_content, const std::string &rec_model_content) {
- _det_predictor = std::unique_ptr(
- new PPredictor{_config.thread_num, NET_OCR, _config.mode});
- _det_predictor->init_nb(det_model_content);
+ _rec_predictor = std::unique_ptr(
+ new PPredictor{_config.thread_num, NET_OCR_INTERNAL, _config.mode});
+ _rec_predictor->init_nb(rec_model_content);
- _rec_predictor = std::unique_ptr(
- new PPredictor{_config.thread_num, NET_OCR_INTERNAL, _config.mode});
- _rec_predictor->init_nb(rec_model_content);
- return RETURN_OK;
+ _cls_predictor = std::unique_ptr(
+ new PPredictor{_config.thread_num, NET_OCR_INTERNAL, _config.mode});
+ _cls_predictor->init_nb(cls_model_content);
+ return RETURN_OK;
}
-int OCR_PPredictor::init_from_file(const std::string &det_model_path, const std::string &rec_model_path){
- _det_predictor = std::unique_ptr(
- new PPredictor{_config.thread_num, NET_OCR, _config.mode});
- _det_predictor->init_from_file(det_model_path);
-
- _rec_predictor = std::unique_ptr(
- new PPredictor{_config.thread_num, NET_OCR_INTERNAL, _config.mode});
- _rec_predictor->init_from_file(rec_model_path);
- return RETURN_OK;
+int OCR_PPredictor::init_from_file(const std::string &det_model_path,
+ const std::string &rec_model_path,
+ const std::string &cls_model_path) {
+ _det_predictor = std::unique_ptr(
+ new PPredictor{_config.thread_num, NET_OCR, _config.mode});
+ _det_predictor->init_from_file(det_model_path);
+
+ _rec_predictor = std::unique_ptr(
+ new PPredictor{_config.thread_num, NET_OCR_INTERNAL, _config.mode});
+ _rec_predictor->init_from_file(rec_model_path);
+
+ _cls_predictor = std::unique_ptr(
+ new PPredictor{_config.thread_num, NET_OCR_INTERNAL, _config.mode});
+ _cls_predictor->init_from_file(cls_model_path);
+ return RETURN_OK;
}
/**
- * 调试用,保存第一步的框选结果
+ * for debug use, show result of First Step
* @param filter_boxes
* @param boxes
* @param srcimg
*/
-static void visual_img(const std::vector>> &filter_boxes,
- const std::vector>> &boxes,
- const cv::Mat &srcimg) {
- // visualization
- cv::Point rook_points[filter_boxes.size()][4];
- for (int n = 0; n < filter_boxes.size(); n++) {
- for (int m = 0; m < filter_boxes[0].size(); m++) {
- rook_points[n][m] = cv::Point(int(filter_boxes[n][m][0]), int(filter_boxes[n][m][1]));
- }
+static void
+visual_img(const std::vector>> &filter_boxes,
+ const std::vector>> &boxes,
+ const cv::Mat &srcimg) {
+ // visualization
+ cv::Point rook_points[filter_boxes.size()][4];
+ for (int n = 0; n < filter_boxes.size(); n++) {
+ for (int m = 0; m < filter_boxes[0].size(); m++) {
+ rook_points[n][m] =
+ cv::Point(int(filter_boxes[n][m][0]), int(filter_boxes[n][m][1]));
}
-
- cv::Mat img_vis;
- srcimg.copyTo(img_vis);
- for (int n = 0; n < boxes.size(); n++) {
- const cv::Point *ppt[1] = {rook_points[n]};
- int npt[] = {4};
- cv::polylines(img_vis, ppt, npt, 1, 1, CV_RGB(0, 255, 0), 2, 8, 0);
- }
- // 调试用,自行替换需要修改的路径
- cv::imwrite("/sdcard/1/vis.png", img_vis);
+ }
+
+ cv::Mat img_vis;
+ srcimg.copyTo(img_vis);
+ for (int n = 0; n < boxes.size(); n++) {
+ const cv::Point *ppt[1] = {rook_points[n]};
+ int npt[] = {4};
+ cv::polylines(img_vis, ppt, npt, 1, 1, CV_RGB(0, 255, 0), 2, 8, 0);
+ }
+ // 调试用,自行替换需要修改的路径
+ cv::imwrite("/sdcard/1/vis.png", img_vis);
}
std::vector
-OCR_PPredictor::infer_ocr(const std::vector &dims, const float *input_data, int input_len,
- int net_flag, cv::Mat &origin) {
+OCR_PPredictor::infer_ocr(const std::vector &dims,
+ const float *input_data, int input_len, int net_flag,
+ cv::Mat &origin) {
+ PredictorInput input = _det_predictor->get_first_input();
+ input.set_dims(dims);
+ input.set_data(input_data, input_len);
+ std::vector results = _det_predictor->infer();
+ PredictorOutput &res = results.at(0);
+ std::vector>> filtered_box = calc_filtered_boxes(
+ res.get_float_data(), res.get_size(), (int)dims[2], (int)dims[3], origin);
+ LOGI("Filter_box size %ld", filtered_box.size());
+ return infer_rec(filtered_box, origin);
+}
- PredictorInput input = _det_predictor->get_first_input();
+std::vector OCR_PPredictor::infer_rec(
+ const std::vector>> &boxes,
+ const cv::Mat &origin_img) {
+ std::vector mean = {0.5f, 0.5f, 0.5f};
+ std::vector scale = {1 / 0.5f, 1 / 0.5f, 1 / 0.5f};
+ std::vector dims = {1, 3, 0, 0};
+ std::vector ocr_results;
+
+ PredictorInput input = _rec_predictor->get_first_input();
+ for (auto bp = boxes.crbegin(); bp != boxes.crend(); ++bp) {
+ const std::vector> &box = *bp;
+ cv::Mat crop_img = get_rotate_crop_image(origin_img, box);
+ crop_img = infer_cls(crop_img);
+
+ float wh_ratio = float(crop_img.cols) / float(crop_img.rows);
+ cv::Mat input_image = crnn_resize_img(crop_img, wh_ratio);
+ input_image.convertTo(input_image, CV_32FC3, 1 / 255.0f);
+ const float *dimg = reinterpret_cast(input_image.data);
+ int input_size = input_image.rows * input_image.cols;
+
+ dims[2] = input_image.rows;
+ dims[3] = input_image.cols;
input.set_dims(dims);
- input.set_data(input_data, input_len);
- std::vector results = _det_predictor->infer();
- PredictorOutput &res = results.at(0);
- std::vector>> filtered_box
- = calc_filtered_boxes(res.get_float_data(), res.get_size(), (int) dims[2], (int) dims[3],
- origin);
- LOGI("Filter_box size %ld", filtered_box.size());
- return infer_rec(filtered_box, origin);
-}
-std::vector
-OCR_PPredictor::infer_rec(const std::vector>> &boxes,
- const cv::Mat &origin_img) {
- std::vector mean = {0.5f, 0.5f, 0.5f};
- std::vector scale = {1 / 0.5f, 1 / 0.5f, 1 / 0.5f};
- std::vector dims = {1, 3, 0, 0};
- std::vector ocr_results;
-
- PredictorInput input = _rec_predictor->get_first_input();
- for (auto bp = boxes.crbegin(); bp != boxes.crend(); ++bp) {
- const std::vector> &box = *bp;
- cv::Mat crop_img = get_rotate_crop_image(origin_img, box);
- float wh_ratio = float(crop_img.cols) / float(crop_img.rows);
- cv::Mat input_image = crnn_resize_img(crop_img, wh_ratio);
- input_image.convertTo(input_image, CV_32FC3, 1 / 255.0f);
- const float *dimg = reinterpret_cast(input_image.data);
- int input_size = input_image.rows * input_image.cols;
-
- dims[2] = input_image.rows;
- dims[3] = input_image.cols;
- input.set_dims(dims);
-
- neon_mean_scale(dimg, input.get_mutable_float_data(), input_size, mean, scale);
-
- std::vector results = _rec_predictor->infer();
-
- OCRPredictResult res;
- res.word_index = postprocess_rec_word_index(results.at(0));
- if (res.word_index.empty()) {
- continue;
- }
- res.score = postprocess_rec_score(results.at(1));
- res.points = box;
- ocr_results.emplace_back(std::move(res));
+ neon_mean_scale(dimg, input.get_mutable_float_data(), input_size, mean,
+ scale);
+
+ std::vector results = _rec_predictor->infer();
+
+ OCRPredictResult res;
+ res.word_index = postprocess_rec_word_index(results.at(0));
+ if (res.word_index.empty()) {
+ continue;
}
- LOGI("ocr_results finished %lu", ocr_results.size());
- return ocr_results;
+ res.score = postprocess_rec_score(results.at(1));
+ res.points = box;
+ ocr_results.emplace_back(std::move(res));
+ }
+ LOGI("ocr_results finished %lu", ocr_results.size());
+ return ocr_results;
+}
+
+cv::Mat OCR_PPredictor::infer_cls(const cv::Mat &img, float thresh) {
+ std::vector mean = {0.5f, 0.5f, 0.5f};
+ std::vector scale = {1 / 0.5f, 1 / 0.5f, 1 / 0.5f};
+ std::vector dims = {1, 3, 0, 0};
+ std::vector ocr_results;
+
+ PredictorInput input = _cls_predictor->get_first_input();
+
+ cv::Mat input_image = cls_resize_img(img);
+ input_image.convertTo(input_image, CV_32FC3, 1 / 255.0f);
+ const float *dimg = reinterpret_cast(input_image.data);
+ int input_size = input_image.rows * input_image.cols;
+
+ dims[2] = input_image.rows;
+ dims[3] = input_image.cols;
+ input.set_dims(dims);
+
+ neon_mean_scale(dimg, input.get_mutable_float_data(), input_size, mean,
+ scale);
+
+ std::vector results = _cls_predictor->infer();
+
+ const float *scores = results.at(0).get_float_data();
+ const int *labels = results.at(1).get_int_data();
+ for (int64_t i = 0; i < results.at(0).get_size(); i++) {
+ LOGI("output scores [%f]", scores[i]);
+ }
+ for (int64_t i = 0; i < results.at(1).get_size(); i++) {
+ LOGI("output label [%d]", labels[i]);
+ }
+ int label_idx = labels[0];
+ float score = scores[label_idx];
+
+ cv::Mat srcimg;
+ img.copyTo(srcimg);
+ if (label_idx % 2 == 1 && score > thresh) {
+ cv::rotate(srcimg, srcimg, 1);
+ }
+ return srcimg;
}
std::vector>>
-OCR_PPredictor::calc_filtered_boxes(const float *pred, int pred_size, int output_height,
- int output_width, const cv::Mat &origin) {
- const double threshold = 0.3;
- const double maxvalue = 1;
-
- cv::Mat pred_map = cv::Mat::zeros(output_height, output_width, CV_32F);
- memcpy(pred_map.data, pred, pred_size * sizeof(float));
- cv::Mat cbuf_map;
- pred_map.convertTo(cbuf_map, CV_8UC1);
-
- cv::Mat bit_map;
- cv::threshold(cbuf_map, bit_map, threshold, maxvalue, cv::THRESH_BINARY);
-
- std::vector>> boxes = boxes_from_bitmap(pred_map, bit_map);
- float ratio_h = output_height * 1.0f / origin.rows;
- float ratio_w = output_width * 1.0f / origin.cols;
- std::vector>> filter_boxes = filter_tag_det_res(boxes, ratio_h,
- ratio_w, origin);
- return filter_boxes;
+OCR_PPredictor::calc_filtered_boxes(const float *pred, int pred_size,
+ int output_height, int output_width,
+ const cv::Mat &origin) {
+ const double threshold = 0.3;
+ const double maxvalue = 1;
+
+ cv::Mat pred_map = cv::Mat::zeros(output_height, output_width, CV_32F);
+ memcpy(pred_map.data, pred, pred_size * sizeof(float));
+ cv::Mat cbuf_map;
+ pred_map.convertTo(cbuf_map, CV_8UC1);
+
+ cv::Mat bit_map;
+ cv::threshold(cbuf_map, bit_map, threshold, maxvalue, cv::THRESH_BINARY);
+
+ std::vector>> boxes =
+ boxes_from_bitmap(pred_map, bit_map);
+ float ratio_h = output_height * 1.0f / origin.rows;
+ float ratio_w = output_width * 1.0f / origin.cols;
+ std::vector>> filter_boxes =
+ filter_tag_det_res(boxes, ratio_h, ratio_w, origin);
+ return filter_boxes;
}
-std::vector OCR_PPredictor::postprocess_rec_word_index(const PredictorOutput &res) {
