Merge pull request #5 from WuHaobo/master
init docs
Showing
docs/Makefile
0 → 100644
docs/conf.py
0 → 100644
187.7 KB
1.7 MB
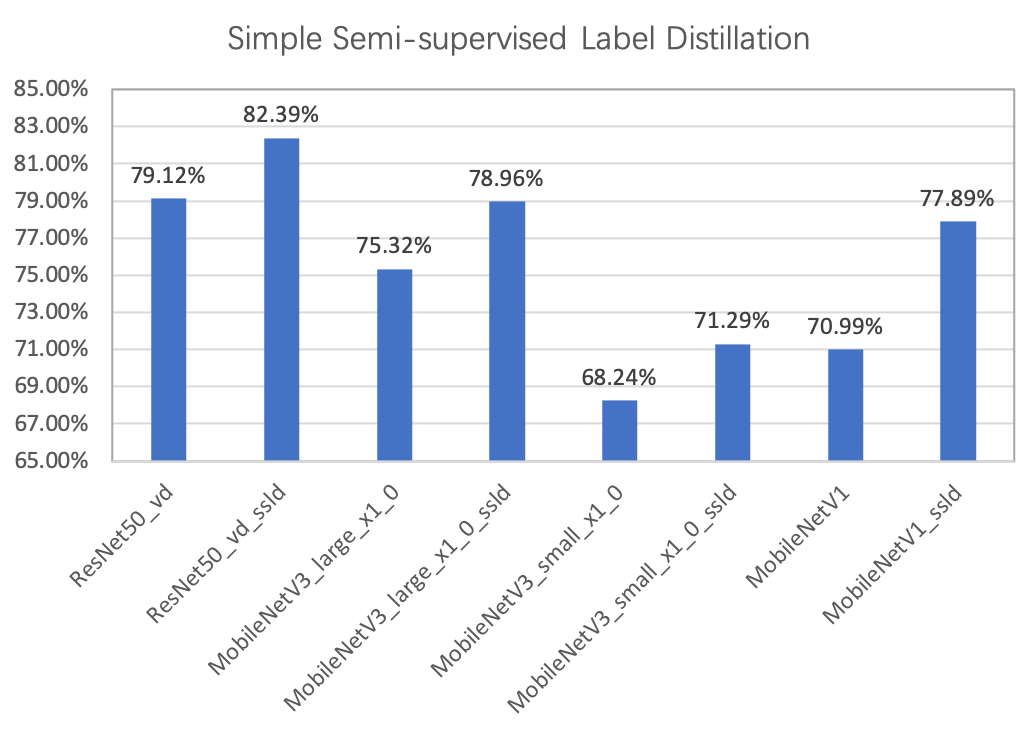
| W: | H:
| W: | H:
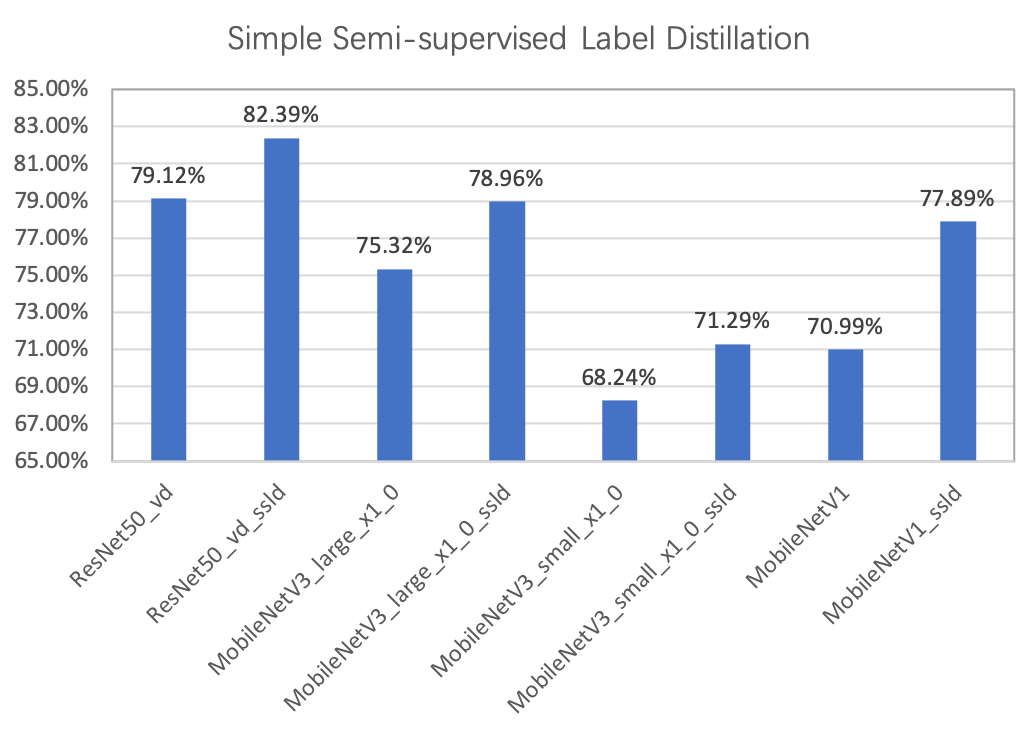
