diff --git a/cmake/version.cmake b/cmake/version.cmake
index 2fa9df4211d384e8900ad7ad74830c3f0dfca471..ac10bdf067be549fe90112aef73fd6e1fbe0ac48 100644
--- a/cmake/version.cmake
+++ b/cmake/version.cmake
@@ -23,15 +23,16 @@ while ("${PADDLE_VERSION}" STREQUAL "")
if (${GIT_BRANCH_NAME} MATCHES "release/${TAG_VERSION_REGEX}")
# Check the tag is a correct version
if (${GIT_TAG_NAME} MATCHES "${COMMIT_VERSION_REGEX}")
- # if no tag was found, set PADDLE_VERSION to latest
- set(PADDLE_VERSION "latest")
+ # if no tag was found, set PADDLE_VERSION to 0.0.0 to represent latest
+ set(PADDLE_VERSION "0.0.0")
elseif (${GIT_TAG_NAME} MATCHES "v${TAG_VERSION_REGEX}")
string(REPLACE "v" "" PADDLE_VERSION ${GIT_TAG_NAME})
else() # otherwise, get the previous git tag name.
set(tmp_version "${GIT_TAG_NAME}~1")
endif()
- else() # otherwise, we always set PADDLE_VERSION to latest
- set(PADDLE_VERSION "latest")
+ else()
+ # otherwise, we always set PADDLE_VERSION to 0.0.0 to represent latest
+ set(PADDLE_VERSION "0.0.0")
endif()
else()
set(PADDLE_VERSION "0.0.0")
diff --git a/doc/fluid/api/layers.rst b/doc/fluid/api/layers.rst
index d443c49657b92583e527035f49e74462cf41487d..ecbd8191ccf5aa6046e7875fe8afa2ed0105e4a0 100644
--- a/doc/fluid/api/layers.rst
+++ b/doc/fluid/api/layers.rst
@@ -1768,3 +1768,11 @@ reverse
.. autofunction:: paddle.fluid.layers.reverse
:noindex:
+.. _api_fluid_layers_rank_loss:
+
+rank_loss
+-------
+
+.. autofunction:: paddle.fluid.layers.rank_loss
+ :noindex:
+
diff --git a/doc/fluid/howto/inference/build_and_install_lib_cn.rst b/doc/fluid/howto/inference/build_and_install_lib_cn.rst
index 84005b54e07cf810649370d2c1f6b6c522434bf6..91357dd8c8da19f2f33c6f285ed7eb234428b1ab 100644
--- a/doc/fluid/howto/inference/build_and_install_lib_cn.rst
+++ b/doc/fluid/howto/inference/build_and_install_lib_cn.rst
@@ -7,13 +7,13 @@
====================== ========================================
版本说明 C++预测库
====================== ========================================
-cpu_avx_mkl `fluid.tgz `_
-cpu_avx_openblas `fluid.tgz `_
-cpu_noavx_openblas `fluid.tgz `_
-cuda7.5_cudnn5_avx_mkl `fluid.tgz `_
-cuda8.0_cudnn5_avx_mkl `fluid.tgz `_
-cuda8.0_cudnn7_avx_mkl `fluid.tgz `_
-cuda9.0_cudnn7_avx_mkl `fluid.tgz `_
+cpu_avx_mkl `fluid.tgz `_
+cpu_avx_openblas `fluid.tgz `_
+cpu_noavx_openblas `fluid.tgz `_
+cuda7.5_cudnn5_avx_mkl `fluid.tgz `_
+cuda8.0_cudnn5_avx_mkl `fluid.tgz `_
+cuda8.0_cudnn7_avx_mkl `fluid.tgz `_
+cuda9.0_cudnn7_avx_mkl `fluid.tgz `_
====================== ========================================
从源码编译
diff --git a/doc/fluid/index_cn.rst b/doc/fluid/index_cn.rst
index d878d192cae7ee9e8b8fdb4f615839c186fdf334..6b1ef3ceed4f7ed5073d42c13ce103e2ab467e58 100644
--- a/doc/fluid/index_cn.rst
+++ b/doc/fluid/index_cn.rst
@@ -1,12 +1,16 @@
- PaddlePaddle Fluid
-==========================
+.. PaddlePaddle Fluid documentation master file, created by
+ sphinx-quickstart on Thu Jun 7 17:04:53 2018.
+ You can adapt this file completely to your liking, but it should at least
+ contain the root `toctree` directive.
+
+##############
+欢迎使用 Fluid
+##############
.. toctree::
- :maxdepth: 1
+ :maxdepth: 1
- getstarted/index_cn.rst
- build_and_install/index_cn.rst
- design/index_cn.rst
- howto/index_cn.rst
- dev/index_cn.rst
- faq/index_cn.rst
+ new_docs/beginners_guide/index.rst
+ new_docs/user_guides/index.rst
+ new_docs/advanced_usage/index.rst
+ new_docs/faq/index_cn.rst
diff --git a/doc/fluid/new_docs/advanced_usage/benchmark.rst b/doc/fluid/new_docs/advanced_usage/benchmark.rst
new file mode 100644
index 0000000000000000000000000000000000000000..7854263bf8f64c840492550fb22152582c7d2361
--- /dev/null
+++ b/doc/fluid/new_docs/advanced_usage/benchmark.rst
@@ -0,0 +1,120 @@
+#################
+如何进行基准测试
+#################
+
+本文介绍如何给深度学习框架做基准测试。基准测试主要包含验证模型的精度和性能两方面,下文包含搭建测试环境,选择基准测试模型,验证测试结果等几方面内容。
+
+验证深度学习框架,可分为训练和测试两个阶段, 验证指标略有不同,本文只介绍训练阶段的指标验证。训练阶段关注的是模型训练集上的精度,训练集是完备的,因此关注大batch\_size下的训练速度,关注吞吐量,例如图像模型常用的batch\_size=128, 多卡情况下会加大;预测阶段关注的是在测试集上的精度,线上服务测试数据不能提前收集,因此关注小batch\_size下的预测速度,关注延迟,例如预测服务常用的batch\_size=1, 4等。
+
+`Fluid `__ 是PaddlePaddle从0.11.0版本开始引入的设计,本文的基准测试在该版本上完成。
+
+
+环境搭建
+""""""""""""
+
+基准测试中模型精度和硬件、框架无关,由模型结构和数据共同决定;性能方面由测试硬件和框架性能决定。框架基准测试为了对比框架之间的差异,控制硬件环境,系统库等版本一致。下文中的对比实验都在相同的硬件条件和系统环境条件下进行.
+
+
+不同架构的GPU卡性能差异巨大,在验证模型在GPU上训练性能时,可使用NVIDIA提供的工具:code `nvidia-smi` 检验当前使用的GPU型号,如果测试多卡训练性能,需确认硬件连接是 `nvlink `__ 或 `PCIe `__ 。 同样地,CPU型号会极大影响模型在CPU上的训练性能。可读取`/proc/cpuinfo`中的参数,确认当前正在使用的CPU型号。
+
+下载GPU对应的Cuda Tool Kit和 Cudnn,或者使用NVIDIA官方发布的nvidia-docker镜像 `nvidia-docker `__, 镜像内包含了Cuda和Cudnn,本文采用这种方式。 Cuda Tool Kit包含了GPU代码使用到的基础库,影响在此基础上编译出的Fluid二进制运行性能。
+
+准备好Cuda环境后,从github上的下载Paddle并源码编译,会生成对应的最适合当前GPU的sm\_arch二进制\ `sm\_arch `__\ 。另外,cudnn对卷积类任务影响巨大,在基准测试中需要小版本一致,例如Cudnn7.0.2与Cudnn7.1.4在Resnet上有5%以上差异。
+
+
+选择基准模型
+""""""""""""
+
+对框架做基准测试,需要覆盖不同训练任务和不同大小的模型,本文中选取了图像和NLP的最为常用的5个模型。
+
+============ ============ ================= ============
+任务种类 模型名称 网络结构 数据集
+============ ============ ================= ============
+图像分类 mnist Lenet mnist
+图像分类 VGG VGG-16 Flowers102
+图像分类 Resnet Resnet-50 Flowers102
+文本分类 Stacked-LSTM Stacked-LSTM IMDB
+机器翻译 seq-seq Stacked-LSTM wmt14
+============ ============ ================= ============
+
+其中mnist, VGG, Resnet属于CNN模型, stacked-lstm, seq2seq代表RNN模型。
+`benchmark `__
+基准模型测试脚本中,均跳过了前几个batch的训练过程,原因是加载数据和分配显存受系统当前运行情况影响,会导致统计性能不准确。运行完若干个轮次后,统计对应指标。
+
+
+基准模型的数据的选择方面,数据量大且验证效果多的公开数据集为首选。图像模型VGG和resnet, 本文选择了 `flowers102 `__ ,图像大小预处理为和Imagenet相同大小,因此性能可直接对比
+NLP模型的公开且影响力大数据集较少,seq2seq模型选择了wmt14数据,stacked-lstm模型中选择了 `imdb `__ 数据。
+
+
+注意,图像模型每条样本大小相同,图像经过变换后大小一致,因此经过的计算路径基本相同,计算速度和显存占用波动较小,可以从若干个batch的数据中采样得到当前的训练性能数据。而NLP模型由于样本长度不定,计算路径和显存占用也不相同,因此只能完整运行若干个轮次后,统计速度和显存消耗。
+显存分配是特别耗时的操作,因此Fluid默认会占用所有可用显存空间形成显存池,用以加速计算过程中的显存分配。如果需要统计模型真实显存消耗,可设置环境变量`FLAGS_fraction_of_gpu_memory_to_use=0.0`,观察最大显存开销。
+
+
+测试过程
+""""""""""""
+
+- CPU 单机单线程测试
+
+测试CPU上单线程的性能,先设置CUDA的环境变量为空,``CUDA_VISIBLE_DEVICES=``,并通过环境变量关闭OpenMP和MKL的多线程 ``OMP_NUM_THREADS=1``, ``MKL_NUM_THREADS=1;``。
+然后代码中设置为使用CPUPlace,如果使用Paddle代码库中的脚本,只需要命令行参数传入 use_gpu=False即可。
+
+.. code-block:: python
+
+ >>> import paddle.fluid as fluid
+ >>> place = fluid.CPUPlace()
+
+.. code:: bash
+
+ docker run -it --name CASE_NAME --security-opt seccomp=unconfined -v $PWD/benchmark:/benchmark paddlepaddle/paddle:latest-dev /bin/bash
+
+
+- GPU 单机单卡测试
+
+本教程使用了Cuda8, Cudnn7.0.1。来源为:code `nvidia/cuda:8.0-cudnn7-devel-ubuntu16.04`
+
+.. code:: bash
+
+ nvidia-docker run -it --name CASE_NAME --security-opt seccomp=unconfined -v $PWD/benchmark:/benchmark -v /usr/lib/x86_64-linux-gnu:/usr/lib/x86_64-linux-gnu paddlepaddle/paddle:latest-dev /bin/bash
+在单卡上测试,设置CUDA的环境变量使用一块GPU,``CUDA_VISIBLE_DEVICES=0``
+然后代码中设置为使用CUDAPlace,如果使用Paddle代码库中的脚本,只需要命令行参数传入 use_gpu=True即可。
+
+.. code-block:: python
+
+ >>> import paddle.fluid as fluid
+ >>> place = fluid.CUDAPlace(0) // 0 指第0块GPU
+
+
+测试结果
+""""""""""""
+
+本教程对比相同环境下的Fluid0.12.0和TensorFlow1.4.0的性能表现。
+硬件环境为 CPU: Intel(R) Xeon(R) CPU E5-2660 v4 @ 2.00GHz, GPU: TITAN X(Pascal) 12G x 1, Nvidia-Driver 384.90。
+系统环境为Ubuntu 16.04.3 LTS, 本文中采用了docker环境,系统版本为nvidia-docker17.05.0-ce。
+测试的Fluid版本为\ `v.0.12.0 `__ 。
+TensorFlow版本为\ `v.1.4.0-rc1 `__ 。
+使用的脚本和配置见\ `benchmark `__ 。
+图表中统计单位为samples/秒。
+
+- CPU 单机单线程测试结果
+
+ ================ ==================== ===================
+ Speed Fluid CPU TensorFlow CPU
+ ================ ==================== ===================
+ mnist 1298.75 samples/s 637.57 samples/s
+ VGG-16 0.4147 images/s 0.1229 images/s
+ Resnet-50 1.6935 images/s 0.3657 images/s
+ Stacked-LSTM 472.3225 words/s 48.2293words/s
+ Seq2Seq 217.1655 words/s 28.6164 words/s
+ ================ ==================== ===================
+
+- GPU 单机单卡测试结果
+
+ =============== ===================== =================
+ Speed Fluid GPU TensorFlow GPU
+ =============== ===================== =================
+ mnist 19710.90 samples/s 15576.3 samples/s
+ VGG-16 59.83327 images/s 40.9967 images/s
+ Resnet-50 105.84412 97.8923 images/s
+ Stacked-LSTM 1319.99315 1608.2526 words/s
+ Seq2Seq 7147.89081 6845.1161 words/s
+ =============== ===================== =================
diff --git a/doc/fluid/new_docs/advanced_usage/deploy/anakin_arm_benchmark.md b/doc/fluid/new_docs/advanced_usage/deploy/anakin_arm_benchmark.md
new file mode 100644
index 0000000000000000000000000000000000000000..08ea379f81d16407ed5f82770b55a34bcf138da8
--- /dev/null
+++ b/doc/fluid/new_docs/advanced_usage/deploy/anakin_arm_benchmark.md
@@ -0,0 +1,56 @@
+# Anakin ARM 性能测试
+
+## 测试环境和参数:
++ 测试模型Mobilenetv1, mobilenetv2, mobilenet-ssd
++ 采用android ndk交叉编译,gcc 4.9,enable neon, ABI: armveabi-v7a with neon -mfloat-abi=softfp
++ 测试平台
+ - 荣耀v9(root): 处理器:麒麟960, 4 big cores in 2.36GHz, 4 little cores in 1.8GHz
+ - nubia z17:处理器:高通835, 4 big cores in 2.36GHz, 4 little cores in 1.9GHz
+ - 360 N5:处理器:高通653, 4 big cores in 1.8GHz, 4 little cores in 1.4GHz
++ 多线程:openmp
++ 时间:warmup10次,运行10次取均值
++ ncnn版本:来源于github的master branch中commits ID:307a77f04be29875f40d337cfff6df747df09de6(msg:convert LogisticRegressionOutput)版本
++ TFlite版本:来源于github的master branch中commits ID:65c05bc2ac19f51f7027e66350bc71652662125c(msg:Removed unneeded file copy that was causing failure in Pi builds)版本
+
+在BenchMark中本文将使用**`ncnn`**、**`TFlite`**和**`Anakin`**进行性能对比分析
+
+## BenchMark model
+
+> 注意在性能测试之前,请先将测试model通过[External Converter](#10003)转换为Anakin model
+> 对这些model,本文在ARM上进行多线程的单batch size测试。
+
+- [Mobilenet v1](#11) *caffe model 可以在[这儿](https://github.com/shicai/MobileNet-Caffe)下载*
+- [Mobilenet v2](#22) *caffe model 可以在[这儿](https://github.com/shicai/MobileNet-Caffe)下载*
+- [mobilenet-ssd](#33) *caffe model 可以在[这儿](https://github.com/chuanqi305/MobileNet-SSD)下载*
+
+### mobilenetv1
+
+ |platform | Anakin (1) | Anakin (2) | Anakin (4) | ncnn (1) | ncnn (2) | ncnn (4) | TFlite (1) | TFlite (2) | TFlite (4)|
+ |:---: | :---: | :---: | :---:| :---:| :---:| :---:| :---:| :---:| :---:|
+ |麒麟960|107.7ms|61.1ms|38.2ms|152.8ms|85.2ms|51.9ms|152.6ms|nan|nan|
+ |高通835|105.7ms|63.1ms|~~46.8ms~~|152.7ms|87.0ms|~~92.7ms~~|146.9ms|nan|nan|
+ |高通653|120.3ms|64.2ms|46.6ms|202.5ms|117.6ms|84.8ms|158.6ms|nan|nan|
+
+### mobilenetv2
+
+ |platform | Anakin (1) | Anakin (2) | Anakin (4) | ncnn (1) | ncnn (2) | ncnn (4) | TFlite (1) | TFlite (2) | TFlite (4)|
+ |:---: | :---: | :---: | :---:| :---:| :---:| :---:| :---:| :---:| :---:|
+ |麒麟960|93.1ms|53.9ms|34.8ms|144.4ms|84.3ms|55.3ms|100.6ms|nan|nan|
+ |高通835|93.0ms|55.6ms|41.1ms|139.1ms|88.4ms|58.1ms|95.2ms|nan|nan|
+ |高通653|106.6ms|64.2ms|48.0ms|199.9ms|125.1ms|98.9ms|108.5ms|nan|nan|
+
+### mobilenet-ssd
+
+ |platform | Anakin (1) | Anakin (2) | Anakin (4) | ncnn (1) | ncnn (2) | ncnn (4) | TFlite (1) | TFlite (2) | TFlite (4)|
+ |:---: | :---: | :---: | :---:| :---:| :---:| :---:| :---:| :---:| :---:|
+ |麒麟960|213.9ms|120.5ms|74.5ms|307.9ms|166.5ms|104.2ms|nan|nan|nan|
+ |高通835|213.0ms|125.7ms|~~98.4ms~~|292.9ms|177.9ms|~~167.8ms~~|nan|nan|nan|
+ |高通653|236.0ms|129.6ms|96.0ms|377.7ms|228.9ms|165.0ms|nan|nan|nan
+
+## How to run those Benchmark models?
+
+1. 首先, 使用[External Converter](../docs/Manual/Converter_en.md)对caffe model 进行转换
+2. 然后将转换后的Anakin model和编译好的benchmark_arm 二进制文件通过'adb push'命令上传至测试机
+3. 接着在测试机含有Anakin model的目录中运行'./benchmark_arm ./ anakin_model.anakin.bin 1 10 10 1' 命令
+4. 最后,终端显示器上将会打印该模型的运行时间
+5. 其中运行命令的参数个数和含义可以通过运行'./benchmark_arm'看到
diff --git a/doc/fluid/new_docs/advanced_usage/deploy/anakin_example.md b/doc/fluid/new_docs/advanced_usage/deploy/anakin_example.md
new file mode 100644
index 0000000000000000000000000000000000000000..e6b9e18fe2d64b3fda6382bb23a6a818a3e17fbe
--- /dev/null
+++ b/doc/fluid/new_docs/advanced_usage/deploy/anakin_example.md
@@ -0,0 +1,28 @@
+# Example
+Anakin目前只支持NCHW的格式
+示例文件在test/framework/net下
+
+## 在NV的GPU上运行CNN模型
+示例文件为打开example_nv_cnn_net.cpp,整体流程如下:
+- 将模型的的path设置为anakin模型的路径,初始化NV平台的图对象。 anakin模型可以通过转换器转化caffe或fluid的模型得到
+- 根据模型设置网络图的输入尺寸,进行图优化
+- 根据优化后的网络图初始化网络执行器
+- 取出网络的输入tensor,将数据拷贝到输入tensor
+- 运行推导
+- 取出网络的输出tensor
+
+以NV平台为例演示Anakin框架的使用方法,注意编译时需要打开GPU编译开关
+
+## 在X86上运行RNN模型
+示例文件为example_x86_rnn_net.cpp
+整体流程与在NV的GPU上运行CNN模型相似,不同之处如下:
+- 使用X86标识初始化图对象和网络执行器对象
+- rnn模型的输入尺寸是可变的,初始化图时的输入维度是维度的最大值,输入维度N代表总的词的个数。还需要设置输入tensor的seq_offset来标示这些词是如何划分为句子的,如{0,5,12}表示共有12个词,其中第0到第4个词是第一句话,第5到第11个词是第二句话
+
+以X86平台为例演示Anakin框架的使用方法,注意编译时需要打开X86编译开关
+
+## 在NV的GPU上使用Anakin的线程池运行CNN模型
+示例文件为example_nv_cnn_net_multi_thread.cpp ,示例使用worker的同步预测接口
+整体流程与在NV的GPU上运行CNN模型相似,不同之处如下:
+- 用模型地址和线程池大小初始化worker对象
+- 将输入tensor注入任务队列,获得输出tensor
diff --git a/doc/fluid/new_docs/advanced_usage/deploy/anakin_gpu_benchmark.md b/doc/fluid/new_docs/advanced_usage/deploy/anakin_gpu_benchmark.md
new file mode 100644
index 0000000000000000000000000000000000000000..667f9396f1169a0d891b9e6b0e912aa5527ab0b8
--- /dev/null
+++ b/doc/fluid/new_docs/advanced_usage/deploy/anakin_gpu_benchmark.md
@@ -0,0 +1,170 @@
+# Anakin GPU Benchmark
+
+## Machine:
+
+> CPU: `12-core Intel(R) Xeon(R) CPU E5-2620 v2 @2.10GHz`
+> GPU: `Tesla P4`
+> cuDNN: `v7`
+
+
+## Counterpart of anakin :
+
+The counterpart of **`Anakin`** is the acknowledged high performance inference engine **`NVIDIA TensorRT 3`** , The models which TensorRT 3 doesn't support we use the custom plugins to support.
+
+## Benchmark Model
+
+The following convolutional neural networks are tested with both `Anakin` and `TenorRT3`.
+ You can use pretrained caffe model or the model trained by youself.
+
+> Please note that you should transform caffe model or others into anakin model with the help of [`external converter ->`](../docs/Manual/Converter_en.md)
+
+
+- [Vgg16](#1) *caffe model can be found [here->](https://gist.github.com/jimmie33/27c1c0a7736ba66c2395)*
+- [Yolo](#2) *caffe model can be found [here->](https://github.com/hojel/caffe-yolo-model)*
+- [Resnet50](#3) *caffe model can be found [here->](https://github.com/KaimingHe/deep-residual-networks#models)*
+- [Resnet101](#4) *caffe model can be found [here->](https://github.com/KaimingHe/deep-residual-networks#models)*
+- [Mobilenet v1](#5) *caffe model can be found [here->](https://github.com/shicai/MobileNet-Caffe)*
+- [Mobilenet v2](#6) *caffe model can be found [here->](https://github.com/shicai/MobileNet-Caffe)*
+- [RNN](#7) *not support yet*
+
+We tested them on single-GPU with single-thread.
+
+### VGG16
+
+- Latency (`ms`) of different batch
+
+| BatchSize | TensorRT | Anakin |
+| --- | --- | --- |
+| 1 | 8.8690 | 8.2815 |
+| 2 | 15.5344 | 13.9116 |
+| 4 | 26.6000 | 21.8747 |
+| 8 | 49.8279 | 40.4076 |
+| 32 | 188.6270 | 163.7660 |
+
+- GPU Memory Used (`MB`)
+
+| BatchSize | TensorRT | Anakin |
+| --- | --- | --- |
+| 1 | 963 | 997 |
+| 2 | 965 | 1039 |
+| 4 | 991 | 1115 |
+| 8 | 1067 | 1269 |
+| 32 | 1715 | 2193 |
+
+
+### Yolo
+
+- Latency (`ms`) of different batch
+
+| BatchSize | TensorRT | Anakin |
+| --- | --- | --- |
+| 1 | 16.4596| 15.2124 |
+| 2 | 26.6347| 25.0442 |
+| 4 | 43.3695| 43.5017 |
+| 8 | 80.9139 | 80.9880 |
+| 32 | 293.8080| 310.8810 |
+
+- GPU Memory Used (`MB`)
+
+| BatchSize | TensorRT | Anakin |
+| --- | --- | --- |
+| 1 | 1569 | 1775 |
+| 2 | 1649 | 1815 |
+| 4 | 1709 | 1887 |
+| 8 | 1731 | 2031 |
+| 32 | 2253 | 2907 |
+
+### Resnet50
+
+- Latency (`ms`) of different batch
+
+| BatchSize | TensorRT | Anakin |
+| --- | --- | --- |
+| 1 | 4.2459 | 4.1061 |
+| 2 | 6.2627 | 6.5159 |
+| 4 | 10.1277 | 11.3327 |
+| 8 | 17.8209 | 20.6680 |
+| 32 | 65.8582 | 77.8858 |
+
+- GPU Memory Used (`MB`)
+
+| BatchSize | TensorRT | Anakin |
+| --- | --- | --- |
+| 1 | 531 | 503 |
+| 2 | 543 | 517 |
+| 4 | 583 | 541 |
+| 8 | 611 | 589 |
+| 32 | 809 | 879 |
+
+### Resnet101
+
+- Latency (`ms`) of different batch
+
+| BatchSize | TensorRT | Anakin |
+| --- | --- | --- |
+| 1 | 7.5562 | 7.0837 |
+| 2 | 11.6023 | 11.4079 |
+| 4 | 18.3650 | 20.0493 |
+| 8 | 32.7632 | 36.0648 |
+| 32 | 123.2550 | 135.4880 |
+
+- GPU Memory Used (`MB)`
+
+| BatchSize | TensorRT | Anakin |
+| --- | --- | --- |
+| 1 | 701 | 683 |
+| 2 | 713 | 697 |
+| 4 | 793 | 721 |
+| 8 | 819 | 769 |
+| 32 | 1043 | 1059 |
+
+### MobileNet V1
+
+- Latency (`ms`) of different batch
+
+| BatchSize | TensorRT | Anakin |
+| --- | --- | --- |
+| 1 | 45.5156 | 1.3947 |
+| 2 | 46.5585 | 2.5483 |
+| 4 | 48.4242 | 4.3404 |
+| 8 | 52.7957 | 8.1513 |
+| 32 | 83.2519 | 31.3178 |
+
+- GPU Memory Used (`MB`)
+
+| BatchSize | TensorRT | Anakin |
+| --- | --- | --- |
+| 1 | 329 | 283 |
+| 2 | 345 | 289 |
+| 4 | 371 | 299 |
+| 8 | 393 | 319 |
+| 32 | 531 | 433 |
+
+### MobileNet V2
+
+- Latency (`ms`) of different batch
+
+| BatchSize | TensorRT | Anakin |
+| --- | --- | --- |
+| 1 | 65.6861 | 2.9842 |
+| 2 | 66.6814 | 4.7472 |
+| 4 | 69.7114 | 7.4163 |
+| 8 | 76.1092 | 12.8779 |
+| 32 | 124.9810 | 47.2142 |
+
+- GPU Memory Used (`MB`)
+
+| BatchSize | TensorRT | Anakin |
+| --- | --- | --- |
+| 1 | 341 | 293 |
+| 2 | 353 | 301 |
+| 4 | 385 | 319 |
+| 8 | 421 | 351 |
+| 32 | 637 | 551 |
+
+## How to run those Benchmark models?
+
+> 1. At first, you should parse the caffe model with [`external converter`](https://github.com/PaddlePaddle/Anakin/blob/b95f31e19993a192e7428b4fcf852b9fe9860e5f/docs/Manual/Converter_en.md).
+> 2. Switch to *source_root/benchmark/CNN* directory. Use 'mkdir ./models' to create ./models and put anakin models into this file.
+> 3. Use command 'sh run.sh', we will create files in logs to save model log with different batch size. Finally, model latency summary will be displayed on the screen.
+> 4. If you want to get more detailed information with op time, you can modify CMakeLists.txt with setting `ENABLE_OP_TIMER` to `YES`, then recompile and run. You will find detailed information in model log file.
diff --git a/doc/fluid/new_docs/advanced_usage/deploy/anakin_tutorial.md b/doc/fluid/new_docs/advanced_usage/deploy/anakin_tutorial.md
new file mode 100644
index 0000000000000000000000000000000000000000..5efbc89abd469871b318c306e8cb03dd95f0c85b
--- /dev/null
+++ b/doc/fluid/new_docs/advanced_usage/deploy/anakin_tutorial.md
@@ -0,0 +1,639 @@
+# Anakin 使用教程 ##
+
+本教程将会简略的介绍Anakin的工作原理,一些基本的Anakin API,以及如何调用这些API。
+
+## 内容 ###
+
+- [Anakin的工作原理](#principle)
+- [Anakin APIs](#api)
+- [示例代码](#example)
+
+## Anakin的工作原理 ###
+
+
+
+用Anakin来进行前向计算主要分为三个步骤:
+
+- 将外部模型通过[Anakin Parser](Converter_ch.md)解析为Anakin模型
+ 在使用Anakin之前,用户必须将所有其他模型转换成Anakin模型,我们提供了转换脚本,用户可通过[Anakin Parser](Converter_ch.md)进行模型转换。
+- 生成Anakin计算图
+ 加载Anakin模型生成原始计算图,然后需要对原始计算图进行优化。你只需要调用相应的API优化即可。
+- 执行计算图
+ Anakin会选择不同硬件平台执行计算图。
+
+
+## Anakin APIs ###
+### Tensor ####
+
+`Tensor`提供基础的数据操作和管理,为ops提供统一的数据接口。`Tensor`包含以下几个属性:
+
+- Buffer
+ 数据存储区
+- Shape
+ 数据的维度信息
+- Event
+ 用于异步计算的同步
+
+ `Tensor` 类包含三个`Shape`对象, 分别是`_shape`, `_valid_shape`和 `offset`。 `_shape`为`tensor`真正空间信息,`_valid_shape`表示当前`tensor`使用的空间信息, `_offset`表示当前`tensor`数据指针相对于真正数据空间的信息。 `Tensor`不同维度与分别与数学中的向量、矩阵等相对应如下表所示。
+
+
+Dimentions | Math entity |
+ :----: | :----:
+1 | vector
+2 | matrix
+3 | 3-tensor
+n | n-tensor
+
+#### 声明tensor对象
+
+`Tensor`接受三个模板参数:
+
+
+```c++
+ template
+ class Tensor .../* Inherit other class */{
+ //some implements
+ ...
