diff --git a/configs/rtdetr/README.md b/configs/rtdetr/README.md
index 92ca0347ba9a088158e765929fb6726723be60e8..e1f2a5be89483b7b253f1b528e8b8cd94eab5e4e 100644
--- a/configs/rtdetr/README.md
+++ b/configs/rtdetr/README.md
@@ -1,34 +1,125 @@
# DETRs Beat YOLOs on Real-time Object Detection
-## Introduction
-We propose a **R**eal-**T**ime **DE**tection **TR**ansformer (RT-DETR), the first real-time end-to-end object detector to our best knowledge. Specifically, we design an efficient hybrid encoder to efficiently process multi-scale features by decoupling the intra-scale interaction and cross-scale fusion, and propose IoU-aware query selection to improve the initialization of object queries. In addition, our proposed detector supports flexibly adjustment of the inference speed by using different decoder layers without the need for retraining, which facilitates the practical application of real-time object detectors. Our RT-DETR-L achieves 53.0% AP on COCO val2017 and 114 FPS on T4 GPU, while RT-DETR-X achieves 54.8% AP and 74 FPS, outperforming all YOLO detectors of the same scale in both speed and accuracy. Furthermore, our RT-DETR-R50 achieves 53.1% AP and 108 FPS, outperforming DINO-Deformable-DETR-R50 by 2.2% AP in accuracy and by about 21 times in FPS. For more details, please refer to our [paper](https://arxiv.org/abs/2304.08069).
+## 最新动态
-
-
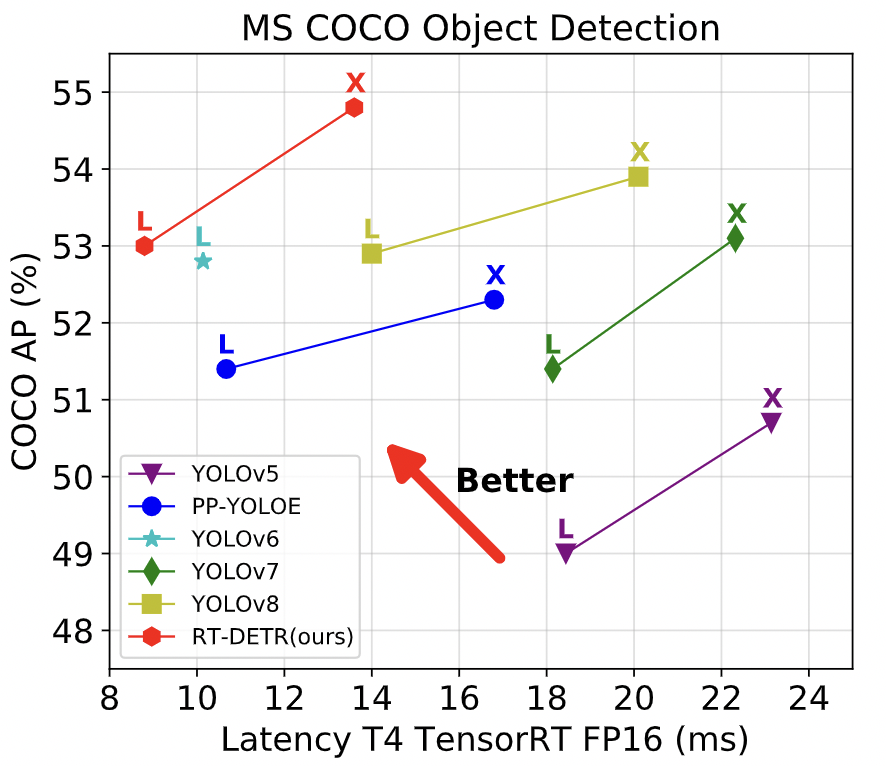
-
+- 发布RT-DETR-R50和RT-DETR-R101的代码和预训练模型。
+- 发布RT-DETR-L和RT-DETR-X的代码和预训练模型。
+## 简介
+
+RT-DETR是第一个实时端到端目标检测器。具体而言,我们设计了一个高效的混合编码器,通过解耦尺度内交互和跨尺度融合来高效处理多尺度特征,并提出了IoU感知的查询选择机制,以优化解码器查询的初始化。此外,RT-DETR支持通过使用不同的解码器层来灵活调整推理速度,而不需要重新训练,这有助于实时目标检测器的实际应用。RT-DETR-L在COCO val2017上实现了53.0%的AP,在T4 GPU上实现了114FPS,RT-DETR-X实现了54.8%的AP和74FPS,在速度和精度方面都优于相同规模的所有YOLO检测器。RT-DETR-R50实现了53.1%的AP和108FPS,RT-DETR-R101实现了54.3%的AP和74FPS,在精度上超过了全部使用相同骨干网络的DETR检测器。
+若要了解更多细节,请参考我们的论文[paper](https://arxiv.org/abs/2304.08069).