- const int *rec_idx = res.get_int_data();
- const std::vector> rec_idx_lod = res.get_lod();
+std::vector
+OCR_PPredictor::postprocess_rec_word_index(const PredictorOutput &res) {
+ const int *rec_idx = res.get_int_data();
+ const std::vector> rec_idx_lod = res.get_lod();
- std::vector pred_idx;
- for (int n = int(rec_idx_lod[0][0]); n < int(rec_idx_lod[0][1] * 2); n += 2) {
- pred_idx.emplace_back(rec_idx[n]);
- }
- return pred_idx;
+ std::vector pred_idx;
+ for (int n = int(rec_idx_lod[0][0]); n < int(rec_idx_lod[0][1] * 2); n += 2) {
+ pred_idx.emplace_back(rec_idx[n]);
+ }
+ return pred_idx;
}
float OCR_PPredictor::postprocess_rec_score(const PredictorOutput &res) {
- const float *predict_batch = res.get_float_data();
- const std::vector predict_shape = res.get_shape();
- const std::vector> predict_lod = res.get_lod();
- int blank = predict_shape[1];
- float score = 0.f;
- int count = 0;
- for (int n = predict_lod[0][0]; n < predict_lod[0][1] - 1; n++) {
- int argmax_idx = argmax(predict_batch + n * predict_shape[1],
- predict_batch + (n + 1) * predict_shape[1]);
- float max_value = predict_batch[n * predict_shape[1] + argmax_idx];
- if (blank - 1 - argmax_idx > 1e-5) {
- score += max_value;
- count += 1;
- }
-
- }
- if (count == 0) {
- LOGE("calc score count 0");
- } else {
- score /= count;
+ const float *predict_batch = res.get_float_data();
+ const std::vector predict_shape = res.get_shape();
+ const std::vector> predict_lod = res.get_lod();
+ int blank = predict_shape[1];
+ float score = 0.f;
+ int count = 0;
+ for (int n = predict_lod[0][0]; n < predict_lod[0][1] - 1; n++) {
+ int argmax_idx = argmax(predict_batch + n * predict_shape[1],
+ predict_batch + (n + 1) * predict_shape[1]);
+ float max_value = predict_batch[n * predict_shape[1] + argmax_idx];
+ if (blank - 1 - argmax_idx > 1e-5) {
+ score += max_value;
+ count += 1;
}
- LOGI("calc score: %f", score);
- return score;
-
+ }
+ if (count == 0) {
+ LOGE("calc score count 0");
+ } else {
+ score /= count;
+ }
+ LOGI("calc score: %f", score);
+ return score;
}
-
-NET_TYPE OCR_PPredictor::get_net_flag() const {
- return NET_OCR;
-}
+NET_TYPE OCR_PPredictor::get_net_flag() const { return NET_OCR; }
}
\ No newline at end of file
diff --git a/deploy/android_demo/app/src/main/cpp/ocr_ppredictor.h b/deploy/android_demo/app/src/main/cpp/ocr_ppredictor.h
index 9adbf1e35214d4c83230ecf89b650aa1c1125a8f..0ec458a4952cbc605e9979ce7850bdeab36c4629 100644
--- a/deploy/android_demo/app/src/main/cpp/ocr_ppredictor.h
+++ b/deploy/android_demo/app/src/main/cpp/ocr_ppredictor.h
@@ -4,109 +4,119 @@
#pragma once
-#include
+#include "ppredictor.h"
#include
#include
-#include "ppredictor.h"
+#include
namespace ppredictor {
/**
- * 配置
+ * Config
*/
struct OCR_Config {
- int thread_num = 4; // 线程数
- paddle::lite_api::PowerMode mode = paddle::lite_api::LITE_POWER_HIGH; // PaddleLite Mode
+ int thread_num = 4; // Thread num
+ paddle::lite_api::PowerMode mode =
+ paddle::lite_api::LITE_POWER_HIGH; // PaddleLite Mode
};
/**
- * 一个四边形内图片的推理结果,
+ * PolyGone Result
*/
struct OCRPredictResult {
- std::vector word_index; //
- std::vector> points;
- float score;
+ std::vector word_index;
+ std::vector> points;
+ float score;
};
/**
- * OCR 一共有2个模型进行推理,
- * 1. 使用第一个模型(det),框选出多个四边形
- * 2. 从原图从抠出这些多边形,使用第二个模型(rec),获取文本
+ * OCR there are 2 models
+ * 1. First model(det),select polygones to show where are the texts
+ * 2. crop from the origin images, use these polygones to infer
*/
class OCR_PPredictor : public PPredictor_Interface {
public:
- OCR_PPredictor(const OCR_Config &config);
-
- virtual ~OCR_PPredictor() {
-
- }
-
- /**
- * 初始化二个模型的Predictor
- * @param det_model_content
- * @param rec_model_content
- * @return
- */
- int init(const std::string &det_model_content, const std::string &rec_model_content);
- int init_from_file(const std::string &det_model_path, const std::string &rec_model_path);
- /**
- * 返回OCR结果
- * @param dims
- * @param input_data
- * @param input_len
- * @param net_flag
- * @param origin
- * @return
- */
- virtual std::vector
- infer_ocr(const std::vector &dims, const float *input_data, int input_len,
- int net_flag, cv::Mat &origin);
-
-
- virtual NET_TYPE get_net_flag() const;
-
+ OCR_PPredictor(const OCR_Config &config);
+
+ virtual ~OCR_PPredictor() {}
+
+ /**
+ * 初始化二个模型的Predictor
+ * @param det_model_content
+ * @param rec_model_content
+ * @return
+ */
+ int init(const std::string &det_model_content,
+ const std::string &rec_model_content,
+ const std::string &cls_model_content);
+ int init_from_file(const std::string &det_model_path,
+ const std::string &rec_model_path,
+ const std::string &cls_model_path);
+ /**
+ * Return OCR result
+ * @param dims
+ * @param input_data
+ * @param input_len
+ * @param net_flag
+ * @param origin
+ * @return
+ */
+ virtual std::vector
+ infer_ocr(const std::vector &dims, const float *input_data,
+ int input_len, int net_flag, cv::Mat &origin);
+
+ virtual NET_TYPE get_net_flag() const;
private:
-
- /**
- * 从第一个模型的结果中计算有文字的四边形
- * @param pred
- * @param output_height
- * @param output_width
- * @param origin
- * @return
- */
- std::vector>>
- calc_filtered_boxes(const float *pred, int pred_size, int output_height, int output_width,
- const cv::Mat &origin);
-
- /**
- * 第二个模型的推理
- *
- * @param boxes
- * @param origin
- * @return
- */
- std::vector
- infer_rec(const std::vector>> &boxes, const cv::Mat &origin);
-
- /**
- * 第二个模型提取文字的后处理
- * @param res
- * @return
- */
- std::vector postprocess_rec_word_index(const PredictorOutput &res);
-
- /**
- * 计算第二个模型的文字的置信度
- * @param res
- * @return
- */
- float postprocess_rec_score(const PredictorOutput &res);
-
- std::unique_ptr _det_predictor;
- std::unique_ptr _rec_predictor;
- OCR_Config _config;
-
+ /**
+ * calcul Polygone from the result image of first model
+ * @param pred
+ * @param output_height
+ * @param output_width
+ * @param origin
+ * @return
+ */
+ std::vector>>
+ calc_filtered_boxes(const float *pred, int pred_size, int output_height,
+ int output_width, const cv::Mat &origin);
+
+ /**
+ * infer for second model
+ *
+ * @param boxes
+ * @param origin
+ * @return
+ */
+ std::vector
+ infer_rec(const std::vector>> &boxes,
+ const cv::Mat &origin);
+
+ /**
+ * infer for cls model
+ *
+ * @param boxes
+ * @param origin
+ * @return
+ */
+ cv::Mat infer_cls(const cv::Mat &origin, float thresh = 0.5);
+
+ /**
+ * Postprocess or sencod model to extract text
+ * @param res
+ * @return
+ */
+ std::vector postprocess_rec_word_index(const PredictorOutput &res);
+
+ /**
+ * calculate confidence of second model text result
+ * @param res
+ * @return
+ */
+ float postprocess_rec_score(const PredictorOutput &res);
+
+ std::unique_ptr _det_predictor;
+ std::unique_ptr _rec_predictor;
+ std::unique_ptr _cls_predictor;
+ OCR_Config _config;
};
}
diff --git a/deploy/android_demo/app/src/main/cpp/ppredictor.h b/deploy/android_demo/app/src/main/cpp/ppredictor.h
index 9cdf3a88170ca2fbae9a2b1d8353fc99ebdfb971..1391109f9197b5e53796c940857c9d01b30a1125 100644
--- a/deploy/android_demo/app/src/main/cpp/ppredictor.h
+++ b/deploy/android_demo/app/src/main/cpp/ppredictor.h
@@ -7,7 +7,7 @@
namespace ppredictor {
/**
- * PaddleLite Preditor 通用接口
+ * PaddleLite Preditor Common Interface
*/
class PPredictor_Interface {
public:
@@ -21,7 +21,7 @@ public:
};
/**
- * 通用推理
+ * Common Predictor
*/
class PPredictor : public PPredictor_Interface {
public:
@@ -33,9 +33,9 @@ public:
}
/**
- * 初始化paddlitelite的opt模型,nb格式,与init_paddle二选一
+ * init paddlitelite opt model,nb format ,or use ini_paddle
* @param model_content
- * @return 0 目前是固定值0, 之后其他值表示失败
+ * @return 0
*/
virtual int init_nb(const std::string &model_content);
diff --git a/deploy/android_demo/app/src/main/cpp/predictor_output.h b/deploy/android_demo/app/src/main/cpp/predictor_output.h
index c56e2d9a4e9890faae89d6d183b81773a9c9a228..ec7086c62f0d5ca555ec17b38b27b6eea824fdb5 100644
--- a/deploy/android_demo/app/src/main/cpp/predictor_output.h
+++ b/deploy/android_demo/app/src/main/cpp/predictor_output.h
@@ -21,10 +21,10 @@ public:
const std::vector> get_lod() const;
const std::vector get_shape() const;
- std::vector data; // 通常是float返回,与下面的data_int二选一
- std::vector data_int; // 少数层是int返回,与 data二选一
- std::vector shape; // PaddleLite输出层的shape
- std::vector> lod; // PaddleLite输出层的lod
+ std::vector data; // return float, or use data_int
+ std::vector data_int; // several layers return int ,or use data
+ std::vector shape; // PaddleLite output shape
+ std::vector> lod; // PaddleLite output lod
private:
std::unique_ptr _tensor;
diff --git a/deploy/android_demo/app/src/main/java/com/baidu/paddle/lite/demo/ocr/AppCompatPreferenceActivity.java b/deploy/android_demo/app/src/main/java/com/baidu/paddle/lite/demo/ocr/AppCompatPreferenceActivity.java
index 397e4e39fe35a541fd534634ac509b94dd4b2b86..49af0afea425561d65d435a8fe67e96e98912680 100644
--- a/deploy/android_demo/app/src/main/java/com/baidu/paddle/lite/demo/ocr/AppCompatPreferenceActivity.java
+++ b/deploy/android_demo/app/src/main/java/com/baidu/paddle/lite/demo/ocr/AppCompatPreferenceActivity.java
@@ -19,15 +19,16 @@ package com.baidu.paddle.lite.demo.ocr;
import android.content.res.Configuration;
import android.os.Bundle;
import android.preference.PreferenceActivity;
-import android.support.annotation.LayoutRes;
-import android.support.annotation.Nullable;
-import android.support.v7.app.ActionBar;
-import android.support.v7.app.AppCompatDelegate;
-import android.support.v7.widget.Toolbar;
import android.view.MenuInflater;
import android.view.View;
import android.view.ViewGroup;
+import androidx.annotation.LayoutRes;
+import androidx.annotation.Nullable;
+import androidx.appcompat.app.ActionBar;
+import androidx.appcompat.app.AppCompatDelegate;
+import androidx.appcompat.widget.Toolbar;
+
/**
* A {@link PreferenceActivity} which implements and proxies the necessary calls
* to be used with AppCompat.