+ };
+```
+
+TargetType是平台类型,如X86,GPU等等,在Anakin内部有相应的标识与之对应;datatype是普通的数据类型,在Anakin内部也有相应的标志与之对应;[LayOutType](#layout)是数据分布类型,如batch x channel x height x width [NxCxHxW], 在Anakin内部用一个struct来标识。 Anakin中数据类型与基本数据类型的对应如下:
+
+1. TargetType
+
+ Anakin TargetType | platform
+ :----: | :----:|
+ NV | NVIDIA GPU
+ ARM | ARM
+ AMD | AMD GPU
+ X86 | X86
+ NVHX86 | NVIDIA GPU with Pinned Memory
+
+2. DataType
+
+Anakin DataType | C++ | Description
+:---: | :---: | :---: |
+AK_HALF | short | fp16
+AK_FLOAT | float | fp32
+AK_DOUBLE | double | fp64
+AK_INT8 | char | int8
+AK_INT16 | short | int16
+AK_INT32 | int | int32
+AK_INT64 | long | int64
+AK_UINT8 | unsigned char | uint8
+AK_UINT16 | unsigned short | uint8
+AK_UINT32 | unsigned int | uint32
+AK_STRING | std::string | /
+AK_BOOL | bool | /
+AK_SHAPE | / | Anakin Shape
+AK_TENSOR | / | Anakin Tensor
+
+
+3. LayOutType
+
+Anakin LayOutType ( Tensor LayOut ) | Tensor Dimention | Tensor Support | Op Support
+:---: | :---: | :---: | :---: |
+W | 1-D | YES | NO
+HW | 2-D | YES | NO
+WH | 2-D | YES | NO
+NW | 2-D | YES | YES
+NHW | 3-D | YES |YES
+NCHW ( default ) | 4-D | YES | YES
+NHWC | 4-D | YES | NO
+NCHW_C4 | 5-D | YES | YES
+
+
+理论上,Anakin支持申明1维以上的tensor,但是对于Anakin中的Op来说,只支持NW、NHW、NCHW、NCHW_C4这四种LayOut,其中NCHW是默认的LayOutType,NCHW_C4是专门针对于int8这种数据类型的。
+
+
+例子
+
+> 下面的代码将展示如何使用tensor, 我们建议先看看这些示例。
+
+> 要想获得更多关于tensor的信息, 请参考 *soure_path/core/tensor.h*
+
+> 1. 使用shape对象初始化tensor
+``` c++
+ //create a null tensor. A null tensor holds for nothing.
+ //tensor's buffer is resident at CPU and its datatype is AK_FLOAT.
+ //tensor's Layout is NCHW(default)
+ Tensor mytensor;
+
+ //1. using shape object to create a tensor.
+ Shape shape1(NUM); //1-D shape. NUM is the number of dimention.
+ Tensor mytensor1(shape1); //1-D tensor.
+
+ // A 4-D shape
+ Shape shape2(N, C, H, W); // batch x channel x height x width
+```
+
+>`注意:Shape的维度必须和tensor的`[LayoutType](#layout)`相同,比如Shape(N,C,H,W), 那么Tensor的 LayoutType必须是NCHW,否则会出错。如下列代码所示`
+
+
+```c++
+ // A 4-D tensor.
+ Tensor mytensor2(shape2); //right
+
+ //A 4-D tensor which is resident at GPU and its datatype is AK_INT8
+ Tensor mytensor3(shape2); //right
+
+ Tensor mytensor4(shape2); //wrong!! shape's dimetion must be equal to tensor's Layout.
+ Tensor mytensor5(shape2); //wrong!!!!
+
+```
+
+> 2. 使用现有的数据和shape初始化tensor
+
+```c++
+
+ /**
+ * A construtor of Tensor.
+ * data_ptr is a pointer to any data type of data
+ * TargetType is type of a platform [Anakin TargetType]
+ * id : device id
+ * shape: a Anakin shape
+ */
+ Tensor(Dtype* data_ptr, TargetType_t target, int id, Shape shape);
+
+ //using existing data feed to a tensor
+ Tensor mytensor(data_ptr, TargetType, device_id, shape); //shape must has dimention (N, C, H, W).
+
+```
+
+> 3. 使用tensor初始化tensor
+
+```c++
+ Tensor tensor(exist_tensor);
+```
+
+
+> 提示: 你可以用` typedef Tensor Tensor4d_X86 `方便定义tensor
+
+
+#### 填充tensor数据区
+
+
+填充数据区得看你申明tensor的方式, 下面展示了如何填充tensor的数据区。
+
+```c++
+首先来看看tensor的四种声明方式:
+
+1. Tensor mytensor;
+2. Tensor mytensor1(shape1);
+3. Tensor mytensor(data_ptr, TargetType, device_id, shape);
+4. Tensor tensor(exist_tensor);
+
+
+相关的声明方式的数据填充方法如下:
+
+1:声明一个空的tensor,此时没有为其分配内存,所以,我们需要手动的为其分配内存。
+
+ //parama shape
+ mytensor.re_alloc(Shape shape);
+
+ //Get writable pointer to mytensor.
+ //parama index (int): where you start to write.
+ //Dtype is your data type such int, float or double.
+ Dtype *p = mytensor.mutable_data(index/*=0*/);
+ //write data to mytensor
+ for(int i = 0; i < mytensor.size(); i++){
+ p[i] = 1.0f;
+ }
+ //do something ...
+
+2: 这种声明方式会自动分配内存
+
+ //Get writable pointer to mytensor.
+ //parama index (int): where you start to write.
+ //Dtype is your data type such int, float or double.
+ Dtype *p = mytensor1.mutable_data(index/*=0*/);
+ //write data to mytensor
+ for(int i = 0; i < mytensor.size(); i++){
+ p[i] = 1.0f;
+ }
+ //do something ...
+
+
+3:在该种声明方式中,我们仍不需要手动为其分配内存。但在构造函数内部是否为其分配内存,得依情况而定。如果data_ptr和申明的
+tensor都在都一个目标平台上,那么该tensor就会与data_ptr共享内存空间,相反,如果他们不在同一个平台上(如data_ptr在X86上,而
+tensor在GPU上),那么此时tensor就会开辟一个新的内存空间,并将data_ptr所指向的数据拷贝到tensor的buffer中。
+
+ //Get writable pointer to mytensor.
+ //parama index (int): where you start to write.
+ //Dtype is your data type such int, float or double.
+ Dtype *p = mytensor.mutable_data(index/*=0*/);
+ //write data to mytensor
+ for(int i = 0; i < mytensor.size(); i++){
+ p[i] = 1.0f;
+ }
+ //do something ...
+
+4:该种方式仍不需要手动分配内存
+
+ //Get writable pointer to mytensor.
+ //parama index (int): where you start to write.
+ //Dtype is your data type such int, float or double.
+ Dtype *p = mytensor.mutable_data(index/*=0*/);
+ //write data to mytensor
+ for(int i = 0; i < mytensor.size(); i++){
+ p[i] = 1.0f;
+ }
+ //do something ...
+
+
+另外,你还可以获取一个tensor的可读指针,示例如下:
+ //Get read-only pointer to mytensor.
+ //parama index (int): where you start to read.
+ //Dtype is your data type such int, float or double.
+ Dtype *p = mytensor.data(index/*=0*/);
+ //do something ...
+```
+
+如果想更详细的了解tensor,请查阅*soure_path/saber/core/tensor.h*
+
+#### 获取tensor的shape
+
+```c++
+//some declarations
+// ...
+Shape shape = mytensor.shape();
+
+//Get a first dimetion size of tesor, if it has.
+int d1 = shape[0];
+
+//Get a second dimention size of tensor, if it has.
+int d2 = shape[1];
+
+...
+
+//Get a n-th dimention size of tensor, if it has.
+int dn = shape[n-1];
+
+
+//Get a tensor's dimention
+int dims = mytensor.dims();
+
+//Get the size of tensor.
+//size = d1 x d2 x ... x dn.
+int size = mytensor.size();
+
+//Get the size of tensor at interval [Di, Dj)
+// form i-th dimention to j-th dimention, but not including the j-th dimention.
+// which means di x (di+1) x ... x (dj -1)
+int size = mytensor.count(start, end);
+```
+
+#### 设置tensor的shape
+
+我们可以用tensor的成员函数set_shape来设置tensor的shape。 下面是set_shape的定义
+
+
+```c++
+/**
+ * \brief set a tensor's shape
+ * \param valid_shape [a Shape object]
+ * \param shape [a Shape object]
+ * \param offset [a Shape object]
+ * \return the status of this operation, that means whether it success * or not.
+ */
+SaberStatus set_shape(Shape valid_shape, Shape shape = Shape::zero(TensorAPI::layout_dims::value), Shape offset = Shape::minusone(TensorAPI::layout_dims::value));
+```
+
+这个成员函数只设置tensor的shape。这些shape对象(valid_shape, shape, offset)的[LayOutType](#layout)必须和当前的tensor的相应三个shape对象的LayOutType相同,如果不同就会出错,返回SaberInvalidValue。 如果相同,那么将成功设置tensor的shape。
+
+```c++
+
+// some declarations
+// ...
+//valid_shape, shape , offset are Shape object;
+//All these Shape object's LayOutType must be equal to mytensor's.
+mytensor.set_shape(valid_shape, shape, offset);
+
+```
+
+#### 重置 tensor的shape
+
+```c++
+//some declarations
+Shape shape, valid_shape, offset;
+
+//do some initializations
+...
+mytensor.reshape(valid_shape, shape, offset);
+```
+
+注意: Reshape操作仍然需要shape的[LayOutType](#layout) 与tensor的相同
+
+
+### Graph ###
+
+`Graph`类负责加载Anakin模型生成计算图、对图进行优化、存储模型等操作。
+
+#### 图的声明
+
+与`Tensor`一样,graph也接受三个模板参数。
+
+```c++
+
+template
+class Graph ... /* inherit other class*/{
+
+ //some implements
+ ...
+
+};
+```
+
+前面已经介绍过[TargetType](#target)和[DataType](#datatype)是Anakin内部自定义数据类型。[TargetType](#target)表示平台类型 (如NV、X86), [DataType](#datatype)是Anakin基本数据类型与C++/C中的基本数据类型相对应。 [Precision](#precision)为op所支持的精度类型, 稍后我们在介绍它。
+
+
+```c++
+
+//Create a empty graph object.
+Graph graph = Graph tmp();
+
+//Create a pointer to a empty graph.
+Graph *graph = new Graph();
+
+//Create a pointer to a empty graph.
+auto graph = new Graph();
+
+```
+
+#### 加载 Anakin 模型
+
+```c++
+//some declarations
+...
+auto graph = new Graph();
+std::string model_path = "the/path/to/where/your/models/are";
+const char *model_path1 = "the/path/to/where/your/models/are";
+
+//Loading Anakin model to generate a compute graph.
+auto status = graph->load(model_path);
+
+//Or this way.
+auto status = graph->load(model_path1);
+//Check whether load operation success.
+if(!status){
+ std::cout << "error" << endl;
+ //do something...
+}
+
+```
+
+#### 优化计算图
+
+```c++
+//some declarations
+...
+//Load graph.
+...
+//According to the ops of loaded graph, optimize compute graph.
+graph->Optimize();
+
+```
+
+> 注意: 第一次加载原始图,必须要优化。
+
+#### 保存模型
+
+你可以在任何时候保存模型, 特别的, 你可以保存一个优化的模型,这样,下次再加载模型时,就不必进行优化操作。
+
+
+```c++
+//some declarations
+...
+//Load graph.
+...
+// save a model
+//save_model_path: the path to where your model is.
+auto status = graph->save(save_model_path);
+
+//Checking
+if(!status){
+ cout << "error" << endl;
+ //do somethin...
+}
+```
+
+#### 重新设置计算图里的tensor的shape
+
+```c++
+//some declarations
+...
+//Load graph.
+...
+vector shape{10, 256, 256, 10};
+//input_name : std::string.
+//Reshape a tensor named input_name.
+graph->Reshape(input_name, shape);//Note: shape is a vector, not a Shape object.
+```
+
+#### 设置 batch size
+
+`Graph` 支持重新设置batch size的大小。
+
+```c++
+//some declarations
+...
+//Load graph.
+...
+//input_name : std::string.
+//Reset a tensor named input_name.
+int new_batch_size = 4;
+graph->ResetBatchSize(input_name, new_batch_size);
+```
+
+### Net ###
+
+
+`Net` 是计算图的执行器。你可以通过Net对象获得输入和输出
+#### Creating a graph executor
+
+`Net`接受四个模板参数。
+
+
+```c++
+template
+class Net{
+ //some implements
+ ...
+
+};
+```
+由于有些Op可能支持多种精度,我们可以通过Precision来指定。OpRunType表示同步或异步类型,异步是默认类型。OpRunType::SYNC表示同步,在GPU上只有单个流;OpRunType::ASYNC表示异步,在GPU上有多个流并以异步方式执行。实际上,Precision和OpRunType都是enum class, 详细设计请参考*source_root/framework/core/types.h*.
+
+
+1. Precision
+
+Precision | Op support
+:---: | :---:
+Precision::INT4 | NO
+Precision::INT8 | NO
+Precision::FP16 | NO
+Precision::FP32 | YES
+Precision::FP64 | NO
+
+现在Op的精度只支持FP32, 但在将来我们会支持剩下的Precision.
+
+
+
+2. OpRunType
+
+OpRunType | Sync/Aync |Description
+:---: | :---: | :---:
+OpRunType::SYNC | Synchronization | single-stream on GPU
+OpRunType::ASYNC | Asynchronization | multi-stream on GPU
+
+用graph对象创建一个执行器。
+```c++
+//some declarations
+...
+//Create a pointer to a graph.
+auto graph = new Graph();
+//do something...
+...
+
+//create a executor
+Net executor(*graph);
+
+```
+
+#### 获取输入输出tensor
+
+
+获取输入输出tensor,并填充输入tensor的buffer。如果想要获取输入和输出tensor,那么必须指定输入的名字,如"input_0", "input_1", "input_2", ..., 必须传入如上字符串才能够获得输入tensor。另外,如果想知道input_i对应哪个输入,你需要去dash board查看,如何使用dash board请看[Anakin Parser](Converter_ch.md)。请看如下示例代码
+
+```c++
+//some declaratinos
+...
+
+//create a executor
+//TargetType is NV [NVIDIA GPU]
+Net executor(*graph);
+
+//Get the first input tensor.
+//The following tensors(tensor_in0, tensor_in2 ...) are resident at GPU.
+//Note: Member function get_in returns an pointer to tensor.
+Tensor* tensor_in0 = executor.get_in("input_0");
+
+//If you have multiple input tensors
+//You just type this code below.
+Tensor* tensor_in1 = executor.get_in("input_1");
+...
+auto tensor_inn = executor.get_in("input_n");
+```
+
+当得到输入tensor之后,就可以填充它的数据区了。
+
+```c++
+//This tensor is resident at GPU.
+auto tensor_d_in = executor.get_in("input_0");
+
+//If we want to feed above tensor, we must feed the tensor which is resident at host. And then copy the host tensor to the device's one.
+
+//using Tensor4d = Tensor;
+Tensor4d tensor_h_in; //host tensor;
+//Tensor tensor_h_in;
+
+//Allocate memory for host tensor.
+tensor_h_in.re_alloc(tensor_d_in->valid_shape());
+//Get a writable pointer to tensor.
+float *h_data = tensor_h_in.mutable_data();
+
+//Feed your tensor.
+/** example
+for(int i = 0; i < tensor_h_in.size(); i++){
+ h_data[i] = 1.0f;
+}
+*/
+//Copy host tensor's data to device tensor.
+tensor_d_in->copy_from(tensor_h_in);
+
+// And then
+```
+
+
+类似的,我们可以利用成员函数get_out来获得输出tensor。但与获得输入tensor不同的是, 我们需要指定输入tensor结点的名字,这个可以从dash board中看到,请从[Anakin Parser](Converter_ch.md)中查看dash board的使用方法。假如有个输出结点叫pred_out, 那么我们可以通过如下代码获得相应的输出tensor:
+```c++
+//Note: this tensor are resident at GPU.
+Tensor* tensor_out_d = executor.get_out("pred_out");
+
+```
+
+
+#### Executing graph
+
+
+当一切准备就绪后,我们就可以执行真正的计算了!
+```c++
+executor.prediction();
+```
+
+## 示例代码 ##
+
+下面的例子展示了如何调用Anakin。
+
+在这儿之前, 请确保你已经有了Anakin模型。如果还没有,那么请使用[Anakin Parser](Converter_ch.md)转换你的模型。
+
+### Single-thread
+
+单线程例子在 *source_root/test/framework/net/net_exec_test.cpp`*
+
+```c++
+
+std::string model_path = "your_Anakin_models/xxxxx.anakin.bin";
+// Create an empty graph object.
+auto graph = new Graph();
+// Load Anakin model.
+auto status = graph->load(model_path);
+if(!status ) {
+ LOG(FATAL) << " [ERROR] " << status.info();
+}
+// Reshape
+graph->Reshape("input_0", {10, 384, 960, 10});
+// You must optimize graph for the first time.
+graph->Optimize();
+// Create a executer.
+Net net_executer(*graph);
+
+//Get your input tensors through some specific string such as "input_0", "input_1", and
+//so on.
+//And then, feed the input tensor.
+//If you don't know Which input do these specific string ("input_0", "input_1") correspond with, you can launch dash board to find out.
+auto d_tensor_in_p = net_executer.get_in("input_0");
+Tensor4d h_tensor_in;
+auto valid_shape_in = d_tensor_in_p->valid_shape();
+for (int i=0; icopy_from(h_tensor_in);
+
+//Do inference.
+net_executer.prediction();
+
+//Get result tensor through the name of output node.
+//And also, you need to see the dash board again to find out how many output nodes are and remember their name.
+
+//For example, you've got a output node named obj_pre_out
+//Then, you can get an output tensor.
+auto d_tensor_out_0_p = net_executer.get_out("obj_pred_out"); //get_out returns a pointer to output tensor.
+auto d_tensor_out_1_p = net_executer.get_out("lc_pred_out"); //get_out returns a pointer to output tensor.
+//......
+// do something else ...
+//...
+//save model.
+//You might not optimize the graph when you load the saved model again.
+std::string save_model_path = model_path + std::string(".saved");
+auto status = graph->save(save_model_path);
+if (!status ) {
+ LOG(FATAL) << " [ERROR] " << status.info();
+}
+
+```
diff --git a/doc/fluid/new_docs/advanced_usage/deploy/build_and_install_lib_cn.rst b/doc/fluid/new_docs/advanced_usage/deploy/build_and_install_lib_cn.rst
new file mode 100644
index 0000000000000000000000000000000000000000..3884284ea020fe94ed9c03ec84c856ee44aa8c3f
--- /dev/null
+++ b/doc/fluid/new_docs/advanced_usage/deploy/build_and_install_lib_cn.rst
@@ -0,0 +1,99 @@
+.. _install_or_build_cpp_inference_lib:
+
+安装与编译C++预测库
+===========================
+
+直接下载安装
+-------------
+
+====================== ========================================
+版本说明 C++预测库
+====================== ========================================
+cpu_avx_mkl `fluid.tgz `_
+cpu_avx_openblas `fluid.tgz `_
+cpu_noavx_openblas `fluid.tgz `_
+cuda7.5_cudnn5_avx_mkl `fluid.tgz `_
+cuda8.0_cudnn5_avx_mkl `fluid.tgz `_
+cuda8.0_cudnn7_avx_mkl `fluid.tgz `_
+cuda9.0_cudnn7_avx_mkl `fluid.tgz `_
+====================== ========================================
+
+从源码编译
+----------
+用户也可以从 PaddlePaddle 核心代码编译C++预测库,只需在编译时配制下面这些编译选项:
+
+================= =========
+选项 值
+================= =========
+CMAKE_BUILD_TYPE Release
+FLUID_INSTALL_DIR 安装路径
+WITH_FLUID_ONLY ON(推荐)
+WITH_SWIG_PY OFF(推荐
+WITH_PYTHON OFF(推荐)
+WITH_GPU ON/OFF
+WITH_MKL ON/OFF
+================= =========
+
+建议按照推荐值设置,以避免链接不必要的库。其它可选编译选项按需进行设定。
+
+下面的代码片段从github拉取最新代码,配制编译选项(需要将PADDLE_ROOT替换为PaddlePaddle预测库的安装路径):
+
+ .. code-block:: bash
+
+ pip install paddlepaddle-gpu
+ PADDLE_ROOT=/path/of/capi
+ git clone https://github.com/PaddlePaddle/Paddle.git
+ cd Paddle
+ mkdir build
+ cd build
+ cmake -DFLUID_INSTALL_DIR=$PADDLE_ROOT \
+ -DCMAKE_BUILD_TYPE=Release \
+ -DWITH_FLUID_ONLY=ON \
+ -DWITH_SWIG_PY=OFF \
+ -DWITH_PYTHON=OFF \
+ -DWITH_MKL=OFF \
+ -DWITH_GPU=OFF \
+ ..
+ make
+ make inference_lib_dist
+
+成功编译后,使用C++预测库所需的依赖(包括:(1)编译出的PaddlePaddle预测库和头文件;(2)第三方链接库和头文件;(3)版本信息与编译选项信息)
+均会存放于PADDLE_ROOT目录中。目录结构如下:
+
+ .. code-block:: text
+
+ PaddleRoot/
+ ├── CMakeCache.txt
+ ├── paddle
+ │ └── fluid
+ │ ├── framework
+ │ ├── inference
+ │ ├── memory
+ │ ├── platform
+ │ ├── pybind
+ │ └── string
+ ├── third_party
+ │ ├── boost
+ │ │ └── boost
+ │ ├── eigen3
+ │ │ ├── Eigen
+ │ │ └── unsupported
+ │ └── install
+ │ ├── gflags
+ │ ├── glog
+ │ ├── mklml
+ │ ├── protobuf
+ │ ├── snappy
+ │ ├── snappystream
+ │ └── zlib
+ └── version.txt
+
+version.txt 中记录了该预测库的版本信息,包括Git Commit ID、使用OpenBlas或MKL数学库、CUDA/CUDNN版本号,如:
+
+ .. code-block:: text
+
+ GIT COMMIT ID: c95cd4742f02bb009e651a00b07b21c979637dc8
+ WITH_MKL: ON
+ WITH_GPU: ON
+ CUDA version: 8.0
+ CUDNN version: v5
diff --git a/doc/fluid/new_docs/advanced_usage/deploy/convert_paddle_to_anakin.md b/doc/fluid/new_docs/advanced_usage/deploy/convert_paddle_to_anakin.md
new file mode 100644
index 0000000000000000000000000000000000000000..56ca582b2b47f404ede777712830731ea7f4e9b5
--- /dev/null
+++ b/doc/fluid/new_docs/advanced_usage/deploy/convert_paddle_to_anakin.md
@@ -0,0 +1,73 @@
+# 模型转换指南
+
+Anakin 支持不同框架的模型预测。但由于格式的差别,Anakin 需要您预先转换模型。本文档介绍如何转换模型。
+
+## 简介
+
+Anakin 模型转换器输入支持 Caffe 和 Fluid 两种格式的预测模型,模型包含网络结构(model 或 prototxt)和权重参数(param 或 caffemodel)。
+
+模型转换的输出是一个 bin 文件,它作为 Anakin 框架的 graph 参数导入。
+
+您还可以使用模型转换器的 launch board 功能生成网络结构的 HTML 预览。
+
+
+## 系统要求
+
+- python 2.7+
+- pyyaml
+- flask
+- protobuf 3.5+
+
+
+## 用法
+
+### 1、环境
+转换器所需的依赖标注于 *系统要求* 一节。
+
+### 2、配置
+您需要对 *config.yaml* 文件进行修改以告知您的需求。工程中给出了 *config.yaml* 示例,下面作进一步说明。
+
+#### config.yaml
+```bash
+OPTIONS:
+ Framework: CAFFE # 依框架类型填写 CAFFE 或 FLUID
+ SavePath: ./output # 转换结束后模型的保存位置
+ ResultName: googlenet # 输出模型的名字
+ Config:
+ LaunchBoard: ON # 是否生成网络结构预览页面
+ Server:
+ ip: 0.0.0.0
+ port: 8888 # 从一个可用端口访问预览页面
+ OptimizedGraph: # 当您使用了 Anakin 框架的 Optimized 功能时,才应该打开此项
+ enable: OFF
+ path: /path/to/anakin_optimized_anakin_model/googlenet.anakin.bin.saved
+ LOGGER:
+ LogToPath: ./log/ # 生成日志的路径
+ WithColor: ON
+
+TARGET:
+ CAFFE:
+ # 当 Framework 为 CAFFE 时需填写
+ ProtoPaths:
+ - /path/to/caffe/src/caffe/proto/caffe.proto
+ PrototxtPath: /path/to/your/googlenet.prototxt
+ ModelPath: /path/to/your/googlenet.caffemodel
+
+ FLUID:
+ # 当 Framework 为 FLUID 时需填写
+ Debug: NULL
+ ProtoPaths:
+ - /
+ PrototxtPath: /path/to/fluid/inference_model
+ ModelPath: /path/to/fluid/inference_model
+ # ...
+```
+
+### 3、转换
+在完成配置文件的修改后,您只需执行 ```python converter.py``` 就可以进行模型转换了。
+
+
+### 4、预览
+最后一步,就是在浏览器中查看令人振奋的转换结果!网址是在 *config.yaml* 中配置的,例如 http://0.0.0.0:8888 。
+
+> 注意:若您使用了默认的 IP 地址 0.0.0.0,请在预览时使用真实的服务器地址 real_ip:port 替代它。
diff --git a/doc/fluid/new_docs/advanced_usage/deploy/how_to_add_anakin_op.md b/doc/fluid/new_docs/advanced_usage/deploy/how_to_add_anakin_op.md
new file mode 100644
index 0000000000000000000000000000000000000000..f2783eb9f591a31443f2a692ce0eb1bcc9b1063a
--- /dev/null
+++ b/doc/fluid/new_docs/advanced_usage/deploy/how_to_add_anakin_op.md
@@ -0,0 +1,405 @@
+# 如何增加新的Operator
+
+## 基本概念
+
+简单介绍下几个同Operator相关的基本概念,详情请参考设计文档。
+
+```framework```: 上层的逻辑代码,负责从parser中获取参数及weights,添加op时主要修改framework/operator目录下的内容。
+
+```saber```: 底层的实现代码,Anakin通过saber封装了不同的backends,不同的实现(impl)分别特化出自己的实现,外层framework通过不同的template进入各自的impl完成调用。各个op的parameter放在saber/saber_funcs_param.h文件中,增加op主要修改saber/funcs下的内容。
+
+saber的文件结构:
+* saber/funcs下的是各个funcs的外部接口,这一层的op与具体的设备实现无关,只与各op完成的功能有关。由于跟实现(impl)无关,本层文件明均不带impl。
+* saber/funcs/impl下是各个op的impl声明,特定设备需要完成该层声明的特化版本,如saber/funcs/impl/x86实现了上一层impl声明的x86特化版本,saber/funcs/impl/cuda实现了上一层impl声明的NV特化版本。当增加新的backends时需要特化出新的实现。本层代码同实现相关,均带有```impl_```前缀。
+* saber/funcs/impl/cuda/base/cuda_c内有cuda```.cu```扩展名的文件,添加cuda的kernel需要在该文件目录下添加。
+* saber/funcs/impl/cuda/base/sass 内有不同架构的汇编代码编译的静态库。
+
+### 涉及到的基类及各个类之前的关系
+
+简单介绍相关的基类
+
+* ```anakin::Operator```: framework的operator基类,位于framework/core/operator/operator.h
+
+* ```anakin::saber::BaseFunc```: saber对外的op接口基类,提供统一的对外接口,位于saber/funcs/base.h。BaseFunc的```compute_output_shape```接口只根据input的shape和param的参数计算输出的shape,并通过```tensor```的```set_shape```接口(只设置shape,不分配空间)设置到output中。```operator()```接口为各个op的计算接口。
+
+* ```ankain::saber::ImplBase```: saber设备实现的op的接口,所有设备相关实现的基类。位于saber/funcs/impl/impl_base.h。实现版本中这里分为两类,一类以```vender_```为前缀,带有```vender_```代码意为使用第三方库来实现该op,如cudnn的conv,或mkl的conv等等,这类op的性能我们难以调优,因此单独列为一类。另一类是带有源码的saber实现,这些实现都带有```saber_```为前缀,此类实现带有源码,能够通过后续优化不断提升性能,实现起名时需要注意这一点。
+
+## 添加operator
+
+添加一个新的op需要以下几步:
+
+1. 添加saber的param
+2. 定义saber的Operator类
+3. 定义新的impl声明
+3. 完成新的impl实现
+4. 增加framework的实现或特化
+
+接下来就针对这几步,以一个简单例子为例介绍实现。
+
+例如我们要添加新的Mul op。给出计算公式如下:$$Out = alpha \dot X * Y$$
+
+### 为operator增加param
+
+涉及到的文件:```saber/saber_funcs_param.h```。如果之前已经存在需要添加的op的param,这一步可以跳过。
+这里```XXXParam```是一个```struct```。包含一个无参数的构造函数,含参数的构造函数,复制构造函数,```operator=()```及```operator==()```。
+```
+template // 能够获得target, datatype, layout
+struct MulParam{
+ MulParam()
+ : alpha(0)
+ {}
+ MulParam(float alpha_in)
+ : alpha(alpha_in)
+ {}
+ MulParam(const MulParam& right)
+ : alpha(right.alpha)
+ {}
+ MulParam &operator=(const MulParam &right) {
+ alpha = right.alpha;
+ }
+ bool operator==(const MulParam &right) {
+ return alpha == right.alpha;
+ }
+ float alpha;
+};
+```
+
+### 定义Operator类
+涉及到的文件:```saber/funcs/mul.h```。如果之前定义过该op的类,这里需要修改输入的impl定义头文件。
+下面给出一个相对完整的定义结构供参考。
+```
+//不同的设备需要包含对应的operator实现.[详见](#impl)
+#ifdef NVIDIA_GPU
+#include "saber/funcs/impl/cuda/saber_mul.h"
+#include "saber/funcs/impl/cuda/vender_mul.h"
+#endif
+//如果一个设备现在还没有对应的operator实现,需要包含声明。[详见](#declare)
+#ifdef USE_X86_PLACE
+#include "saber/funcs/impl/impl_mul.h"
+#endif
+namespace anakin {
+namespace saber {
+template
+class Mul : public BaseFunc<
+ Tensor,
+ Tensor,
+ Tensor,
+ ImplBase, MulParam> {
+public:
+ using BaseFunc<
+ Tensor,
+ Tensor,
+ Tensor,
+ ImplBase, MulParam>::BaseFunc;
+ Mul() = default;
+ typedef Tensor InDataTensor;
+ typedef Tensor OutDataTensor;
+ typedef Tensor OpTensor;
+ typedef MulParam Param_t;
+ typedef std::vector Input_v;
+ typedef std::vector Output_v;
+ typedef std::vector Shape_v;
+
+ virtual SaberStatus compute_output_shape(const Input_v &input,
+ Output_v &output, Param_t ¶m) override {
+ //计算输出的shape,
+ Shape output_shape = (input[0]->valid_shape());
+ /* code */
+ return output[0]->set_shape(output_shape);
+ }
+ virtual SaberStatus init_impl(ImplEnum implenum) override {
+ // 不同设备均使用此init_impl, 此接口创建对应impl的实现。
+ switch (implenum) {
+ case VENDER_IMPL:
+ this->_impl.push_back(new VenderMul );
+ return SaberSuccess;
+ case SABER_IMPL:
+ this->_impl.push_back(new SaberMul );
+ return SaberSuccess;
+ default:
+ return SaberUnImplError;
+ }
+ }
+private:
+ virtual void pick_best_static() override {
+ if (true) // some condition?