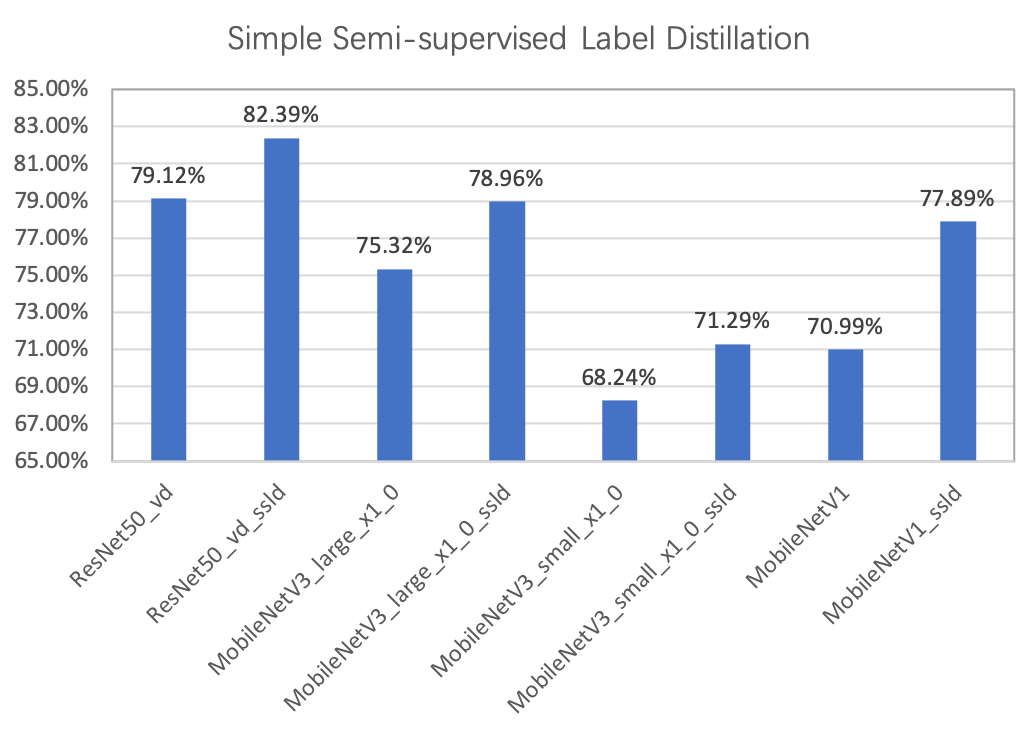
456.6 KB
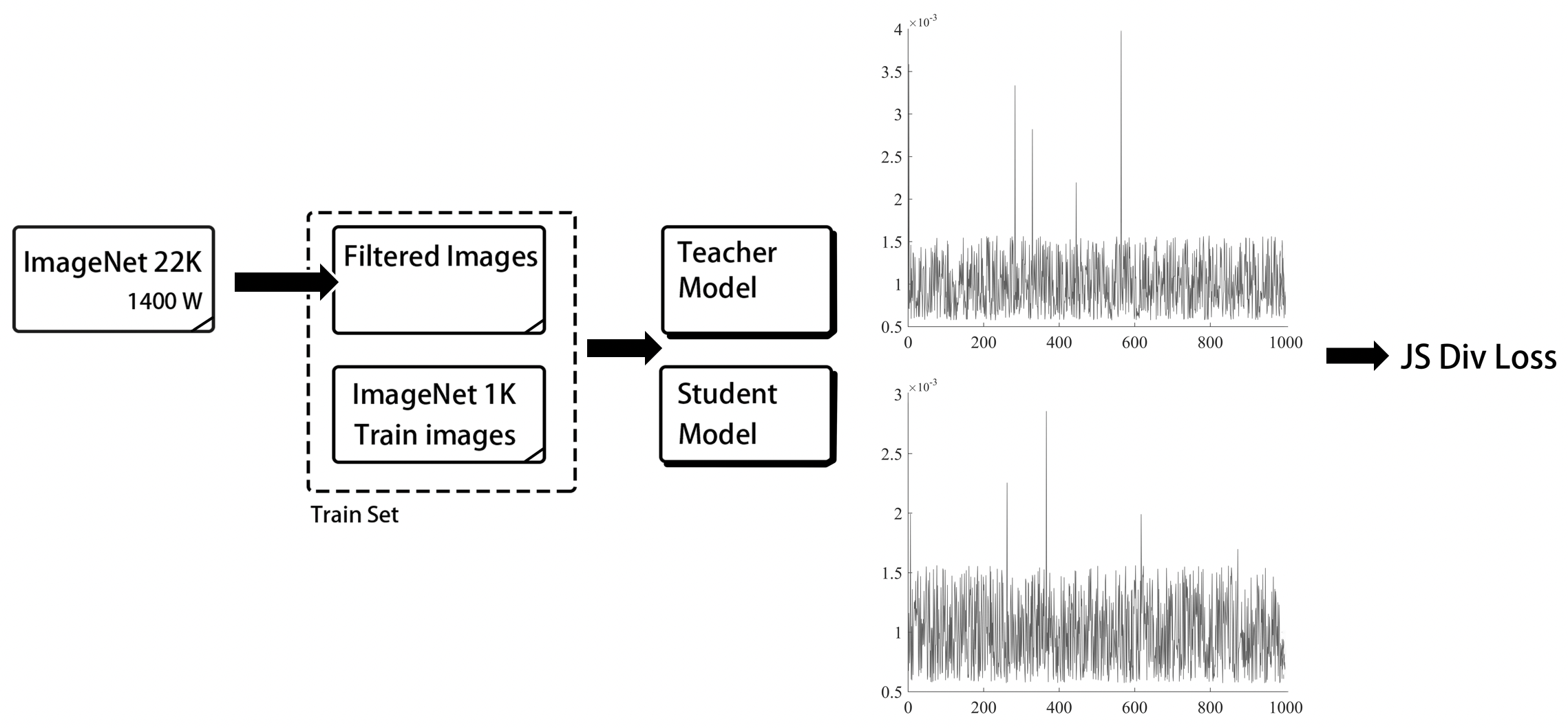
| W: | H:
| W: | H:
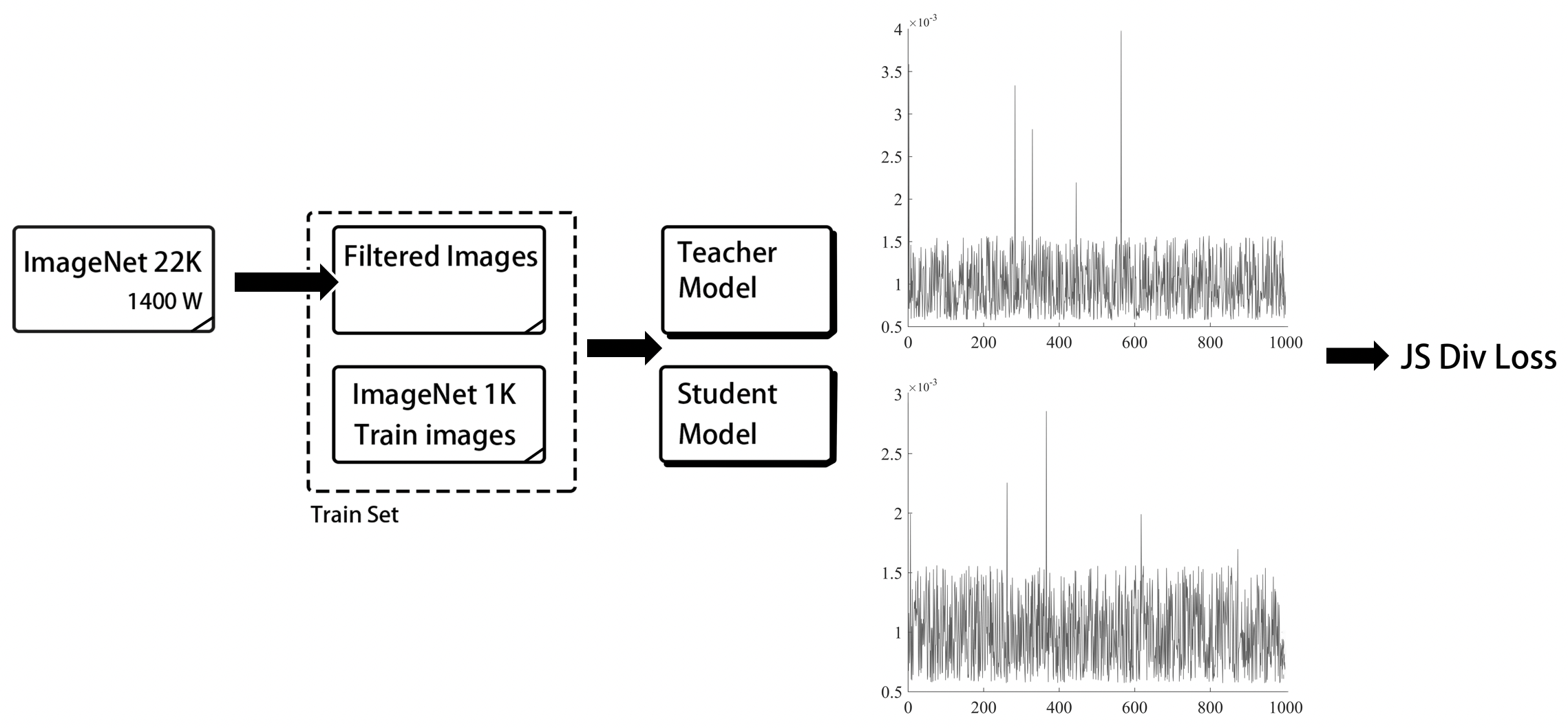
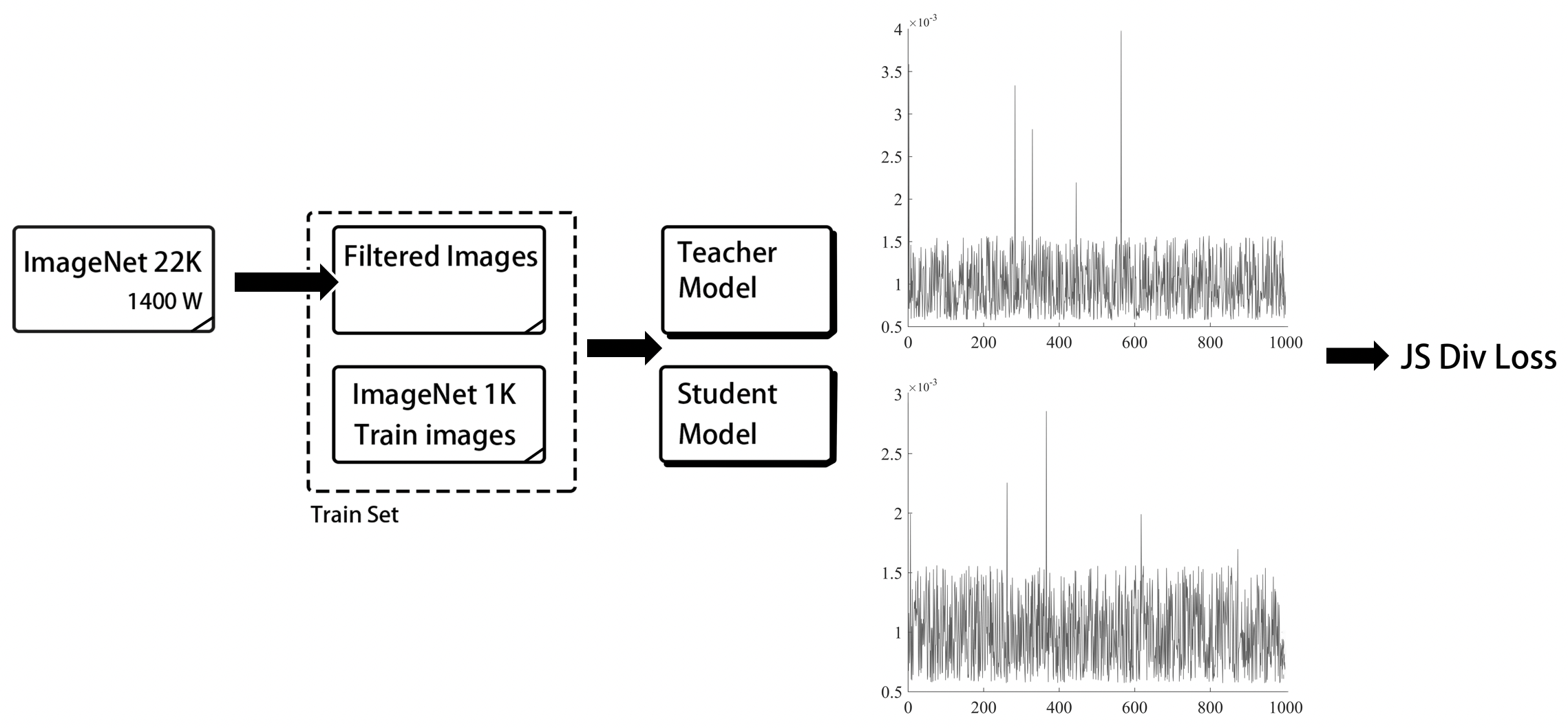
288.9 KB