diff --git a/deploy/android_demo/app/src/main/java/com/baidu/paddle/lite/demo/ocr/MainActivity.java b/deploy/android_demo/app/src/main/java/com/baidu/paddle/lite/demo/ocr/MainActivity.java
index b72d72df47a3c6d769559230185c50823276fe85..afb261dcf2afb2a2eaebf58c8c1f30f89200c902 100644
--- a/deploy/android_demo/app/src/main/java/com/baidu/paddle/lite/demo/ocr/MainActivity.java
+++ b/deploy/android_demo/app/src/main/java/com/baidu/paddle/lite/demo/ocr/MainActivity.java
@@ -3,23 +3,22 @@ package com.baidu.paddle.lite.demo.ocr;
import android.Manifest;
import android.app.ProgressDialog;
import android.content.ContentResolver;
+import android.content.Context;
import android.content.Intent;
import android.content.SharedPreferences;
import android.content.pm.PackageManager;
import android.database.Cursor;
import android.graphics.Bitmap;
import android.graphics.BitmapFactory;
+import android.media.ExifInterface;
import android.net.Uri;
import android.os.Bundle;
+import android.os.Environment;
import android.os.Handler;
import android.os.HandlerThread;
import android.os.Message;
import android.preference.PreferenceManager;
import android.provider.MediaStore;
-import android.support.annotation.NonNull;
-import android.support.v4.app.ActivityCompat;
-import android.support.v4.content.ContextCompat;
-import android.support.v7.app.AppCompatActivity;
import android.text.method.ScrollingMovementMethod;
import android.util.Log;
import android.view.Menu;
@@ -29,9 +28,17 @@ import android.widget.ImageView;
import android.widget.TextView;
import android.widget.Toast;
+import androidx.annotation.NonNull;
+import androidx.appcompat.app.AppCompatActivity;
+import androidx.core.app.ActivityCompat;
+import androidx.core.content.ContextCompat;
+import androidx.core.content.FileProvider;
+
import java.io.File;
import java.io.IOException;
import java.io.InputStream;
+import java.text.SimpleDateFormat;
+import java.util.Date;
public class MainActivity extends AppCompatActivity {
private static final String TAG = MainActivity.class.getSimpleName();
@@ -69,6 +76,7 @@ public class MainActivity extends AppCompatActivity {
protected float[] inputMean = new float[]{};
protected float[] inputStd = new float[]{};
protected float scoreThreshold = 0.1f;
+ private String currentPhotoPath;
protected Predictor predictor = new Predictor();
@@ -368,18 +376,56 @@ public class MainActivity extends AppCompatActivity {
}
private void takePhoto() {
- Intent takePhotoIntent = new Intent(MediaStore.ACTION_IMAGE_CAPTURE);
- if (takePhotoIntent.resolveActivity(getPackageManager()) != null) {
- startActivityForResult(takePhotoIntent, TAKE_PHOTO_REQUEST_CODE);
+ Intent takePictureIntent = new Intent(MediaStore.ACTION_IMAGE_CAPTURE);
+ // Ensure that there's a camera activity to handle the intent
+ if (takePictureIntent.resolveActivity(getPackageManager()) != null) {
+ // Create the File where the photo should go
+ File photoFile = null;
+ try {
+ photoFile = createImageFile();
+ } catch (IOException ex) {
+ Log.e("MainActitity", ex.getMessage(), ex);
+ Toast.makeText(MainActivity.this,
+ "Create Camera temp file failed: " + ex.getMessage(), Toast.LENGTH_SHORT).show();
+ }
+ // Continue only if the File was successfully created
+ if (photoFile != null) {
+ Log.i(TAG, "FILEPATH " + getExternalFilesDir("Pictures").getAbsolutePath());
+ Uri photoURI = FileProvider.getUriForFile(this,
+ "com.baidu.paddle.lite.demo.ocr.fileprovider",
+ photoFile);
+ currentPhotoPath = photoFile.getAbsolutePath();
+ takePictureIntent.putExtra(MediaStore.EXTRA_OUTPUT, photoURI);
+ startActivityForResult(takePictureIntent, TAKE_PHOTO_REQUEST_CODE);
+ Log.i(TAG, "startActivityForResult finished");
+ }
}
+
+ }
+
+ private File createImageFile() throws IOException {
+ // Create an image file name
+ String timeStamp = new SimpleDateFormat("yyyyMMdd_HHmmss").format(new Date());
+ String imageFileName = "JPEG_" + timeStamp + "_";
+ File storageDir = getExternalFilesDir(Environment.DIRECTORY_PICTURES);
+ File image = File.createTempFile(
+ imageFileName, /* prefix */
+ ".bmp", /* suffix */
+ storageDir /* directory */
+ );
+
+ return image;
}
@Override
protected void onActivityResult(int requestCode, int resultCode, Intent data) {
super.onActivityResult(requestCode, resultCode, data);
- if (resultCode == RESULT_OK && data != null) {
+ if (resultCode == RESULT_OK) {
switch (requestCode) {
case OPEN_GALLERY_REQUEST_CODE:
+ if (data == null) {
+ break;
+ }
try {
ContentResolver resolver = getContentResolver();
Uri uri = data.getData();
@@ -393,9 +439,22 @@ public class MainActivity extends AppCompatActivity {
}
break;
case TAKE_PHOTO_REQUEST_CODE:
- Bundle extras = data.getExtras();
- Bitmap image = (Bitmap) extras.get("data");
- onImageChanged(image);
+ if (currentPhotoPath != null) {
+ ExifInterface exif = null;
+ try {
+ exif = new ExifInterface(currentPhotoPath);
+ } catch (IOException e) {
+ e.printStackTrace();
+ }
+ int orientation = exif.getAttributeInt(ExifInterface.TAG_ORIENTATION,
+ ExifInterface.ORIENTATION_UNDEFINED);
+ Log.i(TAG, "rotation " + orientation);
+ Bitmap image = BitmapFactory.decodeFile(currentPhotoPath);
+ image = Utils.rotateBitmap(image, orientation);
+ onImageChanged(image);
+ } else {
+ Log.e(TAG, "currentPhotoPath is null");
+ }
break;
default:
break;
diff --git a/deploy/android_demo/app/src/main/java/com/baidu/paddle/lite/demo/ocr/MiniActivity.java b/deploy/android_demo/app/src/main/java/com/baidu/paddle/lite/demo/ocr/MiniActivity.java
new file mode 100644
index 0000000000000000000000000000000000000000..d5608911db2e043657eb01b9e8e92fe9b79c99b7
--- /dev/null
+++ b/deploy/android_demo/app/src/main/java/com/baidu/paddle/lite/demo/ocr/MiniActivity.java
@@ -0,0 +1,157 @@
+package com.baidu.paddle.lite.demo.ocr;
+
+import android.graphics.Bitmap;
+import android.graphics.BitmapFactory;
+import android.os.Build;
+import android.os.Bundle;
+import android.os.Handler;
+import android.os.HandlerThread;
+import android.os.Message;
+import android.util.Log;
+import android.view.View;
+import android.widget.Button;
+import android.widget.ImageView;
+import android.widget.TextView;
+import android.widget.Toast;
+
+import androidx.appcompat.app.AppCompatActivity;
+
+import java.io.IOException;
+import java.io.InputStream;
+
+public class MiniActivity extends AppCompatActivity {
+
+
+ public static final int REQUEST_LOAD_MODEL = 0;
+ public static final int REQUEST_RUN_MODEL = 1;
+ public static final int REQUEST_UNLOAD_MODEL = 2;
+ public static final int RESPONSE_LOAD_MODEL_SUCCESSED = 0;
+ public static final int RESPONSE_LOAD_MODEL_FAILED = 1;
+ public static final int RESPONSE_RUN_MODEL_SUCCESSED = 2;
+ public static final int RESPONSE_RUN_MODEL_FAILED = 3;
+
+ private static final String TAG = "MiniActivity";
+
+ protected Handler receiver = null; // Receive messages from worker thread
+ protected Handler sender = null; // Send command to worker thread
+ protected HandlerThread worker = null; // Worker thread to load&run model
+ protected volatile Predictor predictor = null;
+
+ private String assetModelDirPath = "models/ocr_v1_for_cpu";
+ private String assetlabelFilePath = "labels/ppocr_keys_v1.txt";
+
+ private Button button;
+ private ImageView imageView; // image result
+ private TextView textView; // text result
+
+ @Override
+ protected void onCreate(Bundle savedInstanceState) {
+ super.onCreate(savedInstanceState);