+ this->_best_impl = this->_impl[0];
+ }
+ virtual void pick_best_specify(ImplEnum implenum) override {
+ this->_best_impl = this->_impl[0];
+ }
+};
+} // namespace saber
+} // namespace anakin
+```
+
+### 为operator增加新的impl声明
+
+涉及的文件:```saber/funcs/impl/impl_mul.h```。不同的设备都特化同一个声明,特化版本放在对应的文件夹下,这里的声明就是给出所有设备的统一声明。下面给出一个参考。
+```
+#include "saber/funcs/impl/impl_macro.h"
+namespace anakin{
+namespace saber{
+DEFINE_OP_CLASS(Mul, MulParam); // 第一个参数是op的名字,第二个是对应param的名字
+}
+}
+```
+
+### 完成新的operator特定后端实现
+
+涉及的文件:```saber/funcs/impl/xxx/vender_mul.h```或```saber/funcs/impl/xxx/saber_mul.h```
+这里```xxx```指代特定的一种设备。```vender```是指的使用第三方库实现的op,```saber```指的源码实现的op。这里以cuda的vender实现为例,简单介绍一下特化出的函数的几个基本接口。
+
+```
+// include 对应的声明
+#include "saber/funcs/impl/impl_mul.h"
+
+namespace anakin{
+namespace saber{
+template
+class VenderMul :
+ public ImplBase<
+ Tensor,
+ Tensor,
+ Tensor,
+ MulParam > >
+{
+public:
+ typedef Tensor DataTensor_in;
+ typedef Tensor DataTensor_out;
+ typedef Tensor OpTensor;
+ typedef typename DataTensor_in::Dtype InDataType;
+ typedef typename DataTensor_out::Dtype OutDataType;
+ typedef typename OpTensor::Dtype OpDataType;
+ VenderMul(){}
+ ~VenderMul() {}
+
+ virtual SaberStatus init(const std::vector& inputs,
+ std::vector& outputs,
+ MulParam& param, Context& ctx) {
+ this->_ctx = ctx;
+ create(inputs, outputs, param, ctx);
+ }
+
+ virtual SaberStatus create(const std::vector& inputs,
+ std::vector& outputs,
+ MulParam& param, Context& ctx) {
+ // set内部参数
+ }
+
+ virtual SaberStatus dispatch(const std::vector& inputs,
+ std::vector& outputs,
+ MulParam& param) {
+ // dispatch kernel.
+ }
+
+private:
+};
+}
+}
+```
+```init```和```create```的区别:```init```接口是第一次初始化op的时候进入的接口,此函数只在第一次初始化op时调用,这个接口一般放一些只需要执行一次的代码,如malloc或者create之类的函数。```create```函数除了第一次init执行外,在输入发生变化或者param发生变化时会再次触发,create一般放置set函数,设置内部变量,当input发生变化时这里执行一些同input或weights直接相关的代码。但create因为触发位置在网络内,如果```create```函数执行了一些严重耗时的操作,这里会拖慢整个op的执行时间,需要慎重选择操作放置的位置。
+### 添加framework的特化
+
+涉及的文件:```framework/operators/mul.h```和```framework/operators/mul.cpp```。
+这里简单介绍下如果添加或修改framework内的operator
+
+```
+#include "framework/core/base.h"
+#include "framework/core/data_types.h"
+#include "framework/core/operator/operator.h"
+#include "utils/logger/logger.h"
+#include "saber/funcs/mul.h" // 需要包对应的saber头文件
+namespace anakin {
+namespace ops {
+template
+class MulHelper;
+
+template
+class Mul : public Operator {
+public:
+ Mul() {}
+ /// forward impl
+ virtual void operator() (OpContext &ctx,
+ const std::vector >& ins,
+ std::vector >& outs) {
+ LOG(ERROR) << "Not Impl Yet Operator power::type>().type_info()<<">";
+ }
+ friend class MulHelper;
+};
+template
+class MulHelper : public OperatorHelper {
+public:
+ MulHelper() = default;
+ ~MulHelper();
+ Status InitParam() override;
+
+ Status Init(OpContext &ctx,
+ const std::vector >& ins,
+ std::vector >& outs) override;
+ Status InferShape(const std::vector >& ins,
+ std::vector >& outs) override;
+
+public:
+ saber::MulParam> _param_mul;
+ saber::Mul _funcs_mul;
+};
+}
+} /* namespace anakin */
+```
+对应的```.cpp```文件如下:
+```
+#include "framework/operators/mul.h"
+
+namespace anakin {
+namespace ops {
+
+#ifdef USE_CUDA
+template<>
+void Mul::operator()(
+ OpContext& ctx,
+ const std::vector >& ins,
+ std::vector >& outs) {
+ auto* impl =
+ static_cast*>(this->_helper);
+ auto& param =
+ static_cast*>(this->_helper)->_param_mul;
+ impl->_funcs_mul(ins, outs, param, ctx);
+}
+#endif
+
+template
+Status MulHelper::InitParam() {
+ auto alpha = GET_PARAMETER(float, alpha);
+ MulParam> param_mul(alpha);
+ _param_mul = param_mul;
+ return Status::OK();
+}
+
+template
+Status MulHelper::Init(OpContext& ctx,
+ const std::vector >& ins,
+ std::vector >& outs) {
+
+ SABER_CHECK(_funcs_mul.init(ins, outs, _param_mul, SPECIFY, VENDER_IMPL, ctx));
+ return Status::OK();
+}
+
+template
+Status MulHelper::InferShape(const
+ std::vector >& ins,
+ std::vector >& outs) {
+ SABER_CHECK(_funcs_mul.compute_output_shape(ins, outs, _param_mul));
+ return Status::OK();
+}
+
+#ifdef USE_CUDA
+template class MulHelper;
+#endif
+#ifdef USE_ARM_PLACE
+template class MulHelper;
+#endif
+// register helper
+#ifdef USE_CUDA
+ANAKIN_REGISTER_OP_HELPER(Mul, MulHelper, NV, AK_FLOAT, Precision::FP32);
+#endif
+#ifdef USE_ARM_PLACE
+ANAKIN_REGISTER_OP_HELPER(Mul, MulHelper, ARM, AK_FLOAT, Precision::FP32);
+#endif
+//! register op
+ANAKIN_REGISTER_OP(Mul)
+.Doc("Mul operator")
+#ifdef USE_CUDA
+.__alias__("mul")
+#endif
+#ifdef USE_ARM_PLACE
+.__alias__("mul")
+#endif
+.num_in(1)
+.num_out(1)
+.Args("alpha", " alpha of Mul "); //注册
+
+} /* namespace ops */
+
+} /* namespace anakin */
+```
+
+## 实现单元测试
+涉及的文件:```test/saber/xxx/test_saber_funcs_mul_xxx.cpp```
+在对应的test下需要添加新的单元测试
+
+```
+TEST(TestSaberFuncNV, test_depthwise_conv) {
+
+ // init tensors and some param.
+
+ // start Reshape & doInfer
+ Context ctx1(0, 1, 1);
+
+ // create param
+ MulParam > param(alpha);
+
+ std::vector*> input;
+ std::vector*> output;
+
+ // create saber op
+ Mul mul;
+
+ // compute output shape
+ mul.compute_output_shape(input, output, param);
+
+ // re_alloc output tensors memory based on output shape
+ output[0]->re_alloc(output[0]->shape());
+
+ // init saber op(calling init and create)
+ mul.init(input, output, param, SPECIFY, VENDER_IMPL, ctx1);
+
+ // call operator()
+ mul(input, output, param, ctx1);
+
+ // cuda specified, record events
+ cudaStream_t cuda_stream = ctx1.get_compute_stream();
+ output[0]->record_event(cuda_stream);
+ output_dev.sync();
+
+ // param changed
+ param.alpha = 2.0;
+ // auto calling saber op(create and dispatch)
+ mul(input, output, param, ctx1);
+
+ cudaDeviceSynchronize();
+ CUDA_CHECK(cudaPeekAtLastError());
+}
+
+int main(int argc, const char** argv){
+ anakin::saber::Env::env_init();
+
+ // initial logger
+ //logger::init(argv[0]);
+ InitTest();
+ RUN_ALL_TESTS(argv[0]);
+ return 0;
+}
+
+```
+## 调试及注意事项
+
+一个op需要有对外的op接口和内部实现,由于存在saber/funcs/impl的非特化版本声明,当有op在某种设备下没有对应实现时,也能够编译,但此时是没有任何实现的空实现,
diff --git a/doc/fluid/new_docs/advanced_usage/deploy/how_to_support_new_device_in_anakin.md b/doc/fluid/new_docs/advanced_usage/deploy/how_to_support_new_device_in_anakin.md
new file mode 100644
index 0000000000000000000000000000000000000000..a1f75f5e95cfb90f26d3782ba30a6d1887a70424
--- /dev/null
+++ b/doc/fluid/new_docs/advanced_usage/deploy/how_to_support_new_device_in_anakin.md
@@ -0,0 +1,459 @@
+# 如何支持一个新的设备
+
+## 概览
+
+添加一个新的设备需要以下3个步骤:
+
+* [在`CMakeList`中添加设备的支持](#0001)
+* [在`saber`中添加设备的实现](#0002)
+* [在`framework`中添加设备的具体化或实例化](#0003)
+
+假设新设备的名称为`TNEW`, 以下将以这个设备名称进行演示。
+
+## 在`CMakeList`中添加设备的支持 ##
+
+* 修改根目录`CMakeList.txt`
+```cmake
+#select the plantform to build
+anakin_option(USE_GPU_PLACE "Select the build mode for GPU place." NO)
+anakin_option(USE_X86_PLACE "Select the build mode for X86 place." NO)
+anakin_option(USE_ARM_PLACE "Select the build mode for ARM place." NO)
+anakin_option(USE_TNEW_PLACE "Select the build mode for ARM place." YES)
+```
+
+* 修改`saber/CMakeList.txt`
+
+根据新增设备的目录完善`saber`目录下的`CMakeList.txt`。
+```cmake
+if(USE_TNEW_PLACE)
+ anakin_fetch_files_with_suffix(${ANAKIN_SABER}/core/impl/tnew "cpp" ANAKIN_SABER_BASE_SRC)
+ anakin_fetch_files_with_suffix(${ANAKIN_SABER}/funcs/impl/tnew "cpp" ANAKIN_SABER_BASE_SRC)
+endif()
+```
+
+* 修改`test/CMakeList.txt`
+
+新增设备的单测文件放在`test/saber/tnew`目录下,修改`test`目录下的`CMakeList.txt`。
+```cmake
+if(USE_TNEW_PLACE)
+ anakin_fetch_files_with_suffix(${ANAKIN_UNIT_TEST}/saber/tnew "cpp" ANAKIN_TEST_CASE_SRC)
+endif()
+```
+
+* 修改`cmake/anakin_config.h.in`
+```c++
+// plantform to use
+#cmakedefine USE_GPU_PLACE
+
+#cmakedefine USE_X86_PLACE
+
+#cmakedefine USE_ARM_PLACE
+
+#cmakedefine USE_TNEW_PLACE
+```
+
+* 其他依赖和编译选项
+修改`cmake`目录下的`compiler_options.cmake`和`find_modules.cmake`
+
+
+## 在`saber`中添加设备的实现 ##
+`saber`是`Anakin`的基础计算库,对外提供设备无关的统一的API,设备相关的实现都会封装到`TargetWrapper`中。
+
+### 在`saber/saber_types.h`中添加设备
+
+```c++
+enum TargetTypeEnum {
+ eINVALID = -1,
+ eNV = 1,
+ eAMD = 2,
+ eARM = 3,
+ eX86 = 4,
+ eNVHX86 = 5,
+ eTNEW = 6
+};
+
+typedef TargetType NV;
+typedef TargetType ARM;
+typedef TargetType AMD;
+typedef TargetType X86;
+typedef TargetType TNEW;
+
+```
+
+### 在`saber/core`中添加设备的实现
+
+1. 在`target_traits.h`中添加新设备
+
+* 增加设备类型
+```c++
+struct __cuda_device{};
+struct __arm_device{};
+struct __amd_device{};
+struct __x86_device{};
+struct __tnew_device{};
+```
+
+* `TargetTypeTraits`模板具体化
+```c++
+template <>
+struct TargetTypeTraits {
+ typedef __xxx_target target_category;//根据实际设备是host端还是device端进行选择
+ typedef __tnew_device target_type;
+};
+```
+
+2. 在`data_traits.h`中特化`DataTrait`模板类
+
+如果设备需要特殊的数据类型,则特化出设备的`DataTrait`类的实现,例如opencl数据类型的实现如下:
+```c++
+#ifdef USE_OPENCL
+struct ClMem{
+ ClMem(){
+ dmem = nullptr;
+ offset = 0;
+ }
+
+ ClMem(cl_mem* mem_in, int offset_in = 0) {
+ dmem = mem_in;
+ offset = offset_in;
+ }
+
+ ClMem(ClMem& right) {
+ dmem = right.dmem;
+ offset = right.offset;
+ }
+
+ ClMem& operator=(ClMem& right) {
+ this->dmem = right.dmem;
+ this->offset = right.offset;
+ return *this;
+ }
+
+ ClMem& operator+(int offset_in) {
+ this->offset += offset_in;
+ return *this;
+ }
+
+ int offset{0};
+ cl_mem* dmem;
+};
+
+template <>
+struct DataTrait {
+ typedef ClMem Dtype;
+ typedef float dtype;
+};
+
+template <>
+struct DataTrait {
+ typedef ClMem Dtype;
+ typedef double dtype;
+};
+
+template <>
+struct DataTrait {
+ typedef ClMem Dtype;
+ typedef char dtype;
+};
+#endif //use_opencl
+```
+
+3. 在`target_wrapper.h`中特化`TargetWrapper`模板类
+
+特化`TargetWrapper`模板类,在`target_wrapper.h`中声明函数,具体如下:
+```c++
+template <>
+struct TargetWrapper { //根据TNEW的具体类型修改__xxx_target,__host_target或者__device_target
+
+ typedef xxx_event event_t; //根据设备实现xxx_event
+ typedef xxx_stream stream_t; //根据设备实现xxx_stream
+
+ static void get_device_count(int& count);
+
+ static void set_device(int id);
+
+ //We should add strategy to avoid malloc directly
+ static void mem_alloc(void** ptr, size_t n);
+
+ static void mem_free(void* ptr);
+
+ static void mem_set(void* ptr, int value, size_t n);
+
+ static void create_event(event_t& event, bool flag = false);
+
+ static void create_stream(stream_t& stream);
+
+ static void create_stream_with_flag(stream_t& stream, unsigned int flag);
+
+ static void create_stream_with_priority(stream_t& stream, unsigned int flag, int priority);
+
+ static void destroy_stream(stream_t& stream);
+
+ static void destroy_event(event_t& event);
+
+ static void record_event(event_t& event, stream_t stream);
+
+ static void query_event(event_t& event);
+
+ static void sync_event(event_t& event);
+
+ static void sync_stream(event_t& event, stream_t& stream);
+
+ static void sync_memcpy(void* dst, int dst_id, const void* src, int src_id, \
+ size_t count, __DtoD);
+
+ static void async_memcpy(void* dst, int dst_id, const void* src, int src_id, \
+ size_t count, stream_t& stream, __DtoD);
+
+ static void sync_memcpy(void* dst, int dst_id, const void* src, int src_id, \
+ size_t count, __HtoD);
+
+ static void async_memcpy(void* dst, int dst_id, const void* src, int src_id, \
+ size_t count, stream_t& stream, __HtoD);
+
+ static void sync_memcpy(void* dst, int dst_id, const void* src, int src_id, \
+ size_t count, __DtoH);
+
+ static void async_memcpy(void* dst, int dst_id, const void* src, int src_id, \
+ size_t count, stream_t& stream, __DtoH);
+
+ static void sync_memcpy_p2p(void* dst, int dst_dev, const void* src, \
+ int src_dev, size_t count);
+
+ static void async_memcpy_p2p(void* dst, int dst_dev, const void* src, \
+ int src_dev, size_t count, stream_t& stream);
+
+ static int get_device_id();
+};
+
+```
+
+4. 在`impl/`目录下添加设备目录和实现
+
+在`saber/core/impl`目录下添加设备目录`tnew`。
+* 实现`TargetWrapper`结构体中各函数的定义。
+如果`TargetWrapper`的实现与默认的模板类一致,则不用特化出该类。
+
+```c++
+typedef TargetWrapper TNEW_API;
+void TNEW_API::get_device_count(int &count) {
+ // add implementation
+}
+
+void TNEW_API::set_device(int id){
+ // add implementation
+}
+
+void TNEW_API::mem_alloc(void** ptr, size_t n){
+ // add implementation
+}
+
+void TNEW_API::mem_free(void* ptr){
+ if(ptr != nullptr){
+ // add implementation
+ }
+}
+...
+
+```
+
+* 特化实现`device.h`中的`Device`
+
+```c++
+template <>
+void Device::create_stream() {
+ // add implementation
+}
+
+template <>
+void Device::get_info() {
+
+ // add implementation
+}
+
+```
+
+### 在`saber/funcs`中实现设备相关的op
+
+参考[如何增加新的Operator](addCustomOp.md)
+
+
+## 在`framework`中添加设备的具体化或实例化 ##
+
+### `framework/core`
+
+* `net.cpp`中添加实例化
+
+```c++
+#ifdef USE_TNEW_PLACE
+template class Net;
+template class Net;
+#endif
+```
+
+* `operator_func.cpp`中添加实例化
+
+```c++
+#ifdef USE_TNEW_PLACE
+template class OperatorFunc;
+#endif
+```
+
+* `worker.cpp`中添加实例化
+
+```c++
+#ifdef USE_TNEW_PLACE
+template class Worker;
+template class Worker;
+#endif
+```
+
+* `operator_attr.cpp`中添加实例化
+
+```c++
+template
+OpAttrWarpper& OpAttrWarpper::__alias__(const std::string& op_name);
+template
+OpAttrWarpper& OpAttrWarpper::__alias__(const std::string& op_name);
+template
+OpAttrWarpper& OpAttrWarpper::__alias__(const std::string& op_name);
+```
+
+* `parameter.h`中添加设备的实现
+
+```c++
+#ifdef USE_TNEW_PLACE
+template
+class PBlock {
+public:
+ typedef Tensor4d::type> type;
+
+ PBlock() {
+ _inner_tensor = std::make_shared();
+ }
+ ...
+}
+#endif //TNEW
+```
+
+* `type_traits_extend.h`中添加设备的实现
+
+```c++
+template<>
+struct target_host {
+ typedef saber::X86 type; //根据TNEW选择正确的host type
+};
+```
+
+### `framework/graph`
+
+* `graph.cpp`中添加实例化
+
+```c++
+ #ifdef USE_TNEW_PLACE
+ template class Graph;
+ template class Graph;
+ template class Graph;
+ #endif
+```
+
+### `framework/model_parser`
+
+* `parser.cpp`中添加实例化
+
+```c++
+ #ifdef USE_TNEW_PLACE
+ template
+ Status load(graph::Graph* graph,
+ const char* model_path);
+ template
+ Status load(graph::Graph* graph,
+ const char* model_path);
+ template
+ Status load(graph::Graph* graph,
+ const char* model_path);
+
+ template
+ Status save(graph::Graph* graph,
+ std::string& model_path);
+ template
+ Status save(graph::Graph* graph,
+ std::string& model_path);
+ template
+ Status save(graph::Graph* graph,
+ std::string& model_path);
+
+ template
+ Status load(graph::Graph* graph,
+ std::string& model_path);
+ template
+ Status load(graph::Graph* graph,
+ std::string& model_path);
+ template
+ Status load(graph::Graph* graph,
+ std::string& model_path);
+
+ template
+ Status save(graph::Graph* graph,
+ const char* model_path);
+ template
+ Status save(graph::Graph* graph,
+ const char* model_path);
+ template
+ Status save(graph::Graph* graph,
+ const char* model_path);
+ #endif
+```
+
+* `model_io.cpp`中添加实例化
+
+```c++
+#ifdef USE_TNEW_PLACE
+template class NodeIO;
+template class NodeIO;
+template class NodeIO;
+#endif
+```
+
+### `framework/operators`
+
+为`framework/operators`目录下所有op添加实例化或具体化
+以`activation.cpp`为例,实例化如下:
+
+```c++
+#ifdef USE_TNEW_PLACE
+INSTANCE_ACTIVATION(TNEW, AK_FLOAT, Precision::FP32);
+INSTANCE_ACTIVATION(TNEW, AK_FLOAT, Precision::FP16);
+INSTANCE_ACTIVATION(TNEW, AK_FLOAT, Precision::INT8);
+template class ActivationHelper;
+ANAKIN_REGISTER_OP_HELPER(Activation, ActivationHelper, TNEW, AK_FLOAT, Precision::FP32);
+#endif
+```
+
+如果TNEW设备函数的实现与现有模板实现不一致,可以特化实现如下(以init()为例):
+```c++
+#ifdef USE_TNEW_PLACE
+INSTANCE_ACTIVATION(TNEW, AK_FLOAT, Precision::FP32);
+INSTANCE_ACTIVATION(TNEW, AK_FLOAT, Precision::FP16);
+INSTANCE_ACTIVATION(TNEW, AK_FLOAT, Precision::INT8);
+template <>
+Status ActivationHelper::Init(OpContext &ctx,\
+ const std::vector >& ins, \
+ std::vector >& outs) {
+ SABER_CHECK(_funcs_activation.init(ins, outs, _param_activation, SPECIFY, SABER_IMPL, ctx)); //在这里选择实现方式
+ return Status::OK();
+}
+ANAKIN_REGISTER_OP_HELPER(Activation, ActivationHelper, TNEW, AK_FLOAT, Precision::FP32);
+#endif
+```
+
+在`ANAKIN_REGISTER_OP(Activation)`中添加TNEW的注册
+
+```c++
+#ifdef USE_TNEW_PLACE
+.__alias__("activation")
+#endif
+```
+
+## 注意事项
+不要修改`Tensor`/`Buffer`/`Env`/`Context`这些类函数的接口和实现
diff --git a/doc/fluid/new_docs/advanced_usage/deploy/index_anakin.rst b/doc/fluid/new_docs/advanced_usage/deploy/index_anakin.rst
new file mode 100644
index 0000000000000000000000000000000000000000..b782242a6632a5d42a512cf3b830d6e047c064ab
--- /dev/null
+++ b/doc/fluid/new_docs/advanced_usage/deploy/index_anakin.rst
@@ -0,0 +1,26 @@
+服务器端部署 - Anakin
+#####################
+
+
+使用文档
+~~~~~~~
+
+.. toctree::
+ :maxdepth: 1
+
+ install_anakin.md
+ convert_paddle_to_anakin.md
+ run_anakin_on_arm.md
+ anakin_tutorial.md
+ anakin_example.md
+ anakin_gpu_benchmark.md
+ anakin_arm_benchmark.md
+
+开发文档
+~~~~~~~
+
+.. toctree::
+ :maxdepth: 1
+
+ how_to_add_anakin_op.md
+ how_to_support_new_device_in_anakin.md
diff --git a/doc/fluid/new_docs/advanced_usage/deploy/index_mobile.rst b/doc/fluid/new_docs/advanced_usage/deploy/index_mobile.rst
new file mode 100644
index 0000000000000000000000000000000000000000..47df6392c123d520c701089db6ee1ae72e4f8ea5
--- /dev/null
+++ b/doc/fluid/new_docs/advanced_usage/deploy/index_mobile.rst
@@ -0,0 +1,9 @@
+移动端部署
+##########
+
+.. toctree::
+ :maxdepth: 2
+
+ mobile_build.md
+ mobile_dev.md
+
diff --git a/doc/fluid/new_docs/advanced_usage/deploy/index_native.rst b/doc/fluid/new_docs/advanced_usage/deploy/index_native.rst
new file mode 100644
index 0000000000000000000000000000000000000000..a5209e8560b31e9f0f776fba9a2b8c5bc150165c
--- /dev/null
+++ b/doc/fluid/new_docs/advanced_usage/deploy/index_native.rst
@@ -0,0 +1,8 @@
+服务器端部署 - 原生引擎
+#######################
+
+.. toctree::
+ :maxdepth: 2
+
+ build_and_install_lib_cn.rst
+ native_infer.rst
diff --git a/doc/fluid/new_docs/advanced_usage/deploy/install_anakin.md b/doc/fluid/new_docs/advanced_usage/deploy/install_anakin.md
new file mode 100644
index 0000000000000000000000000000000000000000..bb7c1950308622e3de292268a718e6ec688e6ae6
--- /dev/null
+++ b/doc/fluid/new_docs/advanced_usage/deploy/install_anakin.md
@@ -0,0 +1,69 @@
+## 从源码编译安装Anakin ##
+
+我们已经在CentOS 7.3上成功的安装和测试了Anakin,对于其他操作系统,我们将很快支持。
+
+### 安装概览 ###
+
+* [在CentOS上安装 Anakin]()
+* [在Ubuntu上安装 Anakin]()
+* [在ARM上安装 Anakin](run_on_arm_ch.md)
+* [验证安装]()
+
+
+### 在CentOS上安装 Anakin ###
+#### 1. 系统要求 ####
+
+* make 3.82+
+* cmake 2.8.12+
+* gcc 4.8.2+
+* g++ 4.8.2+
+* 其他需要补充的。。。
+
+#### 2. 编译CPU版Anakin ####
+
+暂时不支持
+
+#### 3. 编译支持NVIDIA GPU的Anakin ####
+
+- 3.1. 安装依赖
+ - 3.1.1 protobuf
+ >$ git clone https://github.com/google/protobuf
+ >$ cd protobuf
+ >$ git submodule update --init --recursive
+ >$ ./autogen.sh
+ >$ ./configure --prefix=/path/to/your/insall_dir
+ >$ make
+ >$ make check
+ >$ make install
+ >$ sudo ldconfig
+
+
+ 如安装protobuf遇到任何问题,请访问[这里](https://github.com/google/protobuf/blob/master/src/README.md)
+
+- 3.2 CUDA Toolkit
+ - [CUDA 8.0](https://developer.nvidia.com/cuda-zone) or higher. 具体信息参见[NVIDIA's documentation](https://docs.nvidia.com/cuda/cuda-installation-guide-linux/).
+ - [cuDNN v7](https://developer.nvidia.com/cudnn). 具体信息参见[NVIDIA's documentation](https://docs.nvidia.com/cuda/cuda-installation-guide-linux/).
+- 3.3 编译Anakin
+ >$ git clone https:/xxxxx
+ >$ cd anakin
+ >$ mkdir build
+ >$ camke ..
+ >$ make
+
+
+#### 4. 编译支持AMD GPU的Anakin ####
+
+暂时还不支持
+
+
+### 在Ubuntu上安装 Anakin ###
+
+暂时还不支持
+
+
+### 在ARM上安装 Anakin ###
+
+暂时还不支持
+
+### 验证安装 ###
+we are coming soon...
diff --git a/doc/fluid/new_docs/advanced_usage/deploy/mobile_build.md b/doc/fluid/new_docs/advanced_usage/deploy/mobile_build.md
new file mode 100644
index 0000000000000000000000000000000000000000..e51593164987d548e256ddebbc5fa8d960fb5255
--- /dev/null
+++ b/doc/fluid/new_docs/advanced_usage/deploy/mobile_build.md
@@ -0,0 +1,59 @@
+# 环境搭建
+## 使用 docker
+### 1. 安装 docker
+安装 docker 的方式,参考官方文档 [https://docs.docker.com/install/](https://docs.docker.com/install/)
+### 2. 使用 docker 搭建构建环境
+首先进入 paddle-mobile 的目录下,执行 `docker build`
+以 Linux/Mac 为例 (windows 建议在 'Docker Quickstart Terminal' 中执行)
+```
+$ docker build -t paddle-mobile:dev - < Dockerfile
+```
+使用 `docker images` 可以看到我们新建的 image
+```
+$ docker images
+REPOSITORY TAG IMAGE ID CREATED SIZE
+paddle-mobile dev 33b146787711 45 hours ago 372MB
+```
+### 3. 使用 docker 构建
+进入 paddle-mobile 目录,执行 docker run
+```
+$ docker run -it --mount type=bind,source=$PWD,target=/paddle-mobile paddle-mobile:dev
+root@5affd29d4fc5:/ # cd /paddle-mobile
+# 生成构建 android 产出的 Makefile
+root@5affd29d4fc5:/ # rm CMakeCache.txt
+root@5affd29d4fc5:/ # cmake -DCMAKE_TOOLCHAIN_FILE=tools/toolchains/arm-android-neon.cmake
+# 生成构建 linux 产出的 Makefile
+root@5affd29d4fc5:/ # rm CMakeCache.txt
+root@5affd29d4fc5:/ # cmake -DCMAKE_TOOLCHAIN_FILE=tools/toolchains/arm-linux-gnueabi.cmake
+```
+### 4. 设置编译选项
+可以通过 ccmake 设置编译选项
+```
+root@5affd29d4fc5:/ # ccmake .
