diff --git a/configs/smalldet/README.md b/configs/smalldet/README.md
index 042e2b25a4f305f04bca7b58afb0cbed01cd96f3..cdae33ea2c0fe8cdbcb15c9f8f3efc2193948bf2 100644
--- a/configs/smalldet/README.md
+++ b/configs/smalldet/README.md
@@ -1,16 +1,331 @@
-# PP-YOLOE Smalldet 检测模型
+# PP-YOLOE 小目标检测模型
+
+PaddleDetection团队提供了针对VisDrone-DET、DOTA水平框、Xview等小目标场景数据集的基于PP-YOLOE的检测模型,以及提供了一套使用[SAHI](https://github.com/obss/sahi)(Slicing Aided Hyper Inference)工具切图和拼图的方案,用户可以下载模型进行使用。
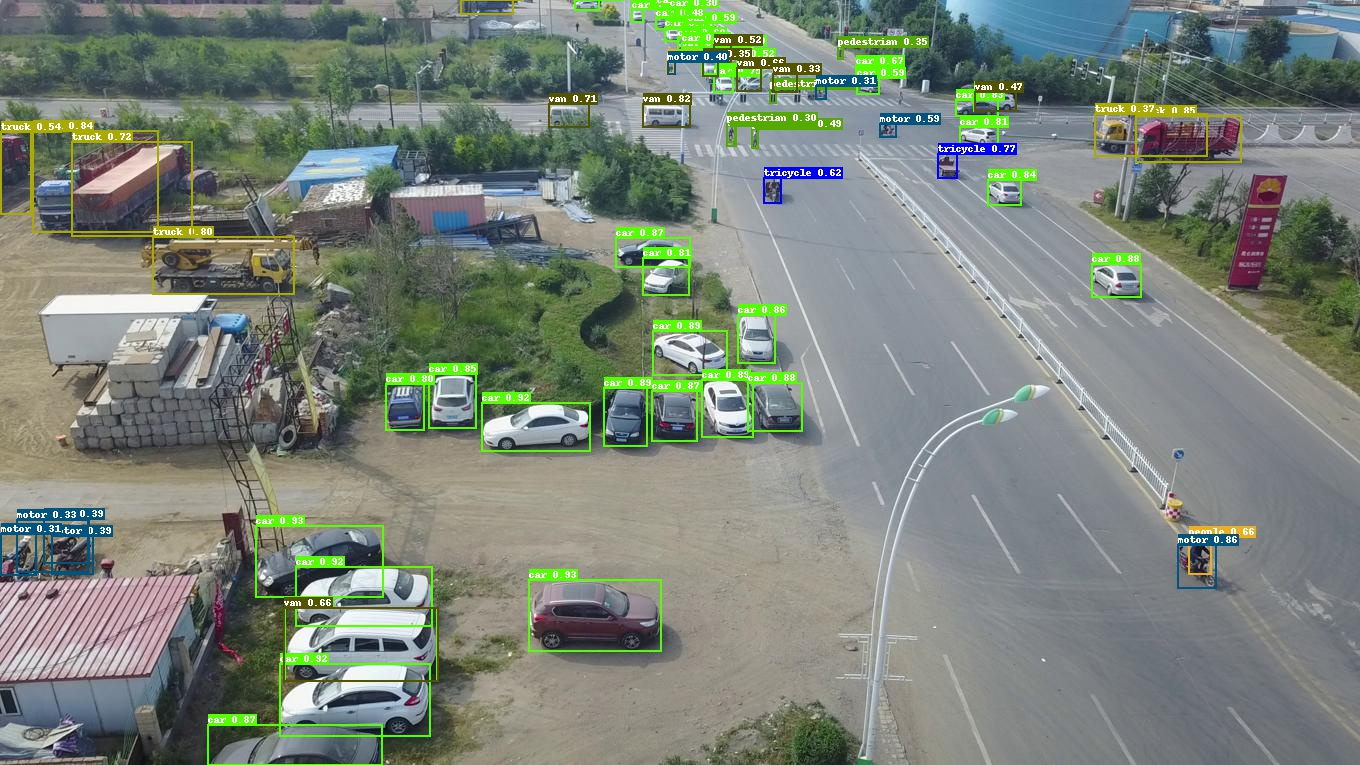
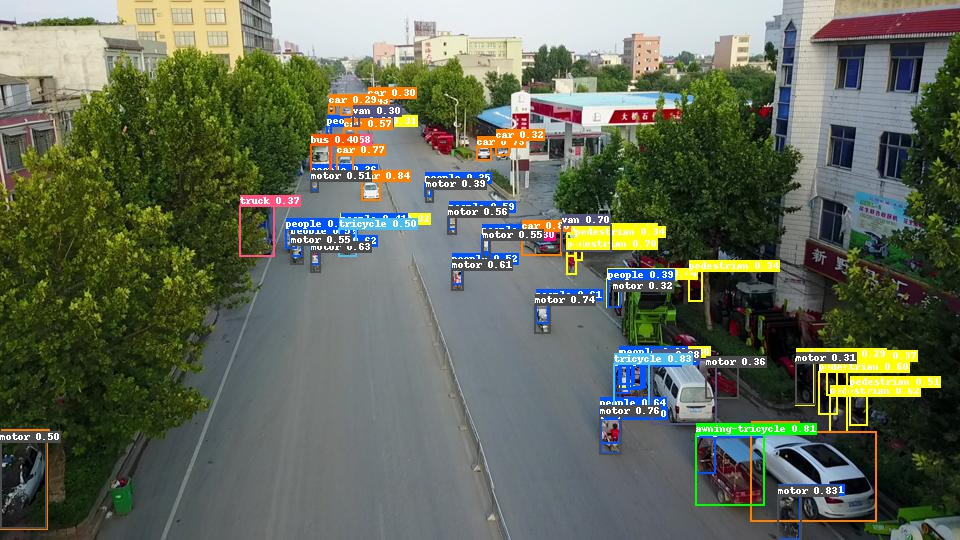
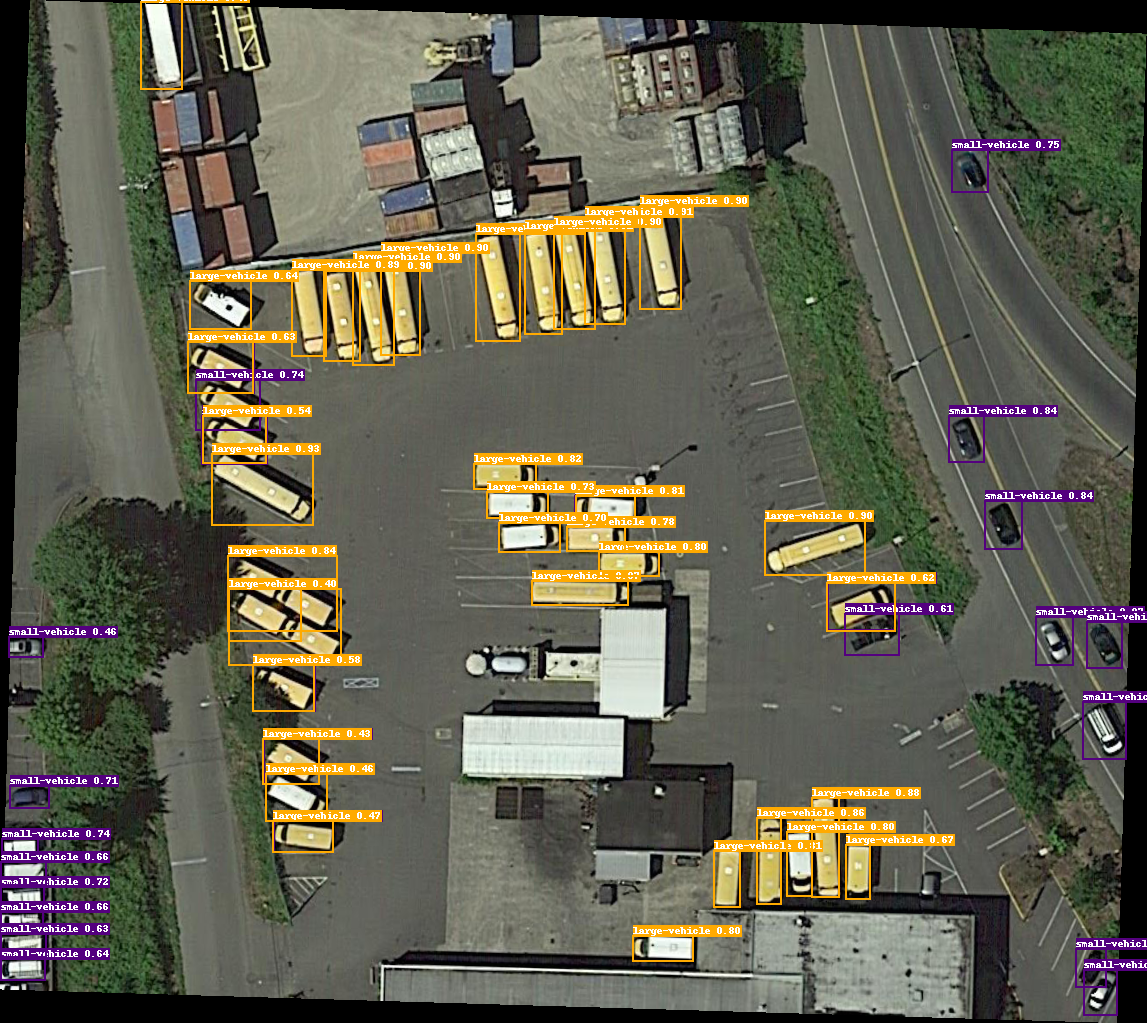
+## 基础模型:
| 模型 | 数据集 | SLICE_SIZE | OVERLAP_RATIO | 类别数 | mAPval
0.5:0.95 | APval
0.5 | 下载链接 | 配置文件 |
|:---------|:---------------:|:---------------:|:---------------:|:------:|:-----------------------:|:-------------------:|:---------:| :-----: |
-|PP-YOLOE-l| Xview | 400 | 0.25 | 60 | 14.5 | 26.8 | [下载链接](https://bj.bcebos.com/v1/paddledet/models/ppyoloe_crn_l_xview_400_025.pdparams) | [配置文件](./ppyoloe_crn_l_80e_sliced_xview_400_025.yml) |
-|PP-YOLOE-l| DOTA | 500 | 0.25 | 15 | 46.8 | 72.6 | [下载链接](https://bj.bcebos.com/v1/paddledet/models/ppyoloe_crn_l_dota_500_025.pdparams) | [配置文件](./ppyoloe_crn_l_80e_sliced_DOTA_500_025.yml) |
-|PP-YOLOE-l| VisDrone | 500 | 0.25 | 10 | 29.7 | 48.5 | [下载链接](https://bj.bcebos.com/v1/paddledet/models/ppyoloe_crn_l_80e_sliced_visdrone_640_025.pdparams) | [配置文件](./ppyoloe_crn_l_80e_sliced_visdrone_640_025.yml) |
+|PP-YOLOE-P2-l| DOTA | 500 | 0.25 | 15 | 53.9 | 78.6 | [下载链接](https://bj.bcebos.com/v1/paddledet/models/ppyoloe_p2_crn_l_80e_sliced_DOTA_500_025.pdparams) | [配置文件](./ppyoloe_p2_crn_l_80e_sliced_DOTA_500_025.yml) |
+|PP-YOLOE-P2-l| Xview | 400 | 0.25 | 60 | 14.9 | 27.0 | [下载链接](https://bj.bcebos.com/v1/paddledet/models/ppyoloe_p2_crn_l_80e_sliced_xview_400_025.pdparams) | [配置文件](./ppyoloe_p2_crn_l_80e_sliced_xview_400_025.yml) |
+|PP-YOLOE-l| VisDrone-DET| 640 | 0.25 | 10 | 38.5 | 60.2 | [下载链接](https://bj.bcebos.com/v1/paddledet/models/ppyoloe_crn_l_80e_sliced_visdrone_640_025.pdparams) | [配置文件](./ppyoloe_crn_l_80e_sliced_visdrone_640_025.yml) |
+
+## 原图评估和拼图评估对比:
+| 模型 | 数据集 | SLICE_SIZE | OVERLAP_RATIO | 类别数 | mAPval
0.5:0.95 | APval
0.5 | 下载链接 | 配置文件 |
+|:---------|:---------------:|:---------------:|:---------------:|:------:|:-----------------------:|:-------------------:|:---------:| :-----: |
+|PP-YOLOE-l| VisDrone-DET| 640 | 0.25 | 10 | 29.7 | 48.5 | [下载链接](https://bj.bcebos.com/v1/paddledet/models/ppyoloe_crn_l_80e_sliced_visdrone_640_025.pdparams) | [配置文件](./ppyoloe_crn_l_80e_sliced_visdrone_640_025.yml) |
+|PP-YOLOE-l (Assembled)| VisDrone-DET| 640 | 0.25 | 10 | 37.2 | 59.4 | [下载链接](https://bj.bcebos.com/v1/paddledet/models/ppyoloe_crn_l_80e_sliced_visdrone_640_025.pdparams) | [配置文件](./ppyoloe_crn_l_80e_sliced_visdrone_640_025.yml) |
**注意:**
-- **SLICE_SIZE**表示使用SAHI工具切图后子图的大小(SLICE_SIZE*SLICE_SIZE);**OVERLAP_RATIO**表示切图重叠率。
+- 使用[SAHI](https://github.com/obss/sahi)切图工具需要首先安装:`pip install sahi`,参考[installation](https://github.com/obss/sahi/blob/main/README.md#installation)。
+- **SLICE_SIZE**表示使用SAHI工具切图后子图的边长大小,**OVERLAP_RATIO**表示切图的子图之间的重叠率,DOTA水平框和Xview数据集均是切图后训练,AP指标为切图后的子图val上的指标。
+- VisDrone-DET数据集请参照[visdrone](../visdrone),可使用原图训练,也可使用切图后训练。
- PP-YOLOE模型训练过程中使用8 GPUs进行混合精度训练,如果**GPU卡数**或者**batch size**发生了改变,你需要按照公式 **lrnew = lrdefault * (batch_sizenew * GPU_numbernew) / (batch_sizedefault * GPU_numberdefault)** 调整学习率。
-- 具体使用教程请参考[ppyoloe](../ppyoloe#getting-start)。
+- 常用训练验证部署等步骤请参考[ppyoloe](../ppyoloe#getting-start)。
+- 自动切图和拼图的推理预测需添加设置`--slice_infer`,具体见下文使用说明。
+- Assembled表示自动切图和拼图。
+
+
+# 使用说明
+
+## 1.训练
+
+首先将你的数据集为COCO数据集格式,然后使用SAHI切图工具进行离线切图,对保存的子图按常规检测模型的训练流程走即可。
+也可直接下载PaddleDetection团队提供的切图后的VisDrone-DET、DOTA水平框、Xview数据集。
+
+执行以下指令使用混合精度训练PP-YOLOE
+
+```bash
+python -m paddle.distributed.launch --gpus 0,1,2,3,4,5,6,7 tools/train.py -c configs/smalldet/ppyoloe_crn_l_80e_sliced_visdrone_640_025.yml --amp --eval
+```
+
+**注意:**
+- 使用默认配置训练需要设置`--amp`以避免显存溢出。
+
+## 2.评估
+
+### 2.1 子图评估:
+
+默认评估方式是子图评估,子图数据集的验证集设置为:
+```
+EvalDataset:
+ !COCODataSet
+ image_dir: val_images_640_025
+ anno_path: val_640_025.json
+ dataset_dir: dataset/visdrone_sliced
+```
+按常规检测模型的评估流程,评估提前切好并存下来的子图上的精度:
+```bash
+CUDA_VISIBLE_DEVICES=0 python tools/eval.py -c configs/smalldet/ppyoloe_crn_l_80e_sliced_visdrone_640_025.yml -o weights=https://paddledet.bj.bcebos.com/models/ppyoloe_crn_l_80e_sliced_visdrone_640_025.pdparams
+```
+
+### 2.2 原图评估:
+修改验证集的标注文件路径为原图标注文件:
+```
+EvalDataset:
+ !COCODataSet
+ image_dir: VisDrone2019-DET-val
+ anno_path: val.json
+ dataset_dir: dataset/visdrone
+```
+直接评估原图上的精度:
+```bash
+CUDA_VISIBLE_DEVICES=0 python tools/eval.py -c configs/smalldet/ppyoloe_crn_l_80e_sliced_visdrone_640_025.yml -o weights=https://paddledet.bj.bcebos.com/models/ppyoloe_crn_l_80e_sliced_visdrone_640_025.pdparams
+```
+
+### 2.3 子图拼图评估:
+修改验证集的标注文件路径为原图标注文件:
+```
+# very slow, preferly eval with a determined weights(xx.pdparams)
+# if you want to eval during training, change SlicedCOCODataSet to COCODataSet and delete sliced_size and overlap_ratio
+EvalDataset:
+ !SlicedCOCODataSet
+ image_dir: VisDrone2019-DET-val
+ anno_path: val.json
+ dataset_dir: dataset/visdrone
+ sliced_size: [640, 640]
+ overlap_ratio: [0.25, 0.25]
+```
+会在评估过程中自动对原图进行切图最后再重组和融合结果来评估原图上的精度:
+```bash
+CUDA_VISIBLE_DEVICES=0 python tools/eval.py -c configs/smalldet/ppyoloe_crn_l_80e_sliced_visdrone_640_025_slice_infer.yml -o weights=https://paddledet.bj.bcebos.com/models/ppyoloe_crn_l_80e_sliced_visdrone_640_025.pdparams --slice_infer --combine_method=nms --match_threshold=0.6 --match_metric=ios
+```
+
+- 设置`--slice_infer`表示切图预测并拼装重组结果,如果不使用则不写;
+- 设置`--slice_size`表示切图的子图尺寸大小,设置`--overlap_ratio`表示子图间重叠率;
+- 设置`--combine_method`表示子图结果重组去重的方式,默认是`nms`;
+- 设置`--match_threshold`表示子图结果重组去重的阈值,默认是0.6;
+- 设置`--match_metric`表示子图结果重组去重的度量标准,默认是`ios`表示交小比(两个框交集面积除以更小框的面积),也可以选择交并比`iou`(两个框交集面积除以并集面积),精度效果因数据集而而异,但选择`ios`预测速度会更快一点;
+
+
+
+**注意:**
+- 设置`--slice_infer`表示切图预测并拼装重组结果,如果不使用则不写,注意需要确保EvalDataset的数据集类是选用的SlicedCOCODataSet而不是COCODataSet;