-## Model Zoo
+
+
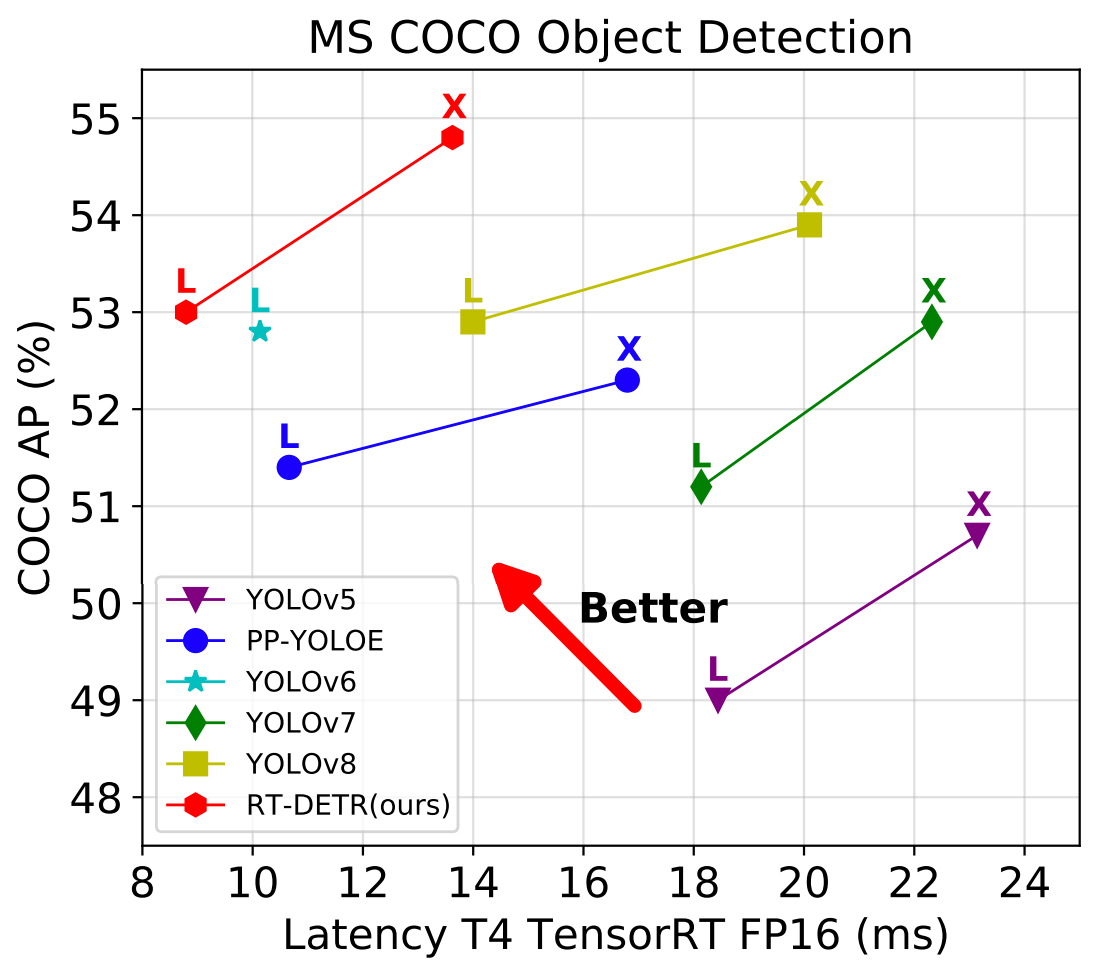
+
-### Model Zoo on COCO
+## 模型
| Model | Epoch | backbone | input shape | $AP^{val}$ | $AP^{val}_{50}$| Params(M) | FLOPs(G) | T4 TensorRT FP16(FPS) | Pretrained Model | config |
|:--------------:|:-----:|:----------:| :-------:|:--------------------------:|:---------------------------:|:---------:|:--------:| :---------------------: |:------------------------------------------------------------------------------------:|:-------------------------------------------:|
| RT-DETR-R50 | 6x | ResNet-50 | 640 | 53.1 | 71.3 | 42 | 136 | 108 | [download](https://bj.bcebos.com/v1/paddledet/models/rtdetr_r50vd_6x_coco.pdparams) | [config](./rtdetr_r50vd_6x_coco.yml)
| RT-DETR-R101 | 6x | ResNet-101 | 640 | 54.3 | 72.7 | 76 | 259 | 74 | [download](https://bj.bcebos.com/v1/paddledet/models/rtdetr_r101vd_6x_coco.pdparams) | [config](./rtdetr_r101vd_6x_coco.yml)
-| RT-DETR-L | 6x | HGNetv2 | 640 | 53.0 | 71.6 | 32 | 110 | 114 | [coming soon](https://bj.bcebos.com/v1/paddledet/models/rtdetr_hgnetv2_l_6x_coco.pdparams) | [coming soon](rtdetr_hgnetv2_l_6x_coco.yml)
-| RT-DETR-X | 6x | HGNetv2 | 640 | 54.8 | 73.1 | 67 | 234 | 74 | [coming soon](https://bj.bcebos.com/v1/paddledet/models/rtdetr_hgnetv2_x_6x_coco.pdparams) | [coming soon](rtdetr_hgnetv2_x_6x_coco.yml)
+| RT-DETR-L | 6x | HGNetv2 | 640 | 53.0 | 71.6 | 32 | 110 | 114 | [download](https://bj.bcebos.com/v1/paddledet/models/rtdetr_hgnetv2_l_6x_coco.pdparams) | [config](rtdetr_hgnetv2_l_6x_coco.yml)