+ Page 1 of 1
+ CMAKE_ASM_FLAGS
+ CMAKE_ASM_FLAGS_DEBUG
+ CMAKE_ASM_FLAGS_RELEASE
+ CMAKE_BUILD_TYPE
+ CMAKE_INSTALL_PREFIX /usr/local
+ CMAKE_TOOLCHAIN_FILE /paddle-mobile/tools/toolchains/arm-android-neon.cmake
+ CPU ON
+ DEBUGING ON
+ FPGA OFF
+ LOG_PROFILE ON
+ MALI_GPU OFF
+ NET googlenet
+ USE_EXCEPTION ON
+ USE_OPENMP OFF
+```
+修改选项后,按 `c`, `g` 更新 Makefile
+### 5. 构建
+使用 make 命令进行构建
+```
+root@5affd29d4fc5:/ # make
+```
+### 6. 查看构建产出
+构架产出可以在 host 机器上查看,在 paddle-mobile 的目录下,build 以及 test/build 下,可以使用 adb 指令或者 scp 传输到 device 上执行
+
+## 不使用 docker
+不使用 docker 的方法,可以直接用 cmake 生成 makefile 后构建。使用 ndk 构建 android 应用需要正确设置 NDK_ROOT。构建 linux 应用需要安装 arm-linux-gnueabi-gcc 或者类似的交叉编译工具,可能需要设置 CC,CXX 环境变量,或者在 tools/toolchains/ 中修改 arm-linux-gnueabi.cmake,或者增加自己需要的 toolchain file。
diff --git a/doc/fluid/new_docs/advanced_usage/deploy/mobile_dev.md b/doc/fluid/new_docs/advanced_usage/deploy/mobile_dev.md
new file mode 100644
index 0000000000000000000000000000000000000000..474380f9dbfd2fb8a06630cb1ca3ca5cd14ca9d9
--- /dev/null
+++ b/doc/fluid/new_docs/advanced_usage/deploy/mobile_dev.md
@@ -0,0 +1,72 @@
+# iOS开发文档
+
+## 编译
+
+### 一. 使用 build.sh 编译
+
+```sh
+sh build.sh ios
+
+# 如果只想编译某个特定模型的 op, 则需执行以下命令
+sh build.sh ios googlenet
+
+# 在这个文件夹下, 你可以拿到生成的 .a 库
+cd ../build/release/ios/build
+
+```
+
+### 二. 使用 xcode 编译
+
+我们提供了 ios 开发更为熟悉的 xcode 编译环境:
+在 ios/ 目录下打开 PaddleMobile.xcworkspace 即可编译 PaddleMobile 或者 运行 Demo
+
+### 三. 集成
+
+#### 如使用 c++ 接口
+将
+
+```
+libpaddle-mobile.a
+io.h
+program.h
+types.h
+lod_tensor.h
+tensor.h
+```
+拖入工程, io.h 为接口文件, 可在 [github](https://github.com/PaddlePaddle/paddle-mobile/blob/develop/src/io/io.h)上查看接口注释
+
+#### 如使用 oc 接口
+将在xcode 编译生成的
+```
+libPaddleMobile.a
+PaddleMobile.h
+```
+拖入工程, 接口如下:
+
+```
+/*
+ 创建单例对象
+*/
++ (instancetype)sharedInstance;
+
+/*
+ load 模型, 开辟内存
+*/
+- (BOOL)load:(NSString *)modelPath andWeightsPath:(NSString *)weighsPath;
+
+/*
+ 进行预测, means 和 scale 为训练模型时的预处理参数, 如训练时没有做这些预处理则直接使用 predict
+*/
+- (NSArray *)predict:(CGImageRef)image means:(NSArray *)means scale:(float)scale;
+
+/*
+ 进行预测
+*/
+- (NSArray *)predict:(CGImageRef)image;
+
+/*
+ 清理内存
+*/
+- (void)clear;
+
+```
diff --git a/doc/fluid/new_docs/advanced_usage/deploy/native_infer.rst b/doc/fluid/new_docs/advanced_usage/deploy/native_infer.rst
new file mode 100644
index 0000000000000000000000000000000000000000..e1eee3f818796e895362caab10846cf59b557162
--- /dev/null
+++ b/doc/fluid/new_docs/advanced_usage/deploy/native_infer.rst
@@ -0,0 +1,108 @@
+Paddle 预测 API
+===============
+
+为了更简单方便的预测部署,Fluid 提供了一套高层 API
+用来隐藏底层不同的优化实现。
+
+`预测库相关代码 `__
+包括
+
+- 头文件 ``paddle_inference_api.h`` 定义了所有的接口
+- 库文件\ ``libpaddle_fluid.so`` 或 ``libpaddle_fluid.a``
+- 库文件 ``libpaddle_inference_api.so`` 或
+ ``libpaddle_inference_api.a``
+
+编译和依赖可以参考 :ref:`install_or_build_cpp_inference_lib` 。
+
+下面是一些 API 概念的介绍
+
+PaddleTensor
+------------
+
+PaddleTensor 定义了预测最基本的输入输出的数据格式,其定义是
+
+.. code:: cpp
+
+ struct PaddleTensor {
+ std::string name; // variable name.
+ std::vector shape;
+ PaddleBuf data; // blob of data.
+ PaddleDType dtype;
+ };
+
+- ``name`` 用于指定输入数据对应的 模型中variable 的名字
+ (暂时没有用,但会在后续支持任意 target 时启用)
+- ``shape`` 表示一个 Tensor 的 shape
+- ``data`` 数据以连续内存的方式存储在\ ``PaddleBuf``
+ 中,\ ``PaddleBuf``
+ 可以接收外面的数据或者独立\ ``malloc``\ 内存,详细可以参考头文件中相关定义。
+- ``dtype`` 表示 Tensor 的数据类型
+
+engine
+------
+
+高层 API 底层有多种优化实现,我们称之为 engine,目前有三种 engine
+
+- 原生 engine,由 paddle 原生的 forward operator
+ 组成,可以天然支持所有paddle 训练出的模型,
+- Anakin engine,封装了
+ `Anakin `__
+ ,在某些模型上性能不错,但只能接受自带模型格式,无法支持所有 paddle
+ 模型,
+- TensorRT mixed engine,用子图的方式支持了
+ `TensorRT `__ ,支持所有paddle
+ 模型,并自动切割部分计算子图到 TensorRT 上加速(WIP)
+
+其实现为
+
+.. code:: cpp
+
+ enum class PaddleEngineKind {
+ kNative = 0, // Use the native Fluid facility.
+ kAnakin, // Use Anakin for inference.
+ kAutoMixedTensorRT // Automatically mixing TensorRT with the Fluid ops.
+ };
+
+预测部署过程
+------------
+
+总体上分为以下步骤
+
+1. 用合适的配置创建 ``PaddlePredictor``
+2. 创建输入用的 ``PaddleTensor``\ ,传入到 ``PaddlePredictor`` 中
+3. 获取输出的 ``PaddleTensor`` ,将结果取出
+
+下面完整演示一个简单的模型,部分细节代码隐去
+
+.. code:: cpp
+
+ #include "paddle_inference_api.h"
+
+ // 创建一个 config,并修改相关设置
+ paddle::NativeConfig config;
+ config.model_dir = "xxx";
+ config.use_gpu = false;
+ // 创建一个原生的 PaddlePredictor
+ auto predictor =
+ paddle::CreatePaddlePredictor(config);
+ // 创建输入 tensor
+ int64_t data[4] = {1, 2, 3, 4};
+ paddle::PaddleTensor tensor{.name = "",
+ .shape = std::vector({4, 1}),
+ .data = PaddleBuf(data, sizeof(data)),
+ .dtype = PaddleDType::INT64};
+ // 创建输出 tensor,输出 tensor 的内存可以复用
+ std::vector outputs;
+ // 执行预测
+ CHECK(predictor->Run(slots, &outputs));
+ // 获取 outputs ...
+
+编译时,联编 ``libpaddle_fluid.a/.so`` 和
+``libpaddle_inference_api.a/.so`` 便可。
+
+详细代码参考
+------------
+
+- `inference
+ demos `__
+- `复杂单线程/多线程例子 `__
diff --git a/doc/fluid/new_docs/advanced_usage/deploy/run_anakin_on_arm.md b/doc/fluid/new_docs/advanced_usage/deploy/run_anakin_on_arm.md
new file mode 100644
index 0000000000000000000000000000000000000000..ebeb38f534ebfc8cb5a41d103abe3bb1de7e379a
--- /dev/null
+++ b/doc/fluid/new_docs/advanced_usage/deploy/run_anakin_on_arm.md
@@ -0,0 +1,151 @@
+## 源码编译 Anakin ##
+
+目前Anakin支持ARM Android平台,采用Android NDK交叉编译工具链,已在mac os和centos上编译和测试通过。
+
+### 安装概览 ###
+
+* [系统需求](#0001)
+* [安装第三方依赖](#0002)
+* [Anakin源码编译](#0003)
+* [验证安装](#0004)
+
+
+### 1. 系统需求 ###
+
+* 宿主机: linux, mac
+* cmake 3.8.2+
+* Android NDK r14, Linux 版本[从这里下载](https://dl.google.com/android/repository/android-ndk-r14b-linux-x86_64.zip)
+
+### 2. 安装第三方依赖 ###
+
+- 2.1 protobuf3.4.0
+ 源码从这里[下载](https://github.com/google/protobuf/releases/tag/v3.4.0)
+ - 2.1.1 为宿主机编译protobuf
+ ```bash
+ $ tar -xzf protobuf-3.4.0.tar.gz
+ $ cd protobuf-3.4.0
+ $ ./autogen.sh
+ $ ./configure
+ $ make
+ $ make check
+ $ make install
+ ```
+ 上述 $make install 执行后,可在 /usr/local/include/google 找到 libprotobuf 所需的头文件,将整个google文件夹拷贝至Anakin/third-party/arm-android/protobuf/下,
+ 如有问题,请点[这里](https://github.com/google/protobuf/blob/v3.4.0/src/README.md)。
+ 然后将已经生成文件清除。
+ ```bash
+ $ make distclean
+ ```
+ - 2.1.1 交叉编译Android`armeabi-v7a`的protobuf,注意设置ANDROID_NDK的路径,以及ARCH_ABI、HOSTOSN的值,
+ ```bash
+
+ $ export ANDROID_NDK=your_ndk_path
+ $ ARCH_ABI="arm-linux-androideabi-4.9"
+ $ HOSTOSN="darwin-x86_64"
+ $ export SYSROOT=$ANDROID_NDK/platforms/android-9/arch-arm
+ $ export PREBUILT=$ANDROID_NDK/toolchains/$ARCH_ABI
+ $ export LDFLAGS="--sysroot=$SYSROOT"
+ $ export LD="$ANDROID_NDK/toolchains/$ARCH_ABI/prebuilt/$HOSTOSN/arm-linux-androideabi/bin/ld $LDFLAGS"
+ $ export LIBS="-llog $ANDROID_NDK/sources/cxx-stl/gnu-libstdc++/4.9/libs/armeabi-v7a/libgnustl_static.a"
+ $ export CPPFLAGS=""
+ $ export INCLUDES="-I$ANDROID_NDK/sources/cxx-stl/gnu-libstdc++/4.9/include/ -I$ANDROID_NDK/platforms/android-9/arch-arm/usr/include/ -I$ANDROID_NDK/sources/cxx-stl/gnu-libstdc++/4.9/libs/armeabi-v7a/include/"
+ $ export CXXFLAGS="-march=armv7-a -mfloat-abi=softfp -DGOOGLE_PROTOBUF_NO_RTTI --sysroot=$SYSROOT"
+ $ export CCFLAGS="$CXXFLAGS"
+ $ export CXX="$PREBUILT/prebuilt/$HOSTOSN/bin/arm-linux-androideabi-g++ $CXXFLAGS"
+ $ export CC="$CXX"
+ $ export RANLIB="$ANDROID_NDK/toolchains/$ARCH_ABI/prebuilt/$HOSTOSN/bin/arm-linux-androideabi-ranlib"
+ $ ./autogen.sh
+ $ ./configure --host=arm-linux-androideabi --with-sysroot=$SYSROOT --enable-cross-compile --with-protoc=protoc --disable-shared CXX="$CXX" CC="$CC" LD="$LD"
+ $ make
+ ```
+
+ 编译生成 *.a 静态库,若希望编译*.so 动态链接库 ,请在./configure参数中改--disable-shared为--disable-static --enable-shared。
+ 生成文件在src/.libs/下,将生成的文件拷贝至Anakin/third-party/arm-android/protobuf/lib下。
+ 在[cmake](../../cmake/find_modules.cmake)中更新`ARM_RPOTO_ROOT`的路径。
+ ```cmake
+ set(ARM_RPOTO_ROOT "${CMAKE_SOURCE_DIR}/third-party/arm-android/protobuf")
+ ```
+
+- 2.2 opencv 2.4.3+(optional)
+ Anakin只在examples示例中使用opencv
+ Android系统的opencv从[这里下载](https://opencv.org/releases.html)
+ 解压后将 `3rdparty/libs/armeabi-v7a`中的库文件拷贝到`libs/armeabi-v7a`
+ 在[cmake](../../cmake/find_modules.cmake)中搜索`anakin_find_opencv`,
+ 并设置 `include_directories` 和 `LINK_DIRECTORIES`为自己安装的库的路径。
+ ```cmake
+ include_directories(${CMAKE_SOURCE_DIR}/third-party/arm-android/opencv/sdk/native/jni/include/)
+ LINK_DIRECTORIES(${CMAKE_SOURCE_DIR}/third-party/arm-android/opencv/sdk/native/libs/armeabi-v7a/)
+ ```
+### 3. Anakin源码编译 ###
+
+#### 编译Android版本
+
+ 克隆[源码](https://github.com/PaddlePaddle/Anakin/tree/arm)
+```bash
+ cd your_dir
+ git clone https://github.com/PaddlePaddle/Anakin.git
+ cd Anakin
+ git fetch origin arm
+ git checkout arm
+ ```
+ 修改`android_build.sh`
+- 修改NDK路径
+ ```bash
+ #modify "your_ndk_path" to your NDK path
+ export ANDROID_NDK=your_ndk_path
+ ```
+- 修改ARM 处理器架构
+ 对于32位ARM处理器, 将ANDROID_ABI 设置为 `armeabi-v7a with NEON`,
+ 对于64位ARM处理器, 可以将ANDROID_ABI 设置为 `armeabi-v7a with NEON`或者`arm64-v8a`。
+ 目前我们只支持 `armeabi-v7a with NEON`;`arm64-v8a` 还在开发中。
+ ```bash
+ -DANDROID_ABI="armeabi-v7a with NEON"
+ ```
+- 设置Android API
+ 根据Android系统的版本设置API level, 例如API Level 21 -> Android 5.0.1
+ ```bash
+ -DANDROID_NATIVE_API_LEVEL=21
+ ```
+
+- 选择编译静态库或动态库
+ 设置`BUILD_SHARED=NO`编译静态库
+ 设置`BUILD_SHARED=YES`编译动态库
+ ```bash
+ -DBUILD_SHARED=NO
+ ```
+- OpenMP多线程支持
+ 设置`USE_OPENMP=YES`开启OpenMP多线程
+ ```bash
+ -DUSE_OPENMP=YES
+ ```
+
+- 编译单测文件
+ 设置`BUILD_WITH_UNIT_TEST=YES`将会编译单测文件
+ ```bash
+ -DBUILD_WITH_UNIT_TEST=YES
+ ```
+
+- 编译示例文件
+ 设置`BUILD_EXAMPLES=YES`将会编译示例文件
+ ```bash
+ -DBUILD_EXAMPLES=YES
+ ```
+
+- 开启opencv
+ 如果使用opencv,设置`USE_OPENCV=YES`
+ ```bash
+ -DUSE_OPENCV=YES
+ ```
+
+- 开始编译
+ 运行脚本 `android_build.sh` 将自动编译Anakin
+ ```bash
+ ./android_build.sh
+ ```
+
+### 4. 验证安装 ###
+ 编译好的库会放在目录`${Anakin_root}/output`下;
+ 编译好的单测文件会放在`${Anakin_root}/output/unit_test`目录下;
+ 编译好的示例文件会放在`${Anakin_root}/output/examples`目录下。
+
+ 对于Android系统,打开设备的调试模式,通过ADB可以访问的目录是`data/local/tmp`,通过ADB push将测试文件、模型和数据发送到设备目录, 运行测试文件。
diff --git a/doc/fluid/new_docs/advanced_usage/development/contribute_to_paddle.md b/doc/fluid/new_docs/advanced_usage/development/contribute_to_paddle.md
new file mode 120000
index 0000000000000000000000000000000000000000..1126df7a829ab6d98e58a44e8f9c6459feae9a8b
--- /dev/null
+++ b/doc/fluid/new_docs/advanced_usage/development/contribute_to_paddle.md
@@ -0,0 +1 @@
+../../../dev/contribute_to_paddle_cn.md
\ No newline at end of file
diff --git a/doc/fluid/new_docs/advanced_usage/development/cpu_profiling_cn.md b/doc/fluid/new_docs/advanced_usage/development/cpu_profiling_cn.md
new file mode 120000
index 0000000000000000000000000000000000000000..1381a3b05f6761c60742eb9365708d94ad8a2642
--- /dev/null
+++ b/doc/fluid/new_docs/advanced_usage/development/cpu_profiling_cn.md
@@ -0,0 +1 @@
+../../../howto/optimization/cpu_profiling_cn.md
\ No newline at end of file
diff --git a/doc/fluid/new_docs/advanced_usage/development/gpu_profiling_cn.rst b/doc/fluid/new_docs/advanced_usage/development/gpu_profiling_cn.rst
new file mode 100644
index 0000000000000000000000000000000000000000..f2396716bddd4810fa77c738d41f5482aa6d6055
--- /dev/null
+++ b/doc/fluid/new_docs/advanced_usage/development/gpu_profiling_cn.rst
@@ -0,0 +1,242 @@
+============
+GPU性能调优
+============
+
+.. contents::
+
+此教程将向您分步介绍如何使用内置的定时工具、 **nvprof** 或 **nvvp** 来运行性能分析和调优。
+
+- 什么是性能分析?
+- 为什么需要性能分析?
+- 如何进行性能分析?
+- 性能分析工具介绍
+- 详细教程
+- 性能分析小技巧
+
+什么是性能分析?
+================
+在软件工程的范畴里,性能分析(Profiling)是一个动态程序分析的术语,它可以指测量一个程序的空间(内存)复杂度或时间复杂度,
+也可以说是某些特定指令的使用情况,或者是函数调用的频率和耗时等。通常情况下,分析得到的信息用于协助进行程序的优化。
+
+简单来说,性能分析工具是用于给应用程序的性能做定量分析的。如果想很好的理解程序的行为,那程序分析工具是必不可少的利器。简单的性能分析,可以告诉您某个操作到底花了多长时间?而更深入的分析,甚至能解释为什么某个操作花了很长时间?
+
+为什么需要性能分析?
+============================
+训练好一个深层神经网络通常要耗费非常长的时间,所以性能也就逐步变成了深度学习领域最重要的指标。
+而优化性能的首要任务,是需要了解哪些步骤拖慢了整体。
+如果某一块根本就不怎么耗时,那也就不需要急着优化性能啦!
+
+如何进行性能分析?
+========================
+为了达到性能最优,您可以采用下面五个步骤:
+
+- 对代码进行性能分析
+- 找到运行慢的部分
+- 找到运行慢的原因
+- 修改成更快的版本
+- 再次对代码进行性能分析
+
+Usually, processor has two key performance limits include float point throughput and
+memory throughput. For GPU, it also need more parallelism to fulfill its potential.
+This is why they can be so fast.
+
+通常情况下,处理器有两个关键性能限制:一个是浮点计算量,另一个是内存操作量。
+GPU则还需要高并行性,才能发挥其全部能力。这正是它们速度快的原因。
+
+性能分析工具介绍
+======================
+就通常的GPU性能分析来说,市面上已经有NVIDIA或第三方提供的众多工具。
+
+**nvprof** 是Nvidia性能分析工具, **nvvp** 则是带GUI的Nvidia可视化性能分析工具。
+在这个教程中,我们主要会介绍nvprof和nvvp。
+
+:code:`test_GpuProfiler` from :code:`paddle/legacy/math/tests` directory will be used to evaluate
+above profilers.
+
+:code:`paddle/legacy/math/test` 目录中的 :code:`test_GpuProfiler` 就是用于展示上述分析工具的用法。
+
+.. literalinclude:: ../../../../paddle/legacy/math/tests/test_GpuProfiler.cpp
+ :language: c++
+ :lines: 137-151
+ :linenos:
+
+上述的代码片段包含了两种方法,您可以任意使用一个或两个来对感兴趣的代码段做性能分析。
+
+1. :code:`REGISTER_TIMER_INFO` 是一个内置的定时器封装,可以用来计算CPU函数或cuda内核的时间消耗。
+
+2. :code:`REGISTER_GPU_PROFILER` is a general purpose wrapper object of :code:`cudaProfilerStart` and :code:`cudaProfilerStop` to avoid
+program crashes when CPU version of PaddlePaddle invokes them.
+
+3. :code:`REGISTER_GPU_PROFILER` 是一个封装对象,封装了 :code:`cudaProfilerStart` 和 :code:`cudaProfileStop` 两个操作;同时其内部实现可以避免纯CPU版本PaddlePaddle在执行本语句时发生崩溃。
+
+您会在接下来的部分中获得更多的细节介绍。
+
+详细教程
+============
+
+内置定时器
+------------
+
+如果想要启用PaddlePaddle的内置定时器,您首先需要在相关代码段中加入 :code:`REGISTER_TIMER_INFO`。
+接下来就可以使用 :code:`printStatus` 或者 :code:`printAllStatus` 函数来将信息输出到界面中。
+下面举个简单的例子:
+
+1. 加入 :code:`REGISTER_TIMER_INFO` 和 :code:`printAllStatus` 函数(如高亮部分)。
+
+ .. literalinclude:: ../../../../paddle/legacy/math/tests/test_GpuProfiler.cpp
+ :language: c++
+ :lines: 137-151
+ :emphasize-lines: 8-12,14
+ :linenos:
+
+2. cmake配置中将 **WITH_TIMER** 打开,重新编译PaddlePaddle。
+
+ .. code-block:: bash
+
+ cmake .. -DWITH_TIMER=ON
+ make
+
+3. 执行您的代码,并观察结果(如高亮部分)。
+
+ .. code-block:: bash
+ :emphasize-lines: 1,12-15
+
+ > ./paddle/legacy/math/tests/test_GpuProfiler
+ I1117 11:13:42.313065 2522362816 Util.cpp:155] commandline: ./paddle/legacy/math/tests/test_GpuProfiler
+ I1117 11:13:42.845065 2522362816 Util.cpp:130] Calling runInitFunctions
+ I1117 11:13:42.845208 2522362816 Util.cpp:143] Call runInitFunctions done.
+ [==========] Running 1 test from 1 test case.
+ [----------] Global test environment set-up.
+ [----------] 1 test from Profiler
+ [ RUN ] Profiler.BilinearFwdBwd
+ I1117 11:13:42.845310 2522362816 test_GpuProfiler.cpp:114] Enable GPU Profiler Stat: [testBilinearFwdBwd] "numSamples = 10, channels = 16, im
+ gSizeX = 64, imgSizeY = 64"
+ I1117 11:13:42.850154 2522362816 ThreadLocal.cpp:37] thread use undeterministic rand seed:20659751
+ I1117 11:13:42.981501 2522362816 Stat.cpp:130] ======= StatSet: [GlobalStatInfo] status ======
+ I1117 11:13:42.981539 2522362816 Stat.cpp:133] Stat=testBilinearFwdBwd total=136.141 avg=136.141 max=136.141 min=136.141 count=1
+ I1117 11:13:42.981572 2522362816 Stat.cpp:141] ======= BarrierStatSet status ======
+ I1117 11:13:42.981575 2522362816 Stat.cpp:154] --------------------------------------------------
+ [ OK ] Profiler.BilinearFwdBwd (136 ms)
+ [----------] 1 test from Profiler (136 ms total)
+
+ [----------] Global test environment tear-down
+ [==========] 1 test from 1 test case ran. (136 ms total)
+ [ PASSED ] 1 test.
+
+nvprof 工具
+----------------
+
+要使用命令行分析工具 **nvprof**,您按如下步骤操作即可:
+
+1. 将 :code:`REGISTER_GPU_PROFILER` 函数加到代码中(参考强调部分)。
+
+ .. literalinclude:: ../../../../paddle/legacy/math/tests/test_GpuProfiler.cpp
+ :language: c++
+ :lines: 137-151
+ :emphasize-lines: 6-7
+ :linenos:
+
+2. cmake中将 **WITH_PROFILER** 配置打开,重新编译PaddlePaddle。
+
+ .. code-block:: bash
+
+ cmake .. -DWITH_PROFILER=ON
+ make
+
+3. 使用 **nvprof** 来分析执行文件。
+
+ .. code-block:: bash
+
+ nvprof ./paddle/legacy/math/tests/test_GpuProfiler
+
+然后,您就能获得如下的分析结果:
+
+.. code-block:: bash
+
+ ==78544== Profiling application: ./paddle/legacy/math/tests/test_GpuProfiler
+ ==78544== Profiling result:
+ Time(%) Time Calls Avg Min Max Name
+ 27.60% 9.6305ms 5 1.9261ms 3.4560us 6.4035ms [CUDA memcpy HtoD]
+ 26.07% 9.0957ms 1 9.0957ms 9.0957ms 9.0957ms KeBilinearInterpBw
+ 23.78% 8.2977ms 1 8.2977ms 8.2977ms 8.2977ms KeBilinearInterpFw
+ 22.55% 7.8661ms 2 3.9330ms 1.5798ms 6.2863ms [CUDA memcpy DtoH]
+
+ ==78544== API calls:
+ Time(%) Time Calls Avg Min Max Name
+ 46.85% 682.28ms 8 85.285ms 12.639us 682.03ms cudaStreamCreateWithFlags
+ 39.83% 580.00ms 4 145.00ms 302ns 550.27ms cudaFree
+ 9.82% 143.03ms 9 15.892ms 8.7090us 142.78ms cudaStreamCreate
+ 1.23% 17.983ms 7 2.5690ms 23.210us 6.4563ms cudaMemcpy
+ 1.23% 17.849ms 2 8.9247ms 8.4726ms 9.3768ms cudaStreamSynchronize
+ 0.66% 9.5969ms 7 1.3710ms 288.43us 2.4279ms cudaHostAlloc
+ 0.13% 1.9530ms 11 177.54us 7.6810us 591.06us cudaMalloc
+ 0.07% 1.0424ms 8 130.30us 1.6970us 453.72us cudaGetDevice
+ 0.04% 527.90us 40 13.197us 525ns 253.99us cudaEventCreateWithFlags
+ 0.03% 435.73us 348 1.2520us 124ns 42.704us cuDeviceGetAttribute
+ 0.03% 419.36us 1 419.36us 419.36us 419.36us cudaGetDeviceCount
+ 0.02% 260.75us 2 130.38us 129.32us 131.43us cudaGetDeviceProperties
+ 0.02% 222.32us 2 111.16us 106.94us 115.39us cudaLaunch
+ 0.01% 214.06us 4 53.514us 28.586us 77.655us cuDeviceGetName
+ 0.01% 115.45us 4 28.861us 9.8250us 44.526us cuDeviceTotalMem
+ 0.01% 83.988us 4 20.997us 578ns 77.760us cudaSetDevice
+ 0.00% 38.918us 1 38.918us 38.918us 38.918us cudaEventCreate
+ 0.00% 34.573us 31 1.1150us 279ns 12.784us cudaDeviceGetAttribute
+ 0.00% 17.767us 1 17.767us 17.767us 17.767us cudaProfilerStart
+ 0.00% 15.228us 2 7.6140us 3.5460us 11.682us cudaConfigureCall
+ 0.00% 14.536us 2 7.2680us 1.1490us 13.387us cudaGetLastError
+ 0.00% 8.6080us 26 331ns 173ns 783ns cudaSetupArgument
+ 0.00% 5.5470us 6 924ns 215ns 2.6780us cuDeviceGet
+ 0.00% 5.4090us 6 901ns 328ns 3.3320us cuDeviceGetCount
+ 0.00% 4.1770us 3 1.3920us 1.0630us 1.8300us cuDriverGetVersion
+ 0.00% 3.4650us 3 1.1550us 1.0810us 1.2680us cuInit
+ 0.00% 830ns 1 830ns 830ns 830ns cudaRuntimeGetVersion
+
+
+nvvp 工具
+--------------
+
+如果想使用可视化的分析器 **nvvp**,您可以导入 :code:`nvprof -o ...` 的输出,或者从工具的界面里运行您的应用。
+
+**备注: nvvp 也支持CPU的性能分析** (需在nvvp界面中选上才能开启)
+
+.. image:: nvvp1.png
+ :align: center
+ :scale: 33%
+
+从内核函数的角度, **nvvp** 可以精确说明一个长耗时操作的具体原因。
+同时,如下图所示, **nvvp** 的内核block使用情况、寄存器使用情况和共享内存使用情况能让我们对GPU的整体使用有更好的理解。
+
+
+.. image:: nvvp2.png
+ :align: center
+ :scale: 33%
+
+而从应用的角度, **nvvp** 可以帮您提供一些定位性能瓶颈的建议。
+例如,下图中就展示了一些关于内存数据迁徙和计算资源利用率的建议,为您做性能调优提供了方向。
+
+.. image:: nvvp3.png
+ :align: center
+ :scale: 33%
+
+.. image:: nvvp4.png
+ :align: center
+ :scale: 33%
+
+性能分析小技巧
+==================
+
+- 开始阶段,从 **nvprof** 和 **nvvp** 的输出信息入手是个不错的选择。
+- 接下来可以考虑下时间线的分析。
+- 如果真想挖掘内核深处的某个秘密,您最好先确认:这一块的耗时比例真的太高,值得深入分析。
+- 可能的情况下,试着让输出的分析数据和理论值对应。
+
+ 1) 例如,如果我知道内核花了10ms来移动1GB数据,那我会期望分析工具统计到速度是100GB/s。
+ 2) 若有不一致之处,很有可能实际应用就是没有按照您的预期情况运行。
+- 了解您的硬件:如果您的GPU理论可以达到6 TFLOPs(6万亿次浮点运算每秒),而当前已经有5.5 TFLOPs了,那估计这里的潜力就没啥好挖的了……
+
+性能分析是性能优化的关键一步。有的时候简简单单的改变就能在性能上产生明显的优化效果!