+ setContentView(R.layout.activity_mini);
+
+ Log.i(TAG, "SHOW in Logcat");
+
+ // Prepare the worker thread for mode loading and inference
+ worker = new HandlerThread("Predictor Worker");
+ worker.start();
+ sender = new Handler(worker.getLooper()) {
+ public void handleMessage(Message msg) {
+ switch (msg.what) {
+ case REQUEST_LOAD_MODEL:
+ // Load model and reload test image
+ if (!onLoadModel()) {
+ runOnUiThread(new Runnable() {
+ @Override
+ public void run() {
+ Toast.makeText(MiniActivity.this, "Load model failed!", Toast.LENGTH_SHORT).show();
+ }
+ });
+ }
+ break;
+ case REQUEST_RUN_MODEL:
+ // Run model if model is loaded
+ final boolean isSuccessed = onRunModel();
+ runOnUiThread(new Runnable() {
+ @Override
+ public void run() {
+ if (isSuccessed){
+ onRunModelSuccessed();
+ }else{
+ Toast.makeText(MiniActivity.this, "Run model failed!", Toast.LENGTH_SHORT).show();
+ }
+ }
+ });
+ break;
+ }
+ }
+ };
+ sender.sendEmptyMessage(REQUEST_LOAD_MODEL); // corresponding to REQUEST_LOAD_MODEL, to call onLoadModel()
+
+ imageView = findViewById(R.id.imageView);
+ textView = findViewById(R.id.sample_text);
+ button = findViewById(R.id.button);
+ button.setOnClickListener(new View.OnClickListener() {
+ @Override
+ public void onClick(View v) {
+ sender.sendEmptyMessage(REQUEST_RUN_MODEL);
+ }
+ });
+
+
+ }
+
+ @Override
+ protected void onDestroy() {
+ onUnloadModel();
+ if (Build.VERSION.SDK_INT >= Build.VERSION_CODES.JELLY_BEAN_MR2) {
+ worker.quitSafely();
+ } else {
+ worker.quit();
+ }
+ super.onDestroy();
+ }
+
+ /**
+ * call in onCreate, model init
+ *
+ * @return
+ */
+ private boolean onLoadModel() {
+ if (predictor == null) {
+ predictor = new Predictor();
+ }
+ return predictor.init(this, assetModelDirPath, assetlabelFilePath);
+ }
+
+ /**
+ * init engine
+ * call in onCreate
+ *
+ * @return
+ */
+ private boolean onRunModel() {
+ try {
+ String assetImagePath = "images/5.jpg";
+ InputStream imageStream = getAssets().open(assetImagePath);
+ Bitmap image = BitmapFactory.decodeStream(imageStream);
+ // Input is Bitmap
+ predictor.setInputImage(image);
+ return predictor.isLoaded() && predictor.runModel();
+ } catch (IOException e) {
+ e.printStackTrace();
+ return false;
+ }
+ }
+
+ private void onRunModelSuccessed() {
+ Log.i(TAG, "onRunModelSuccessed");
+ textView.setText(predictor.outputResult);
+ imageView.setImageBitmap(predictor.outputImage);
+ }
+
+ private void onUnloadModel() {
+ if (predictor != null) {
+ predictor.releaseModel();
+ }
+ }
+}
diff --git a/deploy/android_demo/app/src/main/java/com/baidu/paddle/lite/demo/ocr/OCRPredictorNative.java b/deploy/android_demo/app/src/main/java/com/baidu/paddle/lite/demo/ocr/OCRPredictorNative.java
index 103d5d37aec3ddc026d48a202df17b140e3e4533..7499d4b92689645c0b1009256884733d392ff68d 100644
--- a/deploy/android_demo/app/src/main/java/com/baidu/paddle/lite/demo/ocr/OCRPredictorNative.java
+++ b/deploy/android_demo/app/src/main/java/com/baidu/paddle/lite/demo/ocr/OCRPredictorNative.java
@@ -29,16 +29,16 @@ public class OCRPredictorNative {
public OCRPredictorNative(Config config) {
this.config = config;
loadLibrary();
- nativePointer = init(config.detModelFilename, config.recModelFilename,
+ nativePointer = init(config.detModelFilename, config.recModelFilename,config.clsModelFilename,
config.cpuThreadNum, config.cpuPower);
Log.i("OCRPredictorNative", "load success " + nativePointer);
}
- public void release(){
- if (nativePointer != 0){
+ public void release() {
+ if (nativePointer != 0) {
nativePointer = 0;
- destory(nativePointer);
+// destory(nativePointer);
}
}
@@ -55,10 +55,11 @@ public class OCRPredictorNative {
public String cpuPower;
public String detModelFilename;
public String recModelFilename;
+ public String clsModelFilename;
}
- protected native long init(String detModelPath, String recModelPath, int threadNum, String cpuMode);
+ protected native long init(String detModelPath, String recModelPath,String clsModelPath, int threadNum, String cpuMode);
protected native float[] forward(long pointer, float[] buf, float[] ddims, Bitmap originalImage);
diff --git a/deploy/android_demo/app/src/main/java/com/baidu/paddle/lite/demo/ocr/Predictor.java b/deploy/android_demo/app/src/main/java/com/baidu/paddle/lite/demo/ocr/Predictor.java
index d491481e7b61bca8043c34a65ecb3bbf6a72487d..ddf69ab481618696189a7d0d45264791267e5631 100644
--- a/deploy/android_demo/app/src/main/java/com/baidu/paddle/lite/demo/ocr/Predictor.java
+++ b/deploy/android_demo/app/src/main/java/com/baidu/paddle/lite/demo/ocr/Predictor.java
@@ -38,7 +38,7 @@ public class Predictor {
protected float scoreThreshold = 0.1f;
protected Bitmap inputImage = null;
protected Bitmap outputImage = null;
- protected String outputResult = "";
+ protected volatile String outputResult = "";
protected float preprocessTime = 0;
protected float postprocessTime = 0;
@@ -46,6 +46,16 @@ public class Predictor {
public Predictor() {
}
+ public boolean init(Context appCtx, String modelPath, String labelPath) {
+ isLoaded = loadModel(appCtx, modelPath, cpuThreadNum, cpuPowerMode);
+ if (!isLoaded) {
+ return false;
+ }
+ isLoaded = loadLabel(appCtx, labelPath);
+ return isLoaded;
+ }
+
+
public boolean init(Context appCtx, String modelPath, String labelPath, int cpuThreadNum, String cpuPowerMode,
String inputColorFormat,
long[] inputShape, float[] inputMean,
@@ -76,11 +86,7 @@ public class Predictor {
Log.e(TAG, "Only BGR color format is supported.");
return false;
}
- isLoaded = loadModel(appCtx, modelPath, cpuThreadNum, cpuPowerMode);
- if (!isLoaded) {
- return false;
- }
- isLoaded = loadLabel(appCtx, labelPath);
+ boolean isLoaded = init(appCtx, modelPath, labelPath);
if (!isLoaded) {
return false;
}
@@ -115,7 +121,8 @@ public class Predictor {
config.cpuThreadNum = cpuThreadNum;
config.detModelFilename = realPath + File.separator + "ch_det_mv3_db_opt.nb";
config.recModelFilename = realPath + File.separator + "ch_rec_mv3_crnn_opt.nb";
- Log.e("Predictor", "model path" + config.detModelFilename + " ; " + config.recModelFilename);
+ config.clsModelFilename = realPath + File.separator + "cls_opt_arm.nb";
+ Log.e("Predictor", "model path" + config.detModelFilename + " ; " + config.recModelFilename + ";" + config.clsModelFilename);
config.cpuPower = cpuPowerMode;
paddlePredictor = new OCRPredictorNative(config);
@@ -127,12 +134,12 @@ public class Predictor {
}
public void releaseModel() {
- if (paddlePredictor != null){
+ if (paddlePredictor != null) {
paddlePredictor.release();
paddlePredictor = null;
}
isLoaded = false;
- cpuThreadNum = 4;
+ cpuThreadNum = 1;
cpuPowerMode = "LITE_POWER_HIGH";
modelPath = "";
modelName = "";
@@ -222,7 +229,7 @@ public class Predictor {
for (int i = 0; i < warmupIterNum; i++) {
paddlePredictor.runImage(inputData, width, height, channels, inputImage);
}
- warmupIterNum = 0; // 之后不要再warm了
+ warmupIterNum = 0; // do not need warm
// Run inference
start = new Date();
ArrayList results = paddlePredictor.runImage(inputData, width, height, channels, inputImage);
@@ -287,9 +294,7 @@ public class Predictor {
if (image == null) {
return;
}
- // Scale image to the size of input tensor
- Bitmap rgbaImage = image.copy(Bitmap.Config.ARGB_8888, true);
- this.inputImage = rgbaImage;
+ this.inputImage = image.copy(Bitmap.Config.ARGB_8888, true);
}
private ArrayList postprocess(ArrayList results) {
@@ -310,7 +315,7 @@ public class Predictor {