+- 可以自行修改选择合适的子图尺度sliced_size和子图间重叠率overlap_ratio,如:
+```
+EvalDataset:
+ !SlicedCOCODataSet
+ image_dir: VisDrone2019-DET-val
+ anno_path: val.json
+ dataset_dir: dataset/visdrone
+ sliced_size: [480, 480]
+ overlap_ratio: [0.2, 0.2]
+```
+- 设置`--combine_method`表示子图结果重组去重的方式,默认是`nms`;
+- 设置`--match_threshold`表示子图结果重组去重的阈值,默认是0.6;
+- 设置`--match_metric`表示子图结果重组去重的度量标准,默认是`ios`表示交小比(两个框交集面积除以更小框的面积),也可以选择交并比`iou`(两个框交集面积除以并集面积),精度效果因数据集而而异,但选择`ios`预测速度会更快一点;
+
+
+## 3.预测
+
+### 3.1 子图或原图直接预测:
+与评估流程基本相同,可以在提前切好并存下来的子图上预测,也可以对原图预测,如:
+```bash
+CUDA_VISIBLE_DEVICES=0 python tools/infer.py -c configs/smalldet/ppyoloe_crn_l_80e_sliced_visdrone_640_025.yml -o weights=https://paddledet.bj.bcebos.com/models/ppyoloe_crn_l_80e_sliced_visdrone_640_025.pdparams --infer_img=demo.jpg --draw_threshold=0.25
+```
+
+### 3.2 原图自动切图并拼图预测:
+也可以对原图进行自动切图并拼图重组来预测原图,如:
+```bash
+CUDA_VISIBLE_DEVICES=0 python tools/infer.py -c configs/smalldet/ppyoloe_crn_l_80e_sliced_visdrone_640_025.yml -o weights=https://paddledet.bj.bcebos.com/models/ppyoloe_crn_l_80e_sliced_visdrone_640_025.pdparams --infer_img=demo.jpg --draw_threshold=0.25 --slice_infer --slice_size 640 640 --overlap_ratio 0.25 0.25 --combine_method=nms --match_threshold=0.6 --match_metric=ios
+```
+- 设置`--slice_infer`表示切图预测并拼装重组结果,如果不使用则不写;
+- 设置`--slice_size`表示切图的子图尺寸大小,设置`--overlap_ratio`表示子图间重叠率;
+- 设置`--combine_method`表示子图结果重组去重的方式,默认是`nms`;
+- 设置`--match_threshold`表示子图结果重组去重的阈值,默认是0.6;
+- 设置`--match_metric`表示子图结果重组去重的度量标准,默认是`ios`表示交小比(两个框交集面积除以更小框的面积),也可以选择交并比`iou`(两个框交集面积除以并集面积),精度效果因数据集而而异,但选择`ios`预测速度会更快一点;
+
+
+## 4.部署
+
+### 4.1 导出模型
+```bash
+# export model
+CUDA_VISIBLE_DEVICES=0 python tools/export_model.py -c configs/smalldet/ppyoloe_crn_l_80e_sliced_visdrone_640_025.yml -o weights=https://paddledet.bj.bcebos.com/models/ppyoloe_crn_l_80e_sliced_visdrone_640_025.pdparams
+```
+
+### 4.2 使用原图或子图直接推理:
+```bash
+# deploy infer
+CUDA_VISIBLE_DEVICES=0 python deploy/python/infer.py --model_dir=output_inference/ppyoloe_crn_l_80e_sliced_visdrone_640_025 --image_file=demo.jpg --device=GPU --threshold=0.25
+```
+
+### 4.3 使用原图自动切图并拼图重组结果来推理:
+```bash
+# deploy slice infer
+CUDA_VISIBLE_DEVICES=0 python deploy/python/infer.py --model_dir=output_inference/ppyoloe_crn_l_80e_sliced_visdrone_640_025 --image_file=demo.jpg --device=GPU --threshold=0.25 --slice_infer --slice_size 640 640 --overlap_ratio 0.25 0.25 --combine_method=nms --match_threshold=0.6 --match_metric=ios
+```
+- 设置`--slice_infer`表示切图预测并拼装重组结果,如果不使用则不写;
+- 设置`--slice_size`表示切图的子图尺寸大小,设置`--overlap_ratio`表示子图间重叠率;
+- 设置`--combine_method`表示子图结果重组去重的方式,默认是`nms`;
+- 设置`--match_threshold`表示子图结果重组去重的阈值,默认是0.6;
+- 设置`--match_metric`表示子图结果重组去重的度量标准,默认是`ios`表示交小比(两个框交集面积除以更小框的面积),也可以选择交并比`iou`(两个框交集面积除以并集面积),精度效果因数据集而而异,但选择`ios`预测速度会更快一点;
+
+
+# SAHI切图工具使用说明
+
+## 1. 数据集下载
+
+### VisDrone-DET
+
+VisDrone-DET是一个无人机航拍场景的小目标数据集,整理后的COCO格式VisDrone-DET数据集[下载链接](https://bj.bcebos.com/v1/paddledet/data/smalldet/visdrone.zip),切图后的COCO格式数据集[下载链接](https://bj.bcebos.com/v1/paddledet/data/smalldet/visdrone_sliced.zip),检测其中的**10类**,包括 `pedestrian(1), people(2), bicycle(3), car(4), van(5), truck(6), tricycle(7), awning-tricycle(8), bus(9), motor(10)`,原始数据集[下载链接](https://github.com/VisDrone/VisDrone-Dataset)。
+具体使用和下载请参考[visdrone](../visdrone)。
+
+### DOTA水平框:
+
+DOTA是一个大型的遥感影像公开数据集,这里使用**DOTA-v1.0**水平框数据集,切图后整理的COCO格式的DOTA水平框数据集[下载链接](https://bj.bcebos.com/v1/paddledet/data/smalldet/dota_sliced.zip),检测其中的**15类**,
+包括 `plane(0), baseball-diamond(1), bridge(2), ground-track-field(3), small-vehicle(4), large-vehicle(5), ship(6), tennis-court(7),basketball-court(8), storage-tank(9), soccer-ball-field(10), roundabout(11), harbor(12), swimming-pool(13), helicopter(14)`,
+图片及原始数据集[下载链接](https://captain-whu.github.io/DOAI2019/dataset.html)。
+
+### Xview:
+
+Xview是一个大型的航拍遥感检测数据集,目标极小极多,切图后整理的COCO格式数据集[下载链接](https://bj.bcebos.com/v1/paddledet/data/smalldet/xview_sliced.zip),检测其中的**60类**,
+具体类别为:
+
+
+
+`Fixed-wing Aircraft(0),
+Small Aircraft(1),
+Cargo Plane(2),
+Helicopter(3),
+Passenger Vehicle(4),
+Small Car(5),
+Bus(6),
+Pickup Truck(7),
+Utility Truck(8),
+Truck(9),
+Cargo Truck(10),
+Truck w/Box(11),
+Truck Tractor(12),
+Trailer(13),
+Truck w/Flatbed(14),
+Truck w/Liquid(15),
+Crane Truck(16),
+Railway Vehicle(17),
+Passenger Car(18),
+Cargo Car(19),
+Flat Car(20),
+Tank car(21),
+Locomotive(22),
+Maritime Vessel(23),
+Motorboat(24),
+Sailboat(25),
+Tugboat(26),
+Barge(27),
+Fishing Vessel(28),
+Ferry(29),
+Yacht(30),
+Container Ship(31),
+Oil Tanker(32),
+Engineering Vehicle(33),
+Tower crane(34),
+Container Crane(35),
+Reach Stacker(36),
+Straddle Carrier(37),
+Mobile Crane(38),
+Dump Truck(39),
+Haul Truck(40),
+Scraper/Tractor(41),
+Front loader/Bulldozer(42),
+Excavator(43),
+Cement Mixer(44),
+Ground Grader(45),
+Hut/Tent(46),
+Shed(47),
+Building(48),
+Aircraft Hangar(49),
+Damaged Building(50),
+Facility(51),
+Construction Site(52),
+Vehicle Lot(53),
+Helipad(54),
+Storage Tank(55),
+Shipping container lot(56),
+Shipping Container(57),
+Pylon(58),
+Tower(59)
+`
+
+
+,原始数据集[下载链接](https://challenge.xviewdataset.org/download-links)。
+
+## 2. 统计数据集分布
+
+首先统计所用数据集标注框的平均宽高占图片真实宽高的比例分布:
+
+```bash
+python slice_tools/box_distribution.py --json_path ../../dataset/DOTA/annotations/train.json --out_img box_distribution.jpg
+```
+- `--json_path` :待统计数据集COCO 格式 annotation 的json文件路径
+- `--out_img` :输出的统计分布图路径
+
+以DOTA数据集的train数据集为例,统计结果打印如下:
+```bash
+Median of ratio_w is 0.03799439775910364
+Median of ratio_h is 0.04074914637387802
+all_img with box: 1409
+all_ann: 98905
+Distribution saved as box_distribution.jpg
+```
+
+**注意:**
+- 当原始数据集全部有标注框的图片中,**有1/2以上的图片标注框的平均宽高与原图宽高比例小于0.04时**,建议进行切图训练。
+
+
+## 3. SAHI切图
+
+针对需要切图的数据集,使用[SAHI](https://github.com/obss/sahi)库进行切分:
+
+### 安装SAHI库:
+
+参考[SAHI installation](https://github.com/obss/sahi/blob/main/README.md#installation)进行安装
+
+```bash
+pip install sahi
+```
+
+### 基于SAHI切图:
+
+```bash
+python slice_tools/slice_image.py --image_dir ../../dataset/DOTA/train/ --json_path ../../dataset/DOTA/annotations/train.json --output_dir ../../dataset/dota_sliced --slice_size 500 --overlap_ratio 0.25