+当然,具体情况因人而异。
+
+参考资料
+===========
+Jeremy Appleyard, `GPU Profiling for Deep Learning `_, 2015
diff --git a/doc/fluid/new_docs/advanced_usage/development/host_memory_profiling_cn.md b/doc/fluid/new_docs/advanced_usage/development/host_memory_profiling_cn.md
new file mode 120000
index 0000000000000000000000000000000000000000..904968ba4a8d6cc6489c91a0a751e0a33dcc873c
--- /dev/null
+++ b/doc/fluid/new_docs/advanced_usage/development/host_memory_profiling_cn.md
@@ -0,0 +1 @@
+../../../howto/optimization/host_memory_profiling_cn.md
\ No newline at end of file
diff --git a/doc/fluid/new_docs/advanced_usage/development/new_op.md b/doc/fluid/new_docs/advanced_usage/development/new_op.md
new file mode 120000
index 0000000000000000000000000000000000000000..dce0348585b8c484c1418a03a5fde5d78b0afcc9
--- /dev/null
+++ b/doc/fluid/new_docs/advanced_usage/development/new_op.md
@@ -0,0 +1 @@
+../../../dev/new_op_cn.md
\ No newline at end of file
diff --git a/doc/fluid/new_docs/advanced_usage/development/nvvp1.png b/doc/fluid/new_docs/advanced_usage/development/nvvp1.png
new file mode 100644
index 0000000000000000000000000000000000000000..1af23ac3c52929b2b0645d2f9fa4d4c6db1f6e77
Binary files /dev/null and b/doc/fluid/new_docs/advanced_usage/development/nvvp1.png differ
diff --git a/doc/fluid/new_docs/advanced_usage/development/nvvp2.png b/doc/fluid/new_docs/advanced_usage/development/nvvp2.png
new file mode 100644
index 0000000000000000000000000000000000000000..177c9db708da6863d1075f3e615f5962dbe18b29
Binary files /dev/null and b/doc/fluid/new_docs/advanced_usage/development/nvvp2.png differ
diff --git a/doc/fluid/new_docs/advanced_usage/development/nvvp3.png b/doc/fluid/new_docs/advanced_usage/development/nvvp3.png
new file mode 100644
index 0000000000000000000000000000000000000000..d8f393667d6569b6f1e61ffccac43fae5888b6db
Binary files /dev/null and b/doc/fluid/new_docs/advanced_usage/development/nvvp3.png differ
diff --git a/doc/fluid/new_docs/advanced_usage/development/nvvp4.png b/doc/fluid/new_docs/advanced_usage/development/nvvp4.png
new file mode 100644
index 0000000000000000000000000000000000000000..51f2f3e183295de6cf8ddaf2b3b8a0862aa35f01
Binary files /dev/null and b/doc/fluid/new_docs/advanced_usage/development/nvvp4.png differ
diff --git a/doc/fluid/new_docs/advanced_usage/development/pprof_1.png b/doc/fluid/new_docs/advanced_usage/development/pprof_1.png
new file mode 100644
index 0000000000000000000000000000000000000000..8e9edbf377672d0ef40f2fc7bd39e746923550cb
Binary files /dev/null and b/doc/fluid/new_docs/advanced_usage/development/pprof_1.png differ
diff --git a/doc/fluid/new_docs/advanced_usage/development/pprof_2.png b/doc/fluid/new_docs/advanced_usage/development/pprof_2.png
new file mode 100644
index 0000000000000000000000000000000000000000..172ba20399ba974d27f4c072425277b69b02520b
Binary files /dev/null and b/doc/fluid/new_docs/advanced_usage/development/pprof_2.png differ
diff --git a/doc/fluid/new_docs/advanced_usage/development/timeline.jpeg b/doc/fluid/new_docs/advanced_usage/development/timeline.jpeg
new file mode 100644
index 0000000000000000000000000000000000000000..38ec3f80c982857531f30a8bb0fa26ea5bf05385
Binary files /dev/null and b/doc/fluid/new_docs/advanced_usage/development/timeline.jpeg differ
diff --git a/doc/fluid/new_docs/advanced_usage/development/timeline_cn.md b/doc/fluid/new_docs/advanced_usage/development/timeline_cn.md
new file mode 120000
index 0000000000000000000000000000000000000000..a05540e82a7fa795dcd8e7306261ef9bef57426f
--- /dev/null
+++ b/doc/fluid/new_docs/advanced_usage/development/timeline_cn.md
@@ -0,0 +1 @@
+../../../howto/optimization/timeline_cn.md
\ No newline at end of file
diff --git a/doc/fluid/new_docs/advanced_usage/development/tracing.jpeg b/doc/fluid/new_docs/advanced_usage/development/tracing.jpeg
new file mode 100644
index 0000000000000000000000000000000000000000..3a49fc4f8a401a9463b0157e2f38c164ca02dcc5
Binary files /dev/null and b/doc/fluid/new_docs/advanced_usage/development/tracing.jpeg differ
diff --git a/doc/fluid/new_docs/advanced_usage/development/write_docs.rst b/doc/fluid/new_docs/advanced_usage/development/write_docs.rst
new file mode 120000
index 0000000000000000000000000000000000000000..dc536c8bdd4924758d4418bac8e4181ffbb1f780
--- /dev/null
+++ b/doc/fluid/new_docs/advanced_usage/development/write_docs.rst
@@ -0,0 +1 @@
+../../../dev/write_docs_cn.rst
\ No newline at end of file
diff --git a/doc/fluid/new_docs/advanced_usage/index.rst b/doc/fluid/new_docs/advanced_usage/index.rst
new file mode 100644
index 0000000000000000000000000000000000000000..dea7c236619a0bdbf402f371571d947d1cdbba65
--- /dev/null
+++ b/doc/fluid/new_docs/advanced_usage/index.rst
@@ -0,0 +1,23 @@
+########
+进阶使用
+########
+
+
+.. todo::
+
+ Complete this guide
+
+.. toctree::
+ :maxdepth: 2
+
+ deploy/index_native.rst
+ deploy/index_anakin.rst
+ deploy/index_mobile.rst
+ development/contribute_to_paddle.md
+ development/write_docs.rst
+ development/new_op.md
+ development/cpu_profiling_cn.md
+ development/gpu_profiling_cn.rst
+ development/host_memory_profiling_cn.md
+ development/timeline_cn.md
+ benchmark.rst
diff --git a/doc/fluid/new_docs/advanced_usage/pics/anakin_fm_ch.png b/doc/fluid/new_docs/advanced_usage/pics/anakin_fm_ch.png
new file mode 100644
index 0000000000000000000000000000000000000000..52d4992a22397119af949aa7c11a9ea6365c167c
Binary files /dev/null and b/doc/fluid/new_docs/advanced_usage/pics/anakin_fm_ch.png differ
diff --git a/doc/fluid/new_docs/beginners_guide/basics/image_classification/.gitignore b/doc/fluid/new_docs/beginners_guide/basics/image_classification/.gitignore
new file mode 100644
index 0000000000000000000000000000000000000000..dc7c62b06287ad333dd41082e566b0553d3a5341
--- /dev/null
+++ b/doc/fluid/new_docs/beginners_guide/basics/image_classification/.gitignore
@@ -0,0 +1,8 @@
+*.pyc
+train.log
+output
+data/cifar-10-batches-py/
+data/cifar-10-python.tar.gz
+data/*.txt
+data/*.list
+data/mean.meta
diff --git a/doc/fluid/new_docs/beginners_guide/basics/image_classification/image/dog.png b/doc/fluid/new_docs/beginners_guide/basics/image_classification/image/dog.png
new file mode 100644
index 0000000000000000000000000000000000000000..ca8f858a902ea723d886d2b88c2c0a1005301c50
Binary files /dev/null and b/doc/fluid/new_docs/beginners_guide/basics/image_classification/image/dog.png differ
diff --git a/doc/fluid/new_docs/beginners_guide/basics/image_classification/image/dog_cat.png b/doc/fluid/new_docs/beginners_guide/basics/image_classification/image/dog_cat.png
new file mode 100644
index 0000000000000000000000000000000000000000..38b21f21604b1bb84fc3f6aa96bd5fce45d15a55
Binary files /dev/null and b/doc/fluid/new_docs/beginners_guide/basics/image_classification/image/dog_cat.png differ
diff --git a/doc/fluid/new_docs/beginners_guide/basics/image_classification/image/fea_conv0.png b/doc/fluid/new_docs/beginners_guide/basics/image_classification/image/fea_conv0.png
new file mode 100644
index 0000000000000000000000000000000000000000..647c822e52cd55d50e5f207978f5e6ada86cf34c
Binary files /dev/null and b/doc/fluid/new_docs/beginners_guide/basics/image_classification/image/fea_conv0.png differ
diff --git a/doc/fluid/new_docs/beginners_guide/basics/image_classification/image/flowers.png b/doc/fluid/new_docs/beginners_guide/basics/image_classification/image/flowers.png
new file mode 100644
index 0000000000000000000000000000000000000000..04245cef60fe7126ae4c92ba8085273965078bee
Binary files /dev/null and b/doc/fluid/new_docs/beginners_guide/basics/image_classification/image/flowers.png differ
diff --git a/doc/fluid/new_docs/beginners_guide/basics/image_classification/image/googlenet.jpeg b/doc/fluid/new_docs/beginners_guide/basics/image_classification/image/googlenet.jpeg
new file mode 100644
index 0000000000000000000000000000000000000000..249dbf96df61c3352ea5bd80470f6c4a1e03ff10
Binary files /dev/null and b/doc/fluid/new_docs/beginners_guide/basics/image_classification/image/googlenet.jpeg differ
diff --git a/doc/fluid/new_docs/beginners_guide/basics/image_classification/image/ilsvrc.png b/doc/fluid/new_docs/beginners_guide/basics/image_classification/image/ilsvrc.png
new file mode 100644
index 0000000000000000000000000000000000000000..4660ac122e9d533023a21154d35eee29e3b08d27
Binary files /dev/null and b/doc/fluid/new_docs/beginners_guide/basics/image_classification/image/ilsvrc.png differ
diff --git a/doc/fluid/new_docs/beginners_guide/basics/image_classification/image/inception.png b/doc/fluid/new_docs/beginners_guide/basics/image_classification/image/inception.png
new file mode 100644
index 0000000000000000000000000000000000000000..9591a0c1e8c0165c40ca560be35a7b9a91cd5027
Binary files /dev/null and b/doc/fluid/new_docs/beginners_guide/basics/image_classification/image/inception.png differ
diff --git a/doc/fluid/new_docs/beginners_guide/basics/image_classification/image/lenet.png b/doc/fluid/new_docs/beginners_guide/basics/image_classification/image/lenet.png
new file mode 100644
index 0000000000000000000000000000000000000000..77f785e03bacd38c4c64a817874a58ff3298d2f3
Binary files /dev/null and b/doc/fluid/new_docs/beginners_guide/basics/image_classification/image/lenet.png differ
diff --git a/doc/fluid/new_docs/beginners_guide/basics/image_classification/image/plot.png b/doc/fluid/new_docs/beginners_guide/basics/image_classification/image/plot.png
new file mode 100644
index 0000000000000000000000000000000000000000..57e45cc0c27dd99b9918de2ff1228bc6b65f7424
Binary files /dev/null and b/doc/fluid/new_docs/beginners_guide/basics/image_classification/image/plot.png differ
diff --git a/doc/fluid/new_docs/beginners_guide/basics/image_classification/image/resnet.png b/doc/fluid/new_docs/beginners_guide/basics/image_classification/image/resnet.png
new file mode 100644
index 0000000000000000000000000000000000000000..0aeb4f254639fdbf18e916dc219ca61602596d85
Binary files /dev/null and b/doc/fluid/new_docs/beginners_guide/basics/image_classification/image/resnet.png differ
diff --git a/doc/fluid/new_docs/beginners_guide/basics/image_classification/image/resnet_block.jpg b/doc/fluid/new_docs/beginners_guide/basics/image_classification/image/resnet_block.jpg
new file mode 100644
index 0000000000000000000000000000000000000000..c500eb01a90190ff66150871fe83ec275e2de8d7
Binary files /dev/null and b/doc/fluid/new_docs/beginners_guide/basics/image_classification/image/resnet_block.jpg differ
diff --git a/doc/fluid/new_docs/beginners_guide/basics/image_classification/image/train_and_test.png b/doc/fluid/new_docs/beginners_guide/basics/image_classification/image/train_and_test.png
new file mode 100644
index 0000000000000000000000000000000000000000..c6336a9a69b95dc978719ce68896e3e752e67fed
Binary files /dev/null and b/doc/fluid/new_docs/beginners_guide/basics/image_classification/image/train_and_test.png differ
diff --git a/doc/fluid/new_docs/beginners_guide/basics/image_classification/image/vgg16.png b/doc/fluid/new_docs/beginners_guide/basics/image_classification/image/vgg16.png
new file mode 100644
index 0000000000000000000000000000000000000000..6270eefcfd7071bc1643ee06567e5b81aaf4c177
Binary files /dev/null and b/doc/fluid/new_docs/beginners_guide/basics/image_classification/image/vgg16.png differ
diff --git a/doc/fluid/new_docs/beginners_guide/basics/image_classification/index.md b/doc/fluid/new_docs/beginners_guide/basics/image_classification/index.md
new file mode 100644
index 0000000000000000000000000000000000000000..ce0d2bb1dc0cf73151ee9aceea7e4d7b24af1926
--- /dev/null
+++ b/doc/fluid/new_docs/beginners_guide/basics/image_classification/index.md
@@ -0,0 +1,559 @@
+
+# 图像分类
+
+本教程源代码目录在[book/image_classification](https://github.com/PaddlePaddle/book/tree/develop/03.image_classification), 初次使用请参考PaddlePaddle[安装教程](https://github.com/PaddlePaddle/book/blob/develop/README.cn.md#运行这本书)。
+
+## 背景介绍
+
+图像相比文字能够提供更加生动、容易理解及更具艺术感的信息,是人们转递与交换信息的重要来源。在本教程中,我们专注于图像识别领域的一个重要问题,即图像分类。
+
+图像分类是根据图像的语义信息将不同类别图像区分开来,是计算机视觉中重要的基本问题,也是图像检测、图像分割、物体跟踪、行为分析等其他高层视觉任务的基础。图像分类在很多领域有广泛应用,包括安防领域的人脸识别和智能视频分析等,交通领域的交通场景识别,互联网领域基于内容的图像检索和相册自动归类,医学领域的图像识别等。
+
+
+一般来说,图像分类通过手工特征或特征学习方法对整个图像进行全部描述,然后使用分类器判别物体类别,因此如何提取图像的特征至关重要。在深度学习算法之前使用较多的是基于词袋(Bag of Words)模型的物体分类方法。词袋方法从自然语言处理中引入,即一句话可以用一个装了词的袋子表示其特征,袋子中的词为句子中的单词、短语或字。对于图像而言,词袋方法需要构建字典。最简单的词袋模型框架可以设计为**底层特征抽取**、**特征编码**、**分类器设计**三个过程。
+
+而基于深度学习的图像分类方法,可以通过有监督或无监督的方式**学习**层次化的特征描述,从而取代了手工设计或选择图像特征的工作。深度学习模型中的卷积神经网络(Convolution Neural Network, CNN)近年来在图像领域取得了惊人的成绩,CNN直接利用图像像素信息作为输入,最大程度上保留了输入图像的所有信息,通过卷积操作进行特征的提取和高层抽象,模型输出直接是图像识别的结果。这种基于"输入-输出"直接端到端的学习方法取得了非常好的效果,得到了广泛的应用。
+
+本教程主要介绍图像分类的深度学习模型,以及如何使用PaddlePaddle训练CNN模型。
+
+## 效果展示
+
+图像分类包括通用图像分类、细粒度图像分类等。图1展示了通用图像分类效果,即模型可以正确识别图像上的主要物体。
+
+
+
+图1. 通用图像分类展示
+
+
+
+图2展示了细粒度图像分类-花卉识别的效果,要求模型可以正确识别花的类别。
+
+
+
+图2. 细粒度图像分类展示
+
+
+
+一个好的模型既要对不同类别识别正确,同时也应该能够对不同视角、光照、背景、变形或部分遮挡的图像正确识别(这里我们统一称作图像扰动)。图3展示了一些图像的扰动,较好的模型会像聪明的人类一样能够正确识别。
+
+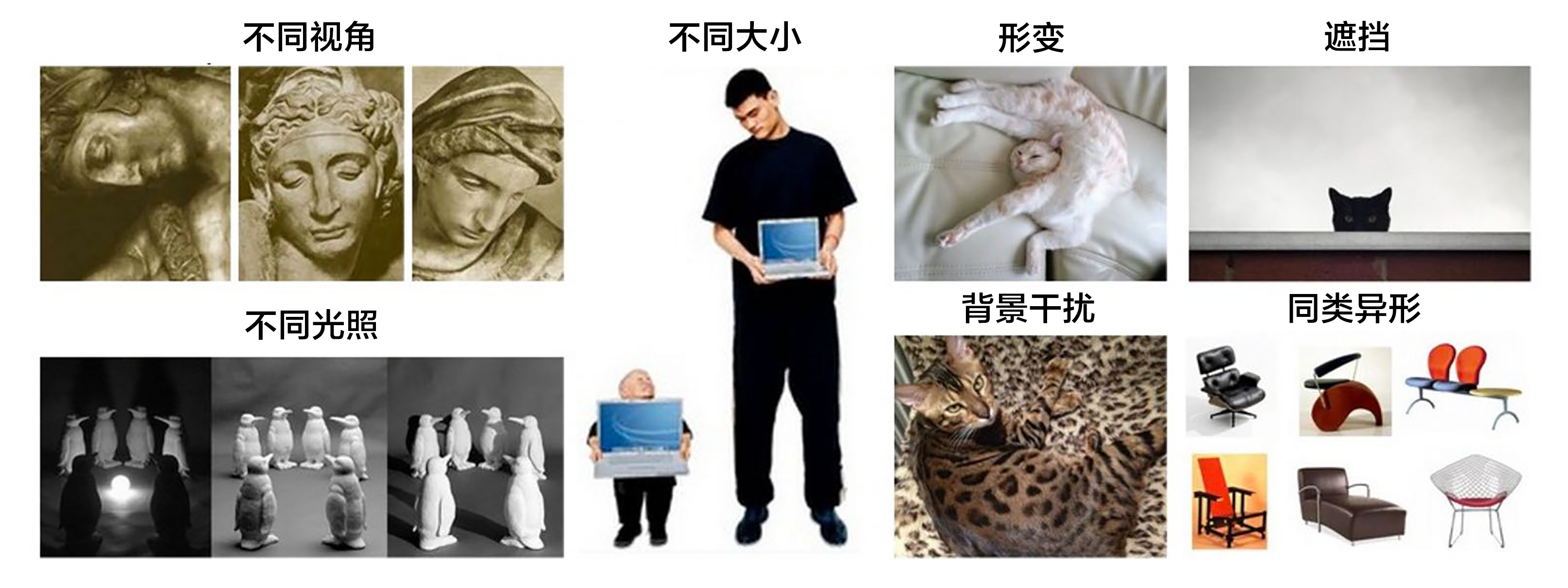
+
+图3. 扰动图片展示[22]
+
+
+## 模型概览
+
+图像识别领域大量的研究成果都是建立在[PASCAL VOC](http://host.robots.ox.ac.uk/pascal/VOC/)、[ImageNet](http://image-net.org/)等公开的数据集上,很多图像识别算法通常在这些数据集上进行测试和比较。PASCAL VOC是2005年发起的一个视觉挑战赛,ImageNet是2010年发起的大规模视觉识别竞赛(ILSVRC)的数据集,在本章中我们基于这些竞赛的一些论文介绍图像分类模型。
+
+在2012年之前的传统图像分类方法可以用背景描述中提到的三步完成,但通常完整建立图像识别模型一般包括底层特征学习、特征编码、空间约束、分类器设计、模型融合等几个阶段。
+1). **底层特征提取**: 通常从图像中按照固定步长、尺度提取大量局部特征描述。常用的局部特征包括SIFT(Scale-Invariant Feature Transform, 尺度不变特征转换) \[[1](#参考文献)\]、HOG(Histogram of Oriented Gradient, 方向梯度直方图) \[[2](#参考文献)\]、LBP(Local Bianray Pattern, 局部二值模式) \[[3](#参考文献)\] 等,一般也采用多种特征描述子,防止丢失过多的有用信息。
+2). **特征编码**: 底层特征中包含了大量冗余与噪声,为了提高特征表达的鲁棒性,需要使用一种特征变换算法对底层特征进行编码,称作特征编码。常用的特征编码包括向量量化编码 \[[4](#参考文献)\]、稀疏编码 \[[5](#参考文献)\]、局部线性约束编码 \[[6](#参考文献)\]、Fisher向量编码 \[[7](#参考文献)\] 等。
+3). **空间特征约束**: 特征编码之后一般会经过空间特征约束,也称作**特征汇聚**。特征汇聚是指在一个空间范围内,对每一维特征取最大值或者平均值,可以获得一定特征不变形的特征表达。金字塔特征匹配是一种常用的特征聚会方法,这种方法提出将图像均匀分块,在分块内做特征汇聚。
+4). **通过分类器分类**: 经过前面步骤之后一张图像可以用一个固定维度的向量进行描述,接下来就是经过分类器对图像进行分类。通常使用的分类器包括SVM(Support Vector Machine, 支持向量机)、随机森林等。而使用核方法的SVM是最为广泛的分类器,在传统图像分类任务上性能很好。
+
+这种方法在PASCAL VOC竞赛中的图像分类算法中被广泛使用 \[[18](#参考文献)\]。[NEC实验室](http://www.nec-labs.com/)在ILSVRC2010中采用SIFT和LBP特征,两个非线性编码器以及SVM分类器获得图像分类的冠军 \[[8](#参考文献)\]。
+
+Alex Krizhevsky在2012年ILSVRC提出的CNN模型 \[[9](#参考文献)\] 取得了历史性的突破,效果大幅度超越传统方法,获得了ILSVRC2012冠军,该模型被称作AlexNet。这也是首次将深度学习用于大规模图像分类中。从AlexNet之后,涌现了一系列CNN模型,不断地在ImageNet上刷新成绩,如图4展示。随着模型变得越来越深以及精妙的结构设计,Top-5的错误率也越来越低,降到了3.5%附近。而在同样的ImageNet数据集上,人眼的辨识错误率大概在5.1%,也就是目前的深度学习模型的识别能力已经超过了人眼。
+
+
+
+图4. ILSVRC图像分类Top-5错误率
+
+
+### CNN
+
+传统CNN包含卷积层、全连接层等组件,并采用softmax多类别分类器和多类交叉熵损失函数,一个典型的卷积神经网络如图5所示,我们先介绍用来构造CNN的常见组件。
+
+
+
+图5. CNN网络示例[20]
+
+
+- 卷积层(convolution layer): 执行卷积操作提取底层到高层的特征,发掘出图片局部关联性质和空间不变性质。
+- 池化层(pooling layer): 执行降采样操作。通过取卷积输出特征图中局部区块的最大值(max-pooling)或者均值(avg-pooling)。降采样也是图像处理中常见的一种操作,可以过滤掉一些不重要的高频信息。
+- 全连接层(fully-connected layer,或者fc layer): 输入层到隐藏层的神经元是全部连接的。
+- 非线性变化: 卷积层、全连接层后面一般都会接非线性变化层,例如Sigmoid、Tanh、ReLu等来增强网络的表达能力,在CNN里最常使用的为ReLu激活函数。
+- Dropout \[[10](#参考文献)\] : 在模型训练阶段随机让一些隐层节点权重不工作,提高网络的泛化能力,一定程度上防止过拟合。
+
+另外,在训练过程中由于每层参数不断更新,会导致下一次输入分布发生变化,这样导致训练过程需要精心设计超参数。如2015年Sergey Ioffe和Christian Szegedy提出了Batch Normalization (BN)算法 \[[14](#参考文献)\] 中,每个batch对网络中的每一层特征都做归一化,使得每层分布相对稳定。BN算法不仅起到一定的正则作用,而且弱化了一些超参数的设计。经过实验证明,BN算法加速了模型收敛过程,在后来较深的模型中被广泛使用。
+
+接下来我们主要介绍VGG,GoogleNet和ResNet网络结构。
+
+### VGG
+
+牛津大学VGG(Visual Geometry Group)组在2014年ILSVRC提出的模型被称作VGG模型 \[[11](#参考文献)\] 。该模型相比以往模型进一步加宽和加深了网络结构,它的核心是五组卷积操作,每两组之间做Max-Pooling空间降维。同一组内采用多次连续的3X3卷积,卷积核的数目由较浅组的64增多到最深组的512,同一组内的卷积核数目是一样的。卷积之后接两层全连接层,之后是分类层。由于每组内卷积层的不同,有11、13、16、19层这几种模型,下图展示一个16层的网络结构。VGG模型结构相对简洁,提出之后也有很多文章基于此模型进行研究,如在ImageNet上首次公开超过人眼识别的模型\[[19](#参考文献)\]就是借鉴VGG模型的结构。
+
+
+
+图6. 基于ImageNet的VGG16模型
+
+
+### GoogleNet
+
+GoogleNet \[[12](#参考文献)\] 在2014年ILSVRC的获得了冠军,在介绍该模型之前我们先来了解NIN(Network in Network)模型 \[[13](#参考文献)\] 和Inception模块,因为GoogleNet模型由多组Inception模块组成,模型设计借鉴了NIN的一些思想。
+
+NIN模型主要有两个特点:1) 引入了多层感知卷积网络(Multi-Layer Perceptron Convolution, MLPconv)代替一层线性卷积网络。MLPconv是一个微小的多层卷积网络,即在线性卷积后面增加若干层1x1的卷积,这样可以提取出高度非线性特征。2) 传统的CNN最后几层一般都是全连接层,参数较多。而NIN模型设计最后一层卷积层包含类别维度大小的特征图,然后采用全局均值池化(Avg-Pooling)替代全连接层,得到类别维度大小的向量,再进行分类。这种替代全连接层的方式有利于减少参数。
+
+Inception模块如下图7所示,图(a)是最简单的设计,输出是3个卷积层和一个池化层的特征拼接。这种设计的缺点是池化层不会改变特征通道数,拼接后会导致特征的通道数较大,经过几层这样的模块堆积后,通道数会越来越大,导致参数和计算量也随之增大。为了改善这个缺点,图(b)引入3个1x1卷积层进行降维,所谓的降维就是减少通道数,同时如NIN模型中提到的1x1卷积也可以修正线性特征。
+
+
+
+图7. Inception模块
+
+
+GoogleNet由多组Inception模块堆积而成。另外,在网络最后也没有采用传统的多层全连接层,而是像NIN网络一样采用了均值池化层;但与NIN不同的是,池化层后面接了一层到类别数映射的全连接层。除了这两个特点之外,由于网络中间层特征也很有判别性,GoogleNet在中间层添加了两个辅助分类器,在后向传播中增强梯度并且增强正则化,而整个网络的损失函数是这个三个分类器的损失加权求和。
+
+GoogleNet整体网络结构如图8所示,总共22层网络:开始由3层普通的卷积组成;接下来由三组子网络组成,第一组子网络包含2个Inception模块,第二组包含5个Inception模块,第三组包含2个Inception模块;然后接均值池化层、全连接层。
+
+
+
+图8. GoogleNet[12]
+
+
+
+上面介绍的是GoogleNet第一版模型(称作GoogleNet-v1)。GoogleNet-v2 \[[14](#参考文献)\] 引入BN层;GoogleNet-v3 \[[16](#参考文献)\] 对一些卷积层做了分解,进一步提高网络非线性能力和加深网络;GoogleNet-v4 \[[17](#参考文献)\] 引入下面要讲的ResNet设计思路。从v1到v4每一版的改进都会带来准确度的提升,介于篇幅,这里不再详细介绍v2到v4的结构。
+
+
+### ResNet
+
+ResNet(Residual Network) \[[15](#参考文献)\] 是2015年ImageNet图像分类、图像物体定位和图像物体检测比赛的冠军。针对训练卷积神经网络时加深网络导致准确度下降的问题,ResNet提出了采用残差学习。在已有设计思路(BN, 小卷积核,全卷积网络)的基础上,引入了残差模块。每个残差模块包含两条路径,其中一条路径是输入特征的直连通路,另一条路径对该特征做两到三次卷积操作得到该特征的残差,最后再将两条路径上的特征相加。
+
+残差模块如图9所示,左边是基本模块连接方式,由两个输出通道数相同的3x3卷积组成。右边是瓶颈模块(Bottleneck)连接方式,之所以称为瓶颈,是因为上面的1x1卷积用来降维(图示例即256->64),下面的1x1卷积用来升维(图示例即64->256),这样中间3x3卷积的输入和输出通道数都较小(图示例即64->64)。
+
+
+
+图9. 残差模块
+
+
+图10展示了50、101、152层网络连接示意图,使用的是瓶颈模块。这三个模型的区别在于每组中残差模块的重复次数不同(见图右上角)。ResNet训练收敛较快,成功的训练了上百乃至近千层的卷积神经网络。
+
+
+
+图10. 基于ImageNet的ResNet模型
+
+
+
+## 数据准备
+
+通用图像分类公开的标准数据集常用的有[CIFAR](https://www.cs.toronto.edu/~kriz/cifar.html)、[ImageNet](http://image-net.org/)、[COCO](http://mscoco.org/)等,常用的细粒度图像分类数据集包括[CUB-200-2011](http://www.vision.caltech.edu/visipedia/CUB-200-2011.html)、[Stanford Dog](http://vision.stanford.edu/aditya86/ImageNetDogs/)、[Oxford-flowers](http://www.robots.ox.ac.uk/~vgg/data/flowers/)等。其中ImageNet数据集规模相对较大,如[模型概览](#模型概览)一章所讲,大量研究成果基于ImageNet。ImageNet数据从2010年来稍有变化,常用的是ImageNet-2012数据集,该数据集包含1000个类别:训练集包含1,281,167张图片,每个类别数据732至1300张不等,验证集包含50,000张图片,平均每个类别50张图片。
+
+由于ImageNet数据集较大,下载和训练较慢,为了方便大家学习,我们使用[CIFAR10]()数据集。CIFAR10数据集包含60,000张32x32的彩色图片,10个类别,每个类包含6,000张。其中50,000张图片作为训练集,10000张作为测试集。图11从每个类别中随机抽取了10张图片,展示了所有的类别。
+
+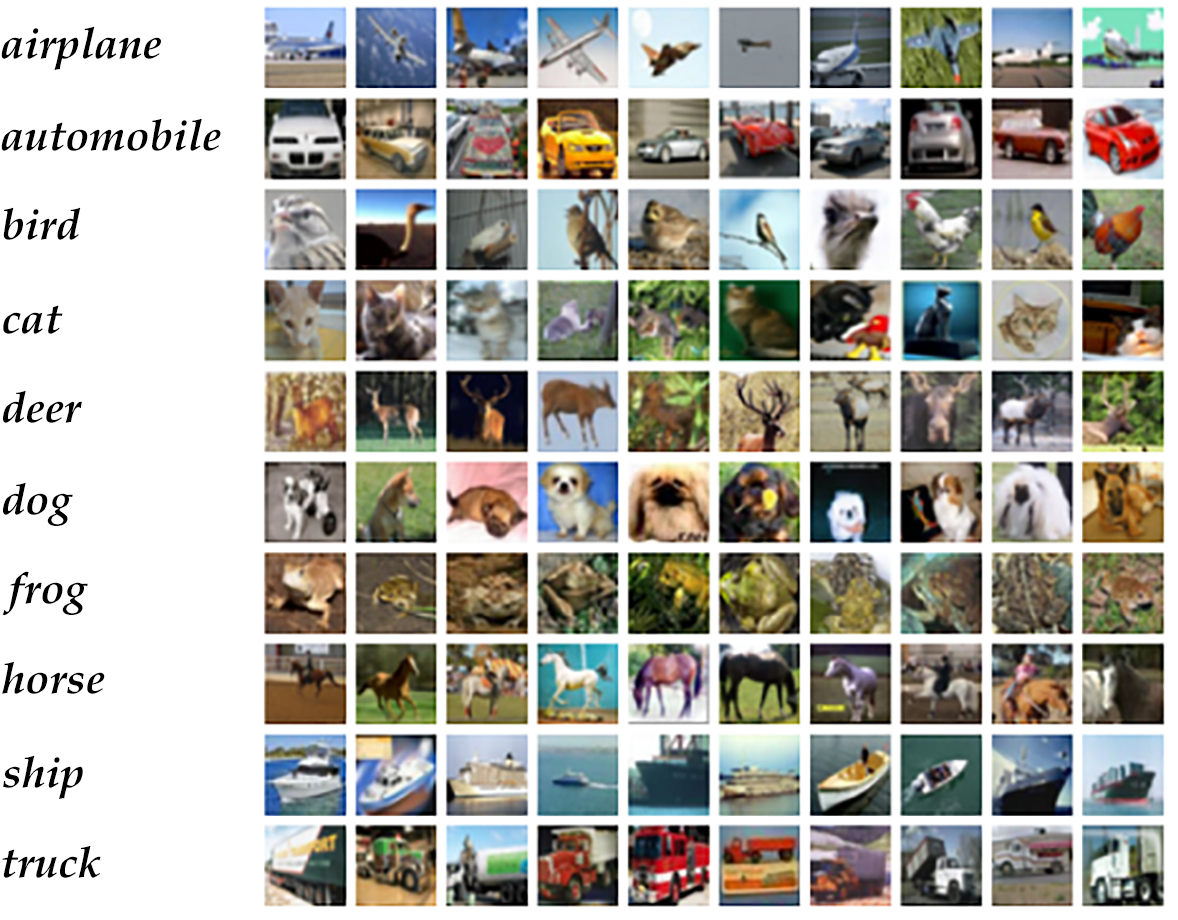
+
+图11. CIFAR10数据集[21]
+
+
+Paddle API提供了自动加载cifar数据集模块 `paddle.dataset.cifar`。
+
+通过输入`python train.py`,就可以开始训练模型了,以下小节将详细介绍`train.py`的相关内容。
+
+### 模型结构
+
+#### Paddle 初始化
+
+让我们从导入 Paddle Fluid API 和辅助模块开始。
+
+```python
+import paddle
+import paddle.fluid as fluid
+import numpy
+import sys
+```
+
+本教程中我们提供了VGG和ResNet两个模型的配置。
+
+#### VGG
+
+首先介绍VGG模型结构,由于CIFAR10图片大小和数量相比ImageNet数据小很多,因此这里的模型针对CIFAR10数据做了一定的适配。卷积部分引入了BN和Dropout操作。
+VGG核心模块的输入是数据层,`vgg_bn_drop` 定义了16层VGG结构,每层卷积后面引入BN层和Dropout层,详细的定义如下:
+
+```python
+def vgg_bn_drop(input):
+def conv_block(ipt, num_filter, groups, dropouts):
+return fluid.nets.img_conv_group(
+input=ipt,
+pool_size=2,
+pool_stride=2,
+conv_num_filter=[num_filter] * groups,
+conv_filter_size=3,
+conv_act='relu',
+conv_with_batchnorm=True,
+conv_batchnorm_drop_rate=dropouts,
+pool_type='max')
+
+conv1 = conv_block(input, 64, 2, [0.3, 0])
+conv2 = conv_block(conv1, 128, 2, [0.4, 0])
+conv3 = conv_block(conv2, 256, 3, [0.4, 0.4, 0])
+conv4 = conv_block(conv3, 512, 3, [0.4, 0.4, 0])
+conv5 = conv_block(conv4, 512, 3, [0.4, 0.4, 0])
+
+drop = fluid.layers.dropout(x=conv5, dropout_prob=0.5)
+fc1 = fluid.layers.fc(input=drop, size=512, act=None)
+bn = fluid.layers.batch_norm(input=fc1, act='relu')
+drop2 = fluid.layers.dropout(x=bn, dropout_prob=0.5)
+fc2 = fluid.layers.fc(input=drop2, size=512, act=None)
+predict = fluid.layers.fc(input=fc2, size=10, act='softmax')
+return predict
+```
+
+1. 首先定义了一组卷积网络,即conv_block。卷积核大小为3x3,池化窗口大小为2x2,窗口滑动大小为2,groups决定每组VGG模块是几次连续的卷积操作,dropouts指定Dropout操作的概率。所使用的`img_conv_group`是在`paddle.networks`中预定义的模块,由若干组 Conv->BN->ReLu->Dropout 和 一组 Pooling 组成。
+
+2. 五组卷积操作,即 5个conv_block。 第一、二组采用两次连续的卷积操作。第三、四、五组采用三次连续的卷积操作。每组最后一个卷积后面Dropout概率为0,即不使用Dropout操作。
+
+3. 最后接两层512维的全连接。
+
+4. 通过上面VGG网络提取高层特征,然后经过全连接层映射到类别维度大小的向量,再通过Softmax归一化得到每个类别的概率,也可称作分类器。
+
+### ResNet
+
+ResNet模型的第1、3、4步和VGG模型相同,这里不再介绍。主要介绍第2步即CIFAR10数据集上ResNet核心模块。
+
+先介绍`resnet_cifar10`中的一些基本函数,再介绍网络连接过程。
+
+- `conv_bn_layer` : 带BN的卷积层。
+- `shortcut` : 残差模块的"直连"路径,"直连"实际分两种形式:残差模块输入和输出特征通道数不等时,采用1x1卷积的升维操作;残差模块输入和输出通道相等时,采用直连操作。
+- `basicblock` : 一个基础残差模块,即图9左边所示,由两组3x3卷积组成的路径和一条"直连"路径组成。
+- `bottleneck` : 一个瓶颈残差模块,即图9右边所示,由上下1x1卷积和中间3x3卷积组成的路径和一条"直连"路径组成。
+- `layer_warp` : 一组残差模块,由若干个残差模块堆积而成。每组中第一个残差模块滑动窗口大小与其他可以不同,以用来减少特征图在垂直和水平方向的大小。
+
+```python
+def conv_bn_layer(input,
+ch_out,
+filter_size,
+stride,
+padding,
+act='relu',
+bias_attr=False):
+tmp = fluid.layers.conv2d(
+input=input,
+filter_size=filter_size,
+num_filters=ch_out,
+stride=stride,
+padding=padding,
+act=None,
+bias_attr=bias_attr)
+return fluid.layers.batch_norm(input=tmp, act=act)
+
+
+def shortcut(input, ch_in, ch_out, stride):
+if ch_in != ch_out:
+return conv_bn_layer(input, ch_out, 1, stride, 0, None)
+else:
+return input
+
+
+def basicblock(input, ch_in, ch_out, stride):
+tmp = conv_bn_layer(input, ch_out, 3, stride, 1)
+tmp = conv_bn_layer(tmp, ch_out, 3, 1, 1, act=None, bias_attr=True)
+short = shortcut(input, ch_in, ch_out, stride)
+return fluid.layers.elementwise_add(x=tmp, y=short, act='relu')
+
+
+def layer_warp(block_func, input, ch_in, ch_out, count, stride):
+tmp = block_func(input, ch_in, ch_out, stride)
+for i in range(1, count):
+tmp = block_func(tmp, ch_out, ch_out, 1)
+return tmp
+```
+
+`resnet_cifar10` 的连接结构主要有以下几个过程。
+
+1. 底层输入连接一层 `conv_bn_layer`,即带BN的卷积层。
+2. 然后连接3组残差模块即下面配置3组 `layer_warp` ,每组采用图 10 左边残差模块组成。
+3. 最后对网络做均值池化并返回该层。
+
+注意:除过第一层卷积层和最后一层全连接层之外,要求三组 `layer_warp` 总的含参层数能够被6整除,即 `resnet_cifar10` 的 depth 要满足 `$(depth - 2) % 6 == 0$` 。
+
+```python
+def resnet_cifar10(ipt, depth=32):
+# depth should be one of 20, 32, 44, 56, 110, 1202
+assert (depth - 2) % 6 == 0
+n = (depth - 2) / 6
+nStages = {16, 64, 128}
+conv1 = conv_bn_layer(ipt, ch_out=16, filter_size=3, stride=1, padding=1)
+res1 = layer_warp(basicblock, conv1, 16, 16, n, 1)
+res2 = layer_warp(basicblock, res1, 16, 32, n, 2)
+res3 = layer_warp(basicblock, res2, 32, 64, n, 2)
+pool = fluid.layers.pool2d(
+input=res3, pool_size=8, pool_type='avg', pool_stride=1)
+predict = fluid.layers.fc(input=pool, size=10, act='softmax')
+return predict
+```
+
+## Infererence Program 配置
+
+网络输入定义为 `data_layer` (数据层),在图像分类中即为图像像素信息。CIFRAR10是RGB 3通道32x32大小的彩色图,因此输入数据大小为3072(3x32x32)。
+
+```python
+def inference_program():
+# The image is 32 * 32 with RGB representation.