+| RT-DETR-X | 6x | HGNetv2 | 640 | 54.8 | 73.1 | 67 | 234 | 74 | [download](https://bj.bcebos.com/v1/paddledet/models/rtdetr_hgnetv2_x_6x_coco.pdparams) | [config](rtdetr_hgnetv2_x_6x_coco.yml)
+
+**注意事项:**
+- RT-DETR 使用4个GPU训练。
+- RT-DETR 在COCO train2017上训练,并在val2017上评估。
+
+## 快速开始
+
+
+依赖包:
+
+- PaddlePaddle == 2.4.1
+
+
+
+
+安装
-**Notes:**
-- RT-DETR uses 4GPU to train.
-- RT-DETR is trained on COCO train2017 dataset and evaluated on val2017 results of `mAP(IoU=0.5:0.95)`.
+- [安装指导文档](https://github.com/PaddlePaddle/PaddleDetection/blob/develop/docs/tutorials/INSTALL.md)
-GPU multi-card training
-```bash
+
+
+
+训练&评估
+
+- 单卡GPU上训练:
+
+```shell
+# training on single-GPU
+export CUDA_VISIBLE_DEVICES=0
+python tools/train.py -c configs/rtdetr/rtdetr_r50vd_6x_coco.yml --eval
+```
+
+- 多卡GPU上训练:
+
+```shell
+# training on multi-GPU
+export CUDA_VISIBLE_DEVICES=0,1,2,3
python -m paddle.distributed.launch --gpus 0,1,2,3 tools/train.py -c configs/rtdetr/rtdetr_r50vd_6x_coco.yml --fleet --eval
```
-## Citations
+- 评估:
+
+```shell
+python tools/eval.py -c configs/rtdetr/rtdetr_r50vd_6x_coco.yml \
+ -o weights=https://bj.bcebos.com/v1/paddledet/models/rtdetr_r50vd_6x_coco.pdparams
+```
+
+- 测试:
+
+```shell
+python tools/infer.py -c configs/rtdetr/rtdetr_r50vd_6x_coco.yml \
+ -o weights=https://bj.bcebos.com/v1/paddledet/models/rtdetr_r50vd_6x_coco.pdparams
+```
+
+详情请参考[快速开始文档](https://github.com/PaddlePaddle/PaddleDetection/blob/develop/docs/tutorials/GETTING_STARTED.md).