+```
+
+- `--image_dir`:原始数据集图片文件夹的路径
+- `--json_path`:原始数据集COCO格式的json标注文件的路径
+- `--output_dir`:切分后的子图及其json标注文件保存的路径
+- `--slice_size`:切分以后子图的边长尺度大小(默认切图后为正方形)
+- `--overlap_ratio`:切分时的子图之间的重叠率
+- 以上述代码为例,切分后的子图文件夹与json标注文件共同保存在`dota_sliced`文件夹下,分别命名为`train_images_500_025`、`train_500_025.json`。
+
+
+# 引用
+```
+@article{akyon2022sahi,
+ title={Slicing Aided Hyper Inference and Fine-tuning for Small Object Detection},
+ author={Akyon, Fatih Cagatay and Altinuc, Sinan Onur and Temizel, Alptekin},
+ journal={arXiv preprint arXiv:2202.06934},
+ year={2022}
+}
+
+@inproceedings{xia2018dota,
+ title={DOTA: A large-scale dataset for object detection in aerial images},
+ author={Xia, Gui-Song and Bai, Xiang and Ding, Jian and Zhu, Zhen and Belongie, Serge and Luo, Jiebo and Datcu, Mihai and Pelillo, Marcello and Zhang, Liangpei},
+ booktitle={Proceedings of the IEEE Conference on Computer Vision and Pattern Recognition},
+ pages={3974--3983},
+ year={2018}
+}
+
+@ARTICLE{9573394,
+ author={Zhu, Pengfei and Wen, Longyin and Du, Dawei and Bian, Xiao and Fan, Heng and Hu, Qinghua and Ling, Haibin},
+ journal={IEEE Transactions on Pattern Analysis and Machine Intelligence},
+ title={Detection and Tracking Meet Drones Challenge},
+ year={2021},
+ volume={},
+ number={},
+ pages={1-1},
+ doi={10.1109/TPAMI.2021.3119563}
+}
+```
diff --git a/configs/smalldet/_base_/DOTA_sliced_500_025_detection.yml b/configs/smalldet/_base_/DOTA_sliced_500_025_detection.yml
index 100d8cbf17ef78e6cc182e14672147c235896be1..d0fc0c389f6ed6e0af1bb9e52406cd2c80205c2c 100644
--- a/configs/smalldet/_base_/DOTA_sliced_500_025_detection.yml
+++ b/configs/smalldet/_base_/DOTA_sliced_500_025_detection.yml
@@ -3,18 +3,18 @@ num_classes: 15
TrainDataset:
!COCODataSet
- image_dir: DOTA_slice_train/train_images_500_025
- anno_path: DOTA_slice_train/train_500_025.json
- dataset_dir: dataset/DOTA
+ image_dir: train_images_500_025
+ anno_path: train_500_025.json
+ dataset_dir: dataset/dota_sliced
data_fields: ['image', 'gt_bbox', 'gt_class', 'is_crowd']
EvalDataset:
!COCODataSet
- image_dir: DOTA_slice_val/val_images_500_025
- anno_path: DOTA_slice_val/val_500_025.json
- dataset_dir: dataset/DOTA
+ image_dir: val_images_500_025
+ anno_path: val_500_025.json
+ dataset_dir: dataset/dota_sliced
TestDataset:
!ImageFolder
- anno_path: dataset/DOTA/DOTA_slice_val/val_500_025.json
- dataset_dir: dataset/DOTA/DOTA_slice_val/val_images_500_025
+ anno_path: val_500_025.json
+ dataset_dir: dataset/dota_sliced
diff --git a/configs/smalldet/_base_/visdrone_sliced_640_025_detection.yml b/configs/smalldet/_base_/visdrone_sliced_640_025_detection.yml
index 2d88b2c00ff5e691cbcda56036a704cbf7cf0a0c..03848ca17549e159d5dab0886c9f83d461c4fdd7 100644
--- a/configs/smalldet/_base_/visdrone_sliced_640_025_detection.yml
+++ b/configs/smalldet/_base_/visdrone_sliced_640_025_detection.yml
@@ -16,5 +16,5 @@ EvalDataset:
TestDataset:
!ImageFolder
- anno_path: dataset/visdrone_sliced/val_640_025.json
- dataset_dir: dataset/visdrone_sliced/val_images_640_025
+ anno_path: val_640_025.json
+ dataset_dir: dataset/visdrone_sliced
diff --git a/configs/smalldet/_base_/xview_sliced_400_025_detection.yml b/configs/smalldet/_base_/xview_sliced_400_025_detection.yml
index b932359db56957b3daca58bbbebc66ff156582b5..c80f545bd7e280b7d97f8ff9e7db25e86162bdf5 100644
--- a/configs/smalldet/_base_/xview_sliced_400_025_detection.yml
+++ b/configs/smalldet/_base_/xview_sliced_400_025_detection.yml
@@ -5,16 +5,16 @@ TrainDataset:
!COCODataSet
image_dir: train_images_400_025
anno_path: train_400_025.json
- dataset_dir: dataset/xview/xview_slic
+ dataset_dir: dataset/xview_sliced
data_fields: ['image', 'gt_bbox', 'gt_class', 'is_crowd']
EvalDataset:
!COCODataSet
image_dir: val_images_400_025
anno_path: val_400_025.json
- dataset_dir: dataset/xview/xview_slic
+ dataset_dir: dataset/xview_sliced
TestDataset:
!ImageFolder
- anno_path: dataset/xview/xview_slic/val_400_025.json
- dataset_dir: dataset/xview/xview_slic/val_images_400_025
+ anno_path: val_400_025.json
+ dataset_dir: dataset/xview_sliced
diff --git a/configs/smalldet/ppyoloe_crn_l_80e_sliced_visdrone_640_025.yml b/configs/smalldet/ppyoloe_crn_l_80e_sliced_visdrone_640_025.yml
index 8d133bb722477c5b56ea2e9e48a1a3f81d155dae..efb573a99b66bec449b360e60beb2dc0ba5648d0 100644
--- a/configs/smalldet/ppyoloe_crn_l_80e_sliced_visdrone_640_025.yml
+++ b/configs/smalldet/ppyoloe_crn_l_80e_sliced_visdrone_640_025.yml
@@ -13,9 +13,14 @@ pretrain_weights: https://paddledet.bj.bcebos.com/models/ppyoloe_crn_l_300e_coco
depth_mult: 1.0
width_mult: 1.0
+
TrainReader:
batch_size: 8
+EvalReader:
+ batch_size: 1
+
+
epoch: 80
LearningRate:
base_lr: 0.01
@@ -34,3 +39,10 @@ PPYOLOEHead:
keep_top_k: 500
score_threshold: 0.01
nms_threshold: 0.6
+
+
+EvalDataset:
+ !COCODataSet
+ image_dir: val_images_640_025
+ anno_path: val_640_025.json
+ dataset_dir: dataset/visdrone_sliced
diff --git a/configs/smalldet/ppyoloe_crn_l_80e_sliced_visdrone_640_025_slice_infer.yml b/configs/smalldet/ppyoloe_crn_l_80e_sliced_visdrone_640_025_slice_infer.yml
new file mode 100644
index 0000000000000000000000000000000000000000..6de6db4c0db8650e5055d067932d11f16d3df54b
--- /dev/null
+++ b/configs/smalldet/ppyoloe_crn_l_80e_sliced_visdrone_640_025_slice_infer.yml
@@ -0,0 +1,52 @@
+_BASE_: [
+ './_base_/visdrone_sliced_640_025_detection.yml',
+ '../runtime.yml',
+ '../ppyoloe/_base_/optimizer_300e.yml',
+ '../ppyoloe/_base_/ppyoloe_crn.yml',
+ '../ppyoloe/_base_/ppyoloe_reader.yml',
+]
+log_iter: 100
+snapshot_epoch: 10
+weights: output/ppyoloe_crn_l_80e_sliced_visdrone_640_025/model_final
+
+pretrain_weights: https://paddledet.bj.bcebos.com/models/ppyoloe_crn_l_300e_coco.pdparams
+depth_mult: 1.0
+width_mult: 1.0
+
+
+TrainReader:
+ batch_size: 8
+
+EvalReader:
+ batch_size: 1 # only support bs=1 when slice infer
+
+
+epoch: 80
+LearningRate:
+ base_lr: 0.01
+ schedulers:
+ - !CosineDecay
+ max_epochs: 96
+ - !LinearWarmup
+ start_factor: 0.