+data_shape = [3, 32, 32]
+images = fluid.layers.data(name='pixel', shape=data_shape, dtype='float32')
+
+predict = resnet_cifar10(images, 32)
+# predict = vgg_bn_drop(images) # un-comment to use vgg net
+return predict
+```
+
+## Train Program 配置
+
+然后我们需要设置训练程序 `train_program`。它首先从推理程序中进行预测。
+在训练期间,它将从预测中计算 `avg_cost`。
+在有监督训练中需要输入图像对应的类别信息,同样通过`fluid.layers.data`来定义。训练中采用多类交叉熵作为损失函数,并作为网络的输出,预测阶段定义网络的输出为分类器得到的概率信息。
+
+**注意:** 训练程序应该返回一个数组,第一个返回参数必须是 `avg_cost`。训练器使用它来计算梯度。
+
+```python
+def train_program():
+predict = inference_program()
+
+label = fluid.layers.data(name='label', shape=[1], dtype='int64')
+cost = fluid.layers.cross_entropy(input=predict, label=label)
+avg_cost = fluid.layers.mean(cost)
+accuracy = fluid.layers.accuracy(input=predict, label=label)
+return [avg_cost, accuracy]
+```
+
+## Optimizer Function 配置
+
+在下面的 `Adam optimizer`,`learning_rate` 是训练的速度,与网络的训练收敛速度有关系。
+
+```python
+def optimizer_program():
+return fluid.optimizer.Adam(learning_rate=0.001)
+```
+
+## 训练模型
+
+### Trainer 配置
+
+现在,我们需要配置 `Trainer`。`Trainer` 需要接受训练程序 `train_program`, `place` 和优化器 `optimizer_func`。
+
+```python
+use_cuda = False
+place = fluid.CUDAPlace(0) if use_cuda else fluid.CPUPlace()
+trainer = fluid.Trainer(
+train_func=train_program,
+optimizer_func=optimizer_program,
+place=place)
+```
+
+### Data Feeders 配置
+
+`cifar.train10()` 每次产生一条样本,在完成shuffle和batch之后,作为训练的输入。
+
+```python
+# Each batch will yield 128 images
+BATCH_SIZE = 128
+
+# Reader for training
+train_reader = paddle.batch(
+paddle.reader.shuffle(paddle.dataset.cifar.train10(), buf_size=50000),
+batch_size=BATCH_SIZE)
+
+# Reader for testing. A separated data set for testing.
+test_reader = paddle.batch(
+paddle.dataset.cifar.test10(), batch_size=BATCH_SIZE)
+```
+
+### Event Handler
+
+可以使用`event_handler`回调函数来观察训练过程,或进行测试等, 该回调函数是`trainer.train`函数里设定。
+
+`event_handler_plot`可以用来利用回调数据来打点画图:
+
+
+
+```python
+params_dirname = "image_classification_resnet.inference.model"
+
+from paddle.v2.plot import Ploter
+
+train_title = "Train cost"
+test_title = "Test cost"
+cost_ploter = Ploter(train_title, test_title)
+
+step = 0
+def event_handler_plot(event):
+global step
+if isinstance(event, fluid.EndStepEvent):
+if step % 1 == 0:
+cost_ploter.append(train_title, step, event.metrics[0])
+cost_ploter.plot()
+step += 1
+if isinstance(event, fluid.EndEpochEvent):
+avg_cost, accuracy = trainer.test(
+reader=test_reader,
+feed_order=['pixel', 'label'])
+cost_ploter.append(test_title, step, avg_cost)
+
+# save parameters
+if params_dirname is not None:
+trainer.save_params(params_dirname)
+```
+
+`event_handler` 用来在训练过程中输出文本日志
+
+```python
+params_dirname = "image_classification_resnet.inference.model"
+
+# event handler to track training and testing process
+def event_handler(event):
+if isinstance(event, fluid.EndStepEvent):
+if event.step % 100 == 0:
+print("\nPass %d, Batch %d, Cost %f, Acc %f" %
+(event.step, event.epoch, event.metrics[0],
+event.metrics[1]))
+else:
+sys.stdout.write('.')
+sys.stdout.flush()
+
+if isinstance(event, fluid.EndEpochEvent):
+# Test against with the test dataset to get accuracy.
+avg_cost, accuracy = trainer.test(
+reader=test_reader, feed_order=['pixel', 'label'])
+
+print('\nTest with Pass {0}, Loss {1:2.2}, Acc {2:2.2}'.format(event.epoch, avg_cost, accuracy))
+
+# save parameters
+if params_dirname is not None:
+trainer.save_params(params_dirname)
+```
+
+### 训练
+
+通过`trainer.train`函数训练:
+
+**注意:** CPU,每个 Epoch 将花费大约15~20分钟。这部分可能需要一段时间。请随意修改代码,在GPU上运行测试,以提高培训速度。
+
+```python
+trainer.train(
+reader=train_reader,
+num_epochs=2,
+event_handler=event_handler,
+feed_order=['pixel', 'label'])
+```
+
+一轮训练log示例如下所示,经过1个pass, 训练集上平均 Accuracy 为0.59 ,测试集上平均 Accuracy 为0.6 。
+
+```text
+Pass 0, Batch 0, Cost 3.869598, Acc 0.164062
+...................................................................................................
+Pass 100, Batch 0, Cost 1.481038, Acc 0.460938
+...................................................................................................
+Pass 200, Batch 0, Cost 1.340323, Acc 0.523438
+...................................................................................................
+Pass 300, Batch 0, Cost 1.223424, Acc 0.593750
+..........................................................................................
+Test with Pass 0, Loss 1.1, Acc 0.6
+```
+
+图12是训练的分类错误率曲线图,运行到第200个pass后基本收敛,最终得到测试集上分类错误率为8.54%。
+
+
+
+图12. CIFAR10数据集上VGG模型的分类错误率
+
+
+## 应用模型
+
+可以使用训练好的模型对图片进行分类,下面程序展示了如何使用 `fluid.Inferencer` 接口进行推断,可以打开注释,更改加载的模型。
+
+### 生成预测输入数据
+
+`dog.png` is an example image of a dog. Turn it into an numpy array to match the data feeder format.
+
+```python
+# Prepare testing data.
+from PIL import Image
+import numpy as np
+import os
+
+def load_image(file):
+im = Image.open(file)
+im = im.resize((32, 32), Image.ANTIALIAS)
+
+im = np.array(im).astype(np.float32)
+# The storage order of the loaded image is W(width),
+# H(height), C(channel). PaddlePaddle requires
+# the CHW order, so transpose them.
+im = im.transpose((2, 0, 1)) # CHW
+im = im / 255.0
+
+# Add one dimension to mimic the list format.
+im = numpy.expand_dims(im, axis=0)
+return im
+
+cur_dir = os.getcwd()
+img = load_image(cur_dir + '/image/dog.png')
+```
+
+### Inferencer 配置和预测
+
+`Inferencer` 需要一个 `infer_func` 和 `param_path` 来设置网络和经过训练的参数。
+我们可以简单地插入前面定义的推理程序。
+现在我们准备做预测。
+
+```python
+inferencer = fluid.Inferencer(
+infer_func=inference_program, param_path=params_dirname, place=place)
+
+# inference
+results = inferencer.infer({'pixel': img})
+print("infer results: ", results)
+```
+
+## 总结
+
+传统图像分类方法由多个阶段构成,框架较为复杂,而端到端的CNN模型结构可一步到位,而且大幅度提升了分类准确率。本文我们首先介绍VGG、GoogleNet、ResNet三个经典的模型;然后基于CIFAR10数据集,介绍如何使用PaddlePaddle配置和训练CNN模型,尤其是VGG和ResNet模型;最后介绍如何使用PaddlePaddle的API接口对图片进行预测和特征提取。对于其他数据集比如ImageNet,配置和训练流程是同样的,大家可以自行进行实验。
+
+
+## 参考文献
+
+[1] D. G. Lowe, [Distinctive image features from scale-invariant keypoints](http://www.cs.ubc.ca/~lowe/papers/ijcv04.pdf). IJCV, 60(2):91-110, 2004.
+
+[2] N. Dalal, B. Triggs, [Histograms of Oriented Gradients for Human Detection](http://vision.stanford.edu/teaching/cs231b_spring1213/papers/CVPR05_DalalTriggs.pdf), Proc. IEEE Conf. Computer Vision and Pattern Recognition, 2005.
+
+[3] Ahonen, T., Hadid, A., and Pietikinen, M. (2006). [Face description with local binary patterns: Application to face recognition](http://ieeexplore.ieee.org/document/1717463/). PAMI, 28.
+
+[4] J. Sivic, A. Zisserman, [Video Google: A Text Retrieval Approach to Object Matching in Videos](http://www.robots.ox.ac.uk/~vgg/publications/papers/sivic03.pdf), Proc. Ninth Int'l Conf. Computer Vision, pp. 1470-1478, 2003.
+
+[5] B. Olshausen, D. Field, [Sparse Coding with an Overcomplete Basis Set: A Strategy Employed by V1?](http://redwood.psych.cornell.edu/papers/olshausen_field_1997.pdf), Vision Research, vol. 37, pp. 3311-3325, 1997.
+
+[6] Wang, J., Yang, J., Yu, K., Lv, F., Huang, T., and Gong, Y. (2010). [Locality-constrained Linear Coding for image classification](http://ieeexplore.ieee.org/abstract/document/5540018/). In CVPR.
+
+[7] Perronnin, F., Sánchez, J., & Mensink, T. (2010). [Improving the fisher kernel for large-scale image classification](http://dl.acm.org/citation.cfm?id=1888101). In ECCV (4).
+
+[8] Lin, Y., Lv, F., Cao, L., Zhu, S., Yang, M., Cour, T., Yu, K., and Huang, T. (2011). [Large-scale image clas- sification: Fast feature extraction and SVM training](http://ieeexplore.ieee.org/document/5995477/). In CVPR.
+
+[9] Krizhevsky, A., Sutskever, I., and Hinton, G. (2012). [ImageNet classification with deep convolutional neu- ral networks](http://www.cs.toronto.edu/~kriz/imagenet_classification_with_deep_convolutional.pdf). In NIPS.
+
+[10] G.E. Hinton, N. Srivastava, A. Krizhevsky, I. Sutskever, and R.R. Salakhutdinov. [Improving neural networks by preventing co-adaptation of feature detectors](https://arxiv.org/abs/1207.0580). arXiv preprint arXiv:1207.0580, 2012.
+
+[11] K. Chatfield, K. Simonyan, A. Vedaldi, A. Zisserman. [Return of the Devil in the Details: Delving Deep into Convolutional Nets](https://arxiv.org/abs/1405.3531). BMVC, 2014。
+
+[12] Szegedy, C., Liu, W., Jia, Y., Sermanet, P., Reed, S., Anguelov, D., Erhan, D., Vanhoucke, V., Rabinovich, A., [Going deeper with convolutions](https://arxiv.org/abs/1409.4842). In: CVPR. (2015)
+
+[13] Lin, M., Chen, Q., and Yan, S. [Network in network](https://arxiv.org/abs/1312.4400). In Proc. ICLR, 2014.
+
+[14] S. Ioffe and C. Szegedy. [Batch normalization: Accelerating deep network training by reducing internal covariate shift](https://arxiv.org/abs/1502.03167). In ICML, 2015.
+
+[15] K. He, X. Zhang, S. Ren, J. Sun. [Deep Residual Learning for Image Recognition](https://arxiv.org/abs/1512.03385). CVPR 2016.
+
+[16] Szegedy, C., Vanhoucke, V., Ioffe, S., Shlens, J., Wojna, Z. [Rethinking the incep-tion architecture for computer vision](https://arxiv.org/abs/1512.00567). In: CVPR. (2016).
+
+[17] Szegedy, C., Ioffe, S., Vanhoucke, V. [Inception-v4, inception-resnet and the impact of residual connections on learning](https://arxiv.org/abs/1602.07261). arXiv:1602.07261 (2016).
+
+[18] Everingham, M., Eslami, S. M. A., Van Gool, L., Williams, C. K. I., Winn, J. and Zisserman, A. [The Pascal Visual Object Classes Challenge: A Retrospective]((http://link.springer.com/article/10.1007/s11263-014-0733-5)). International Journal of Computer Vision, 111(1), 98-136, 2015.
+
+[19] He, K., Zhang, X., Ren, S., and Sun, J. [Delving Deep into Rectifiers: Surpassing Human-Level Performance on ImageNet Classification](https://arxiv.org/abs/1502.01852). ArXiv e-prints, February 2015.
+
+[20] http://deeplearning.net/tutorial/lenet.html
+
+[21] https://www.cs.toronto.edu/~kriz/cifar.html
+
+[22] http://cs231n.github.io/classification/
+
+
+
本教程 由 PaddlePaddle 创作,采用 知识共享 署名-相同方式共享 4.0 国际 许可协议进行许可。
diff --git a/doc/fluid/new_docs/beginners_guide/basics/index.rst b/doc/fluid/new_docs/beginners_guide/basics/index.rst
new file mode 100644
index 0000000000000000000000000000000000000000..d16f8b947253a535567ddc8d7b227dd153d9b154
--- /dev/null
+++ b/doc/fluid/new_docs/beginners_guide/basics/index.rst
@@ -0,0 +1,18 @@
+################
+深度学习基础知识
+################
+
+
+.. todo::
+
+ 概述
+
+.. toctree::
+ :maxdepth: 2
+
+ image_classification/index.md
+ word2vec/index.md
+ recommender_system/index.md
+ understand_sentiment/index.md
+ label_semantic_roles/index.md
+ machine_translation/index.md
diff --git a/doc/fluid/new_docs/beginners_guide/basics/label_semantic_roles/.gitignore b/doc/fluid/new_docs/beginners_guide/basics/label_semantic_roles/.gitignore
new file mode 100644
index 0000000000000000000000000000000000000000..29b5622a53a1b0847e9f53febf1cc50dcf4f044a
--- /dev/null
+++ b/doc/fluid/new_docs/beginners_guide/basics/label_semantic_roles/.gitignore
@@ -0,0 +1,12 @@
+data/train.list
+data/test.*
+data/conll05st-release.tar.gz
+data/conll05st-release
+data/predicate_dict
+data/label_dict
+data/word_dict
+data/emb
+data/feature
+output
+predict.res
+train.log
diff --git a/doc/fluid/new_docs/beginners_guide/basics/label_semantic_roles/image/bidirectional_stacked_lstm.png b/doc/fluid/new_docs/beginners_guide/basics/label_semantic_roles/image/bidirectional_stacked_lstm.png
new file mode 100644
index 0000000000000000000000000000000000000000..e63f5ebd6d00f2e4ecf97b9ab2027e74683013f2
Binary files /dev/null and b/doc/fluid/new_docs/beginners_guide/basics/label_semantic_roles/image/bidirectional_stacked_lstm.png differ
diff --git a/doc/fluid/new_docs/beginners_guide/basics/label_semantic_roles/image/bidirectional_stacked_lstm_en.png b/doc/fluid/new_docs/beginners_guide/basics/label_semantic_roles/image/bidirectional_stacked_lstm_en.png
new file mode 100755
index 0000000000000000000000000000000000000000..f0a195c24d9ee493f96bb93c28a99e70566be7a4
Binary files /dev/null and b/doc/fluid/new_docs/beginners_guide/basics/label_semantic_roles/image/bidirectional_stacked_lstm_en.png differ
diff --git a/doc/fluid/new_docs/beginners_guide/basics/label_semantic_roles/image/bio_example.png b/doc/fluid/new_docs/beginners_guide/basics/label_semantic_roles/image/bio_example.png
new file mode 100755
index 0000000000000000000000000000000000000000..e5f7151c9fcc50a7cf7af485cbbc7e4fccab0c20
Binary files /dev/null and b/doc/fluid/new_docs/beginners_guide/basics/label_semantic_roles/image/bio_example.png differ
diff --git a/doc/fluid/new_docs/beginners_guide/basics/label_semantic_roles/image/bio_example_en.png b/doc/fluid/new_docs/beginners_guide/basics/label_semantic_roles/image/bio_example_en.png
new file mode 100755
index 0000000000000000000000000000000000000000..93b44dd4874402ef29ad7bd7d94147609b92e309
Binary files /dev/null and b/doc/fluid/new_docs/beginners_guide/basics/label_semantic_roles/image/bio_example_en.png differ
diff --git a/doc/fluid/new_docs/beginners_guide/basics/label_semantic_roles/image/db_lstm_network.png b/doc/fluid/new_docs/beginners_guide/basics/label_semantic_roles/image/db_lstm_network.png
new file mode 100644
index 0000000000000000000000000000000000000000..592f7ee23bdc88a9a35059612e5ab880bbc9d34b
Binary files /dev/null and b/doc/fluid/new_docs/beginners_guide/basics/label_semantic_roles/image/db_lstm_network.png differ
diff --git a/doc/fluid/new_docs/beginners_guide/basics/label_semantic_roles/image/db_lstm_network_en.png b/doc/fluid/new_docs/beginners_guide/basics/label_semantic_roles/image/db_lstm_network_en.png
new file mode 100755
index 0000000000000000000000000000000000000000..c3646312e48db977402fb353dc0c9b4d02269bf4
Binary files /dev/null and b/doc/fluid/new_docs/beginners_guide/basics/label_semantic_roles/image/db_lstm_network_en.png differ
diff --git a/doc/fluid/new_docs/beginners_guide/basics/label_semantic_roles/image/dependency_parsing.png b/doc/fluid/new_docs/beginners_guide/basics/label_semantic_roles/image/dependency_parsing.png
new file mode 100755
index 0000000000000000000000000000000000000000..9265b671735940ed6549e2980064d2ce08baae64
Binary files /dev/null and b/doc/fluid/new_docs/beginners_guide/basics/label_semantic_roles/image/dependency_parsing.png differ
diff --git a/doc/fluid/new_docs/beginners_guide/basics/label_semantic_roles/image/dependency_parsing_en.png b/doc/fluid/new_docs/beginners_guide/basics/label_semantic_roles/image/dependency_parsing_en.png
new file mode 100755
index 0000000000000000000000000000000000000000..23f4f45b603e3d60702af2b2464d10fc8deed061
Binary files /dev/null and b/doc/fluid/new_docs/beginners_guide/basics/label_semantic_roles/image/dependency_parsing_en.png differ
diff --git a/doc/fluid/new_docs/beginners_guide/basics/label_semantic_roles/image/linear_chain_crf.png b/doc/fluid/new_docs/beginners_guide/basics/label_semantic_roles/image/linear_chain_crf.png
new file mode 100644
index 0000000000000000000000000000000000000000..0778fda74b2ad22ce4b631791a7b028cdef780a5
Binary files /dev/null and b/doc/fluid/new_docs/beginners_guide/basics/label_semantic_roles/image/linear_chain_crf.png differ
diff --git a/doc/fluid/new_docs/beginners_guide/basics/label_semantic_roles/image/stacked_lstm.png b/doc/fluid/new_docs/beginners_guide/basics/label_semantic_roles/image/stacked_lstm.png
new file mode 100644
index 0000000000000000000000000000000000000000..3d2914c726b5f4c46e66dfa85d4e88649fede6b3
Binary files /dev/null and b/doc/fluid/new_docs/beginners_guide/basics/label_semantic_roles/image/stacked_lstm.png differ
diff --git a/doc/fluid/new_docs/beginners_guide/basics/label_semantic_roles/image/stacked_lstm_en.png b/doc/fluid/new_docs/beginners_guide/basics/label_semantic_roles/image/stacked_lstm_en.png
new file mode 100755
index 0000000000000000000000000000000000000000..0b944ef91e8b5ba4b14d2a35bd8879f261cf8f61
Binary files /dev/null and b/doc/fluid/new_docs/beginners_guide/basics/label_semantic_roles/image/stacked_lstm_en.png differ
diff --git a/doc/fluid/new_docs/beginners_guide/basics/label_semantic_roles/index.md b/doc/fluid/new_docs/beginners_guide/basics/label_semantic_roles/index.md
new file mode 100644
index 0000000000000000000000000000000000000000..828ca738317992270487647e66b08b6d2f80e209
--- /dev/null
+++ b/doc/fluid/new_docs/beginners_guide/basics/label_semantic_roles/index.md
@@ -0,0 +1,568 @@
+# 语义角色标注
+
+本教程源代码目录在[book/label_semantic_roles](https://github.com/PaddlePaddle/book/tree/develop/07.label_semantic_roles), 初次使用请参考PaddlePaddle[安装教程](https://github.com/PaddlePaddle/book/blob/develop/README.cn.md#运行这本书)。
+
+## 背景介绍
+
+自然语言分析技术大致分为三个层面:词法分析、句法分析和语义分析。语义角色标注是实现浅层语义分析的一种方式。在一个句子中,谓词是对主语的陈述或说明,指出“做什么”、“是什么”或“怎么样,代表了一个事件的核心,跟谓词搭配的名词称为论元。语义角色是指论元在动词所指事件中担任的角色。主要有:施事者(Agent)、受事者(Patient)、客体(Theme)、经验者(Experiencer)、受益者(Beneficiary)、工具(Instrument)、处所(Location)、目标(Goal)和来源(Source)等。
+
+请看下面的例子,“遇到” 是谓词(Predicate,通常简写为“Pred”),“小明”是施事者(Agent),“小红”是受事者(Patient),“昨天” 是事件发生的时间(Time),“公园”是事情发生的地点(Location)。
+
+$$\mbox{[小明]}_{\mbox{Agent}}\mbox{[昨天]}_{\mbox{Time}}\mbox{[晚上]}_{\mbox{Time}}\mbox{在[公园]}_{\mbox{Location}}\mbox{[遇到]}_{\mbox{Predicate}}\mbox{了[小红]}_{\mbox{Patient}}\mbox{。}$$
+
+语义角色标注(Semantic Role Labeling,SRL)以句子的谓词为中心,不对句子所包含的语义信息进行深入分析,只分析句子中各成分与谓词之间的关系,即句子的谓词(Predicate)- 论元(Argument)结构,并用语义角色来描述这些结构关系,是许多自然语言理解任务(如信息抽取,篇章分析,深度问答等)的一个重要中间步骤。在研究中一般都假定谓词是给定的,所要做的就是找出给定谓词的各个论元和它们的语义角色。
+
+传统的SRL系统大多建立在句法分析基础之上,通常包括5个流程:
+
+1. 构建一棵句法分析树,例如,图1是对上面例子进行依存句法分析得到的一棵句法树。
+2. 从句法树上识别出给定谓词的候选论元。
+3. 候选论元剪除;一个句子中的候选论元可能很多,候选论元剪除就是从大量的候选项中剪除那些最不可能成为论元的候选项。
+4. 论元识别:这个过程是从上一步剪除之后的候选中判断哪些是真正的论元,通常当做一个二分类问题来解决。
+5. 对第4步的结果,通过多分类得到论元的语义角色标签。可以看到,句法分析是基础,并且后续步骤常常会构造的一些人工特征,这些特征往往也来自句法分析。
+
+
+
+图1. 依存句法分析句法树示例
+
+
+然而,完全句法分析需要确定句子所包含的全部句法信息,并确定句子各成分之间的关系,是一个非常困难的任务,目前技术下的句法分析准确率并不高,句法分析的细微错误都会导致SRL的错误。为了降低问题的复杂度,同时获得一定的句法结构信息,“浅层句法分析”的思想应运而生。浅层句法分析也称为部分句法分析(partial parsing)或语块划分(chunking)。和完全句法分析得到一颗完整的句法树不同,浅层句法分析只需要识别句子中某些结构相对简单的独立成分,例如:动词短语,这些被识别出来的结构称为语块。为了回避 “无法获得准确率较高的句法树” 所带来的困难,一些研究\[[1](#参考文献)\]也提出了基于语块(chunk)的SRL方法。基于语块的SRL方法将SRL作为一个序列标注问题来解决。序列标注任务一般都会采用BIO表示方式来定义序列标注的标签集,我们先来介绍这种表示方法。在BIO表示法中,B代表语块的开始,I代表语块的中间,O代表语块结束。通过B、I、O 三种标记将不同的语块赋予不同的标签,例如:对于一个角色为A的论元,将它所包含的第一个语块赋予标签B-A,将它所包含的其它语块赋予标签I-A,不属于任何论元的语块赋予标签O。
+
+我们继续以上面的这句话为例,图1展示了BIO表示方法。
+
+
+
+图2. BIO标注方法示例
+
+
+从上面的例子可以看到,根据序列标注结果可以直接得到论元的语义角色标注结果,是一个相对简单的过程。这种简单性体现在:(1)依赖浅层句法分析,降低了句法分析的要求和难度;(2)没有了候选论元剪除这一步骤;(3)论元的识别和论元标注是同时实现的。这种一体化处理论元识别和论元标注的方法,简化了流程,降低了错误累积的风险,往往能够取得更好的结果。
+
+与基于语块的SRL方法类似,在本教程中我们也将SRL看作一个序列标注问题,不同的是,我们只依赖输入文本序列,不依赖任何额外的语法解析结果或是复杂的人造特征,利用深度神经网络构建一个端到端学习的SRL系统。我们以[CoNLL-2004 and CoNLL-2005 Shared Tasks](http://www.cs.upc.edu/~srlconll/)任务中SRL任务的公开数据集为例,实践下面的任务:给定一句话和这句话里的一个谓词,通过序列标注的方式,从句子中找到谓词对应的论元,同时标注它们的语义角色。