+
+
+
+## 部署
+
+### 导出及转换模型
+
+
+1. 导出模型
+
+```shell
+cd PaddleDetection
+python tools/export_model.py -c configs/rtdetr/rtdetr_r50vd_6x_coco.yml \
+ -o weights=https://bj.bcebos.com/v1/paddledet/models/rtdetr_r50vd_6x_coco.pdparams trt=True \
+ --output_dir=output_inference
+```
+
+
+
+
+2. 转换模型至ONNX (点击展开)
+
+- 安装[Paddle2ONNX](https://github.com/PaddlePaddle/Paddle2ONNX) 和 ONNX
+
+```shell
+pip install onnx==1.13.0
+pip install paddle2onnx==1.0.5
+```
+
+- 转换模型:
+
+```shell
+paddle2onnx --model_dir=./output_inference/rtdetr_r50vd_6x_coco/ \
+ --model_filename model.pdmodel \
+ --params_filename model.pdiparams \
+ --opset_version 16 \
+ --save_file rtdetr_r50vd_6x_coco.onnx
+```
+
+
+
+## 引用RT-DETR
+如果需要在你的研究中使用RT-DETR,请通过以下方式引用我们的论文:
```
@misc{lv2023detrs,
title={DETRs Beat YOLOs on Real-time Object Detection},
diff --git a/configs/rtdetr/rtdetr_hgnetv2_l_6x_coco.yml b/configs/rtdetr/rtdetr_hgnetv2_l_6x_coco.yml
new file mode 100644
index 0000000000000000000000000000000000000000..4f3e77df187fca27a0cf46b547c0071bef789bd0
--- /dev/null
+++ b/configs/rtdetr/rtdetr_hgnetv2_l_6x_coco.yml
@@ -0,0 +1,24 @@
+_BASE_: [
+ '../datasets/coco_detection.yml',
+ '../runtime.yml',
+ '_base_/optimizer_6x.yml',
+ '_base_/rtdetr_r50vd.yml',
+ '_base_/rtdetr_reader.yml',
+]
+
+weights: output/rtdetr_hgnetv2_l_6x_coco/model_final
+pretrain_weights: https://bj.bcebos.com/v1/paddledet/models/pretrained/PPHGNetV2_L_ssld_pretrained.pdparams
+find_unused_parameters: True
+log_iter: 200
+
+
+DETR:
+ backbone: PPHGNetV2
+
+PPHGNetV2:
+ arch: 'L'
+ return_idx: [1, 2, 3]
+ freeze_stem_only: True
+ freeze_at: 0
+ freeze_norm: True
+ lr_mult_list: [0., 0.05, 0.05, 0.05, 0.05]
diff --git a/configs/rtdetr/rtdetr_hgnetv2_x_6x_coco.yml b/configs/rtdetr/rtdetr_hgnetv2_x_6x_coco.yml
new file mode 100644
index 0000000000000000000000000000000000000000..37f5d17930cb433c55bdbbdf547a11798d86cc23
--- /dev/null
+++ b/configs/rtdetr/rtdetr_hgnetv2_x_6x_coco.yml
@@ -0,0 +1,40 @@
+_BASE_: [
+ '../datasets/coco_detection.yml',
+ '../runtime.yml',
+ '_base_/optimizer_6x.yml',
+ '_base_/rtdetr_r50vd.yml',
+ '_base_/rtdetr_reader.yml',
+]
+
+weights: output/rtdetr_hgnetv2_l_6x_coco/model_final
+pretrain_weights: https://bj.bcebos.com/v1/paddledet/models/pretrained/PPHGNetV2_X_ssld_pretrained.pdparams
+find_unused_parameters: True
+log_iter: 200
+
+
+
+DETR:
+ backbone: PPHGNetV2
+
+
+PPHGNetV2:
+ arch: 'X'
+ return_idx: [1, 2, 3]
+ freeze_stem_only: True
+ freeze_at: 0
+ freeze_norm: True
+ lr_mult_list: [0., 0.01, 0.01, 0.01, 0.01]
+
+
+HybridEncoder:
+ hidden_dim: 384
+ use_encoder_idx: [2]
+ num_encoder_layers: 1
+ encoder_layer:
+ name: TransformerLayer
+ d_model: 384
+ nhead: 8
+ dim_feedforward: 2048
+ dropout: 0.