+ epochs: 1
+
+PPYOLOEHead:
+ static_assigner_epoch: -1
+ nms:
+ name: MultiClassNMS
+ nms_top_k: 10000
+ keep_top_k: 500
+ score_threshold: 0.01
+ nms_threshold: 0.6
+
+
+# very slow, preferly eval with a determined weights(xx.pdparams)
+# if you want to eval during training, change SlicedCOCODataSet to COCODataSet and delete sliced_size/overlap_ratio
+EvalDataset:
+ !SlicedCOCODataSet
+ image_dir: VisDrone2019-DET-val
+ anno_path: val.json
+ dataset_dir: dataset/visdrone
+ sliced_size: [640, 640]
+ overlap_ratio: [0.25, 0.25]
diff --git a/configs/smalldet/ppyoloe_crn_l_80e_sliced_DOTA_500_025.yml b/configs/smalldet/ppyoloe_p2_crn_l_80e_sliced_DOTA_500_025.yml
similarity index 73%
rename from configs/smalldet/ppyoloe_crn_l_80e_sliced_DOTA_500_025.yml
rename to configs/smalldet/ppyoloe_p2_crn_l_80e_sliced_DOTA_500_025.yml
index 6a1429d56b1d8118d0763d3c68931f77267d4704..f7bdb583769cc4277d033836010f705539f6dca0 100644
--- a/configs/smalldet/ppyoloe_crn_l_80e_sliced_DOTA_500_025.yml
+++ b/configs/smalldet/ppyoloe_p2_crn_l_80e_sliced_DOTA_500_025.yml
@@ -7,14 +7,27 @@ _BASE_: [
]
log_iter: 100
snapshot_epoch: 10
-weights: output/ppyoloe_crn_l_80e_sliced_DOTA_500_025/model_final
+weights: output/ppyoloe_p2_crn_l_80e_sliced_DOTA_500_025/model_final
pretrain_weights: https://paddledet.bj.bcebos.com/models/ppyoloe_crn_l_300e_coco.pdparams
depth_mult: 1.0
width_mult: 1.0
+
+CSPResNet:
+ return_idx: [0, 1, 2, 3]
+ use_alpha: True
+
+CustomCSPPAN:
+ out_channels: [768, 384, 192, 64]
+
+
TrainReader:
- batch_size: 8
+ batch_size: 4
+
+EvalReader:
+ batch_size: 1
+
epoch: 80
LearningRate:
@@ -27,6 +40,7 @@ LearningRate:
epochs: 1
PPYOLOEHead:
+ fpn_strides: [32, 16, 8, 4]
static_assigner_epoch: -1
nms:
name: MultiClassNMS
diff --git a/configs/smalldet/ppyoloe_crn_l_80e_sliced_xview_400_025.yml b/configs/smalldet/ppyoloe_p2_crn_l_80e_sliced_xview_400_025.yml
similarity index 72%
rename from configs/smalldet/ppyoloe_crn_l_80e_sliced_xview_400_025.yml
rename to configs/smalldet/ppyoloe_p2_crn_l_80e_sliced_xview_400_025.yml
index 7c9d80ea5ba869e13e5eb19450c041eb5350b65d..cbf20b2c23d2cb4990a89ac68af50de8de3176ba 100644
--- a/configs/smalldet/ppyoloe_crn_l_80e_sliced_xview_400_025.yml
+++ b/configs/smalldet/ppyoloe_p2_crn_l_80e_sliced_xview_400_025.yml
@@ -7,14 +7,27 @@ _BASE_: [
]
log_iter: 100
snapshot_epoch: 10
-weights: output/ppyoloe_crn_l_80e_sliced_xview_400_025/model_final
+weights: output/ppyoloe_p2_crn_l_80e_sliced_xview_400_025/model_final
pretrain_weights: https://paddledet.bj.bcebos.com/models/ppyoloe_crn_l_300e_coco.pdparams
depth_mult: 1.0
width_mult: 1.0
+
+CSPResNet:
+ return_idx: [0, 1, 2, 3]
+ use_alpha: True
+
+CustomCSPPAN:
+ out_channels: [768, 384, 192, 64]
+
+
TrainReader:
- batch_size: 8
+ batch_size: 4
+
+EvalReader:
+ batch_size: 1
+
epoch: 80
LearningRate:
@@ -27,6 +40,7 @@ LearningRate:
epochs: 1
PPYOLOEHead:
+ fpn_strides: [32, 16, 8, 4]
static_assigner_epoch: -1
nms:
name: MultiClassNMS
diff --git a/configs/visdrone/README.md b/configs/visdrone/README.md
index 8fb78190c8fcb73163bc9674d42a4b7ab2673e85..04de6870b618040d631fa99701d7bd88b50796b6 100644
--- a/configs/visdrone/README.md
+++ b/configs/visdrone/README.md
@@ -18,11 +18,13 @@ PaddleDetection团队提供了针对VisDrone-DET小目标数航拍场景的基
|PP-YOLOE-Alpha-largesize-l| 41.9 | 65.0 | 32.3 | 53.0 | 37.13 | 61.15 | [下载链接](https://paddledet.bj.bcebos.com/models/ppyoloe_crn_l_alpha_largesize_80e_visdrone.pdparams) | [配置文件](./ppyoloe_crn_l_alpha_largesize_80e_visdrone.yml) |
|PP-YOLOE-P2-Alpha-largesize-l| 41.3 | 64.5 | 32.4 | 53.1 | 37.49 | 51.54 | [下载链接](https://paddledet.bj.bcebos.com/models/ppyoloe_crn_l_p2_alpha_largesize_80e_visdrone.pdparams) | [配置文件](./ppyoloe_crn_l_p2_alpha_largesize_80e_visdrone.yml) |
-## 切图训练:
-| 模型 | COCOAPI mAPval
0.5:0.95 | COCOAPI mAPval
0.5 | COCOAPI mAPtest_dev
0.5:0.95 | COCOAPI mAPtest_dev
0.5 | MatlabAPI mAPtest_dev
0.5:0.95 | MatlabAPI mAPtest_dev
0.5 | 下载 | 配置文件 |
-|:---------|:------:|:------:| :----: | :------:| :------: | :------:| :----: | :------:|
-|PP-YOLOE-l| 29.7 | 48.5 | 23.3 | 39.9 | - | - | [下载链接](https://paddledet.bj.bcebos.com/models/ppyoloe_crn_l_80e_sliced_visdrone_640_025.pdparams) | [配置文件](../smalldet/ppyoloe_crn_l_80e_sliced_visdrone_640_025.yml) |
+## 原图评估和拼图评估对比:
+
+| 模型 | 数据集 | SLICE_SIZE | OVERLAP_RATIO | 类别数 | mAPval
0.5:0.95 | APval
0.5 | 下载链接 | 配置文件 |
+|:---------|:---------------:|:---------------:|:---------------:|:------:|:-----------------------:|:-------------------:|:---------:| :-----: |
+|PP-YOLOE-l| VisDrone-DET| 640 | 0.25 | 10 | 29.7 | 48.5 | [下载链接](https://bj.bcebos.com/v1/paddledet/models/ppyoloe_crn_l_80e_sliced_visdrone_640_025.pdparams) | [配置文件](../smalldet/ppyoloe_crn_l_80e_sliced_visdrone_640_025.yml) |
+|PP-YOLOE-l (Assembled)| VisDrone-DET| 640 | 0.25 | 10 | 37.2 | 59.4 | [下载链接](https://bj.bcebos.com/v1/paddledet/models/ppyoloe_crn_l_80e_sliced_visdrone_640_025.pdparams) | [配置文件](../smalldet/ppyoloe_crn_l_80e_sliced_visdrone_640_025.yml) |
**注意:**
diff --git a/configs/visdrone/ppyoloe_crn_l_80e_visdrone.yml b/configs/visdrone/ppyoloe_crn_l_80e_visdrone.yml
index 4a51e696ac6684adcaff42d5b26033d01413ca68..93f8b40c8811f6a83596050547d4f631c27bbc8c 100644
--- a/configs/visdrone/ppyoloe_crn_l_80e_visdrone.yml
+++ b/configs/visdrone/ppyoloe_crn_l_80e_visdrone.yml
@@ -13,9 +13,14 @@ pretrain_weights: https://paddledet.bj.bcebos.com/models/ppyoloe_crn_l_300e_coco
depth_mult: 1.0
width_mult: 1.0
+
TrainReader:
batch_size: 8
+EvalReader:
+ batch_size: 1
+
+
epoch: 80
LearningRate:
base_lr: 0.01
diff --git a/configs/visdrone/ppyoloe_crn_l_alpha_largesize_80e_visdrone.yml b/configs/visdrone/ppyoloe_crn_l_alpha_largesize_80e_visdrone.yml
index 998f0fcb5344eb33574dd24a51f2753fb4dd1831..dcea2687abdc99536e76d44b4d773c5424d30a1c 100644
--- a/configs/visdrone/ppyoloe_crn_l_alpha_largesize_80e_visdrone.yml
+++ b/configs/visdrone/ppyoloe_crn_l_alpha_largesize_80e_visdrone.yml
@@ -1,6 +1,8 @@
_BASE_: [
'ppyoloe_crn_l_80e_visdrone.yml',
]
+log_iter: 100
+snapshot_epoch: 10
weights: output/ppyoloe_crn_l_alpha_largesize_80e_visdrone/model_final
pretrain_weights: https://paddledet.bj.bcebos.com/models/ppyoloe_crn_l_300e_coco.pdparams
@@ -42,7 +44,7 @@ EvalReader:
- Resize: {target_size: *eval_size, keep_ratio: False, interp: 2}
- NormalizeImage: {mean: [0.485, 0.456, 0.406], std: [0.229, 0.224, 0.225], is_scale: True}
- Permute: {}
- batch_size: 2
+ batch_size: 1
TestReader:
inputs_def:
diff --git a/configs/visdrone/ppyoloe_crn_l_p2_alpha_80e_visdrone.yml b/configs/visdrone/ppyoloe_crn_l_p2_alpha_80e_visdrone.yml
index 718f02903bf4069366910a302e561f68aafc3a62..cc0b5440c9db3ddd7f9fdb2e43e8c710a0d356aa 100644
--- a/configs/visdrone/ppyoloe_crn_l_p2_alpha_80e_visdrone.yml
+++ b/configs/visdrone/ppyoloe_crn_l_p2_alpha_80e_visdrone.yml
@@ -1,6 +1,8 @@
_BASE_: [
'ppyoloe_crn_l_80e_visdrone.yml',
]
+log_iter: 100
+snapshot_epoch: 10
weights: output/ppyoloe_crn_l_p2_alpha_80e_visdrone/model_final
pretrain_weights: https://paddledet.bj.bcebos.com/models/ppyoloe_crn_l_300e_coco.pdparams
@@ -8,6 +10,10 @@ pretrain_weights: https://paddledet.bj.bcebos.com/models/ppyoloe_crn_l_300e_coco