private void drawResults(ArrayList results) {
StringBuffer outputResultSb = new StringBuffer("");
- for (int i=0;i
-
-
\ No newline at end of file
+
\ No newline at end of file
diff --git a/deploy/android_demo/app/src/main/res/layout/activity_mini.xml b/deploy/android_demo/app/src/main/res/layout/activity_mini.xml
new file mode 100644
index 0000000000000000000000000000000000000000..ec4622ae5c21334769a0ef7f084f73a3ac6a05ab
--- /dev/null
+++ b/deploy/android_demo/app/src/main/res/layout/activity_mini.xml
@@ -0,0 +1,46 @@
+
+
+
+
+
+
+
+
+
+
+
\ No newline at end of file
diff --git a/deploy/android_demo/app/src/main/res/xml/file_paths.xml b/deploy/android_demo/app/src/main/res/xml/file_paths.xml
new file mode 100644
index 0000000000000000000000000000000000000000..3d985443b3357dcf39c5631d3021f08000e047c7
--- /dev/null
+++ b/deploy/android_demo/app/src/main/res/xml/file_paths.xml
@@ -0,0 +1,4 @@
+
+
+
+
\ No newline at end of file
diff --git a/deploy/android_demo/gradle/wrapper/gradle-wrapper.properties b/deploy/android_demo/gradle/wrapper/gradle-wrapper.properties
index 578b5482ad45045124272fa3e54d065a77c2eea2..63dac4e990eb3ca985e9c1018c84f26fbab0ac78 100644
--- a/deploy/android_demo/gradle/wrapper/gradle-wrapper.properties
+++ b/deploy/android_demo/gradle/wrapper/gradle-wrapper.properties
@@ -1,4 +1,4 @@
-#Thu Aug 22 15:05:37 CST 2019
+#Wed Jul 22 23:48:44 CST 2020
distributionBase=GRADLE_USER_HOME
distributionPath=wrapper/dists
zipStoreBase=GRADLE_USER_HOME
diff --git a/deploy/cpp_infer/CMakeLists.txt b/deploy/cpp_infer/CMakeLists.txt
index 1415e2cb89e1d42cda4d5ee15963f513e728a0cb..466c2be8f79c11a9e6cf39631ef2dc5a2a213321 100644
--- a/deploy/cpp_infer/CMakeLists.txt
+++ b/deploy/cpp_infer/CMakeLists.txt
@@ -1,8 +1,17 @@
project(ocr_system CXX C)
+
option(WITH_MKL "Compile demo with MKL/OpenBlas support, default use MKL." ON)
option(WITH_GPU "Compile demo with GPU/CPU, default use CPU." OFF)
option(WITH_STATIC_LIB "Compile demo with static/shared library, default use static." ON)
-option(USE_TENSORRT "Compile demo with TensorRT." OFF)
+option(WITH_TENSORRT "Compile demo with TensorRT." OFF)
+
+SET(PADDLE_LIB "" CACHE PATH "Location of libraries")
+SET(OPENCV_DIR "" CACHE PATH "Location of libraries")
+SET(CUDA_LIB "" CACHE PATH "Location of libraries")
+SET(CUDNN_LIB "" CACHE PATH "Location of libraries")
+SET(TENSORRT_DIR "" CACHE PATH "Compile demo with TensorRT")
+
+set(DEMO_NAME "ocr_system")
macro(safe_set_static_flag)
@@ -15,24 +24,60 @@ macro(safe_set_static_flag)
endforeach(flag_var)
endmacro()
-set(CMAKE_CXX_FLAGS "${CMAKE_CXX_FLAGS} -std=c++11 -g -fpermissive")
-set(CMAKE_STATIC_LIBRARY_PREFIX "")
-message("flags" ${CMAKE_CXX_FLAGS})
-set(CMAKE_CXX_FLAGS_RELEASE "-O3")
+if (WITH_MKL)
+ ADD_DEFINITIONS(-DUSE_MKL)
+endif()
if(NOT DEFINED PADDLE_LIB)
message(FATAL_ERROR "please set PADDLE_LIB with -DPADDLE_LIB=/path/paddle/lib")
endif()
-if(NOT DEFINED DEMO_NAME)
- message(FATAL_ERROR "please set DEMO_NAME with -DDEMO_NAME=demo_name")
+
+if(NOT DEFINED OPENCV_DIR)
+ message(FATAL_ERROR "please set OPENCV_DIR with -DOPENCV_DIR=/path/opencv")
endif()
-set(OPENCV_DIR ${OPENCV_DIR})
-find_package(OpenCV REQUIRED PATHS ${OPENCV_DIR}/share/OpenCV NO_DEFAULT_PATH)
+if (WIN32)
+ include_directories("${PADDLE_LIB}/paddle/fluid/inference")
+ include_directories("${PADDLE_LIB}/paddle/include")
+ link_directories("${PADDLE_LIB}/paddle/fluid/inference")
+ find_package(OpenCV REQUIRED PATHS ${OPENCV_DIR}/build/ NO_DEFAULT_PATH)
+
+else ()
+ find_package(OpenCV REQUIRED PATHS ${OPENCV_DIR}/share/OpenCV NO_DEFAULT_PATH)
+ include_directories("${PADDLE_LIB}/paddle/include")
+ link_directories("${PADDLE_LIB}/paddle/lib")
+endif ()
include_directories(${OpenCV_INCLUDE_DIRS})
-include_directories("${PADDLE_LIB}/paddle/include")
+if (WIN32)
+ add_definitions("/DGOOGLE_GLOG_DLL_DECL=")
+ set(CMAKE_C_FLAGS_DEBUG "${CMAKE_C_FLAGS_DEBUG} /bigobj /MTd")
+ set(CMAKE_C_FLAGS_RELEASE "${CMAKE_C_FLAGS_RELEASE} /bigobj /MT")
+ set(CMAKE_CXX_FLAGS_DEBUG "${CMAKE_CXX_FLAGS_DEBUG} /bigobj /MTd")
+ set(CMAKE_CXX_FLAGS_RELEASE "${CMAKE_CXX_FLAGS_RELEASE} /bigobj /MT")
+ if (WITH_STATIC_LIB)
+ safe_set_static_flag()
+ add_definitions(-DSTATIC_LIB)
+ endif()
+else()
+ set(CMAKE_CXX_FLAGS "${CMAKE_CXX_FLAGS} -g -o3 -std=c++11")
+ set(CMAKE_STATIC_LIBRARY_PREFIX "")
+endif()
+message("flags" ${CMAKE_CXX_FLAGS})
+
+
+if (WITH_GPU)
+ if (NOT DEFINED CUDA_LIB OR ${CUDA_LIB} STREQUAL "")
+ message(FATAL_ERROR "please set CUDA_LIB with -DCUDA_LIB=/path/cuda-8.0/lib64")
+ endif()
+ if (NOT WIN32)
+ if (NOT DEFINED CUDNN_LIB)
+ message(FATAL_ERROR "please set CUDNN_LIB with -DCUDNN_LIB=/path/cudnn_v7.4/cuda/lib64")
+ endif()
+ endif(NOT WIN32)
+endif()
+
include_directories("${PADDLE_LIB}/third_party/install/protobuf/include")
include_directories("${PADDLE_LIB}/third_party/install/glog/include")
include_directories("${PADDLE_LIB}/third_party/install/gflags/include")
@@ -43,10 +88,12 @@ include_directories("${PADDLE_LIB}/third_party/eigen3")
include_directories("${CMAKE_SOURCE_DIR}/")
-if (USE_TENSORRT AND WITH_GPU)
- include_directories("${TENSORRT_ROOT}/include")
- link_directories("${TENSORRT_ROOT}/lib")
-endif()
+if (NOT WIN32)
+ if (WITH_TENSORRT AND WITH_GPU)
+ include_directories("${TENSORRT_DIR}/include")
+ link_directories("${TENSORRT_DIR}/lib")
+ endif()
+endif(NOT WIN32)
link_directories("${PADDLE_LIB}/third_party/install/zlib/lib")
@@ -57,17 +104,24 @@ link_directories("${PADDLE_LIB}/third_party/install/xxhash/lib")
link_directories("${PADDLE_LIB}/paddle/lib")
-AUX_SOURCE_DIRECTORY(./src SRCS)
-add_executable(${DEMO_NAME} ${SRCS})
-
if(WITH_MKL)
include_directories("${PADDLE_LIB}/third_party/install/mklml/include")
- set(MATH_LIB ${PADDLE_LIB}/third_party/install/mklml/lib/libmklml_intel${CMAKE_SHARED_LIBRARY_SUFFIX}
- ${PADDLE_LIB}/third_party/install/mklml/lib/libiomp5${CMAKE_SHARED_LIBRARY_SUFFIX})
+ if (WIN32)
+ set(MATH_LIB ${PADDLE_LIB}/third_party/install/mklml/lib/mklml.lib
+ ${PADDLE_LIB}/third_party/install/mklml/lib/libiomp5md.lib)
+ else ()
+ set(MATH_LIB ${PADDLE_LIB}/third_party/install/mklml/lib/libmklml_intel${CMAKE_SHARED_LIBRARY_SUFFIX}
+ ${PADDLE_LIB}/third_party/install/mklml/lib/libiomp5${CMAKE_SHARED_LIBRARY_SUFFIX})
+ execute_process(COMMAND cp -r ${PADDLE_LIB}/third_party/install/mklml/lib/libmklml_intel${CMAKE_SHARED_LIBRARY_SUFFIX} /usr/lib)
+ endif ()
set(MKLDNN_PATH "${PADDLE_LIB}/third_party/install/mkldnn")
if(EXISTS ${MKLDNN_PATH})
include_directories("${MKLDNN_PATH}/include")
- set(MKLDNN_LIB ${MKLDNN_PATH}/lib/libmkldnn.so.0)
+ if (WIN32)
+ set(MKLDNN_LIB ${MKLDNN_PATH}/lib/mkldnn.lib)
+ else ()
+ set(MKLDNN_LIB ${MKLDNN_PATH}/lib/libmkldnn.so.0)
+ endif ()
endif()
else()
set(MATH_LIB ${PADDLE_LIB}/third_party/install/openblas/lib/libopenblas${CMAKE_STATIC_LIBRARY_SUFFIX})
@@ -82,24 +136,66 @@ else()
${PADDLE_LIB}/paddle/lib/libpaddle_fluid${CMAKE_SHARED_LIBRARY_SUFFIX})
endif()
-set(EXTERNAL_LIB "-lrt -ldl -lpthread -lm")
+if (NOT WIN32)
+ set(DEPS ${DEPS}
+ ${MATH_LIB} ${MKLDNN_LIB}
+ glog gflags protobuf z xxhash
+ )
+ if(EXISTS "${PADDLE_LIB}/third_party/install/snappystream/lib")
+ set(DEPS ${DEPS} snappystream)
+ endif()
+ if (EXISTS "${PADDLE_LIB}/third_party/install/snappy/lib")
+ set(DEPS ${DEPS} snappy)
+ endif()
+else()
+ set(DEPS ${DEPS}
+ ${MATH_LIB} ${MKLDNN_LIB}
+ glog gflags_static libprotobuf xxhash)
+ set(DEPS ${DEPS} libcmt shlwapi)
+ if (EXISTS "${PADDLE_LIB}/third_party/install/snappy/lib")