+ activation: 'gelu'
+ expansion: 1.0
diff --git a/ppdet/modeling/backbones/__init__.py b/ppdet/modeling/backbones/__init__.py
index a20189c948768fcf7edef3e4b420c6fc655263a8..e61ff711186e3191b14f4e41a6363dbf8886e6c6 100644
--- a/ppdet/modeling/backbones/__init__.py
+++ b/ppdet/modeling/backbones/__init__.py
@@ -37,6 +37,7 @@ from . import mobileone
from . import trans_encoder
from . import focalnet
from . import vit_mae
+from . import hgnet_v2
from .vgg import *
from .resnet import *
@@ -63,4 +64,5 @@ from .mobileone import *
from .trans_encoder import *
from .focalnet import *
from .vitpose import *
-from .vit_mae import *
\ No newline at end of file
+from .vit_mae import *
+from .hgnet_v2 import *
diff --git a/ppdet/modeling/backbones/hgnet_v2.py b/ppdet/modeling/backbones/hgnet_v2.py
new file mode 100644
index 0000000000000000000000000000000000000000..c4cc0787a2364fa076f189de263687395431c64f
--- /dev/null
+++ b/ppdet/modeling/backbones/hgnet_v2.py
@@ -0,0 +1,446 @@
+# copyright (c) 2023 PaddlePaddle Authors. All Rights Reserve.
+#
+# Licensed under the Apache License, Version 2.0 (the "License");
+# you may not use this file except in compliance with the License.
+# You may obtain a copy of the License at
+#
+# http://www.apache.org/licenses/LICENSE-2.0
+#
+# Unless required by applicable law or agreed to in writing, software
+# distributed under the License is distributed on an "AS IS" BASIS,
+# WITHOUT WARRANTIES OR CONDITIONS OF ANY KIND, either express or implied.
+# See the License for the specific language governing permissions and
+# limitations under the License.
+
+import paddle
+import paddle.nn as nn
+import paddle.nn.functional as F
+from paddle.nn.initializer import KaimingNormal, Constant
+from paddle.nn import Conv2D, BatchNorm2D, ReLU, AdaptiveAvgPool2D, MaxPool2D
+from paddle.regularizer import L2Decay
+from paddle import ParamAttr
+
+import copy
+
+from ppdet.core.workspace import register, serializable
+from ..shape_spec import ShapeSpec
+
+__all__ = ['PPHGNetV2']
+
+kaiming_normal_ = KaimingNormal()
+zeros_ = Constant(value=0.)
+ones_ = Constant(value=1.)