TrainReader:
batch_size: 4
+EvalReader:
+ batch_size: 1
+
+
LearningRate:
base_lr: 0.005
diff --git a/configs/visdrone/ppyoloe_crn_l_p2_alpha_largesize_80e_visdrone.yml b/configs/visdrone/ppyoloe_crn_l_p2_alpha_largesize_80e_visdrone.yml
index 1cd8dc671dd5f112742126a434d83a9196853a0f..5aca08856a8c6935fcf2fef216103f027aaf2509 100644
--- a/configs/visdrone/ppyoloe_crn_l_p2_alpha_largesize_80e_visdrone.yml
+++ b/configs/visdrone/ppyoloe_crn_l_p2_alpha_largesize_80e_visdrone.yml
@@ -1,6 +1,8 @@
_BASE_: [
'ppyoloe_crn_l_80e_visdrone.yml',
]
+log_iter: 100
+snapshot_epoch: 10
weights: output/ppyoloe_crn_l_p2_alpha_largesize_80e_visdrone/model_final
pretrain_weights: https://paddledet.bj.bcebos.com/models/ppyoloe_crn_l_300e_coco.pdparams
@@ -49,7 +51,7 @@ EvalReader:
- Resize: {target_size: *eval_size, keep_ratio: False, interp: 2}
- NormalizeImage: {mean: [0.485, 0.456, 0.406], std: [0.229, 0.224, 0.225], is_scale: True}
- Permute: {}
- batch_size: 2
+ batch_size: 1
TestReader:
inputs_def:
diff --git a/configs/visdrone/ppyoloe_crn_s_80e_visdrone.yml b/configs/visdrone/ppyoloe_crn_s_80e_visdrone.yml
index db3d93d628f8754aac3be50060f17a14c4dda04d..555dab200d4d6a4a712f0dbe0f27ce0152d7a959 100644
--- a/configs/visdrone/ppyoloe_crn_s_80e_visdrone.yml
+++ b/configs/visdrone/ppyoloe_crn_s_80e_visdrone.yml
@@ -13,9 +13,14 @@ pretrain_weights: https://paddledet.bj.bcebos.com/models/ppyoloe_crn_s_300e_coco
depth_mult: 0.33
width_mult: 0.50
+
TrainReader:
batch_size: 8
+EvalReader:
+ batch_size: 1
+
+
epoch: 80
LearningRate:
base_lr: 0.01
diff --git a/configs/visdrone/ppyoloe_crn_s_p2_alpha_80e_visdrone.yml b/configs/visdrone/ppyoloe_crn_s_p2_alpha_80e_visdrone.yml
index 17d6299bb89e6e70dd420b0ec01743ae26c2af8c..d82d25b98eceb04afd83caa36d4ae30767956665 100644
--- a/configs/visdrone/ppyoloe_crn_s_p2_alpha_80e_visdrone.yml
+++ b/configs/visdrone/ppyoloe_crn_s_p2_alpha_80e_visdrone.yml
@@ -1,13 +1,19 @@
_BASE_: [
'ppyoloe_crn_s_80e_visdrone.yml',
]
+log_iter: 100
+snapshot_epoch: 10
weights: output/ppyoloe_crn_s_p2_alpha_80e_visdrone/model_final
+pretrain_weights: https://paddledet.bj.bcebos.com/models/ppyoloe_crn_s_300e_coco.pdparams
-pretrain_weights: https://paddledet.bj.bcebos.com/models/ppyoloe_crn_l_300e_coco.pdparams
TrainReader:
batch_size: 4
+EvalReader:
+ batch_size: 1
+
+
LearningRate:
base_lr: 0.005
diff --git a/deploy/python/infer.py b/deploy/python/infer.py
index a2199a2be62f04af5e1e940704a1dce426596f46..0b385f81703e8df7d82163d0efecc8dc858557f0 100644
--- a/deploy/python/infer.py
+++ b/deploy/python/infer.py
@@ -36,7 +36,7 @@ from picodet_postprocess import PicoDetPostProcess
from preprocess import preprocess, Resize, NormalizeImage, Permute, PadStride, LetterBoxResize, WarpAffine, Pad, decode_image
from keypoint_preprocess import EvalAffine, TopDownEvalAffine, expand_crop
from visualize import visualize_box_mask
-from utils import argsparser, Timer, get_current_memory_mb
+from utils import argsparser, Timer, get_current_memory_mb, multiclass_nms
# Global dictionary
SUPPORT_MODELS = {
@@ -218,6 +218,93 @@ class Detector(object):
def get_timer(self):
return self.det_times
+ def predict_image_slice(self,
+ img_list,
+ slice_size=[640, 640],
+ overlap_ratio=[0.25, 0.25],
+ combine_method='nms',
+ match_threshold=0.6,
+ match_metric='iou',
+ visual=True,
+ save_file=None):
+ # slice infer only support bs=1
+ results = []
+ try:
+ import sahi
+ from sahi.slicing import slice_image
+ except Exception as e:
+ logger.error(
+ 'sahi not found, plaese install sahi. '
+ 'for example: `pip install sahi`, see https://github.com/obss/sahi.'
+ )
+ raise e
+ num_classes = len(self.pred_config.labels)
+ for i in range(len(img_list)):
+ ori_image = img_list[i]
+ slice_image_result = sahi.slicing.slice_image(
+ image=ori_image,
+ slice_height=slice_size[0],
+ slice_width=slice_size[1],
+ overlap_height_ratio=overlap_ratio[0],
+ overlap_width_ratio=overlap_ratio[1])
+ sub_img_num = len(slice_image_result)
+ merged_bboxs = []
+ for _ind in range(sub_img_num):
+ im = slice_image_result.images[_ind]
+ self.det_times.preprocess_time_s.start()
+ inputs = self.preprocess([im]) # should be list
+ self.det_times.preprocess_time_s.end()
+
+ # model prediction
+ self.det_times.inference_time_s.start()
+ result = self.predict()
+ self.det_times.inference_time_s.end()
+
+ # postprocess
+ self.det_times.postprocess_time_s.start()
+ result = self.postprocess(inputs, result)
+ self.det_times.postprocess_time_s.end()
+ self.det_times.img_num += 1
+
+ shift_amount = slice_image_result.starting_pixels[_ind]
+ result['boxes'][:, 2:4] = result['boxes'][:, 2:4] + shift_amount
+ result['boxes'][:, 4:6] = result['boxes'][:, 4:6] + shift_amount
+ merged_bboxs.append(result['boxes'])
+
+ merged_results = {'boxes': []}
+ if combine_method == 'nms':
+ final_boxes = multiclass_nms(
+ np.concatenate(merged_bboxs), num_classes, match_threshold,
+ match_metric)
+ merged_results['boxes'] = np.concatenate(final_boxes)
+ elif combine_method == 'concat':
+ merged_results['boxes'] = np.concatenate(merged_bboxs)
+ else:
+ raise ValueError(
+ "Now only support 'nms' or 'concat' to fuse detection results."
+ )
+ merged_results['boxes_num'] = np.array(
+ [len(merged_results['boxes'])], dtype=np.int32)
+
+ if visual:
+ visualize(
+ [ori_image], # should be list
+ merged_results,
+ self.pred_config.labels,
+ output_dir=self.output_dir,
+ threshold=self.threshold)
+
+ results.append(merged_results)
+ if visual:
+ print('Test iter {}'.format(i))
+
+ if save_file is not None:
+ Path(self.output_dir).mkdir(exist_ok=True)
+ self.format_coco_results(image_list, results, save_file=save_file)
+
+ results = self.merge_batch_result(results)
+ return results
+
def predict_image(self,
image_list,
run_benchmark=False,
@@ -871,8 +958,18 @@ def main():
img_list = get_test_images(FLAGS.image_dir, FLAGS.image_file)
save_file = os.path.join(FLAGS.output_dir,
'results.json') if FLAGS.save_results else None
- detector.predict_image(
- img_list, FLAGS.run_benchmark, repeats=100, save_file=save_file)
+ if FLAGS.slice_infer:
+ detector.predict_image_slice(
+ img_list,
+ FLAGS.slice_size,
+ FLAGS.overlap_ratio,
+ FLAGS.combine_method,
+ FLAGS.match_threshold,
+ FLAGS.match_metric,
+ save_file=save_file)
+ else:
+ detector.predict_image(
+ img_list, FLAGS.run_benchmark, repeats=100, save_file=save_file)
if not FLAGS.run_benchmark:
detector.det_times.info(average=True)
else:
diff --git a/deploy/python/utils.py b/deploy/python/utils.py
index 41dc7ae9e81f49bdd08e0917d50b21ac00f2e527..fb166f7705c1eb56640624620125fb5219479820 100644
--- a/deploy/python/utils.py
+++ b/deploy/python/utils.py
@@ -16,6 +16,7 @@ import time
import os
import ast
import argparse
+import numpy as np
def argsparser():
@@ -161,7 +162,39 @@ def argsparser():
type=bool,
default=False,
help="Whether save detection result to file using coco format")
-
+ parser.add_argument(
+ "--slice_infer",
+ action='store_true',
+ help="Whether to slice the image and merge the inference results for small object detection."