+
+## 模型概览
+
+循环神经网络(Recurrent Neural Network)是一种对序列建模的重要模型,在自然语言处理任务中有着广泛地应用。不同于前馈神经网络(Feed-forward Neural Network),RNN能够处理输入之间前后关联的问题。LSTM是RNN的一种重要变种,常用来学习长序列中蕴含的长程依赖关系,我们在[情感分析](https://github.com/PaddlePaddle/book/tree/develop/05.understand_sentiment)一篇中已经介绍过,这一篇中我们依然利用LSTM来解决SRL问题。
+
+### 栈式循环神经网络(Stacked Recurrent Neural Network)
+
+深层网络有助于形成层次化特征,网络上层在下层已经学习到的初级特征基础上,形成更复杂的高级特征。尽管LSTM沿时间轴展开后等价于一个非常“深”的前馈网络,但由于LSTM各个时间步参数共享,`$t-1$`时刻状态到`$t$`时刻的映射,始终只经过了一次非线性映射,也就是说单层LSTM对状态转移的建模是 “浅” 的。堆叠多个LSTM单元,令前一个LSTM`$t$`时刻的输出,成为下一个LSTM单元`$t$`时刻的输入,帮助我们构建起一个深层网络,我们把它称为第一个版本的栈式循环神经网络。深层网络提高了模型拟合复杂模式的能力,能够更好地建模跨不同时间步的模式\[[2](#参考文献)\]。
+
+然而,训练一个深层LSTM网络并非易事。纵向堆叠多个LSTM单元可能遇到梯度在纵向深度上传播受阻的问题。通常,堆叠4层LSTM单元可以正常训练,当层数达到4~8层时,会出现性能衰减,这时必须考虑一些新的结构以保证梯度纵向顺畅传播,这是训练深层LSTM网络必须解决的问题。我们可以借鉴LSTM解决 “梯度消失梯度爆炸” 问题的智慧之一:在记忆单元(Memory Cell)这条信息传播的路线上没有非线性映射,当梯度反向传播时既不会衰减、也不会爆炸。因此,深层LSTM模型也可以在纵向上添加一条保证梯度顺畅传播的路径。
+
+一个LSTM单元完成的运算可以被分为三部分:(1)输入到隐层的映射(input-to-hidden) :每个时间步输入信息`$x$`会首先经过一个矩阵映射,再作为遗忘门,输入门,记忆单元,输出门的输入,注意,这一次映射没有引入非线性激活;(2)隐层到隐层的映射(hidden-to-hidden):这一步是LSTM计算的主体,包括遗忘门,输入门,记忆单元更新,输出门的计算;(3)隐层到输出的映射(hidden-to-output):通常是简单的对隐层向量进行激活。我们在第一个版本的栈式网络的基础上,加入一条新的路径:除上一层LSTM输出之外,将前层LSTM的输入到隐层的映射作为的一个新的输入,同时加入一个线性映射去学习一个新的变换。
+
+图3是最终得到的栈式循环神经网络结构示意图。
+
+
+
+图3. 基于LSTM的栈式循环神经网络结构示意图
+
+
+### 双向循环神经网络(Bidirectional Recurrent Neural Network)
+
+在LSTM中,`$t$`时刻的隐藏层向量编码了到`$t$`时刻为止所有输入的信息,但`$t$`时刻的LSTM可以看到历史,却无法看到未来。在绝大多数自然语言处理任务中,我们几乎总是能拿到整个句子。这种情况下,如果能够像获取历史信息一样,得到未来的信息,对序列学习任务会有很大的帮助。
+
+为了克服这一缺陷,我们可以设计一种双向循环网络单元,它的思想简单且直接:对上一节的栈式循环神经网络进行一个小小的修改,堆叠多个LSTM单元,让每一层LSTM单元分别以:正向、反向、正向 …… 的顺序学习上一层的输出序列。于是,从第2层开始,`$t$`时刻我们的LSTM单元便总是可以看到历史和未来的信息。图4是基于LSTM的双向循环神经网络结构示意图。
+
+
+
+图4. 基于LSTM的双向循环神经网络结构示意图
+
+
+需要说明的是,这种双向RNN结构和Bengio等人在机器翻译任务中使用的双向RNN结构\[[3](#参考文献), [4](#参考文献)\] 并不相同,我们会在后续[机器翻译](https://github.com/PaddlePaddle/book/blob/develop/08.machine_translation/README.cn.md)任务中,介绍另一种双向循环神经网络。
+
+### 条件随机场 (Conditional Random Field)
+
+使用神经网络模型解决问题的思路通常是:前层网络学习输入的特征表示,网络的最后一层在特征基础上完成最终的任务。在SRL任务中,深层LSTM网络学习输入的特征表示,条件随机场(Conditional Random Filed, CRF)在特征的基础上完成序列标注,处于整个网络的末端。
+
+CRF是一种概率化结构模型,可以看作是一个概率无向图模型,结点表示随机变量,边表示随机变量之间的概率依赖关系。简单来讲,CRF学习条件概率`$P(X|Y)$`,其中 `$X = (x_1, x_2, ... , x_n)$` 是输入序列,`$Y = (y_1, y_2, ... , y_n)$` 是标记序列;解码过程是给定 `$X$`序列求解令`$P(Y|X)$`最大的`$Y$`序列,即`$Y^* = \mbox{arg max}_{Y} P(Y | X)$`。
+
+序列标注任务只需要考虑输入和输出都是一个线性序列,并且由于我们只是将输入序列作为条件,不做任何条件独立假设,因此输入序列的元素之间并不存在图结构。综上,在序列标注任务中使用的是如图5所示的定义在链式图上的CRF,称之为线性链条件随机场(Linear Chain Conditional Random Field)。
+
+
+
+图5. 序列标注任务中使用的线性链条件随机场
+
+
+根据线性链条件随机场上的因子分解定理\[[5](#参考文献)\],在给定观测序列`$X$`时,一个特定标记序列`$Y$`的概率可以定义为:
+
+$$p(Y | X) = \frac{1}{Z(X)} \text{exp}\left(\sum_{i=1}^{n}\left(\sum_{j}\lambda_{j}t_{j} (y_{i - 1}, y_{i}, X, i) + \sum_{k} \mu_k s_k (y_i, X, i)\right)\right)$$
+
+其中`$Z(X)$`是归一化因子,`$t_j$` 是定义在边上的特征函数,依赖于当前和前一个位置,称为转移特征,表示对于输入序列`$X$`及其标注序列在 `$i$`及`$i - 1$`位置上标记的转移概率。`$s_k$`是定义在结点上的特征函数,称为状态特征,依赖于当前位置,表示对于观察序列`$X$`及其`$i$`位置的标记概率。`$\lambda_j$` 和 `$\mu_k$` 分别是转移特征函数和状态特征函数对应的权值。实际上,`$t$`和`$s$`可以用相同的数学形式表示,再对转移特征和状态特在各个位置`$i$`求和有:`$f_{k}(Y, X) = \sum_{i=1}^{n}f_k({y_{i - 1}, y_i, X, i})$`,把`$f$`统称为特征函数,于是`$P(Y|X)$`可表示为:
+
+$$p(Y|X, W) = \frac{1}{Z(X)}\text{exp}\sum_{k}\omega_{k}f_{k}(Y, X)$$
+
+`$\omega$`是特征函数对应的权值,是CRF模型要学习的参数。训练时,对于给定的输入序列和对应的标记序列集合`$D = \left[(X_1, Y_1), (X_2 , Y_2) , ... , (X_N, Y_N)\right]$` ,通过正则化的极大似然估计,求解如下优化目标:
+
+$$\DeclareMathOperator*{\argmax}{arg\,max} L(\lambda, D) = - \text{log}\left(\prod_{m=1}^{N}p(Y_m|X_m, W)\right) + C \frac{1}{2}\lVert W\rVert^{2}$$
+
+这个优化目标可以通过反向传播算法和整个神经网络一起求解。解码时,对于给定的输入序列`$X$`,通过解码算法(通常有:维特比算法、Beam Search)求令出条件概率`$\bar{P}(Y|X)$`最大的输出序列 `$\bar{Y}$`。
+
+### 深度双向LSTM(DB-LSTM)SRL模型
+
+在SRL任务中,输入是 “谓词” 和 “一句话”,目标是从这句话中找到谓词的论元,并标注论元的语义角色。如果一个句子含有`$n$`个谓词,这个句子会被处理`$n$`次。一个最为直接的模型是下面这样:
+
+1. 构造输入;
+- 输入1是谓词,输入2是句子
+- 将输入1扩展成和输入2一样长的序列,用one-hot方式表示;
+2. one-hot方式的谓词序列和句子序列通过词表,转换为实向量表示的词向量序列;
+3. 将步骤2中的2个词向量序列作为双向LSTM的输入,学习输入序列的特征表示;
+4. CRF以步骤3中模型学习到的特征为输入,以标记序列为监督信号,实现序列标注;
+
+大家可以尝试上面这种方法。这里,我们提出一些改进,引入两个简单但对提高系统性能非常有效的特征:
+
+- 谓词上下文:上面的方法中,只用到了谓词的词向量表达谓词相关的所有信息,这种方法始终是非常弱的,特别是如果谓词在句子中出现多次,有可能引起一定的歧义。从经验出发,谓词前后若干个词的一个小片段,能够提供更丰富的信息,帮助消解歧义。于是,我们把这样的经验也添加到模型中,为每个谓词同时抽取一个“谓词上下文” 片段,也就是从这个谓词前后各取`$n$`个词构成的一个窗口片段;
+- 谓词上下文区域标记:为句子中的每一个词引入一个0-1二值变量,表示它们是否在“谓词上下文”片段中;
+
+修改后的模型如下(图6是一个深度为4的模型结构示意图):
+
+1. 构造输入
+- 输入1是句子序列,输入2是谓词序列,输入3是谓词上下文,从句子中抽取这个谓词前后各`$n$`个词,构成谓词上下文,用one-hot方式表示,输入4是谓词上下文区域标记,标记了句子中每一个词是否在谓词上下文中;
+- 将输入2~3均扩展为和输入1一样长的序列;
+2. 输入1~4均通过词表取词向量转换为实向量表示的词向量序列;其中输入1、3共享同一个词表,输入2和4各自独有词表;
+3. 第2步的4个词向量序列作为双向LSTM模型的输入;LSTM模型学习输入序列的特征表示,得到新的特性表示序列;
+4. CRF以第3步中LSTM学习到的特征为输入,以标记序列为监督信号,完成序列标注;
+
+
+
+图6. SRL任务上的深层双向LSTM模型
+
+
+
+## 数据介绍
+
+在此教程中,我们选用[CoNLL 2005](http://www.cs.upc.edu/~srlconll/)SRL任务开放出的数据集作为示例。需要特别说明的是,CoNLL 2005 SRL任务的训练数集和开发集在比赛之后并非免费进行公开,目前,能够获取到的只有测试集,包括Wall Street Journal的23节和Brown语料集中的3节。在本教程中,我们以测试集中的WSJ数据为训练集来讲解模型。但是,由于测试集中样本的数量远远不够,如果希望训练一个可用的神经网络SRL系统,请考虑付费获取全量数据。
+
+原始数据中同时包括了词性标注、命名实体识别、语法解析树等多种信息。本教程中,我们使用test.wsj文件夹中的数据进行训练和测试,并只会用到words文件夹(文本序列)和props文件夹(标注结果)下的数据。本教程使用的数据目录如下:
+
+```text
+conll05st-release/
+└── test.wsj
+├── props # 标注结果
+└── words # 输入文本序列
+```
+
+标注信息源自Penn TreeBank\[[7](#参考文献)\]和PropBank\[[8](#参考文献)\]的标注结果。PropBank标注结果的标签和我们在文章一开始示例中使用的标注结果标签不同,但原理是相同的,关于标注结果标签含义的说明,请参考论文\[[9](#参考文献)\]。
+
+原始数据需要进行数据预处理才能被PaddlePaddle处理,预处理包括下面几个步骤:
+
+1. 将文本序列和标记序列其合并到一条记录中;
+2. 一个句子如果含有`$n$`个谓词,这个句子会被处理`$n$`次,变成`$n$`条独立的训练样本,每个样本一个不同的谓词;
+3. 抽取谓词上下文和构造谓词上下文区域标记;
+4. 构造以BIO法表示的标记;
+5. 依据词典获取词对应的整数索引。
+
+
+```python
+# import paddle.v2.dataset.conll05 as conll05
+# conll05.corpus_reader函数完成上面第1步和第2步.
+# conll05.reader_creator函数完成上面第3步到第5步.
+# conll05.test函数可以获取处理之后的每条样本来供PaddlePaddle训练.
+```
+
+预处理完成之后一条训练样本包含9个特征,分别是:句子序列、谓词、谓词上下文(占 5 列)、谓词上下区域标志、标注序列。下表是一条训练样本的示例。
+
+| 句子序列 | 谓词 | 谓词上下文(窗口 = 5) | 谓词上下文区域标记 | 标注序列 |
+|---|---|---|---|---|
+| A | set | n't been set . × | 0 | B-A1 |
+| record | set | n't been set . × | 0 | I-A1 |
+| date | set | n't been set . × | 0 | I-A1 |
+| has | set | n't been set . × | 0 | O |
+| n't | set | n't been set . × | 1 | B-AM-NEG |
+| been | set | n't been set . × | 1 | O |
+| set | set | n't been set . × | 1 | B-V |
+| . | set | n't been set . × | 1 | O |
+
+
+除数据之外,我们同时提供了以下资源:
+
+| 文件名称 | 说明 |
+|---|---|
+| word_dict | 输入句子的词典,共计44068个词 |
+| label_dict | 标记的词典,共计106个标记 |
+| predicate_dict | 谓词的词典,共计3162个词 |
+| emb | 一个训练好的词表,32维 |
+
+我们在英文维基百科上训练语言模型得到了一份词向量用来初始化SRL模型。在SRL模型训练过程中,词向量不再被更新。关于语言模型和词向量可以参考[词向量](https://github.com/PaddlePaddle/book/blob/develop/04.word2vec/README.cn.md) 这篇教程。我们训练语言模型的语料共有995,000,000个token,词典大小控制为4900,000词。CoNLL 2005训练语料中有5%的词不在这4900,000个词中,我们将它们全部看作未登录词,用``表示。
+
+获取词典,打印词典大小:
+
+```python
+import math, os
+import numpy as np
+import paddle
+import paddle.v2.dataset.conll05 as conll05
+import paddle.fluid as fluid
+import time
+
+with_gpu = os.getenv('WITH_GPU', '0') != '0'
+
+word_dict, verb_dict, label_dict = conll05.get_dict()
+word_dict_len = len(word_dict)
+label_dict_len = len(label_dict)
+pred_dict_len = len(verb_dict)
+
+print word_dict_len
+print label_dict_len
+print pred_dict_len
+```
+
+## 模型配置说明
+
+- 定义输入数据维度及模型超参数。
+
+```python
+mark_dict_len = 2 # 谓上下文区域标志的维度,是一个0-1 2值特征,因此维度为2
+word_dim = 32 # 词向量维度
+mark_dim = 5 # 谓词上下文区域通过词表被映射为一个实向量,这个是相邻的维度
+hidden_dim = 512 # LSTM隐层向量的维度 : 512 / 4
+depth = 8 # 栈式LSTM的深度
+mix_hidden_lr = 1e-3
+
+IS_SPARSE = True
+PASS_NUM = 10
+BATCH_SIZE = 10
+
+embedding_name = 'emb'
+```
+
+这里需要特别说明的是hidden_dim = 512指定了LSTM隐层向量的维度为128维,关于这一点请参考PaddlePaddle官方文档中[lstmemory](http://www.paddlepaddle.org/doc/ui/api/trainer_config_helpers/layers.html#lstmemory)的说明。
+
+- 如上文提到,我们用基于英文维基百科训练好的词向量来初始化序列输入、谓词上下文总共6个特征的embedding层参数,在训练中不更新。
+
+```python
+# 这里加载PaddlePaddle上版保存的二进制模型
+def load_parameter(file_name, h, w):
+with open(file_name, 'rb') as f:
+f.read(16) # skip header.
+return np.fromfile(f, dtype=np.float32).reshape(h, w)
+```
+
+- 8个LSTM单元以“正向/反向”的顺序对所有输入序列进行学习。
+
+```python
+def db_lstm(word, predicate, ctx_n2, ctx_n1, ctx_0, ctx_p1, ctx_p2, mark,
+**ignored):
+# 8 features
+predicate_embedding = fluid.layers.embedding(
+input=predicate,
+size=[pred_dict_len, word_dim],
+dtype='float32',
+is_sparse=IS_SPARSE,
+param_attr='vemb')
+
+mark_embedding = fluid.layers.embedding(
+input=mark,
+size=[mark_dict_len, mark_dim],
+dtype='float32',
+is_sparse=IS_SPARSE)
+
+word_input = [word, ctx_n2, ctx_n1, ctx_0, ctx_p1, ctx_p2]
+# Since word vector lookup table is pre-trained, we won't update it this time.
+# trainable being False prevents updating the lookup table during training.
+emb_layers = [
+fluid.layers.embedding(
+size=[word_dict_len, word_dim],
+input=x,
+param_attr=fluid.ParamAttr(
+name=embedding_name, trainable=False)) for x in word_input
+]
+emb_layers.append(predicate_embedding)
+emb_layers.append(mark_embedding)
+
+# 8 LSTM units are trained through alternating left-to-right / right-to-left order
+# denoted by the variable `reverse`.
+hidden_0_layers = [
+fluid.layers.fc(input=emb, size=hidden_dim, act='tanh')
+for emb in emb_layers
+]
+
+hidden_0 = fluid.layers.sums(input=hidden_0_layers)
+
+lstm_0 = fluid.layers.dynamic_lstm(
+input=hidden_0,
+size=hidden_dim,
+candidate_activation='relu',
+gate_activation='sigmoid',
+cell_activation='sigmoid')
+
+# stack L-LSTM and R-LSTM with direct edges
+input_tmp = [hidden_0, lstm_0]
+
+# In PaddlePaddle, state features and transition features of a CRF are implemented
+# by a fully connected layer and a CRF layer seperately. The fully connected layer
+# with linear activation learns the state features, here we use fluid.layers.sums
+# (fluid.layers.fc can be uesed as well), and the CRF layer in PaddlePaddle:
+# fluid.layers.linear_chain_crf only
+# learns the transition features, which is a cost layer and is the last layer of the network.
+# fluid.layers.linear_chain_crf outputs the log probability of true tag sequence
+# as the cost by given the input sequence and it requires the true tag sequence
+# as target in the learning process.
+
+for i in range(1, depth):
+mix_hidden = fluid.layers.sums(input=[
+fluid.layers.fc(input=input_tmp[0], size=hidden_dim, act='tanh'),
+fluid.layers.fc(input=input_tmp[1], size=hidden_dim, act='tanh')
+])
+
+lstm = fluid.layers.dynamic_lstm(
+input=mix_hidden,
+size=hidden_dim,
+candidate_activation='relu',
+gate_activation='sigmoid',
+cell_activation='sigmoid',
+is_reverse=((i % 2) == 1))
+
+input_tmp = [mix_hidden, lstm]
+
+# 取最后一个栈式LSTM的输出和这个LSTM单元的输入到隐层映射,
+# 经过一个全连接层映射到标记字典的维度,来学习 CRF 的状态特征
+feature_out = fluid.layers.sums(input=[
+fluid.layers.fc(input=input_tmp[0], size=label_dict_len, act='tanh'),
+fluid.layers.fc(input=input_tmp[1], size=label_dict_len, act='tanh')
+])
+
+return feature_out
+```
+
+## 训练模型
+
+- 我们根据网络拓扑结构和模型参数来构造出trainer用来训练,在构造时还需指定优化方法,这里使用最基本的SGD方法(momentum设置为0),同时设定了学习率、正则等。
+
+- 数据介绍部分提到CoNLL 2005训练集付费,这里我们使用测试集训练供大家学习。conll05.test()每次产生一条样本,包含9个特征,shuffle和组完batch后作为训练的输入。
+
+- 通过feeding来指定每一个数据和data_layer的对应关系。 例如 下面feeding表示: conll05.test()产生数据的第0列对应word_data层的特征。
+
+- 可以使用event_handler回调函数来观察训练过程,或进行测试等。这里我们打印了训练过程的cost,该回调函数是trainer.train函数里设定。
+
+- 通过trainer.train函数训练
+
+```python
+def train(use_cuda, save_dirname=None, is_local=True):
+# define network topology
+
+# 句子序列
+word = fluid.layers.data(
+name='word_data', shape=[1], dtype='int64', lod_level=1)
+
+# 谓词
+predicate = fluid.layers.data(
+name='verb_data', shape=[1], dtype='int64', lod_level=1)
+
+# 谓词上下文5个特征
+ctx_n2 = fluid.layers.data(
+name='ctx_n2_data', shape=[1], dtype='int64', lod_level=1)
+ctx_n1 = fluid.layers.data(
+name='ctx_n1_data', shape=[1], dtype='int64', lod_level=1)
+ctx_0 = fluid.layers.data(
+name='ctx_0_data', shape=[1], dtype='int64', lod_level=1)
+ctx_p1 = fluid.layers.data(
+name='ctx_p1_data', shape=[1], dtype='int64', lod_level=1)
+ctx_p2 = fluid.layers.data(
+name='ctx_p2_data', shape=[1], dtype='int64', lod_level=1)
+
+# 谓词上下区域标志
+mark = fluid.layers.data(
+name='mark_data', shape=[1], dtype='int64', lod_level=1)
+
+# define network topology
+feature_out = db_lstm(**locals())
+
+# 标注序列
+target = fluid.layers.data(
+name='target', shape=[1], dtype='int64', lod_level=1)
+
+# 学习 CRF 的转移特征
+crf_cost = fluid.layers.linear_chain_crf(
+input=feature_out,
+label=target,
+param_attr=fluid.ParamAttr(
+name='crfw', learning_rate=mix_hidden_lr))
+
+avg_cost = fluid.layers.mean(crf_cost)
+
+sgd_optimizer = fluid.optimizer.SGD(
+learning_rate=fluid.layers.exponential_decay(
+learning_rate=0.01,
+decay_steps=100000,
+decay_rate=0.5,
+staircase=True))
+
+sgd_optimizer.minimize(avg_cost)
+
+# The CRF decoding layer is used for evaluation and inference.
+# It shares weights with CRF layer. The sharing of parameters among multiple layers
+# is specified by using the same parameter name in these layers. If true tag sequence
+# is provided in training process, `fluid.layers.crf_decoding` calculates labelling error
+# for each input token and sums the error over the entire sequence.
+# Otherwise, `fluid.layers.crf_decoding` generates the labelling tags.
+crf_decode = fluid.layers.crf_decoding(
+input=feature_out, param_attr=fluid.ParamAttr(name='crfw'))
+
+train_data = paddle.batch(
+paddle.reader.shuffle(
+paddle.dataset.conll05.test(), buf_size=8192),
+batch_size=BATCH_SIZE)
+
+place = fluid.CUDAPlace(0) if use_cuda else fluid.CPUPlace()
+
+
+feeder = fluid.DataFeeder(
+feed_list=[
+word, ctx_n2, ctx_n1, ctx_0, ctx_p1, ctx_p2, predicate, mark, target
+],
+place=place)
+exe = fluid.Executor(place)
+
+def train_loop(main_program):
+exe.run(fluid.default_startup_program())
+embedding_param = fluid.global_scope().find_var(
+embedding_name).get_tensor()
+embedding_param.set(
+load_parameter(conll05.get_embedding(), word_dict_len, word_dim),
+place)
+
+start_time = time.time()
+batch_id = 0
+for pass_id in xrange(PASS_NUM):
+for data in train_data():
+cost = exe.run(main_program,
+feed=feeder.feed(data),
+fetch_list=[avg_cost])
+cost = cost[0]
+
+if batch_id % 10 == 0:
+print("avg_cost:" + str(cost))
+if batch_id != 0:
+print("second per batch: " + str((time.time(
+) - start_time) / batch_id))
+# Set the threshold low to speed up the CI test
+if float(cost) < 60.0:
+if save_dirname is not None:
+fluid.io.save_inference_model(save_dirname, [
+'word_data', 'verb_data', 'ctx_n2_data',
+'ctx_n1_data', 'ctx_0_data', 'ctx_p1_data',
+'ctx_p2_data', 'mark_data'
+], [feature_out], exe)
+return
+
+batch_id = batch_id + 1
+
+train_loop(fluid.default_main_program())
+```
+
+
+## 应用模型
+
+训练完成之后,需要依据某个我们关心的性能指标选择最优的模型进行预测,可以简单的选择测试集上标记错误最少的那个模型。以下我们给出一个使用训练后的模型进行预测的示例。
+
+```python
+def infer(use_cuda, save_dirname=None):
+if save_dirname is None:
+return
+
+place = fluid.CUDAPlace(0) if use_cuda else fluid.CPUPlace()
+exe = fluid.Executor(place)
+
+inference_scope = fluid.core.Scope()
+with fluid.scope_guard(inference_scope):
+# Use fluid.io.load_inference_model to obtain the inference program desc,
+# the feed_target_names (the names of variables that will be fed
+# data using feed operators), and the fetch_targets (variables that
+# we want to obtain data from using fetch operators).
+[inference_program, feed_target_names,
+fetch_targets] = fluid.io.load_inference_model(save_dirname, exe)
+
+# Setup inputs by creating LoDTensors to represent sequences of words.
+# Here each word is the basic element of these LoDTensors and the shape of
+# each word (base_shape) should be [1] since it is simply an index to
+# look up for the corresponding word vector.
+# Suppose the length_based level of detail (lod) info is set to [[3, 4, 2]],
+# which has only one lod level. Then the created LoDTensors will have only
+# one higher level structure (sequence of words, or sentence) than the basic
+# element (word). Hence the LoDTensor will hold data for three sentences of
+# length 3, 4 and 2, respectively.
+# Note that lod info should be a list of lists.
+lod = [[3, 4, 2]]
+base_shape = [1]
+# The range of random integers is [low, high]
+word = fluid.create_random_int_lodtensor(
+lod, base_shape, place, low=0, high=word_dict_len - 1)
+pred = fluid.create_random_int_lodtensor(
+lod, base_shape, place, low=0, high=pred_dict_len - 1)
+ctx_n2 = fluid.create_random_int_lodtensor(
+lod, base_shape, place, low=0, high=word_dict_len - 1)
+ctx_n1 = fluid.create_random_int_lodtensor(
+lod, base_shape, place, low=0, high=word_dict_len - 1)
+ctx_0 = fluid.create_random_int_lodtensor(
+lod, base_shape, place, low=0, high=word_dict_len - 1)
+ctx_p1 = fluid.create_random_int_lodtensor(
+lod, base_shape, place, low=0, high=word_dict_len - 1)
+ctx_p2 = fluid.create_random_int_lodtensor(
+lod, base_shape, place, low=0, high=word_dict_len - 1)
+mark = fluid.create_random_int_lodtensor(
+lod, base_shape, place, low=0, high=mark_dict_len - 1)
+
+# Construct feed as a dictionary of {feed_target_name: feed_target_data}
+# and results will contain a list of data corresponding to fetch_targets.
+assert feed_target_names[0] == 'word_data'
+assert feed_target_names[1] == 'verb_data'
+assert feed_target_names[2] == 'ctx_n2_data'
+assert feed_target_names[3] == 'ctx_n1_data'
+assert feed_target_names[4] == 'ctx_0_data'
+assert feed_target_names[5] == 'ctx_p1_data'
+assert feed_target_names[6] == 'ctx_p2_data'
+assert feed_target_names[7] == 'mark_data'
+
+results = exe.run(inference_program,
+feed={
+feed_target_names[0]: word,
+feed_target_names[1]: pred,
+feed_target_names[2]: ctx_n2,
+feed_target_names[3]: ctx_n1,
+feed_target_names[4]: ctx_0,
+feed_target_names[5]: ctx_p1,
+feed_target_names[6]: ctx_p2,
+feed_target_names[7]: mark
+},
+fetch_list=fetch_targets,
+return_numpy=False)
+print(results[0].lod())
+np_data = np.array(results[0])
+print("Inference Shape: ", np_data.shape)
+```
+
+整个程序的入口如下:
+
+```python
+def main(use_cuda, is_local=True):
+if use_cuda and not fluid.core.is_compiled_with_cuda():
+return
+
+# Directory for saving the trained model
+save_dirname = "label_semantic_roles.inference.model"
+
+train(use_cuda, save_dirname, is_local)
+infer(use_cuda, save_dirname)
+
+
+main(use_cuda=False)
+```
+
+## 总结
+
+语义角色标注是许多自然语言理解任务的重要中间步骤。这篇教程中我们以语义角色标注任务为例,介绍如何利用PaddlePaddle进行序列标注任务。教程中所介绍的模型来自我们发表的论文\[[10](#参考文献)\]。由于 CoNLL 2005 SRL任务的训练数据目前并非完全开放,教程中只使用测试数据作为示例。在这个过程中,我们希望减少对其它自然语言处理工具的依赖,利用神经网络数据驱动、端到端学习的能力,得到一个和传统方法可比、甚至更好的模型。在论文中我们证实了这种可能性。关于模型更多的信息和讨论可以在论文中找到。
+
+## 参考文献
+1. Sun W, Sui Z, Wang M, et al. [Chinese semantic role labeling with shallow parsing](http://www.aclweb.org/anthology/D09-1#page=1513)[C]//Proceedings of the 2009 Conference on Empirical Methods in Natural Language Processing: Volume 3-Volume 3. Association for Computational Linguistics, 2009: 1475-1483.