+
+
+class LearnableAffineBlock(nn.Layer):
+ def __init__(self,
+ scale_value=1.0,
+ bias_value=0.0,
+ lr_mult=1.0,
+ lab_lr=0.01):
+ super().__init__()
+ self.scale = self.create_parameter(
+ shape=[1, ],
+ default_initializer=Constant(value=scale_value),
+ attr=ParamAttr(learning_rate=lr_mult * lab_lr))
+ self.add_parameter("scale", self.scale)
+ self.bias = self.create_parameter(
+ shape=[1, ],
+ default_initializer=Constant(value=bias_value),
+ attr=ParamAttr(learning_rate=lr_mult * lab_lr))
+ self.add_parameter("bias", self.bias)
+
+ def forward(self, x):
+ return self.scale * x + self.bias
+
+
+class ConvBNAct(nn.Layer):
+ def __init__(self,
+ in_channels,
+ out_channels,
+ kernel_size=3,
+ stride=1,
+ padding=1,
+ groups=1,
+ use_act=True,
+ use_lab=False,
+ lr_mult=1.0):
+ super().__init__()
+ self.use_act = use_act
+ self.use_lab = use_lab
+ self.conv = Conv2D(
+ in_channels,
+ out_channels,
+ kernel_size,
+ stride,
+ padding=padding
+ if isinstance(padding, str) else (kernel_size - 1) // 2,
+ groups=groups,
+ bias_attr=False)
+ self.bn = BatchNorm2D(
+ out_channels,
+ weight_attr=ParamAttr(
+ regularizer=L2Decay(0.0), learning_rate=lr_mult),
+ bias_attr=ParamAttr(
+ regularizer=L2Decay(0.0), learning_rate=lr_mult))
+ if self.use_act:
+ self.act = ReLU()
+ if self.use_lab:
+ self.lab = LearnableAffineBlock(lr_mult=lr_mult)
+
+ def forward(self, x):
+ x = self.conv(x)
+ x = self.bn(x)
+ if self.use_act:
+ x = self.act(x)
+ if self.use_lab:
+ x = self.lab(x)
+ return x
+
+
+class LightConvBNAct(nn.Layer):
+ def __init__(self,
+ in_channels,
+ out_channels,
+ kernel_size,
+ stride,
+ groups=1,
+ use_lab=False,
+ lr_mult=1.0):
+ super().__init__()
+ self.conv1 = ConvBNAct(
+ in_channels=in_channels,
+ out_channels=out_channels,
+ kernel_size=1,
+ use_act=False,
+ use_lab=use_lab,
+ lr_mult=lr_mult)
+ self.conv2 = ConvBNAct(
+ in_channels=out_channels,
+ out_channels=out_channels,
+ kernel_size=kernel_size,
+ groups=out_channels,
+ use_act=True,
+ use_lab=use_lab,
+ lr_mult=lr_mult)
+
+ def forward(self, x):
+ x = self.conv1(x)
+ x = self.conv2(x)
+ return x
+
+
+class StemBlock(nn.Layer):
+ def __init__(self,
+ in_channels,
+ mid_channels,
+ out_channels,
+ use_lab=False,
+ lr_mult=1.0):
+ super().__init__()
+ self.stem1 = ConvBNAct(
+ in_channels=in_channels,
+ out_channels=mid_channels,
+ kernel_size=3,
+ stride=2,
+ use_lab=use_lab,
+ lr_mult=lr_mult)
+ self.stem2a = ConvBNAct(
+ in_channels=mid_channels,
+ out_channels=mid_channels // 2,
+ kernel_size=2,
+ stride=1,
+ padding="SAME",
+ use_lab=use_lab,
+ lr_mult=lr_mult)
+ self.stem2b = ConvBNAct(
+ in_channels=mid_channels // 2,
+ out_channels=mid_channels,
+ kernel_size=2,
+ stride=1,
+ padding="SAME",
+ use_lab=use_lab,
+ lr_mult=lr_mult)
+ self.stem3 = ConvBNAct(
+ in_channels=mid_channels * 2,
+ out_channels=mid_channels,
+ kernel_size=3,
+ stride=2,
+ use_lab=use_lab,
+ lr_mult=lr_mult)
+ self.stem4 = ConvBNAct(
+ in_channels=mid_channels,
+ out_channels=out_channels,
+ kernel_size=1,
+ stride=1,
+ use_lab=use_lab,
+ lr_mult=lr_mult)
+ self.pool = nn.MaxPool2D(
+ kernel_size=2, stride=1, ceil_mode=True, padding="SAME")
+
+ def forward(self, x):
+ x = self.stem1(x)
+ x2 = self.stem2a(x)
+ x2 = self.stem2b(x2)
+ x1 = self.pool(x)
+ x = paddle.concat([x1, x2], 1)
+ x = self.stem3(x)