+ )
+ parser.add_argument(
+ '--slice_size',
+ nargs='+',
+ type=int,
+ default=[640, 640],
+ help="Height of the sliced image.")
+ parser.add_argument(
+ "--overlap_ratio",
+ nargs='+',
+ type=float,
+ default=[0.25, 0.25],
+ help="Overlap height ratio of the sliced image.")
+ parser.add_argument(
+ "--combine_method",
+ type=str,
+ default='nms',
+ help="Combine method of the sliced images' detection results, choose in ['nms', 'nmm', 'concat']."
+ )
+ parser.add_argument(
+ "--match_threshold",
+ type=float,
+ default=0.6,
+ help="Combine method matching threshold.")
+ parser.add_argument(
+ "--match_metric",
+ type=str,
+ default='iou',
+ help="Combine method matching metric, choose in ['iou', 'ios'].")
return parser
@@ -288,3 +321,68 @@ def get_current_memory_mb():
meminfo = pynvml.nvmlDeviceGetMemoryInfo(handle)
gpu_mem = meminfo.used / 1024. / 1024.
return round(cpu_mem, 4), round(gpu_mem, 4), round(gpu_percent, 4)
+
+
+def multiclass_nms(bboxs, num_classes, match_threshold=0.6, match_metric='iou'):
+ final_boxes = []
+ for c in range(num_classes):
+ idxs = bboxs[:, 0] == c
+ if np.count_nonzero(idxs) == 0: continue
+ r = nms(bboxs[idxs, 1:], match_threshold, match_metric)
+ final_boxes.append(np.concatenate([np.full((r.shape[0], 1), c), r], 1))
+ return final_boxes
+
+
+def nms(dets, match_threshold=0.6, match_metric='iou'):
+ """ Apply NMS to avoid detecting too many overlapping bounding boxes.
+ Args:
+ dets: shape [N, 5], [score, x1, y1, x2, y2]
+ match_metric: 'iou' or 'ios'
+ match_threshold: overlap thresh for match metric.
+ """
+ if dets.shape[0] == 0:
+ return dets[[], :]
+ scores = dets[:, 0]
+ x1 = dets[:, 1]
+ y1 = dets[:, 2]
+ x2 = dets[:, 3]
+ y2 = dets[:, 4]
+ areas = (x2 - x1 + 1) * (y2 - y1 + 1)
+ order = scores.argsort()[::-1]
+
+ ndets = dets.shape[0]
+ suppressed = np.zeros((ndets), dtype=np.int)
+
+ for _i in range(ndets):
+ i = order[_i]
+ if suppressed[i] == 1:
+ continue
+ ix1 = x1[i]
+ iy1 = y1[i]
+ ix2 = x2[i]
+ iy2 = y2[i]
+ iarea = areas[i]
+ for _j in range(_i + 1, ndets):
+ j = order[_j]
+ if suppressed[j] == 1:
+ continue
+ xx1 = max(ix1, x1[j])
+ yy1 = max(iy1, y1[j])
+ xx2 = min(ix2, x2[j])
+ yy2 = min(iy2, y2[j])
+ w = max(0.0, xx2 - xx1 + 1)
+ h = max(0.0, yy2 - yy1 + 1)
+ inter = w * h
+ if match_metric == 'iou':
+ union = iarea + areas[j] - inter
+ match_value = inter / union
+ elif match_metric == 'ios':
+ smaller = min(iarea, areas[j])
+ match_value = inter / smaller
+ else:
+ raise ValueError()
+ if match_value >= match_threshold:
+ suppressed[j] = 1
+ keep = np.where(suppressed == 0)[0]
+ dets = dets[keep, :]
+ return dets
diff --git a/ppdet/data/source/coco.py b/ppdet/data/source/coco.py
index 5f226ec0a71b57bb2e8dddd46da1f96d7a0097ff..95a51deeb82a4679d6d658518354afd91abefa52 100644
--- a/ppdet/data/source/coco.py
+++ b/ppdet/data/source/coco.py
@@ -253,3 +253,126 @@ class COCODataSet(DetDataset):
empty_records = self._sample_empty(empty_records, len(records))
records += empty_records
self.roidbs = records
+
+
+@register
+@serializable
+class SlicedCOCODataSet(COCODataSet):
+ """Sliced COCODataSet"""
+
+ def __init__(
+ self,
+ dataset_dir=None,
+ image_dir=None,
+ anno_path=None,
+ data_fields=['image'],
+ sample_num=-1,
+ load_crowd=False,
+ allow_empty=False,
+ empty_ratio=1.,
+ repeat=1,
+ sliced_size=[640, 640],
+ overlap_ratio=[0.25, 0.25], ):
+ super(SlicedCOCODataSet, self).__init__(
+ dataset_dir=dataset_dir,
+ image_dir=image_dir,
+ anno_path=anno_path,
+ data_fields=data_fields,
+ sample_num=sample_num,
+ load_crowd=load_crowd,
+ allow_empty=allow_empty,
+ empty_ratio=empty_ratio,
+ repeat=repeat, )
+ self.sliced_size = sliced_size
+ self.overlap_ratio = overlap_ratio
+
+ def parse_dataset(self):
+ anno_path = os.path.join(self.dataset_dir, self.anno_path)
+ image_dir = os.path.join(self.dataset_dir, self.image_dir)
+
+ assert anno_path.endswith('.json'), \
+ 'invalid coco annotation file: ' + anno_path
+ from pycocotools.coco import COCO
+ coco = COCO(anno_path)
+ img_ids = coco.getImgIds()
+ img_ids.sort()
+ cat_ids = coco.getCatIds()
+ records = []
+ empty_records = []
+ ct = 0
+ ct_sub = 0
+
+ self.catid2clsid = dict({catid: i for i, catid in enumerate(cat_ids)})
+ self.cname2cid = dict({
+ coco.loadCats(catid)[0]['name']: clsid
+ for catid, clsid in self.catid2clsid.items()
+ })
+
+ if 'annotations' not in coco.dataset:
+ self.load_image_only = True
+ logger.warning('Annotation file: {} does not contains ground truth '
+ 'and load image information only.'.format(anno_path))
+ try:
+ import sahi
+ from sahi.slicing import slice_image
+ except Exception as e:
+ logger.error(
+ 'sahi not found, plaese install sahi. '
+ 'for example: `pip install sahi`, see https://github.com/obss/sahi.'
+ )
+ raise e
+
+ sub_img_ids = 0
+ for img_id in img_ids:
+ img_anno = coco.loadImgs([img_id])[0]
+ im_fname = img_anno['file_name']
+ im_w = float(img_anno['width'])
+ im_h = float(img_anno['height'])
+
+ im_path = os.path.join(image_dir,
+ im_fname) if image_dir else im_fname
+ is_empty = False
+ if not os.path.exists(im_path):
+ logger.warning('Illegal image file: {}, and it will be '
+ 'ignored'.format(im_path))
+ continue
+
+ if im_w < 0 or im_h < 0:
+ logger.warning('Illegal width: {} or height: {} in annotation, '
+ 'and im_id: {} will be ignored'.format(
+ im_w, im_h, img_id))
+ continue
+
+ slice_image_result = sahi.slicing.slice_image(
+ image=im_path,
+ slice_height=self.sliced_size[0],
+ slice_width=self.sliced_size[1],
+ overlap_height_ratio=self.overlap_ratio[0],
+ overlap_width_ratio=self.overlap_ratio[1])
+
+ sub_img_num = len(slice_image_result)
+ for _ind in range(sub_img_num):
+ im = slice_image_result.images[_ind]
+ coco_rec = {
+ 'image': im,
+ 'im_id': np.array([sub_img_ids + _ind]),
+ 'h': im.shape[0],
+ 'w': im.shape[1],
+ 'ori_im_id': np.array([img_id]),
+ 'st_pix': np.array(
+ slice_image_result.starting_pixels[_ind],
+ dtype=np.float32),
+ 'is_last': 1 if _ind == sub_img_num - 1 else 0,
+ } if 'image' in self.data_fields else {}
+ records.append(coco_rec)
+ ct_sub += sub_img_num
+ ct += 1
+ if self.sample_num > 0 and ct >= self.sample_num:
+ break
+ assert ct > 0, 'not found any coco record in %s' % (anno_path)
+ logger.info('{} samples and slice to {} sub_samples in file {}'.format(
+ ct, ct_sub, anno_path))
+ if self.allow_empty and len(empty_records) > 0:
+ empty_records = self._sample_empty(empty_records, len(records))
+ records += empty_records
+ self.roidbs = records
diff --git a/ppdet/data/source/dataset.py b/ppdet/data/source/dataset.py
index d735cfc4a2ac2b709e74cb797a61832d70bd9a51..4f54494a3adfad7dd835b77603bb6a5133066570 100644
--- a/ppdet/data/source/dataset.py
+++ b/ppdet/data/source/dataset.py
@@ -206,6 +206,55 @@ class ImageFolder(DetDataset):
self.image_dir = images
self.roidbs = self._load_images()
+ def set_slice_images(self,
+ images,
+ slice_size=[640, 640],
+ overlap_ratio=[0.25, 0.25]):
+ self.image_dir = images
+ ori_records = self._load_images()
+ try:
+ import sahi
+ from sahi.slicing import slice_image
+ except Exception as e:
+ logger.error(
+ 'sahi not found, plaese install sahi. '
+ 'for example: `pip install sahi`, see https://github.com/obss/sahi.'