330.1 KB
274.3 KB
234.7 KB
263.8 KB
196.7 KB
288.0 KB
254.9 KB
docs/images/models/DPN.png
0 → 100644
341.6 KB
438.0 KB
docs/images/models/HRNet.png
0 → 100644
326.6 KB
docs/images/models/Inception.png
0 → 100644
344.0 KB
docs/images/models/ResNet.png
0 → 100644
439.7 KB
docs/images/models/SeResNeXt.png
0 → 100644
581.4 KB
474.4 KB
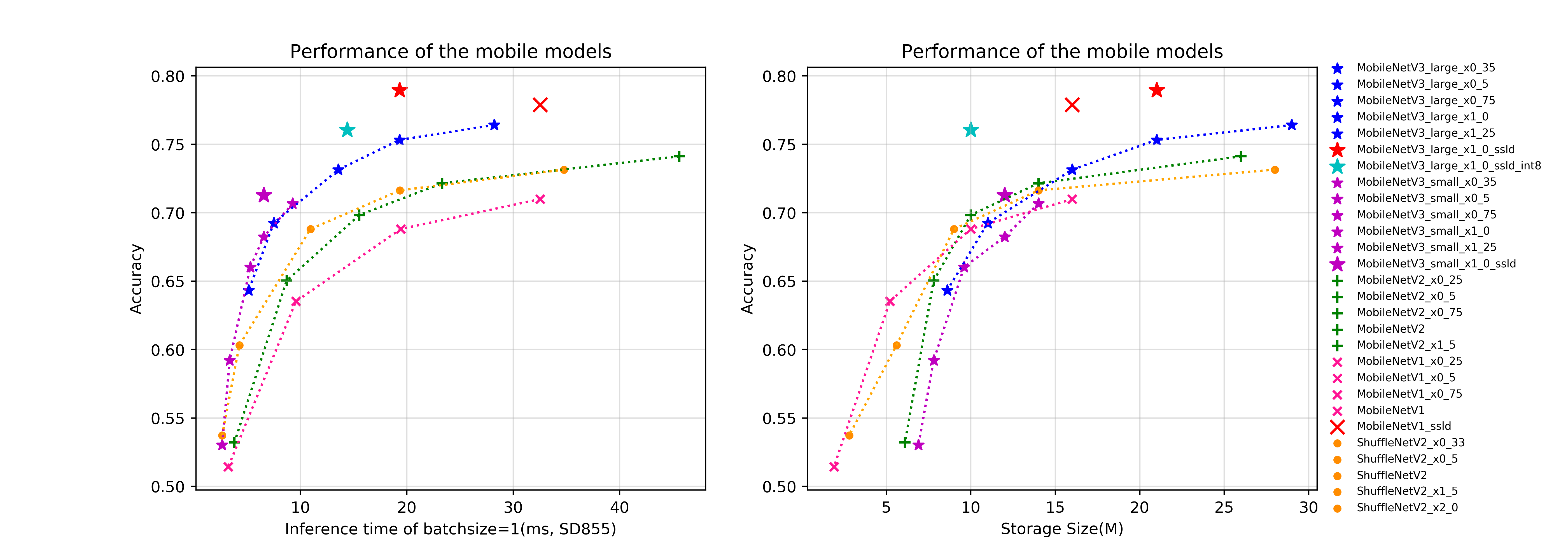
| W: | H:
| W: | H:
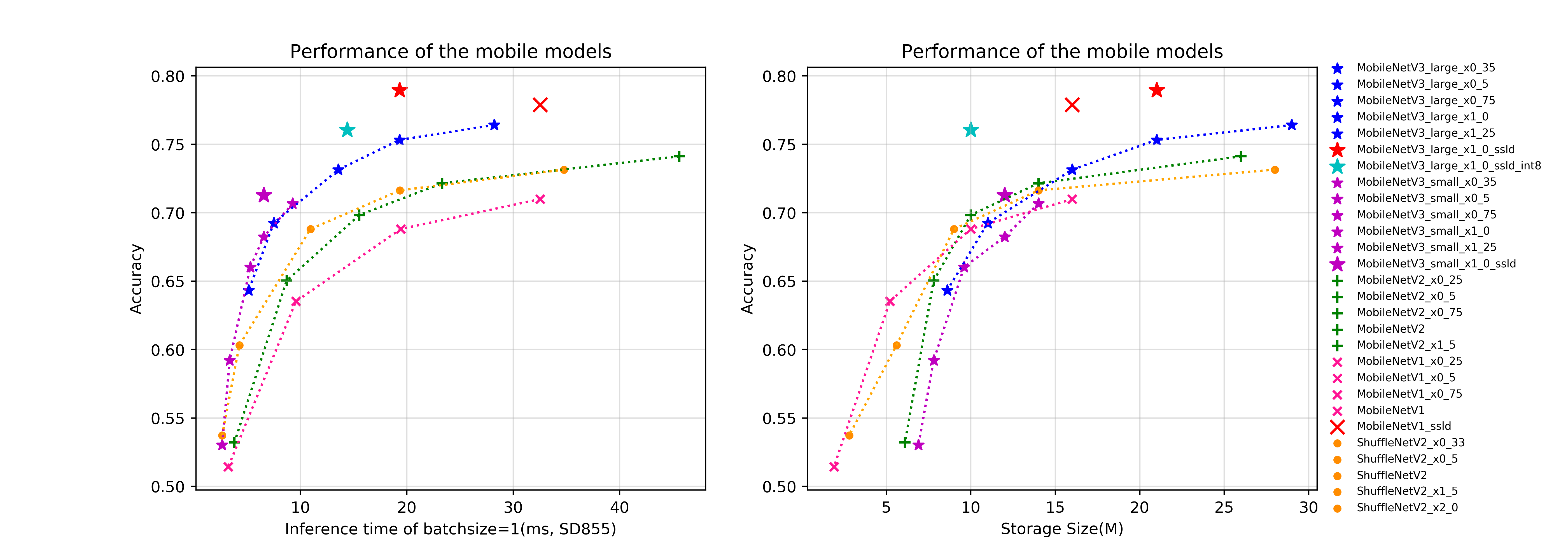