+ x = self.stem4(x)
+
+ return x
+
+
+class HG_Block(nn.Layer):
+ def __init__(self,
+ in_channels,
+ mid_channels,
+ out_channels,
+ kernel_size=3,
+ layer_num=6,
+ identity=False,
+ light_block=True,
+ use_lab=False,
+ lr_mult=1.0):
+ super().__init__()
+ self.identity = identity
+
+ self.layers = nn.LayerList()
+ block_type = "LightConvBNAct" if light_block else "ConvBNAct"
+ for i in range(layer_num):
+ self.layers.append(
+ eval(block_type)(in_channels=in_channels
+ if i == 0 else mid_channels,
+ out_channels=mid_channels,
+ stride=1,
+ kernel_size=kernel_size,
+ use_lab=use_lab,
+ lr_mult=lr_mult))
+ # feature aggregation
+ total_channels = in_channels + layer_num * mid_channels
+ self.aggregation_squeeze_conv = ConvBNAct(
+ in_channels=total_channels,
+ out_channels=out_channels // 2,
+ kernel_size=1,
+ stride=1,
+ use_lab=use_lab,
+ lr_mult=lr_mult)
+ self.aggregation_excitation_conv = ConvBNAct(
+ in_channels=out_channels // 2,
+ out_channels=out_channels,
+ kernel_size=1,
+ stride=1,
+ use_lab=use_lab,
+ lr_mult=lr_mult)
+
+ def forward(self, x):
+ identity = x
+ output = []
+ output.append(x)
+ for layer in self.layers:
+ x = layer(x)
+ output.append(x)
+ x = paddle.concat(output, axis=1)
+ x = self.aggregation_squeeze_conv(x)
+ x = self.aggregation_excitation_conv(x)
+ if self.identity:
+ x += identity
+ return x
+
+
+class HG_Stage(nn.Layer):
+ def __init__(self,
+ in_channels,
+ mid_channels,
+ out_channels,
+ block_num,
+ layer_num=6,
+ downsample=True,
+ light_block=True,
+ kernel_size=3,
+ use_lab=False,
+ lr_mult=1.0):
+ super().__init__()
+ self.downsample = downsample
+ if downsample:
+ self.downsample = ConvBNAct(
+ in_channels=in_channels,
+ out_channels=in_channels,
+ kernel_size=3,
+ stride=2,
+ groups=in_channels,
+ use_act=False,
+ use_lab=use_lab,
+ lr_mult=lr_mult)
+
+ blocks_list = []
+ for i in range(block_num):
+ blocks_list.append(
+ HG_Block(
+ in_channels=in_channels if i == 0 else out_channels,
+ mid_channels=mid_channels,
+ out_channels=out_channels,
+ kernel_size=kernel_size,
+ layer_num=layer_num,
+ identity=False if i == 0 else True,
+ light_block=light_block,
+ use_lab=use_lab,
+ lr_mult=lr_mult))
+ self.blocks = nn.Sequential(*blocks_list)
+
+ def forward(self, x):
+ if self.downsample:
+ x = self.downsample(x)
+ x = self.blocks(x)
+ return x
+
+
+def _freeze_norm(m: nn.BatchNorm2D):
+ param_attr = ParamAttr(
+ learning_rate=0., regularizer=L2Decay(0.), trainable=False)
+ bias_attr = ParamAttr(
+ learning_rate=0., regularizer=L2Decay(0.), trainable=False)
+ global_stats = True
+ norm = nn.BatchNorm2D(
+ m._num_features,
+ weight_attr=param_attr,
+ bias_attr=bias_attr,
+ use_global_stats=global_stats)
+ for param in norm.parameters():
+ param.stop_gradient = True
+ return norm
+
+
+def reset_bn(model: nn.Layer, reset_func=_freeze_norm):
+ if isinstance(model, nn.BatchNorm2D):
+ model = reset_func(model)
+ else:
+ for name, child in model.named_children():
+ _child = reset_bn(child, reset_func)
+ if _child is not child:
+ setattr(model, name, _child)
+ return model
+
+
+@register
+@serializable
+class PPHGNetV2(nn.Layer):
+ """
+ PPHGNetV2
+ Args:
+ stem_channels: list. Number of channels for the stem block.
+ stage_type: str. The stage configuration of PPHGNet. such as the number of channels, stride, etc.