+ )
+ raise e
+
+ sub_img_ids = 0
+ ct = 0
+ ct_sub = 0
+ records = []
+ for i, ori_rec in enumerate(ori_records):
+ im_path = ori_rec['im_file']
+ slice_image_result = sahi.slicing.slice_image(
+ image=im_path,
+ slice_height=slice_size[0],
+ slice_width=slice_size[1],
+ overlap_height_ratio=overlap_ratio[0],
+ overlap_width_ratio=overlap_ratio[1])
+
+ sub_img_num = len(slice_image_result)
+ for _ind in range(sub_img_num):
+ im = slice_image_result.images[_ind]
+ rec = {
+ 'image': im,
+ 'im_id': np.array([sub_img_ids + _ind]),
+ 'h': im.shape[0],
+ 'w': im.shape[1],
+ 'ori_im_id': np.array([ori_rec['im_id'][0]]),
+ 'st_pix': np.array(
+ slice_image_result.starting_pixels[_ind],
+ dtype=np.float32),
+ 'is_last': 1 if _ind == sub_img_num - 1 else 0,
+ } if 'image' in self.data_fields else {}
+ records.append(rec)
+ ct_sub += sub_img_num
+ ct += 1
+ print('{} samples and slice to {} sub_samples'.format(ct, ct_sub))
+ self.roidbs = records
+
def get_label_list(self):
# Only VOC dataset needs label list in ImageFold
return self.anno_path
diff --git a/ppdet/data/transform/operators.py b/ppdet/data/transform/operators.py
index ec4ef2dc9cb61ec5e1bad6a5505959264cca7c3a..ad0bfdf2ad72035291d9111c4be2ba18b0615621 100644
--- a/ppdet/data/transform/operators.py
+++ b/ppdet/data/transform/operators.py
@@ -122,12 +122,15 @@ class Decode(BaseOperator):
sample['image'] = f.read()
sample.pop('im_file')
- im = sample['image']
- data = np.frombuffer(im, dtype='uint8')
- im = cv2.imdecode(data, 1) # BGR mode, but need RGB mode
- if 'keep_ori_im' in sample and sample['keep_ori_im']:
- sample['ori_image'] = im
- im = cv2.cvtColor(im, cv2.COLOR_BGR2RGB)
+ try:
+ im = sample['image']
+ data = np.frombuffer(im, dtype='uint8')
+ im = cv2.imdecode(data, 1) # BGR mode, but need RGB mode
+ if 'keep_ori_im' in sample and sample['keep_ori_im']:
+ sample['ori_image'] = im
+ im = cv2.cvtColor(im, cv2.COLOR_BGR2RGB)
+ except:
+ im = sample['image']
sample['image'] = im
if 'h' not in sample:
diff --git a/ppdet/engine/trainer.py b/ppdet/engine/trainer.py
index c253b40aa58a32f2b8cbe89d902480a3a537e1e9..803306e284cd03fd171566cbfa45bac48a7a034a 100644
--- a/ppdet/engine/trainer.py
+++ b/ppdet/engine/trainer.py
@@ -45,6 +45,7 @@ from ppdet.data.source.category import get_categories
import ppdet.utils.stats as stats
from ppdet.utils.fuse_utils import fuse_conv_bn
from ppdet.utils import profiler
+from ppdet.modeling.post_process import multiclass_nms
from .callbacks import Callback, ComposeCallback, LogPrinter, Checkpointer, WiferFaceEval, VisualDLWriter, SniperProposalsGenerator, WandbCallback
from .export_utils import _dump_infer_config, _prune_input_spec
@@ -617,6 +618,194 @@ class Trainer(object):
with paddle.no_grad():
self._eval_with_loader(self.loader)
+ def _eval_with_loader_slice(self,
+ loader,
+ slice_size=[640, 640],
+ overlap_ratio=[0.25, 0.25],
+ combine_method='nms',
+ match_threshold=0.6,
+ match_metric='iou'):
+ sample_num = 0
+ tic = time.time()
+ self._compose_callback.on_epoch_begin(self.status)
+ self.status['mode'] = 'eval'
+ self.model.eval()
+ if self.cfg.get('print_flops', False):
+ flops_loader = create('{}Reader'.format(self.mode.capitalize()))(
+ self.dataset, self.cfg.worker_num, self._eval_batch_sampler)
+ self._flops(flops_loader)
+
+ merged_bboxs = []
+ for step_id, data in enumerate(loader):
+ self.status['step_id'] = step_id
+ self._compose_callback.on_step_begin(self.status)
+ # forward
+ if self.use_amp:
+ with paddle.amp.auto_cast(
+ enable=self.cfg.use_gpu,
+ custom_white_list=self.custom_white_list,
+ custom_black_list=self.custom_black_list,
+ level=self.amp_level):
+ outs = self.model(data)
+ else:
+ outs = self.model(data)
+
+ shift_amount = data['st_pix']
+ outs['bbox'][:, 2:4] = outs['bbox'][:, 2:4] + shift_amount
+ outs['bbox'][:, 4:6] = outs['bbox'][:, 4:6] + shift_amount
+ merged_bboxs.append(outs['bbox'])
+
+ if data['is_last'] > 0:
+ # merge matching predictions
+ merged_results = {'bbox': []}
+ if combine_method == 'nms':
+ final_boxes = multiclass_nms(
+ np.concatenate(merged_bboxs), self.cfg.num_classes,
+ match_threshold, match_metric)
+ merged_results['bbox'] = np.concatenate(final_boxes)
+ elif combine_method == 'concat':
+ merged_results['bbox'] = np.concatenate(merged_bboxs)
+ else:
+ raise ValueError(
+ "Now only support 'nms' or 'concat' to fuse detection results."
+ )
+ merged_results['im_id'] = np.array([[0]])
+ merged_results['bbox_num'] = np.array(
+ [len(merged_results['bbox'])])
+
+ merged_bboxs = []
+ data['im_id'] = data['ori_im_id']
+ # update metrics
+ for metric in self._metrics:
+ metric.update(data, merged_results)
+
+ # multi-scale inputs: all inputs have same im_id
+ if isinstance(data, typing.Sequence):
+ sample_num += data[0]['im_id'].numpy().shape[0]
+ else:
+ sample_num += data['im_id'].numpy().shape[0]
+
+ self._compose_callback.on_step_end(self.status)
+
+ self.status['sample_num'] = sample_num
+ self.status['cost_time'] = time.time() - tic
+
+ # accumulate metric to log out
+ for metric in self._metrics:
+ metric.accumulate()
+ metric.log()
+ self._compose_callback.on_epoch_end(self.status)
+ # reset metric states for metric may performed multiple times
+ self._reset_metrics()
+
+ def evaluate_slice(self,
+ slice_size=[640, 640],
+ overlap_ratio=[0.25, 0.25],
+ combine_method='nms',
+ match_threshold=0.6,
+ match_metric='iou'):
+ with paddle.no_grad():
+ self._eval_with_loader_slice(self.loader, slice_size, overlap_ratio,
+ combine_method, match_threshold,
+ match_metric)
+
+ def slice_predict(self,
+ images,
+ slice_size=[640, 640],
+ overlap_ratio=[0.25, 0.25],
+ combine_method='nms',
+ match_threshold=0.6,
+ match_metric='iou',
+ draw_threshold=0.5,
+ output_dir='output',
+ save_results=False):
+ self.dataset.set_slice_images(images, slice_size, overlap_ratio)
+ loader = create('TestReader')(self.dataset, 0)
+
+ imid2path = self.dataset.get_imid2path()
+
+ anno_file = self.dataset.get_anno()
+ clsid2catid, catid2name = get_categories(
+ self.cfg.metric, anno_file=anno_file)
+
+ # Run Infer
+ self.status['mode'] = 'test'
+ self.model.eval()
+ if self.cfg.get('print_flops', False):
+ flops_loader = create('TestReader')(self.dataset, 0)
+ self._flops(flops_loader)
+
+ results = [] # all images
+ merged_bboxs = [] # single image
+ for step_id, data in enumerate(tqdm(loader)):
+ self.status['step_id'] = step_id
+ # forward
+ outs = self.model(data)
+
+ outs['bbox'] = outs['bbox'].numpy() # only in test mode
+ shift_amount = data['st_pix']
+ outs['bbox'][:, 2:4] = outs['bbox'][:, 2:4] + shift_amount.numpy()
+ outs['bbox'][:, 4:6] = outs['bbox'][:, 4:6] + shift_amount.numpy()
+ merged_bboxs.append(outs['bbox'])
+
+ if data['is_last'] > 0:
+ # merge matching predictions
+ merged_results = {'bbox': []}
+ if combine_method == 'nms':
+ final_boxes = multiclass_nms(
+ np.concatenate(merged_bboxs), self.cfg.num_classes,
+ match_threshold, match_metric)
+ merged_results['bbox'] = np.concatenate(final_boxes)
+ elif combine_method == 'concat':
+ merged_results['bbox'] = np.concatenate(merged_bboxs)
+ else:
+ raise ValueError(
+ "Now only support 'nms' or 'concat' to fuse detection results."
+ )
+ merged_results['im_id'] = np.array([[0]])
+ merged_results['bbox_num'] = np.array(
+ [len(merged_results['bbox'])])
+
+ merged_bboxs = []
+ data['im_id'] = data['ori_im_id']
+
+ for key in ['im_shape', 'scale_factor', 'im_id']:
+ if isinstance(data, typing.Sequence):
+ outs[key] = data[0][key]
+ else:
+ outs[key] = data[key]
+ for key, value in merged_results.items():
+ if hasattr(value, 'numpy'):
+ merged_results[key] = value.numpy()
+ results.append(merged_results)
+
+ # visualize results
+ for outs in results:
+ batch_res = get_infer_results(outs, clsid2catid)
+ bbox_num = outs['bbox_num']
+ start = 0
+ for i, im_id in enumerate(outs['im_id']):
+ image_path = imid2path[int(im_id)]
+ image = Image.open(image_path).convert('RGB')
+ image = ImageOps.exif_transpose(image)
+ self.status['original_image'] = np.array(image.copy())
+ end = start + bbox_num[i]
+ bbox_res = batch_res['bbox'][start:end] \
+ if 'bbox' in batch_res else None
+ mask_res, segm_res, keypoint_res = None, None, None
+ image = visualize_results(
+ image, bbox_res, mask_res, segm_res, keypoint_res,
+ int(im_id), catid2name, draw_threshold)
+ self.status['result_image'] = np.array(image.copy())
+ if self._compose_callback:
+ self._compose_callback.on_step_end(self.status)
+ # save image with detection
+ save_name = self._get_save_image_name(output_dir, image_path)
+ logger.info("Detection bbox results save in {}".format(
+ save_name))
+ image.save(save_name, quality=95)
+ start = end
+
def predict(self,
images,
draw_threshold=0.5,
diff --git a/ppdet/modeling/post_process.py b/ppdet/modeling/post_process.py
index 15060e7a8489cd6b0eb12af53273fd20e8731177..ceee73a79582d88ccaaf6fe92b2e0045669cd03f 100644
--- a/ppdet/modeling/post_process.py
+++ b/ppdet/modeling/post_process.py
@@ -617,8 +617,23 @@ class SparsePostProcess(object):
return bbox_pred, bbox_num
-def nms(dets, thresh):
- """Apply classic DPM-style greedy NMS."""
+def multiclass_nms(bboxs, num_classes, match_threshold=0.6, match_metric='iou'):
+ final_boxes = []
+ for c in range(num_classes):
+ idxs = bboxs[:, 0] == c
+ if np.count_nonzero(idxs) == 0: continue
+ r = nms(bboxs[idxs, 1:], match_threshold, match_metric)
+ final_boxes.append(np.concatenate([np.full((r.shape[0], 1), c), r], 1))
+ return final_boxes
+
+
+def nms(dets, match_threshold=0.6, match_metric='iou'):
+ """ Apply NMS to avoid detecting too many overlapping bounding boxes.
+ Args:
+ dets: shape [N, 5], [score, x1, y1, x2, y2]
+ match_metric: 'iou' or 'ios'
+ match_threshold: overlap thresh for match metric.
+ """
if dets.shape[0] == 0:
return dets[[], :]
scores = dets[:, 0]
@@ -626,25 +641,12 @@ def nms(dets, thresh):
y1 = dets[:, 2]
x2 = dets[:, 3]
y2 = dets[:, 4]
-
areas = (x2 - x1 + 1) * (y2 - y1 + 1)
order = scores.argsort()[::-1]
ndets = dets.shape[0]
suppressed = np.zeros((ndets), dtype=np.int)
- # nominal indices
- # _i, _j
- # sorted indices
- # i, j
- # temp variables for box i's (the box currently under consideration)
- # ix1, iy1, ix2, iy2, iarea
-
- # variables for computing overlap with box j (lower scoring box)
- # xx1, yy1, xx2, yy2
- # w, h
- # inter, ovr
-
for _i in range(ndets):
i = order[_i]
if suppressed[i] == 1:
@@ -665,8 +667,15 @@ def nms(dets, thresh):
w = max(0.0, xx2 - xx1 + 1)
h = max(0.0, yy2 - yy1 + 1)
inter = w * h
- ovr = inter / (iarea + areas[j] - inter)
- if ovr >= thresh:
+ if match_metric == 'iou':
+ union = iarea + areas[j] - inter
+ match_value = inter / union
+ elif match_metric == 'ios':
+ smaller = min(iarea, areas[j])
+ match_value = inter / smaller
+ else:
+ raise ValueError()
+ if match_value >= match_threshold:
suppressed[j] = 1
keep = np.where(suppressed == 0)[0]
dets = dets[keep, :]
diff --git a/tools/box_distribution.py b/tools/box_distribution.py
new file mode 100644
index 0000000000000000000000000000000000000000..c2e2cb8e0af9225f3a17238c9da26426c988c789
--- /dev/null
+++ b/tools/box_distribution.py
@@ -0,0 +1,108 @@
+# Copyright (c) 2022 PaddlePaddle Authors. All Rights Reserved.