+ set(DEPS ${DEPS} snappy)
+ endif()
+ if(EXISTS "${PADDLE_LIB}/third_party/install/snappystream/lib")
+ set(DEPS ${DEPS} snappystream)
+ endif()
+endif(NOT WIN32)
-set(DEPS ${DEPS}
- ${MATH_LIB} ${MKLDNN_LIB}
- glog gflags protobuf z xxhash
- ${EXTERNAL_LIB} ${OpenCV_LIBS})
if(WITH_GPU)
- if (USE_TENSORRT)
- set(DEPS ${DEPS}
- ${TENSORRT_ROOT}/lib/libnvinfer${CMAKE_SHARED_LIBRARY_SUFFIX})
- set(DEPS ${DEPS}
- ${TENSORRT_ROOT}/lib/libnvinfer_plugin${CMAKE_SHARED_LIBRARY_SUFFIX})
+ if(NOT WIN32)
+ if (WITH_TENSORRT)
+ set(DEPS ${DEPS} ${TENSORRT_DIR}/lib/libnvinfer${CMAKE_SHARED_LIBRARY_SUFFIX})
+ set(DEPS ${DEPS} ${TENSORRT_DIR}/lib/libnvinfer_plugin${CMAKE_SHARED_LIBRARY_SUFFIX})
+ endif()
+ set(DEPS ${DEPS} ${CUDA_LIB}/libcudart${CMAKE_SHARED_LIBRARY_SUFFIX})
+ set(DEPS ${DEPS} ${CUDNN_LIB}/libcudnn${CMAKE_SHARED_LIBRARY_SUFFIX})
+ else()
+ set(DEPS ${DEPS} ${CUDA_LIB}/cudart${CMAKE_STATIC_LIBRARY_SUFFIX} )
+ set(DEPS ${DEPS} ${CUDA_LIB}/cublas${CMAKE_STATIC_LIBRARY_SUFFIX} )
+ set(DEPS ${DEPS} ${CUDNN_LIB}/cudnn${CMAKE_STATIC_LIBRARY_SUFFIX})
endif()
- set(DEPS ${DEPS} ${CUDA_LIB}/libcudart${CMAKE_SHARED_LIBRARY_SUFFIX})
- set(DEPS ${DEPS} ${CUDA_LIB}/libcudart${CMAKE_SHARED_LIBRARY_SUFFIX} )
- set(DEPS ${DEPS} ${CUDA_LIB}/libcublas${CMAKE_SHARED_LIBRARY_SUFFIX} )
- set(DEPS ${DEPS} ${CUDNN_LIB}/libcudnn${CMAKE_SHARED_LIBRARY_SUFFIX} )
endif()
+
+if (NOT WIN32)
+ set(EXTERNAL_LIB "-ldl -lrt -lgomp -lz -lm -lpthread")
+ set(DEPS ${DEPS} ${EXTERNAL_LIB})
+endif()
+
+set(DEPS ${DEPS} ${OpenCV_LIBS})
+
+AUX_SOURCE_DIRECTORY(./src SRCS)
+add_executable(${DEMO_NAME} ${SRCS})
+
target_link_libraries(${DEMO_NAME} ${DEPS})
+
+if (WIN32 AND WITH_MKL)
+ add_custom_command(TARGET ${DEMO_NAME} POST_BUILD
+ COMMAND ${CMAKE_COMMAND} -E copy_if_different ${PADDLE_LIB}/third_party/install/mklml/lib/mklml.dll ./mklml.dll
+ COMMAND ${CMAKE_COMMAND} -E copy_if_different ${PADDLE_LIB}/third_party/install/mklml/lib/libiomp5md.dll ./libiomp5md.dll
+ COMMAND ${CMAKE_COMMAND} -E copy_if_different ${PADDLE_LIB}/third_party/install/mkldnn/lib/mkldnn.dll ./mkldnn.dll
+ COMMAND ${CMAKE_COMMAND} -E copy_if_different ${PADDLE_LIB}/third_party/install/mklml/lib/mklml.dll ./release/mklml.dll
+ COMMAND ${CMAKE_COMMAND} -E copy_if_different ${PADDLE_LIB}/third_party/install/mklml/lib/libiomp5md.dll ./release/libiomp5md.dll
+ COMMAND ${CMAKE_COMMAND} -E copy_if_different ${PADDLE_LIB}/third_party/install/mkldnn/lib/mkldnn.dll ./release/mkldnn.dll
+ )
+endif()
\ No newline at end of file
diff --git a/deploy/cpp_infer/docs/windows_vs2019_build.md b/deploy/cpp_infer/docs/windows_vs2019_build.md
new file mode 100644
index 0000000000000000000000000000000000000000..21fbf4e0eb95ee82475164047d8051e90e9e224f
--- /dev/null
+++ b/deploy/cpp_infer/docs/windows_vs2019_build.md
@@ -0,0 +1,95 @@
+# Visual Studio 2019 Community CMake 编译指南
+
+PaddleOCR在Windows 平台下基于`Visual Studio 2019 Community` 进行了测试。微软从`Visual Studio 2017`开始即支持直接管理`CMake`跨平台编译项目,但是直到`2019`才提供了稳定和完全的支持,所以如果你想使用CMake管理项目编译构建,我们推荐你使用`Visual Studio 2019`环境下构建。
+
+
+## 前置条件
+* Visual Studio 2019
+* CUDA 9.0 / CUDA 10.0,cudnn 7+ (仅在使用GPU版本的预测库时需要)
+* CMake 3.0+
+
+请确保系统已经安装好上述基本软件,我们使用的是`VS2019`的社区版。
+
+**下面所有示例以工作目录为 `D:\projects`演示**。
+
+### Step1: 下载PaddlePaddle C++ 预测库 fluid_inference
+
+PaddlePaddle C++ 预测库针对不同的`CPU`和`CUDA`版本提供了不同的预编译版本,请根据实际情况下载: [C++预测库下载列表](https://www.paddlepaddle.org.cn/documentation/docs/zh/develop/advanced_guide/inference_deployment/inference/windows_cpp_inference.html)
+
+解压后`D:\projects\fluid_inference`目录包含内容为:
+```
+fluid_inference
+├── paddle # paddle核心库和头文件
+|
+├── third_party # 第三方依赖库和头文件
+|
+└── version.txt # 版本和编译信息
+```
+
+### Step2: 安装配置OpenCV
+
+1. 在OpenCV官网下载适用于Windows平台的3.4.6版本, [下载地址](https://sourceforge.net/projects/opencvlibrary/files/3.4.6/opencv-3.4.6-vc14_vc15.exe/download)
+2. 运行下载的可执行文件,将OpenCV解压至指定目录,如`D:\projects\opencv`
+3. 配置环境变量,如下流程所示
+ - 我的电脑->属性->高级系统设置->环境变量
+ - 在系统变量中找到Path(如没有,自行创建),并双击编辑
+ - 新建,将opencv路径填入并保存,如`D:\projects\opencv\build\x64\vc14\bin`
+
+### Step3: 使用Visual Studio 2019直接编译CMake
+
+1. 打开Visual Studio 2019 Community,点击`继续但无需代码`
+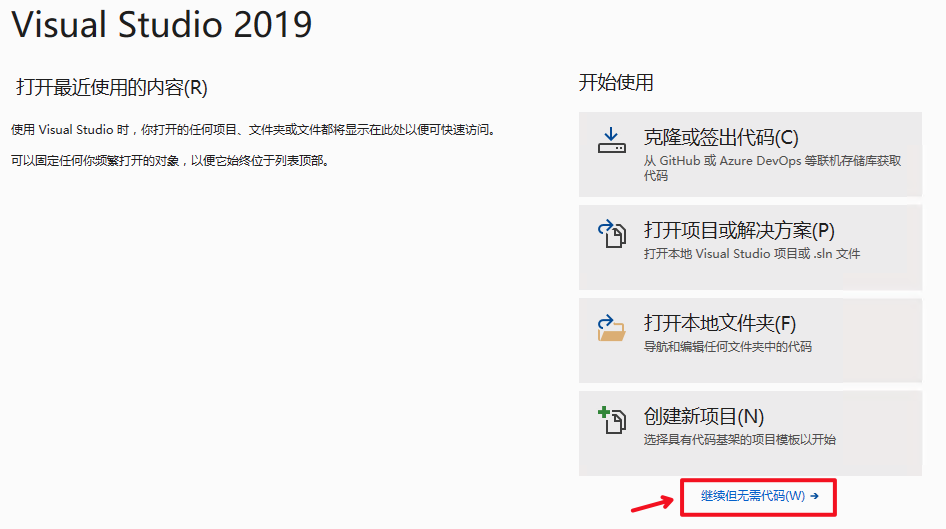
+2. 点击: `文件`->`打开`->`CMake`
+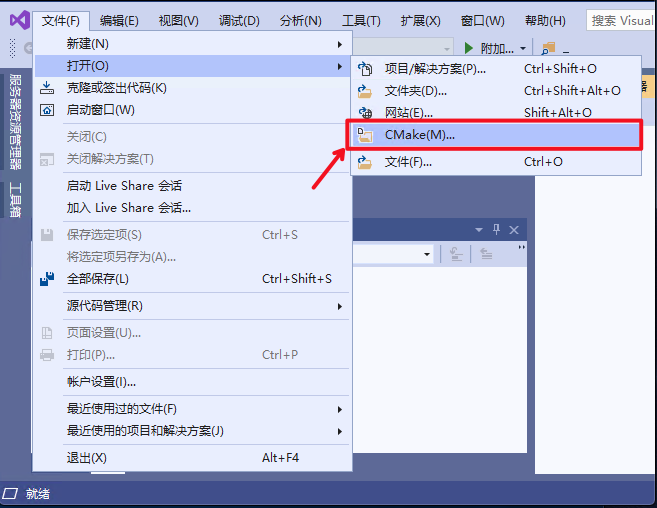
+
+选择项目代码所在路径,并打开`CMakeList.txt`:
+
+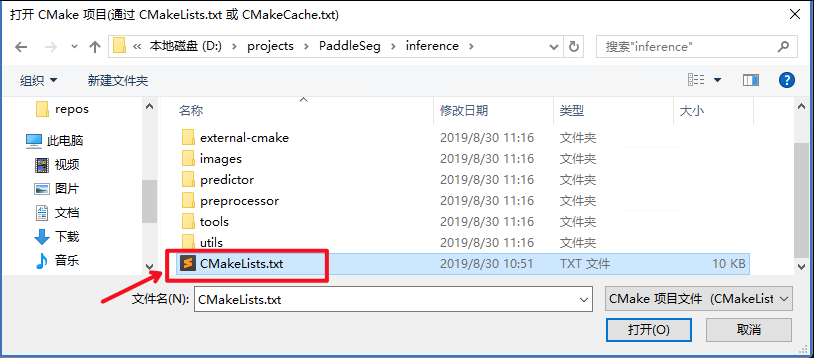
+
+3. 点击:`项目`->`cpp_inference_demo的CMake设置`
+
+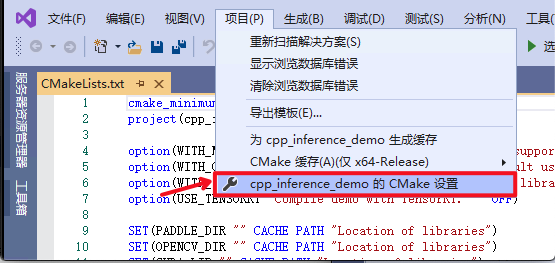
+
+4. 点击`浏览`,分别设置编译选项指定`CUDA`、`CUDNN_LIB`、`OpenCV`、`Paddle预测库`的路径
+
+三个编译参数的含义说明如下(带`*`表示仅在使用**GPU版本**预测库时指定, 其中CUDA库版本尽量对齐,**使用9.0、10.0版本,不使用9.2、10.1等版本CUDA库**):
+
+| 参数名 | 含义 |
+| ---- | ---- |
+| *CUDA_LIB | CUDA的库路径 |
+| *CUDNN_LIB | CUDNN的库路径 |
+| OPENCV_DIR | OpenCV的安装路径 |
+| PADDLE_LIB | Paddle预测库的路径 |
+
+**注意:**
+ 1. 使用`CPU`版预测库,请把`WITH_GPU`的勾去掉
+ 2. 如果使用的是`openblas`版本,请把`WITH_MKL`勾去掉
+
+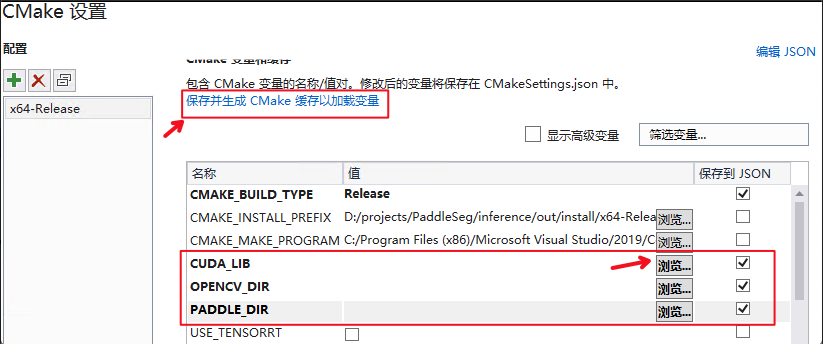
+
+**设置完成后**, 点击上图中`保存并生成CMake缓存以加载变量`。
+
+5. 点击`生成`->`全部生成`
+
+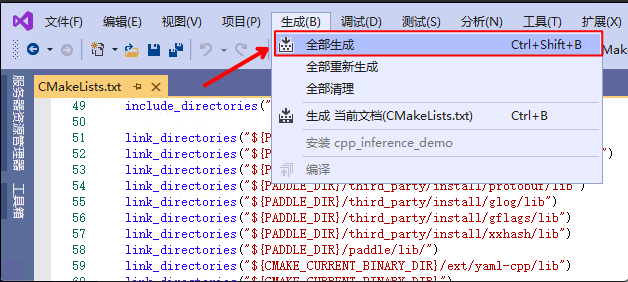
+
+
+### Step4: 预测及可视化
+
+上述`Visual Studio 2019`编译产出的可执行文件在`out\build\x64-Release`目录下,打开`cmd`,并切换到该目录:
+
+```
+cd D:\projects\PaddleOCR\deploy\cpp_infer\out\build\x64-Release
+```
+可执行文件`ocr_system.exe`即为样例的预测程序,其主要使用方法如下
+
+```shell
+#预测图片 `D:\projects\PaddleOCR\doc\imgs\10.jpg`
+.\ocr_system.exe D:\projects\PaddleOCR\deploy\cpp_infer\tools\config.txt D:\projects\PaddleOCR\doc\imgs\10.jpg
+```
+
+第一个参数为配置文件路径,第二个参数为需要预测的图片路径。
+
+
+### 注意
+* 在Windows下的终端中执行文件exe时,可能会发生乱码的现象,此时需要在终端中输入`CHCP 65001`,将终端的编码方式由GBK编码(默认)改为UTF-8编码,更加具体的解释可以参考这篇博客:[https://blog.csdn.net/qq_35038153/article/details/78430359](https://blog.csdn.net/qq_35038153/article/details/78430359)。
diff --git a/deploy/cpp_infer/include/config.h b/deploy/cpp_infer/include/config.h
index 2adefb73675b3607b3dd993f48517e51e9a3d941..27539ea7934dc192e86bca3ea6bfd7999ee229a3 100644
--- a/deploy/cpp_infer/include/config.h
+++ b/deploy/cpp_infer/include/config.h
@@ -41,13 +41,15 @@ public:
this->use_mkldnn = bool(stoi(config_map_["use_mkldnn"]));
+ this->use_zero_copy_run = bool(stoi(config_map_["use_zero_copy_run"]));
+
this->max_side_len = stoi(config_map_["max_side_len"]);
this->det_db_thresh = stod(config_map_["det_db_thresh"]);
this->det_db_box_thresh = stod(config_map_["det_db_box_thresh"]);
- this->det_db_box_thresh = stod(config_map_["det_db_box_thresh"]);
+ this->det_db_unclip_ratio = stod(config_map_["det_db_unclip_ratio"]);
this->det_model_dir.assign(config_map_["det_model_dir"]);
@@ -55,6 +57,12 @@ public:
this->char_list_file.assign(config_map_["char_list_file"]);
+ this->use_angle_cls = bool(stoi(config_map_["use_angle_cls"]));
+
+ this->cls_model_dir.assign(config_map_["cls_model_dir"]);
+
+ this->cls_thresh = stod(config_map_["cls_thresh"]);
+
this->visualize = bool(stoi(config_map_["visualize"]));
}
@@ -68,6 +76,8 @@ public:
bool use_mkldnn = false;
+ bool use_zero_copy_run = false;
+
int max_side_len = 960;
double det_db_thresh = 0.3;
@@ -80,8 +90,14 @@ public:
std::string rec_model_dir;
+ bool use_angle_cls;
+
std::string char_list_file;
+ std::string cls_model_dir;
+
+ double cls_thresh;
+
bool visualize = true;
void PrintConfigInfo();
diff --git a/deploy/cpp_infer/include/ocr_cls.h b/deploy/cpp_infer/include/ocr_cls.h
new file mode 100644
index 0000000000000000000000000000000000000000..38a37cff3c035eafe3617d83b2cc15ca47f30186
--- /dev/null
+++ b/deploy/cpp_infer/include/ocr_cls.h
@@ -0,0 +1,81 @@
+// Copyright (c) 2020 PaddlePaddle Authors. All Rights Reserved.