+ use_lab: boolean. Whether to use LearnableAffineBlock in network.
+ lr_mult_list: list. Control the learning rate of different stages.
+ Returns:
+ model: nn.Layer. Specific PPHGNetV2 model depends on args.
+ """
+
+ arch_configs = {
+ 'L': {
+ 'stem_channels': [3, 32, 48],
+ 'stage_config': {
+ # in_channels, mid_channels, out_channels, num_blocks, downsample, light_block, kernel_size, layer_num
+ "stage1": [48, 48, 128, 1, False, False, 3, 6],
+ "stage2": [128, 96, 512, 1, True, False, 3, 6],
+ "stage3": [512, 192, 1024, 3, True, True, 5, 6],
+ "stage4": [1024, 384, 2048, 1, True, True, 5, 6],
+ }
+ },
+ 'X': {
+ 'stem_channels': [3, 32, 64],
+ 'stage_config': {
+ # in_channels, mid_channels, out_channels, num_blocks, downsample, light_block, kernel_size, layer_num
+ "stage1": [64, 64, 128, 1, False, False, 3, 6],
+ "stage2": [128, 128, 512, 2, True, False, 3, 6],
+ "stage3": [512, 256, 1024, 5, True, True, 5, 6],
+ "stage4": [1024, 512, 2048, 2, True, True, 5, 6],
+ }
+ }
+ }
+
+ def __init__(self,
+ arch,
+ use_lab=False,
+ lr_mult_list=[1.0, 1.0, 1.0, 1.0, 1.0],
+ return_idx=[1, 2, 3],
+ freeze_stem_only=True,
+ freeze_at=0,
+ freeze_norm=True):
+ super().__init__()
+ self.use_lab = use_lab
+ self.return_idx = return_idx
+
+ stem_channels = self.arch_configs[arch]['stem_channels']
+ stage_config = self.arch_configs[arch]['stage_config']
+
+ self._out_strides = [4, 8, 16, 32]
+ self._out_channels = [stage_config[k][2] for k in stage_config]
+
+ # stem
+ self.stem = StemBlock(
+ in_channels=stem_channels[0],
+ mid_channels=stem_channels[1],
+ out_channels=stem_channels[2],
+ use_lab=use_lab,
+ lr_mult=lr_mult_list[0])
+
+ # stages
+ self.stages = nn.LayerList()
+ for i, k in enumerate(stage_config):
+ in_channels, mid_channels, out_channels, block_num, downsample, light_block, kernel_size, layer_num = stage_config[
+ k]
+ self.stages.append(
+ HG_Stage(
+ in_channels,
+ mid_channels,
+ out_channels,
+ block_num,
+ layer_num,
+ downsample,
+ light_block,
+ kernel_size,
+ use_lab,
+ lr_mult=lr_mult_list[i + 1]))
+
+ if freeze_at >= 0:
+ self._freeze_parameters(self.stem)
+ if not freeze_stem_only:
+ for i in range(min(freeze_at + 1, len(self.stages))):
+ self._freeze_parameters(self.stages[i])
+
+ if freeze_norm:
+ reset_bn(self, reset_func=_freeze_norm)
+
+ self._init_weights()
+
+ def _freeze_parameters(self, m):
+ for p in m.parameters():
+ p.stop_gradient = True
+
+ def _init_weights(self):
+ for m in self.sublayers():
+ if isinstance(m, nn.Conv2D):
+ kaiming_normal_(m.weight)
+ elif isinstance(m, (nn.BatchNorm2D)):
+ ones_(m.weight)
+ zeros_(m.bias)
+ elif isinstance(m, nn.Linear):
+ zeros_(m.bias)
+
+ @property
+ def out_shape(self):
+ return [
+ ShapeSpec(
+ channels=self._out_channels[i], stride=self._out_strides[i])
+ for i in self.return_idx
+ ]
+
+ def forward(self, inputs):
+ x = inputs['image']
+ x = self.stem(x)
+ outs = []
+ for idx, stage in enumerate(self.stages):
+ x = stage(x)
+ if idx in self.return_idx:
+ outs.append(x)
+ return outs