+#
+# Licensed under the Apache License, Version 2.0 (the "License");
+# you may not use this file except in compliance with the License.
+# You may obtain a copy of the License at
+#
+# http://www.apache.org/licenses/LICENSE-2.0
+#
+# Unless required by applicable law or agreed to in writing, software
+# distributed under the License is distributed on an "AS IS" BASIS,
+# WITHOUT WARRANTIES OR CONDITIONS OF ANY KIND, either express or implied.
+# See the License for the specific language governing permissions and
+# limitations under the License.
+
+import matplotlib.pyplot as plt
+import json
+import numpy as np
+import argparse
+
+
+def median(data):
+ data.sort()
+ mid = len(data) // 2
+ median = (data[mid] + data[~mid]) / 2
+ return median
+
+
+def draw_distribution(width, height, out_path):
+ w_bins = int((max(width) - min(width)) // 10)
+ h_bins = int((max(height) - min(height)) // 10)
+ plt.figure()
+ plt.subplot(221)
+ plt.hist(width, bins=w_bins, color='green')
+ plt.xlabel('Width rate *1000')
+ plt.ylabel('number')
+ plt.title('Distribution of Width')
+ plt.subplot(222)
+ plt.hist(height, bins=h_bins, color='blue')
+ plt.xlabel('Height rate *1000')
+ plt.title('Distribution of Height')
+ plt.savefig(out_path)
+ print(f'Distribution saved as {out_path}')
+ plt.show()
+
+
+def get_ratio_infos(jsonfile, out_img):
+ allannjson = json.load(open(jsonfile, 'r'))
+ be_im_id = 1
+ be_im_w = []
+ be_im_h = []
+ ratio_w = []
+ ratio_h = []
+ images = allannjson['images']
+ for i, ann in enumerate(allannjson['annotations']):
+ if ann['iscrowd']:
+ continue
+ x0, y0, w, h = ann['bbox'][:]
+ if be_im_id == ann['image_id']:
+ be_im_w.append(w)
+ be_im_h.append(h)
+ else:
+ im_w = images[be_im_id - 1]['width']
+ im_h = images[be_im_id - 1]['height']
+ im_m_w = np.mean(be_im_w)
+ im_m_h = np.mean(be_im_h)
+ dis_w = im_m_w / im_w
+ dis_h = im_m_h / im_h
+ ratio_w.append(dis_w)
+ ratio_h.append(dis_h)
+ be_im_id = ann['image_id']
+ be_im_w = [w]
+ be_im_h = [h]
+
+ im_w = images[be_im_id - 1]['width']
+ im_h = images[be_im_id - 1]['height']
+ im_m_w = np.mean(be_im_w)
+ im_m_h = np.mean(be_im_h)
+ dis_w = im_m_w / im_w
+ dis_h = im_m_h / im_h
+ ratio_w.append(dis_w)
+ ratio_h.append(dis_h)
+ mid_w = median(ratio_w)
+ mid_h = median(ratio_h)
+ ratio_w = [i * 1000 for i in ratio_w]
+ ratio_h = [i * 1000 for i in ratio_h]
+ print(f'Median of ratio_w is {mid_w}')
+ print(f'Median of ratio_h is {mid_h}')
+ print('all_img with box: ', len(ratio_h))
+ print('all_ann: ', len(allannjson['annotations']))
+ draw_distribution(ratio_w, ratio_h, out_img)
+
+
+def main():
+ parser = argparse.ArgumentParser()
+ parser.add_argument(
+ '--json_path', type=str, default=None, help="Dataset json path.")
+ parser.add_argument(
+ '--out_img',
+ type=str,
+ default='box_distribution.jpg',
+ help="Name of distibution img.")
+ args = parser.parse_args()
+
+ get_ratio_infos(args.json_path, args.out_img)
+
+
+if __name__ == "__main__":
+ main()
diff --git a/tools/eval.py b/tools/eval.py
index f2e4fd0490ab44e40a2b0479ef60bb1f9cbbb76b..231d7ce09b54a664f6290a7f629fc258bb96624f 100755
--- a/tools/eval.py
+++ b/tools/eval.py
@@ -83,6 +83,40 @@ def parse_args():
default=False,
help="Enable auto mixed precision eval.")
+ # for smalldet slice_infer
+ parser.add_argument(
+ "--slice_infer",
+ action='store_true',
+ help="Whether to slice the image and merge the inference results for small object detection."
+ )
+ parser.add_argument(
+ '--slice_size',
+ nargs='+',
+ type=int,
+ default=[640, 640],
+ help="Height of the sliced image.")
+ parser.add_argument(
+ "--overlap_ratio",
+ nargs='+',
+ type=float,
+ default=[0.25, 0.25],
+ help="Overlap height ratio of the sliced image.")
+ parser.add_argument(
+ "--combine_method",
+ type=str,
+ default='nms',
+ help="Combine method of the sliced images' detection results, choose in ['nms', 'nmm', 'concat']."
+ )
+ parser.add_argument(
+ "--match_threshold",
+ type=float,
+ default=0.6,
+ help="Combine method matching threshold.")
+ parser.add_argument(
+ "--match_metric",
+ type=str,
+ default='iou',
+ help="Combine method matching metric, choose in ['iou', 'ios'].")
args = parser.parse_args()
return args
@@ -109,7 +143,15 @@ def run(FLAGS, cfg):
trainer.load_weights(cfg.weights)
# training
- trainer.evaluate()
+ if FLAGS.slice_infer:
+ trainer.evaluate_slice(
+ slice_size=FLAGS.slice_size,
+ overlap_ratio=FLAGS.overlap_ratio,
+ combine_method=FLAGS.combine_method,
+ match_threshold=FLAGS.match_threshold,
+ match_metric=FLAGS.match_metric)
+ else:
+ trainer.evaluate()
def main():
diff --git a/tools/infer.py b/tools/infer.py
index 3a5674e7b913739b40e7a84ea75232c96fa0025a..311cf8cf098a05f910731a7676750f13b4c292ae 100755
--- a/tools/infer.py
+++ b/tools/infer.py
@@ -81,6 +81,39 @@ def parse_args():
type=bool,
default=False,
help="Whether to save inference results to output_dir.")
+ parser.add_argument(
+ "--slice_infer",
+ action='store_true',
+ help="Whether to slice the image and merge the inference results for small object detection."
+ )
+ parser.add_argument(
+ '--slice_size',
+ nargs='+',
+ type=int,
+ default=[640, 640],
+ help="Height of the sliced image.")
+ parser.add_argument(
+ "--overlap_ratio",
+ nargs='+',
+ type=float,
+ default=[0.25, 0.25],
+ help="Overlap height ratio of the sliced image.")
+ parser.add_argument(
+ "--combine_method",
+ type=str,
+ default='nms',
+ help="Combine method of the sliced images' detection results, choose in ['nms', 'nmm', 'concat']."
+ )
+ parser.add_argument(
+ "--match_threshold",
+ type=float,
+ default=0.6,
+ help="Combine method matching threshold.")
+ parser.add_argument(
+ "--match_metric",
+ type=str,
+ default='iou',
+ help="Combine method matching metric, choose in ['iou', 'ios'].")
args = parser.parse_args()
return args
@@ -127,11 +160,23 @@ def run(FLAGS, cfg):
images = get_test_images(FLAGS.infer_dir, FLAGS.infer_img)
# inference
- trainer.predict(
- images,
- draw_threshold=FLAGS.draw_threshold,
- output_dir=FLAGS.output_dir,
- save_results=FLAGS.save_results)
+ if FLAGS.slice_infer:
+ trainer.slice_predict(
+ images,
+ slice_size=FLAGS.slice_size,
+ overlap_ratio=FLAGS.overlap_ratio,
+ combine_method=FLAGS.combine_method,
+ match_threshold=FLAGS.match_threshold,
+ match_metric=FLAGS.match_metric,
+ draw_threshold=FLAGS.draw_threshold,
+ output_dir=FLAGS.output_dir,
+ save_results=FLAGS.save_results)
+ else:
+ trainer.predict(
+ images,
+ draw_threshold=FLAGS.draw_threshold,
+ output_dir=FLAGS.output_dir,
+ save_results=FLAGS.save_results)
def main():
diff --git a/tools/slice_image.py b/tools/slice_image.py
new file mode 100644
index 0000000000000000000000000000000000000000..f739d74244b0e4672a5b2ed3430f89b936f0bef5
--- /dev/null
+++ b/tools/slice_image.py
@@ -0,0 +1,56 @@
+# Copyright (c) 2022 PaddlePaddle Authors. All Rights Reserved.
+#
+# Licensed under the Apache License, Version 2.0 (the "License");
+# you may not use this file except in compliance with the License.
+# You may obtain a copy of the License at
+#
+# http://www.apache.org/licenses/LICENSE-2.0
+#
+# Unless required by applicable law or agreed to in writing, software
+# distributed under the License is distributed on an "AS IS" BASIS,
+# WITHOUT WARRANTIES OR CONDITIONS OF ANY KIND, either express or implied.
+# See the License for the specific language governing permissions and
+# limitations under the License.
+
+import argparse
+from tqdm import tqdm
+
+
+def slice_data(image_dir, dataset_json_path, output_dir, slice_size,
+ overlap_ratio):
+ try:
+ from sahi.scripts.slice_coco import slice
+ except Exception as e:
+ raise RuntimeError(
+ 'Unable to use sahi to slice images, please install sahi, for example: `pip install sahi`, see https://github.com/obss/sahi'
+ )
+ tqdm.write(
+ f" slicing for slice_size={slice_size}, overlap_ratio={overlap_ratio}")
+ slice(
+ image_dir=image_dir,
+ dataset_json_path=dataset_json_path,
+ output_dir=output_dir,
+ slice_size=slice_size,
+ overlap_ratio=overlap_ratio, )
+
+
+def main():
+ parser = argparse.ArgumentParser()
+ parser.add_argument(
+ '--image_dir', type=str, default=None, help="The image folder path.")
+ parser.add_argument(
+ '--json_path', type=str, default=None, help="Dataset json path.")
+ parser.add_argument(
+ '--output_dir', type=str, default=None, help="Output dir.")
+ parser.add_argument(
+ '--slice_size', type=int, default=500, help="slice_size")
+ parser.add_argument(
+ '--overlap_ratio', type=float, default=0.25, help="overlap_ratio")
+ args = parser.parse_args()
+
+ slice_data(args.image_dir, args.json_path, args.output_dir, args.slice_size,
+ args.overlap_ratio)
+
+
+if __name__ == "__main__":
+ main()