+//
+// Licensed under the Apache License, Version 2.0 (the "License");
+// you may not use this file except in compliance with the License.
+// You may obtain a copy of the License at
+//
+// http://www.apache.org/licenses/LICENSE-2.0
+//
+// Unless required by applicable law or agreed to in writing, software
+// distributed under the License is distributed on an "AS IS" BASIS,
+// WITHOUT WARRANTIES OR CONDITIONS OF ANY KIND, either express or implied.
+// See the License for the specific language governing permissions and
+// limitations under the License.
+
+#include "opencv2/core.hpp"
+#include "opencv2/imgcodecs.hpp"
+#include "opencv2/imgproc.hpp"
+#include "paddle_api.h"
+#include "paddle_inference_api.h"
+#include
+#include
+#include
+#include
+#include
+
+#include
+#include
+#include
+
+#include
+#include
+
+namespace PaddleOCR {
+
+class Classifier {
+public:
+ explicit Classifier(const std::string &model_dir, const bool &use_gpu,
+ const int &gpu_id, const int &gpu_mem,
+ const int &cpu_math_library_num_threads,
+ const bool &use_mkldnn, const bool &use_zero_copy_run,
+ const double &cls_thresh) {
+ this->use_gpu_ = use_gpu;
+ this->gpu_id_ = gpu_id;
+ this->gpu_mem_ = gpu_mem;
+ this->cpu_math_library_num_threads_ = cpu_math_library_num_threads;
+ this->use_mkldnn_ = use_mkldnn;
+ this->use_zero_copy_run_ = use_zero_copy_run;
+
+ this->cls_thresh = cls_thresh;
+
+ LoadModel(model_dir);
+ }
+
+ // Load Paddle inference model
+ void LoadModel(const std::string &model_dir);
+
+ cv::Mat Run(cv::Mat &img);
+
+private:
+ std::shared_ptr predictor_;
+
+ bool use_gpu_ = false;
+ int gpu_id_ = 0;
+ int gpu_mem_ = 4000;
+ int cpu_math_library_num_threads_ = 4;
+ bool use_mkldnn_ = false;
+ bool use_zero_copy_run_ = false;
+ double cls_thresh = 0.5;
+
+ std::vector mean_ = {0.5f, 0.5f, 0.5f};
+ std::vector scale_ = {1 / 0.5f, 1 / 0.5f, 1 / 0.5f};
+ bool is_scale_ = true;
+
+ // pre-process
+ ClsResizeImg resize_op_;
+ Normalize normalize_op_;
+ Permute permute_op_;
+
+}; // class Classifier
+
+} // namespace PaddleOCR
diff --git a/deploy/cpp_infer/include/ocr_det.h b/deploy/cpp_infer/include/ocr_det.h
index ed2667eecfea9a09d7da77df37f43a7b9e9bb349..0308d07f3bac67a275452500184e0959b16e8003 100644
--- a/deploy/cpp_infer/include/ocr_det.h
+++ b/deploy/cpp_infer/include/ocr_det.h
@@ -39,8 +39,8 @@ public:
explicit DBDetector(const std::string &model_dir, const bool &use_gpu,
const int &gpu_id, const int &gpu_mem,
const int &cpu_math_library_num_threads,
- const bool &use_mkldnn, const int &max_side_len,
- const double &det_db_thresh,
+ const bool &use_mkldnn, const bool &use_zero_copy_run,
+ const int &max_side_len, const double &det_db_thresh,
const double &det_db_box_thresh,
const double &det_db_unclip_ratio,
const bool &visualize) {
@@ -49,6 +49,7 @@ public:
this->gpu_mem_ = gpu_mem;
this->cpu_math_library_num_threads_ = cpu_math_library_num_threads;
this->use_mkldnn_ = use_mkldnn;
+ this->use_zero_copy_run_ = use_zero_copy_run;
this->max_side_len_ = max_side_len;
@@ -75,6 +76,7 @@ private:
int gpu_mem_ = 4000;
int cpu_math_library_num_threads_ = 4;
bool use_mkldnn_ = false;
+ bool use_zero_copy_run_ = false;
int max_side_len_ = 960;
diff --git a/deploy/cpp_infer/include/ocr_rec.h b/deploy/cpp_infer/include/ocr_rec.h
index 18b31b6b16dc7b45f7ea3622feffdcc33188bda9..a8b99a5960ac3e6238dfea2285ec51c9e80e1749 100644
--- a/deploy/cpp_infer/include/ocr_rec.h
+++ b/deploy/cpp_infer/include/ocr_rec.h
@@ -27,6 +27,7 @@
#include
#include
+#include
#include
#include
#include
@@ -38,14 +39,17 @@ public:
explicit CRNNRecognizer(const std::string &model_dir, const bool &use_gpu,
const int &gpu_id, const int &gpu_mem,
const int &cpu_math_library_num_threads,
- const bool &use_mkldnn, const string &label_path) {
+ const bool &use_mkldnn, const bool &use_zero_copy_run,
+ const string &label_path) {
this->use_gpu_ = use_gpu;
this->gpu_id_ = gpu_id;
this->gpu_mem_ = gpu_mem;
this->cpu_math_library_num_threads_ = cpu_math_library_num_threads;
this->use_mkldnn_ = use_mkldnn;
+ this->use_zero_copy_run_ = use_zero_copy_run;
this->label_list_ = Utility::ReadDict(label_path);
+ this->label_list_.push_back(" ");
LoadModel(model_dir);
}
@@ -53,7 +57,8 @@ public:
// Load Paddle inference model
void LoadModel(const std::string &model_dir);
- void Run(std::vector>> boxes, cv::Mat &img);
+ void Run(std::vector>> boxes, cv::Mat &img,
+ Classifier *cls);
private:
std::shared_ptr predictor_;
@@ -63,6 +68,7 @@ private:
int gpu_mem_ = 4000;
int cpu_math_library_num_threads_ = 4;
bool use_mkldnn_ = false;
+ bool use_zero_copy_run_ = false;
std::vector label_list_;
@@ -83,4 +89,4 @@ private:
}; // class CrnnRecognizer
-} // namespace PaddleOCR
\ No newline at end of file
+} // namespace PaddleOCR
diff --git a/deploy/cpp_infer/include/preprocess_op.h b/deploy/cpp_infer/include/preprocess_op.h
index 309d7fd4386330149afc91b474c330212fadd5e8..5cbc5cd7134238c4f09f536ca6b1153d2d703023 100644
--- a/deploy/cpp_infer/include/preprocess_op.h
+++ b/deploy/cpp_infer/include/preprocess_op.h
@@ -56,4 +56,10 @@ public:
const std::vector &rec_image_shape = {3, 32, 320});
};
+class ClsResizeImg {
+public:
+ virtual void Run(const cv::Mat &img, cv::Mat &resize_img,
+ const std::vector &rec_image_shape = {3, 32, 320});
+};
+
} // namespace PaddleOCR
\ No newline at end of file
diff --git a/deploy/cpp_infer/readme.md b/deploy/cpp_infer/readme.md
index 47aa3a1133267a6c9597ea2da08aa0daa43c83d1..571ed2eb2b071574aec3cabdff01b6c9d7f17440 100644
--- a/deploy/cpp_infer/readme.md
+++ b/deploy/cpp_infer/readme.md
@@ -7,6 +7,9 @@
### 运行准备
- Linux环境,推荐使用docker。
+- Windows环境,目前支持基于`Visual Studio 2019 Community`进行编译。
+
+* 该文档主要介绍基于Linux环境的PaddleOCR C++预测流程,如果需要在Windows下基于预测库进行C++预测,具体编译方法请参考[Windows下编译教程](./docs/windows_vs2019_build.md)
### 1.1 编译opencv库
@@ -184,12 +187,15 @@ make -j
### 运行demo
-* 执行以下命令,完成对一幅图像的OCR识别与检测,最终输出
+* 执行以下命令,完成对一幅图像的OCR识别与检测。
```shell
sh tools/run.sh
```
+* 若需要使用方向分类器,则需要将`tools/config.txt`中的`use_angle_cls`参数修改为1,表示开启方向分类器的预测。
+
+
最终屏幕上会输出检测结果如下。
diff --git a/deploy/cpp_infer/readme_en.md b/deploy/cpp_infer/readme_en.md
new file mode 100644
index 0000000000000000000000000000000000000000..a545b8606cda0b476b439543382d997065721892
--- /dev/null
+++ b/deploy/cpp_infer/readme_en.md
@@ -0,0 +1,215 @@
+# Server-side C++ inference
+
+
+In this tutorial, we will introduce the detailed steps of deploying PaddleOCR ultra-lightweight Chinese detection and recognition models on the server side.
+
+
+## 1. Prepare the environment
+
+### Environment
+
+- Linux, docker is recommended.
+
+
+### 1.1 Compile opencv
+
+* First of all, you need to download the source code compiled package in the Linux environment from the opencv official website. Taking opencv3.4.7 as an example, the download command is as follows.
+
+```
+wget https://github.com/opencv/opencv/archive/3.4.7.tar.gz
+tar -xf 3.4.7.tar.gz
+```
+
+Finally, you can see the folder of `opencv-3.4.7/` in the current directory.
+
+* Compile opencv, the opencv source path (`root_path`) and installation path (`install_path`) should be set by yourself. Enter the opencv source code path and compile it in the following way.
+
+
+```shell
+root_path=your_opencv_root_path
+install_path=${root_path}/opencv3
+
+rm -rf build
+mkdir build
+cd build
+
+cmake .. \
+ -DCMAKE_INSTALL_PREFIX=${install_path} \
+ -DCMAKE_BUILD_TYPE=Release \
+ -DBUILD_SHARED_LIBS=OFF \
+ -DWITH_IPP=OFF \
+ -DBUILD_IPP_IW=OFF \
+ -DWITH_LAPACK=OFF \
+ -DWITH_EIGEN=OFF \
+ -DCMAKE_INSTALL_LIBDIR=lib64 \
+ -DWITH_ZLIB=ON \
+ -DBUILD_ZLIB=ON \
+ -DWITH_JPEG=ON \
+ -DBUILD_JPEG=ON \
+ -DWITH_PNG=ON \
+ -DBUILD_PNG=ON \
+ -DWITH_TIFF=ON \
+ -DBUILD_TIFF=ON
+
+make -j
+make install
+```
+
+Among them, `root_path` is the downloaded opencv source code path, and `install_path` is the installation path of opencv. After `make install` is completed, the opencv header file and library file will be generated in this folder for later OCR source code compilation.
+
+
+
+The final file structure under the opencv installation path is as follows.
+
+```
+opencv3/
+|-- bin
+|-- include
+|-- lib
+|-- lib64
+|-- share
+```
+
+### 1.2 Compile or download or the Paddle inference library
+
+* There are 2 ways to obtain the Paddle inference library, described in detail below.
+
+
+#### 1.2.1 Compile from the source code
+* If you want to get the latest Paddle inference library features, you can download the latest code from Paddle github repository and compile the inference library from the source code.