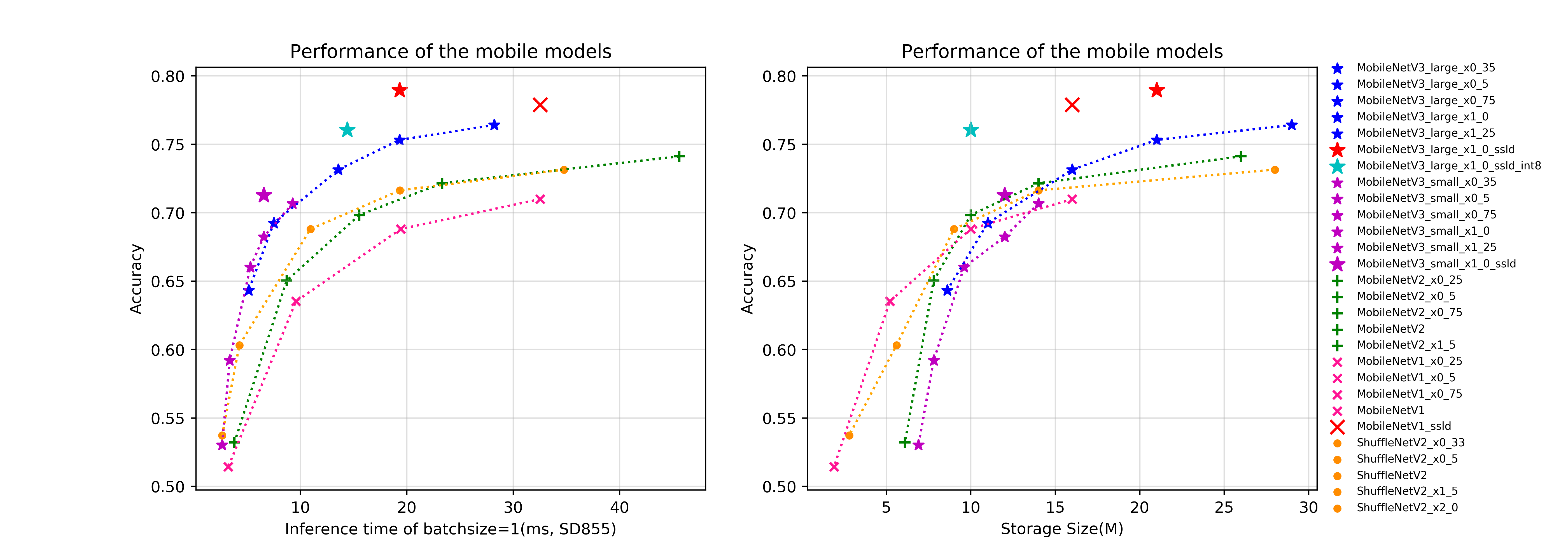
docs/images/models/mobile_trt.png
0 → 100644
590.3 KB
docs/images/paddlepaddle.jpg
0 → 100644
3.4 KB
docs/index.rst
0 → 100644
docs/zh_cn/Makefile
0 → 100644
docs/zh_cn/models/DPN_DenseNet.md
0 → 100644
docs/zh_cn/models/HRNet.md
0 → 100644
docs/zh_cn/models/Inception.md
0 → 100644
docs/zh_cn/models/Mobile.md
0 → 100644
docs/zh_cn/models/Others.md
0 → 100644
docs/zh_cn/models/Tricks.md
0 → 100644
docs/zh_cn/models/index.rst
0 → 100644
docs/zh_cn/models/models_intro.md
0 → 100644
docs/zh_cn/tutorials/config.md
0 → 100644
docs/zh_cn/tutorials/index.rst
0 → 100644
docs/zh_cn/tutorials/install.md
0 → 100644