+* You can refer to [Paddle inference library] (https://www.paddlepaddle.org.cn/documentation/docs/en/advanced_guide/inference_deployment/inference/build_and_install_lib_en.html) to get the Paddle source code from github, and then compile To generate the latest inference library. The method of using git to access the code is as follows.
+
+
+```shell
+git clone https://github.com/PaddlePaddle/Paddle.git
+```
+
+* After entering the Paddle directory, the compilation method is as follows.
+
+```shell
+rm -rf build
+mkdir build
+cd build
+
+cmake .. \
+ -DWITH_CONTRIB=OFF \
+ -DWITH_MKL=ON \
+ -DWITH_MKLDNN=ON \
+ -DWITH_TESTING=OFF \
+ -DCMAKE_BUILD_TYPE=Release \
+ -DWITH_INFERENCE_API_TEST=OFF \
+ -DON_INFER=ON \
+ -DWITH_PYTHON=ON
+make -j
+make inference_lib_dist
+```
+
+For more compilation parameter options, please refer to the official website of the Paddle C++ inference library:[https://www.paddlepaddle.org.cn/documentation/docs/en/advanced_guide/inference_deployment/inference/build_and_install_lib_en.html](https://www.paddlepaddle.org.cn/documentation/docs/en/advanced_guide/inference_deployment/inference/build_and_install_lib_en.html).
+
+
+* After the compilation process, you can see the following files in the folder of `build/fluid_inference_install_dir/`.
+
+```
+build/fluid_inference_install_dir/
+|-- CMakeCache.txt
+|-- paddle
+|-- third_party
+|-- version.txt
+```
+
+Among them, `paddle` is the Paddle library required for C++ prediction later, and `version.txt` contains the version information of the current inference library.
+
+
+
+#### 1.2.2 Direct download and installation
+
+* Different cuda versions of the Linux inference library (based on GCC 4.8.2) are provided on the
+[Paddle inference library official website](https://www.paddlepaddle.org.cn/documentation/docs/en/advanced_guide/inference_deployment/inference/build_and_install_lib_en.html). You can view and select the appropriate version of the inference library on the official website.
+
+
+* After downloading, use the following method to uncompress.
+
+```
+tar -xf fluid_inference.tgz
+```
+
+Finally you can see the following files in the folder of `fluid_inference/`.
+
+
+## 2. Compile and run the demo
+
+### 2.1 Export the inference model
+
+* You can refer to [Model inference](../../doc/doc_ch/inference.md),export the inference model. After the model is exported, assuming it is placed in the `inference` directory, the directory structure is as follows.
+
+```
+inference/
+|-- det_db
+| |--model
+| |--params
+|-- rec_rcnn
+| |--model
+| |--params
+```
+
+
+### 2.2 Compile PaddleOCR C++ inference demo
+
+
+* The compilation commands are as follows. The addresses of Paddle C++ inference library, opencv and other Dependencies need to be replaced with the actual addresses on your own machines.
+
+```shell
+sh tools/build.sh
+```
+
+Specifically, the content in `tools/build.sh` is as follows.
+
+```shell
+OPENCV_DIR=your_opencv_dir
+LIB_DIR=your_paddle_inference_dir
+CUDA_LIB_DIR=your_cuda_lib_dir
+CUDNN_LIB_DIR=your_cudnn_lib_dir
+
+BUILD_DIR=build
+rm -rf ${BUILD_DIR}
+mkdir ${BUILD_DIR}
+cd ${BUILD_DIR}
+cmake .. \
+ -DPADDLE_LIB=${LIB_DIR} \
+ -DWITH_MKL=ON \
+ -DDEMO_NAME=ocr_system \
+ -DWITH_GPU=OFF \
+ -DWITH_STATIC_LIB=OFF \
+ -DUSE_TENSORRT=OFF \
+ -DOPENCV_DIR=${OPENCV_DIR} \
+ -DCUDNN_LIB=${CUDNN_LIB_DIR} \
+ -DCUDA_LIB=${CUDA_LIB_DIR} \
+
+make -j
+```
+
+`OPENCV_DIR` is the opencv installation path; `LIB_DIR` is the download (`fluid_inference` folder) or the generated Paddle inference library path (`build/fluid_inference_install_dir` folder); `CUDA_LIB_DIR` is the cuda library file path, in docker; it is `/usr/local/cuda/lib64`; `CUDNN_LIB_DIR` is the cudnn library file path, in docker it is `/usr/lib/x86_64-linux-gnu/`.
+
+
+* After the compilation is completed, an executable file named `ocr_system` will be generated in the `build` folder.
+
+
+### Run the demo
+* Execute the following command to complete the OCR recognition and detection of an image.
+
+```shell
+sh tools/run.sh
+```
+
+* If you want to orientation classifier to correct the detected boxes, you can set `use_angle_cls` in the file `tools/config.txt` as 1 to enable the function.
+
+The detection results will be shown on the screen, which is as follows.
+
+
+
+
+
+
+### 2.3 Note
+
+* `MKLDNN` is disabled by default for C++ inference (`use_mkldnn` in `tools/config.txt` is set to 0), if you need to use MKLDNN for inference acceleration, you need to modify `use_mkldnn` to 1, and use the latest version of the Paddle source code to compile the inference library. When using MKLDNN for CPU prediction, if multiple images are predicted at the same time, there will be a memory leak problem (the problem is not present if MKLDNN is disabled). The problem is currently being fixed, and the temporary solution is: when predicting multiple pictures, Re-initialize the recognition (`CRNNRecognizer`) and detection class (`DBDetector`) every 30 pictures or so.
diff --git a/deploy/cpp_infer/src/config.cpp b/deploy/cpp_infer/src/config.cpp
index 228c874d193771648d0ce559f00f55865a750fee..52dfa209b049c6d47285bcba40e41de846de610f 100644
--- a/deploy/cpp_infer/src/config.cpp
+++ b/deploy/cpp_infer/src/config.cpp
@@ -44,7 +44,7 @@ Config::LoadConfig(const std::string &config_path) {
std::map dict;
for (int i = 0; i < config.size(); i++) {
// pass for empty line or comment
- if (config[i].size() <= 1 or config[i][0] == '#') {
+ if (config[i].size() <= 1 || config[i][0] == '#') {
continue;
}
std::vector res = split(config[i], " ");
diff --git a/deploy/cpp_infer/src/main.cpp b/deploy/cpp_infer/src/main.cpp
index 27c98e5b84367de09f95c901d168c2d318902c43..e708a6e341e6dd5ba66abe46456e2d74a89e0cb5 100644
--- a/deploy/cpp_infer/src/main.cpp
+++ b/deploy/cpp_infer/src/main.cpp
@@ -48,20 +48,30 @@ int main(int argc, char **argv) {
cv::Mat srcimg = cv::imread(img_path, cv::IMREAD_COLOR);
- DBDetector det(config.det_model_dir, config.use_gpu, config.gpu_id,
- config.gpu_mem, config.cpu_math_library_num_threads,
- config.use_mkldnn, config.max_side_len, config.det_db_thresh,
- config.det_db_box_thresh, config.det_db_unclip_ratio,
- config.visualize);
+ DBDetector det(
+ config.det_model_dir, config.use_gpu, config.gpu_id, config.gpu_mem,
+ config.cpu_math_library_num_threads, config.use_mkldnn,
+ config.use_zero_copy_run, config.max_side_len, config.det_db_thresh,
+ config.det_db_box_thresh, config.det_db_unclip_ratio, config.visualize);
+
+ Classifier *cls = nullptr;
+ if (config.use_angle_cls == true) {
+ cls = new Classifier(config.cls_model_dir, config.use_gpu, config.gpu_id,
+ config.gpu_mem, config.cpu_math_library_num_threads,
+ config.use_mkldnn, config.use_zero_copy_run,
+ config.cls_thresh);
+ }
+
CRNNRecognizer rec(config.rec_model_dir, config.use_gpu, config.gpu_id,
config.gpu_mem, config.cpu_math_library_num_threads,
- config.use_mkldnn, config.char_list_file);
+ config.use_mkldnn, config.use_zero_copy_run,
+ config.char_list_file);
auto start = std::chrono::system_clock::now();
std::vector>> boxes;
det.Run(srcimg, boxes);
- rec.Run(boxes, srcimg);
+ rec.Run(boxes, srcimg, cls);
auto end = std::chrono::system_clock::now();
auto duration =
diff --git a/deploy/cpp_infer/src/ocr_cls.cpp b/deploy/cpp_infer/src/ocr_cls.cpp
new file mode 100644
index 0000000000000000000000000000000000000000..7cdaaab40108026edffe5cb1ca53ac3972768cc6
--- /dev/null
+++ b/deploy/cpp_infer/src/ocr_cls.cpp
@@ -0,0 +1,110 @@
+// Copyright (c) 2020 PaddlePaddle Authors. All Rights Reserved.
+//
+// Licensed under the Apache License, Version 2.0 (the "License");
+// you may not use this file except in compliance with the License.
+// You may obtain a copy of the License at
+//
+// http://www.apache.org/licenses/LICENSE-2.0
+//
+// Unless required by applicable law or agreed to in writing, software
+// distributed under the License is distributed on an "AS IS" BASIS,
+// WITHOUT WARRANTIES OR CONDITIONS OF ANY KIND, either express or implied.
+// See the License for the specific language governing permissions and
+// limitations under the License.
+
+#include
+
+namespace PaddleOCR {
+
+cv::Mat Classifier::Run(cv::Mat &img) {
+ cv::Mat src_img;
+ img.copyTo(src_img);
+ cv::Mat resize_img;
+
+ std::vector rec_image_shape = {3, 32, 100};
+ int index = 0;
+ float wh_ratio = float(img.cols) / float(img.rows);
+
+ this->resize_op_.Run(img, resize_img, rec_image_shape);
+
+ this->normalize_op_.Run(&resize_img, this->mean_, this->scale_,
+ this->is_scale_);
+
+ std::vector input(1 * 3 * resize_img.rows * resize_img.cols, 0.0f);
+
+ this->permute_op_.Run(&resize_img, input.data());
+
+ // Inference.
+ if (this->use_zero_copy_run_) {
+ auto input_names = this->predictor_->GetInputNames();
+ auto input_t = this->predictor_->GetInputTensor(input_names[0]);
+ input_t->Reshape({1, 3, resize_img.rows, resize_img.cols});
+ input_t->copy_from_cpu(input.data());
+ this->predictor_->ZeroCopyRun();
+ } else {
+ paddle::PaddleTensor input_t;
+ input_t.shape = {1, 3, resize_img.rows, resize_img.cols};
+ input_t.data =
+ paddle::PaddleBuf(input.data(), input.size() * sizeof(float));
+ input_t.dtype = PaddleDType::FLOAT32;
+ std::vector outputs;
+ this->predictor_->Run({input_t}, &outputs, 1);
+ }
+
+ std::vector softmax_out;
+ std::vector label_out;
+ auto output_names = this->predictor_->GetOutputNames();
+ auto softmax_out_t = this->predictor_->GetOutputTensor(output_names[0]);
+ auto label_out_t = this->predictor_->GetOutputTensor(output_names[1]);
+ auto softmax_shape_out = softmax_out_t->shape();
+ auto label_shape_out = label_out_t->shape();
+
+ int softmax_out_num =
+ std::accumulate(softmax_shape_out.begin(), softmax_shape_out.end(), 1,
+ std::multiplies());
+
+ int label_out_num =
+ std::accumulate(label_shape_out.begin(), label_shape_out.end(), 1,
+ std::multiplies());
+ softmax_out.resize(softmax_out_num);
+ label_out.resize(label_out_num);
+
+ softmax_out_t->copy_to_cpu(softmax_out.data());
+ label_out_t->copy_to_cpu(label_out.data());
+
+ int label = label_out[0];
+ float score = softmax_out[label];
+ // std::cout << "\nlabel "<