+2. Pascanu R, Gulcehre C, Cho K, et al. [How to construct deep recurrent neural networks](https://arxiv.org/abs/1312.6026)[J]. arXiv preprint arXiv:1312.6026, 2013.
+3. Cho K, Van Merriënboer B, Gulcehre C, et al. [Learning phrase representations using RNN encoder-decoder for statistical machine translation](https://arxiv.org/abs/1406.1078)[J]. arXiv preprint arXiv:1406.1078, 2014.
+4. Bahdanau D, Cho K, Bengio Y. [Neural machine translation by jointly learning to align and translate](https://arxiv.org/abs/1409.0473)[J]. arXiv preprint arXiv:1409.0473, 2014.
+5. Lafferty J, McCallum A, Pereira F. [Conditional random fields: Probabilistic models for segmenting and labeling sequence data](http://www.jmlr.org/papers/volume15/doppa14a/source/biblio.bib.old)[C]//Proceedings of the eighteenth international conference on machine learning, ICML. 2001, 1: 282-289.
+6. 李航. 统计学习方法[J]. 清华大学出版社, 北京, 2012.
+7. Marcus M P, Marcinkiewicz M A, Santorini B. [Building a large annotated corpus of English: The Penn Treebank](http://repository.upenn.edu/cgi/viewcontent.cgi?article=1246&context=cis_reports)[J]. Computational linguistics, 1993, 19(2): 313-330.
+8. Palmer M, Gildea D, Kingsbury P. [The proposition bank: An annotated corpus of semantic roles](http://www.mitpressjournals.org/doi/pdfplus/10.1162/0891201053630264)[J]. Computational linguistics, 2005, 31(1): 71-106.
+9. Carreras X, Màrquez L. [Introduction to the CoNLL-2005 shared task: Semantic role labeling](http://www.cs.upc.edu/~srlconll/st05/papers/intro.pdf)[C]//Proceedings of the Ninth Conference on Computational Natural Language Learning. Association for Computational Linguistics, 2005: 152-164.
+10. Zhou J, Xu W. [End-to-end learning of semantic role labeling using recurrent neural networks](http://www.aclweb.org/anthology/P/P15/P15-1109.pdf)[C]//Proceedings of the Annual Meeting of the Association for Computational Linguistics. 2015.
+
+
+
本教程 由 PaddlePaddle 创作,采用 知识共享 署名-相同方式共享 4.0 国际 许可协议进行许可。
diff --git a/doc/fluid/new_docs/beginners_guide/basics/learning_materials.md b/doc/fluid/new_docs/beginners_guide/basics/learning_materials.md
new file mode 100644
index 0000000000000000000000000000000000000000..a27499c6ed8d1149c6d519006086febbcae943fa
--- /dev/null
+++ b/doc/fluid/new_docs/beginners_guide/basics/learning_materials.md
@@ -0,0 +1,54 @@
+# 学习资料
+
+## 要读的第一本书
+基础理论习得的最直接来源就是书本。按机器学习理论、深度学习理论、编程语言三方面划分,这里推荐如下书籍辅助您。
+
+
+### 机器学习理论
+
+在开启深度学习之前,您需要先行掌握机器学习的理论。深度学习是机器学习中的一个分支,两者内在的理论基础存在强关联。
+机器学习理论的书籍教材比较多,这里推荐一本易懂易学的书籍,可以重点关注神经网络部分。
+
+书名:《机器学习》(周志华著,清华大学出版社,2016年版)
+
+### 深度学习理论
+
+打好机器学习的理论功底后,您可以开始钻研深度学习的理论。通常深度学习理论会给人留下抽象难懂的印象,且和数学结合紧密。
+为了让您能够顺利入门,这里推荐一份易学易用的教材,无论深度学习理论还是数学理论即可一本搞定。
+
+书名:《Deep Learning(深度学习)》(Goodfellow, Bengio, Courville合著,赵申剑、黎彧君、符天凡和李凯合译,人民邮电出版社,2017年版)
+此书电子版在Github上已经开源,详情可参考此链接 [《深度学习》](https://github.com/exacity/deeplearningbook-chinese)
+
+### 编程语言
+
+Python方向:这里推荐您学习Python,一方面各大主流深度学习框架的主力支撑编程语言均为Python;另一方面,对比其他语言,Python较为简单易学。
+Python的教材种类较多,这里推荐一本实操和理论性都兼顾的教材,只要完成书中52个习题,跑代码然后发现问题解决,就能逐步上手。
+
+书名:《“笨办法”学Python》(Zed Shaw著,王巍巍译,人民邮电出版社,2014年11月版)
+
+
+C++方向:C++语言在底层框架中使用较多,您逐步掌握开源框架的基本操作后,在更高阶的框架应用中会用到这个技能点。
+同前面提到的Python一样,学习C++时需要多上手操作。这里推荐迅速上手C++的书籍,不但能够学习功能和结构,还提供了解决方案的示例。
+
+书名:《Essential C++》【美】李普曼(Lippman,S.B.)著,侯捷译,电子工业出版社2013年8月版
+
+
+
+## 要看的视频公开课
+
+在学习一门新技术的同时,除了看书,如果有老师面对面教授,可以更快更好的学会知识。相比于线下授课,视频公开课能够在省钱省力的同时,达到易学易掌握的效果。
+目前深度学习的课程多是公开免费的,通过学习您可以更轻松的理解深度学习中的抽象理论,并在实操方面不绕弯路。
+综合课程生动性、可操作性、紧凑性、连续性这些特点,这里推荐如下课程,同步附上网址,便于您查找学习。
+
+### 理论知识详解视频课
+[机器学习](http://open.163.com/special/opencourse/machinelearning.html) 斯坦福大学教授吴恩达公开课程,包含相关算法的详细讲解。
+
+[AI技术](https://ai.baidu.com/paddlepaddle/player?id=13) 百度推出的“AI核心技术掌握”课程,每节课在20-30分钟左右,从AI技术到深度学习进行全面细致的解读。
+
+[深度学习](http://speech.ee.ntu.edu.tw/~tlkagk/courses_ML17_2.html) 台湾李宏毅教授的在线课程,其中是英文课程,会结合国外的科研成果,但也适合新手入门和理解深度学习。
+
+[编程语言](https://ai.baidu.com/paddlepaddle/openCourses) Python操作课程,从基础到进阶操作都提供详细说明,每节课时长20分钟左右。
+
+### PaddlePaddle实操视频课
+掌握好理论基础,具备编程能力后,您可以开始使用PaddlePaddle Fluid进行实操,从初阶开始学习,向着中高阶努力。
+目前已有PaddlePaddle官方视频公开课在官网呈现,内含PaddlePaddle实战、PaddlePaddle应用场景和机器学习模型讲解课程,帮助开发者从零开始使用PaddlePaddle,从简单场景逐步过渡到工业级应用。[点击这里](http://ai.baidu.com/paddlepaddle/openCourses)您即可开始视频课的学习之旅。
diff --git a/doc/fluid/new_docs/beginners_guide/basics/machine_translation/.gitignore b/doc/fluid/new_docs/beginners_guide/basics/machine_translation/.gitignore
new file mode 100644
index 0000000000000000000000000000000000000000..6129b9e8645010fcb8372d9dc3dbb568dfa80907
--- /dev/null
+++ b/doc/fluid/new_docs/beginners_guide/basics/machine_translation/.gitignore
@@ -0,0 +1,9 @@
+data/wmt14
+data/pre-wmt14
+pretrained/wmt14_model
+gen.log
+gen_result
+train.log
+dataprovider_copy_1.py
+*.pyc
+multi-bleu.perl
diff --git a/doc/fluid/new_docs/beginners_guide/basics/machine_translation/image/bi_rnn.png b/doc/fluid/new_docs/beginners_guide/basics/machine_translation/image/bi_rnn.png
new file mode 100644
index 0000000000000000000000000000000000000000..9d8efd50a49d0305586f550344472ab94c93bed3
Binary files /dev/null and b/doc/fluid/new_docs/beginners_guide/basics/machine_translation/image/bi_rnn.png differ
diff --git a/doc/fluid/new_docs/beginners_guide/basics/machine_translation/image/bi_rnn_en.png b/doc/fluid/new_docs/beginners_guide/basics/machine_translation/image/bi_rnn_en.png
new file mode 100755
index 0000000000000000000000000000000000000000..4b35c88fc8ea2c503473c0c15711744e784d6af6
Binary files /dev/null and b/doc/fluid/new_docs/beginners_guide/basics/machine_translation/image/bi_rnn_en.png differ
diff --git a/doc/fluid/new_docs/beginners_guide/basics/machine_translation/image/decoder_attention.png b/doc/fluid/new_docs/beginners_guide/basics/machine_translation/image/decoder_attention.png
new file mode 100644
index 0000000000000000000000000000000000000000..1b355e7786d25487a3f564af758c2c52c43b4690
Binary files /dev/null and b/doc/fluid/new_docs/beginners_guide/basics/machine_translation/image/decoder_attention.png differ
diff --git a/doc/fluid/new_docs/beginners_guide/basics/machine_translation/image/decoder_attention_en.png b/doc/fluid/new_docs/beginners_guide/basics/machine_translation/image/decoder_attention_en.png
new file mode 100755
index 0000000000000000000000000000000000000000..3728f782ee09d9308d02b42305027b2735467ead
Binary files /dev/null and b/doc/fluid/new_docs/beginners_guide/basics/machine_translation/image/decoder_attention_en.png differ
diff --git a/doc/fluid/new_docs/beginners_guide/basics/machine_translation/image/encoder_attention.png b/doc/fluid/new_docs/beginners_guide/basics/machine_translation/image/encoder_attention.png
new file mode 100644
index 0000000000000000000000000000000000000000..28d7a15a3bd65262bde22a3f41b5aa78b46b368a
Binary files /dev/null and b/doc/fluid/new_docs/beginners_guide/basics/machine_translation/image/encoder_attention.png differ
diff --git a/doc/fluid/new_docs/beginners_guide/basics/machine_translation/image/encoder_attention_en.png b/doc/fluid/new_docs/beginners_guide/basics/machine_translation/image/encoder_attention_en.png
new file mode 100755
index 0000000000000000000000000000000000000000..ea8585565da1ecaf241654c278c6f9b15e283286
Binary files /dev/null and b/doc/fluid/new_docs/beginners_guide/basics/machine_translation/image/encoder_attention_en.png differ
diff --git a/doc/fluid/new_docs/beginners_guide/basics/machine_translation/image/encoder_decoder.png b/doc/fluid/new_docs/beginners_guide/basics/machine_translation/image/encoder_decoder.png
new file mode 100755
index 0000000000000000000000000000000000000000..60aee0017de73f462e35708b1055aff8992c03e1
Binary files /dev/null and b/doc/fluid/new_docs/beginners_guide/basics/machine_translation/image/encoder_decoder.png differ
diff --git a/doc/fluid/new_docs/beginners_guide/basics/machine_translation/image/encoder_decoder_en.png b/doc/fluid/new_docs/beginners_guide/basics/machine_translation/image/encoder_decoder_en.png
new file mode 100755
index 0000000000000000000000000000000000000000..6b73798fe632e0873b35c117b86f347c8cf3116a
Binary files /dev/null and b/doc/fluid/new_docs/beginners_guide/basics/machine_translation/image/encoder_decoder_en.png differ
diff --git a/doc/fluid/new_docs/beginners_guide/basics/machine_translation/image/gru.png b/doc/fluid/new_docs/beginners_guide/basics/machine_translation/image/gru.png
new file mode 100644
index 0000000000000000000000000000000000000000..0cde685b84106650a4df18ce335a23e6338d3d11
Binary files /dev/null and b/doc/fluid/new_docs/beginners_guide/basics/machine_translation/image/gru.png differ
diff --git a/doc/fluid/new_docs/beginners_guide/basics/machine_translation/image/gru_en.png b/doc/fluid/new_docs/beginners_guide/basics/machine_translation/image/gru_en.png
new file mode 100755
index 0000000000000000000000000000000000000000..a6af429f23f0f7e82650139bbd8dcbef27a34abe
Binary files /dev/null and b/doc/fluid/new_docs/beginners_guide/basics/machine_translation/image/gru_en.png differ
diff --git a/doc/fluid/new_docs/beginners_guide/basics/machine_translation/image/nmt.png b/doc/fluid/new_docs/beginners_guide/basics/machine_translation/image/nmt.png
new file mode 100644
index 0000000000000000000000000000000000000000..bf56d73ebf297fadf522389c7b6836dd379aa097
Binary files /dev/null and b/doc/fluid/new_docs/beginners_guide/basics/machine_translation/image/nmt.png differ
diff --git a/doc/fluid/new_docs/beginners_guide/basics/machine_translation/image/nmt_en.png b/doc/fluid/new_docs/beginners_guide/basics/machine_translation/image/nmt_en.png
new file mode 100755
index 0000000000000000000000000000000000000000..557310e044b2b6687e5ea6895417ed946ac7bc11
Binary files /dev/null and b/doc/fluid/new_docs/beginners_guide/basics/machine_translation/image/nmt_en.png differ
diff --git a/doc/fluid/new_docs/beginners_guide/basics/machine_translation/index.md b/doc/fluid/new_docs/beginners_guide/basics/machine_translation/index.md
new file mode 100644
index 0000000000000000000000000000000000000000..fc161aaae9c37b0e1a596204e7138025a98adb1d
--- /dev/null
+++ b/doc/fluid/new_docs/beginners_guide/basics/machine_translation/index.md
@@ -0,0 +1,448 @@
+# 机器翻译
+
+本教程源代码目录在[book/machine_translation](https://github.com/PaddlePaddle/book/tree/develop/08.machine_translation), 初次使用请参考PaddlePaddle[安装教程](https://github.com/PaddlePaddle/book/blob/develop/README.cn.md#运行这本书)。
+
+## 背景介绍
+
+机器翻译(machine translation, MT)是用计算机来实现不同语言之间翻译的技术。被翻译的语言通常称为源语言(source language),翻译成的结果语言称为目标语言(target language)。机器翻译即实现从源语言到目标语言转换的过程,是自然语言处理的重要研究领域之一。
+
+早期机器翻译系统多为基于规则的翻译系统,需要由语言学家编写两种语言之间的转换规则,再将这些规则录入计算机。该方法对语言学家的要求非常高,而且我们几乎无法总结一门语言会用到的所有规则,更何况两种甚至更多的语言。因此,传统机器翻译方法面临的主要挑战是无法得到一个完备的规则集合\[[1](#参考文献)\]。
+
+为解决以上问题,统计机器翻译(Statistical Machine Translation, SMT)技术应运而生。在统计机器翻译技术中,转化规则是由机器自动从大规模的语料中学习得到的,而非我们人主动提供规则。因此,它克服了基于规则的翻译系统所面临的知识获取瓶颈的问题,但仍然存在许多挑战:1)人为设计许多特征(feature),但永远无法覆盖所有的语言现象;2)难以利用全局的特征;3)依赖于许多预处理环节,如词语对齐、分词或符号化(tokenization)、规则抽取、句法分析等,而每个环节的错误会逐步累积,对翻译的影响也越来越大。
+
+近年来,深度学习技术的发展为解决上述挑战提供了新的思路。将深度学习应用于机器翻译任务的方法大致分为两类:1)仍以统计机器翻译系统为框架,只是利用神经网络来改进其中的关键模块,如语言模型、调序模型等(见图1的左半部分);2)不再以统计机器翻译系统为框架,而是直接用神经网络将源语言映射到目标语言,即端到端的神经网络机器翻译(End-to-End Neural Machine Translation, End-to-End NMT)(见图1的右半部分),简称为NMT模型。
+
+
+图1. 基于神经网络的机器翻译系统
+
+
+本教程主要介绍NMT模型,以及如何用PaddlePaddle来训练一个NMT模型。
+
+## 效果展示
+
+以中英翻译(中文翻译到英文)的模型为例,当模型训练完毕时,如果输入如下已分词的中文句子:
+```text
+这些 是 希望 的 曙光 和 解脱 的 迹象 .
+```
+如果设定显示翻译结果的条数(即[柱搜索算法](#柱搜索算法)的宽度)为3,生成的英语句子如下:
+```text
+0 -5.36816 These are signs of hope and relief .
+1 -6.23177 These are the light of hope and relief .
+2 -7.7914 These are the light of hope and the relief of hope .
+```
+- 左起第一列是生成句子的序号;左起第二列是该条句子的得分(从大到小),分值越高越好;左起第三列是生成的英语句子。
+- 另外有两个特殊标志:``表示句子的结尾,``表示未登录词(unknown word),即未在训练字典中出现的词。
+
+## 模型概览
+
+本节依次介绍双向循环神经网络(Bi-directional Recurrent Neural Network),NMT模型中典型的编码器-解码器(Encoder-Decoder)框架以及柱搜索(beam search)算法。
+
+### 双向循环神经网络
+
+我们已经在[语义角色标注](https://github.com/PaddlePaddle/book/blob/develop/07.label_semantic_roles/README.cn.md)一章中介绍了一种双向循环神经网络,这里介绍Bengio团队在论文\[[2](#参考文献),[4](#参考文献)\]中提出的另一种结构。该结构的目的是输入一个序列,得到其在每个时刻的特征表示,即输出的每个时刻都用定长向量表示到该时刻的上下文语义信息。
+
+具体来说,该双向循环神经网络分别在时间维以顺序和逆序——即前向(forward)和后向(backward)——依次处理输入序列,并将每个时间步RNN的输出拼接成为最终的输出层。这样每个时间步的输出节点,都包含了输入序列中当前时刻完整的过去和未来的上下文信息。下图展示的是一个按时间步展开的双向循环神经网络。该网络包含一个前向和一个后向RNN,其中有六个权重矩阵:输入到前向隐层和后向隐层的权重矩阵(`$W_1, W_3$`),隐层到隐层自己的权重矩阵(`$W_2,W_5$`),前向隐层和后向隐层到输出层的权重矩阵(`$W_4, W_6$`)。注意,该网络的前向隐层和后向隐层之间没有连接。
+
+
+
+图3. 按时间步展开的双向循环神经网络
+
+
+### 编码器-解码器框架
+
+编码器-解码器(Encoder-Decoder)\[[2](#参考文献)\]框架用于解决由一个任意长度的源序列到另一个任意长度的目标序列的变换问题。即编码阶段将整个源序列编码成一个向量,解码阶段通过最大化预测序列概率,从中解码出整个目标序列。编码和解码的过程通常都使用RNN实现。
+
+
+图4. 编码器-解码器框架
+
+
+#### 编码器
+
+编码阶段分为三步:
+
+1. one-hot vector表示:将源语言句子`$x=\left \{ x_1,x_2,...,x_T \right \}$`的每个词`$x_i$`表示成一个列向量`$w_i\epsilon \left \{ 0,1 \right \}^{\left | V \right |},i=1,2,...,T$`。这个向量`$w_i$`的维度与词汇表大小`$\left | V \right |$` 相同,并且只有一个维度上有值1(该位置对应该词在词汇表中的位置),其余全是0。
+
+2. 映射到低维语义空间的词向量:one-hot vector表示存在两个问题,1)生成的向量维度往往很大,容易造成维数灾难;2)难以刻画词与词之间的关系(如语义相似性,也就是无法很好地表达语义)。因此,需再one-hot vector映射到低维的语义空间,由一个固定维度的稠密向量(称为词向量)表示。记映射矩阵为`$C\epsilon R^{K\times \left | V \right |}$`,用`$s_i=Cw_i$`表示第`$i$`个词的词向量,`$K$`为向量维度。
+
+3. 用RNN编码源语言词序列:这一过程的计算公式为`$h_i=\varnothing _\theta \left ( h_{i-1}, s_i \right )$`,其中`$h_0$`是一个全零的向量,`$\varnothing _\theta$`是一个非线性激活函数,最后得到的`$\mathbf{h}=\left \{ h_1,..., h_T \right \}$`就是RNN依次读入源语言`$T$`个词的状态编码序列。整句话的向量表示可以采用`$\mathbf{h}$`在最后一个时间步`$T$`的状态编码,或使用时间维上的池化(pooling)结果。
+
+第3步也可以使用双向循环神经网络实现更复杂的句编码表示,具体可以用双向GRU实现。前向GRU按照词序列`$(x_1,x_2,...,x_T)$`的顺序依次编码源语言端词,并得到一系列隐层状态`$(\overrightarrow{h_1},\overrightarrow{h_2},...,\overrightarrow{h_T})$`。类似的,后向GRU按照`$(x_T,x_{T-1},...,x_1)$`的顺序依次编码源语言端词,得到`$(\overleftarrow{h_1},\overleftarrow{h_2},...,\overleftarrow{h_T})$`。最后对于词`$x_i$`,通过拼接两个GRU的结果得到它的隐层状态,即`$h_i=\left [ \overrightarrow{h_i^T},\overleftarrow{h_i^T} \right ]^{T}$`。
+
+
+
+图5. 使用双向GRU的编码器
+
+
+#### 解码器
+
+机器翻译任务的训练过程中,解码阶段的目标是最大化下一个正确的目标语言词的概率。思路是:
+
+1. 每一个时刻,根据源语言句子的编码信息(又叫上下文向量,context vector)`$c$`、真实目标语言序列的第`$i$`个词`$u_i$`和`$i$`时刻RNN的隐层状态`$z_i$`,计算出下一个隐层状态`$z_{i+1}$`。计算公式如下:
+
+$$z_{i+1}=\phi _{\theta '}\left ( c,u_i,z_i \right )$$
+
+其中`$\phi _{\theta '}$`是一个非线性激活函数;`$c=q\mathbf{h}$`是源语言句子的上下文向量,在不使用[注意力机制](#注意力机制)时,如果[编码器](#编码器)的输出是源语言句子编码后的最后一个元素,则可以定义`$c=h_T$`;`$u_i$`是目标语言序列的第`$i$`个单词,`$u_0$`是目标语言序列的开始标记``,表示解码开始;`$z_i$`是`$i$`时刻解码RNN的隐层状态,`$z_0$`是一个全零的向量。
+
+2. 将`$z_{i+1}$`通过`softmax`归一化,得到目标语言序列的第`$i+1$`个单词的概率分布`$p_{i+1}$`。概率分布公式如下:
+
+$$p\left ( u_{i+1}|u_{<i+1},\mathbf{x} \right )=softmax(W_sz_{i+1}+b_z)$$
+
+其中`$W_sz_{i+1}+b_z$`是对每个可能的输出单词进行打分,再用softmax归一化就可以得到第`$i+1$`个词的概率`$p_{i+1}$`。
+
+3. 根据`$p_{i+1}$`和`$u_{i+1}$`计算代价。
+4. 重复步骤1~3,直到目标语言序列中的所有词处理完毕。
+
+机器翻译任务的生成过程,通俗来讲就是根据预先训练的模型来翻译源语言句子。生成过程中的解码阶段和上述训练过程的有所差异,具体介绍请见[柱搜索算法](#柱搜索算法)。
+
+### 柱搜索算法
+
+柱搜索([beam search](http://en.wikipedia.org/wiki/Beam_search))是一种启发式图搜索算法,用于在图或树中搜索有限集合中的最优扩展节点,通常用在解空间非常大的系统(如机器翻译、语音识别)中,原因是内存无法装下图或树中所有展开的解。如在机器翻译任务中希望翻译“`你好`”,就算目标语言字典中只有3个词(``, ``, `hello`),也可能生成无限句话(`hello`循环出现的次数不定),为了找到其中较好的翻译结果,我们可采用柱搜索算法。
+
+柱搜索算法使用广度优先策略建立搜索树,在树的每一层,按照启发代价(heuristic cost)(本教程中,为生成词的log概率之和)对节点进行排序,然后仅留下预先确定的个数(文献中通常称为beam width、beam size、柱宽度等)的节点。只有这些节点会在下一层继续扩展,其他节点就被剪掉了,也就是说保留了质量较高的节点,剪枝了质量较差的节点。因此,搜索所占用的空间和时间大幅减少,但缺点是无法保证一定获得最优解。
+
+使用柱搜索算法的解码阶段,目标是最大化生成序列的概率。思路是:
+
+1. 每一个时刻,根据源语言句子的编码信息`$c$`、生成的第`$i$`个目标语言序列单词`$u_i$`和`$i$`时刻RNN的隐层状态`$z_i$`,计算出下一个隐层状态`$z_{i+1}$`。
+2. 将`$z_{i+1}$`通过`softmax`归一化,得到目标语言序列的第`$i+1$`个单词的概率分布`$p_{i+1}$`。
+3. 根据`$p_{i+1}$`采样出单词`$u_{i+1}$`。
+4. 重复步骤1~3,直到获得句子结束标记``或超过句子的最大生成长度为止。
+
+注意:`$z_{i+1}$`和`$p_{i+1}$`的计算公式同[解码器](#解码器)中的一样。且由于生成时的每一步都是通过贪心法实现的,因此并不能保证得到全局最优解。
+
+## 数据介绍
+
+本教程使用[WMT-14](http://www-lium.univ-lemans.fr/~schwenk/cslm_joint_paper/)数据集中的[bitexts(after selection)](http://www-lium.univ-lemans.fr/~schwenk/cslm_joint_paper/data/bitexts.tgz)作为训练集,[dev+test data](http://www-lium.univ-lemans.fr/~schwenk/cslm_joint_paper/data/dev+test.tgz)作为测试集和生成集。
+
+### 数据预处理
+
+我们的预处理流程包括两步:
+- 将每个源语言到目标语言的平行语料库文件合并为一个文件:
+- 合并每个`XXX.src`和`XXX.trg`文件为`XXX`。
+- `XXX`中的第`$i$`行内容为`XXX.src`中的第`$i$`行和`XXX.trg`中的第`$i$`行连接,用'\t'分隔。
+- 创建训练数据的“源字典”和“目标字典”。每个字典都有**DICTSIZE**个单词,包括:语料中词频最高的(DICTSIZE - 3)个单词,和3个特殊符号``(序列的开始)、``(序列的结束)和``(未登录词)。
+
+### 示例数据
+
+因为完整的数据集数据量较大,为了验证训练流程,PaddlePaddle接口paddle.dataset.wmt14中默认提供了一个经过预处理的[较小规模的数据集](http://paddlepaddle.bj.bcebos.com/demo/wmt_shrinked_data/wmt14.tgz)。
+
+该数据集有193319条训练数据,6003条测试数据,词典长度为30000。因为数据规模限制,使用该数据集训练出来的模型效果无法保证。
+
+## 模型配置说明
+
+下面我们开始根据输入数据的形式配置模型。首先引入所需的库函数以及定义全局变量。
+
+```python
+import contextlib
+
+import numpy as np
+import paddle
+import paddle.fluid as fluid
+import paddle.fluid.framework as framework
+import paddle.fluid.layers as pd
+from paddle.fluid.executor import Executor
+from functools import partial
+import os
+
+dict_size = 30000
+source_dict_dim = target_dict_dim = dict_size
+hidden_dim = 32
+word_dim = 16
+batch_size = 2
+max_length = 8
+topk_size = 50
+beam_size = 2
+
+decoder_size = hidden_dim
+```
+
+然后如下实现编码器框架:
+
+```python
+def encoder(is_sparse):
+src_word_id = pd.data(
+name="src_word_id", shape=[1], dtype='int64', lod_level=1)
+src_embedding = pd.embedding(
+input=src_word_id,
+size=[dict_size, word_dim],
+dtype='float32',
+is_sparse=is_sparse,
+param_attr=fluid.ParamAttr(name='vemb'))
+
+fc1 = pd.fc(input=src_embedding, size=hidden_dim * 4, act='tanh')
+lstm_hidden0, lstm_0 = pd.dynamic_lstm(input=fc1, size=hidden_dim * 4)
+encoder_out = pd.sequence_last_step(input=lstm_hidden0)
+return encoder_out
+```
+
+再实现训练模式下的解码器:
+
+```python
+def train_decoder(context, is_sparse):
+trg_language_word = pd.data(
+name="target_language_word", shape=[1], dtype='int64', lod_level=1)
+trg_embedding = pd.embedding(
+input=trg_language_word,
+size=[dict_size, word_dim],
+dtype='float32',
+is_sparse=is_sparse,
+param_attr=fluid.ParamAttr(name='vemb'))
+
+rnn = pd.DynamicRNN()
+with rnn.block():
+current_word = rnn.step_input(trg_embedding)
+pre_state = rnn.memory(init=context)
+current_state = pd.fc(input=[current_word, pre_state],
+size=decoder_size,
+act='tanh')
+
+current_score = pd.fc(input=current_state,
+size=target_dict_dim,
+act='softmax')
+rnn.update_memory(pre_state, current_state)
+rnn.output(current_score)
+
+return rnn()
+```
+
+实现推测模式下的解码器:
+
+```python
+def decode(context, is_sparse):
+init_state = context
+array_len = pd.fill_constant(shape=[1], dtype='int64', value=max_length)
+counter = pd.zeros(shape=[1], dtype='int64', force_cpu=True)
+
+# fill the first element with init_state
+state_array = pd.create_array('float32')
+pd.array_write(init_state, array=state_array, i=counter)
+
+# ids, scores as memory
+ids_array = pd.create_array('int64')
+scores_array = pd.create_array('float32')
+
+init_ids = pd.data(name="init_ids", shape=[1], dtype="int64", lod_level=2)
+init_scores = pd.data(
+name="init_scores", shape=[1], dtype="float32", lod_level=2)
+
+pd.array_write(init_ids, array=ids_array, i=counter)
+pd.array_write(init_scores, array=scores_array, i=counter)
+
+cond = pd.less_than(x=counter, y=array_len)
+
+while_op = pd.While(cond=cond)
+with while_op.block():
+pre_ids = pd.array_read(array=ids_array, i=counter)
+pre_state = pd.array_read(array=state_array, i=counter)
+pre_score = pd.array_read(array=scores_array, i=counter)
+
+# expand the lod of pre_state to be the same with pre_score
+pre_state_expanded = pd.sequence_expand(pre_state, pre_score)
+
+pre_ids_emb = pd.embedding(
+input=pre_ids,
+size=[dict_size, word_dim],
+dtype='float32',
+is_sparse=is_sparse)
+
+# use rnn unit to update rnn
+current_state = pd.fc(input=[pre_state_expanded, pre_ids_emb],
+size=decoder_size,
+act='tanh')
+current_state_with_lod = pd.lod_reset(x=current_state, y=pre_score)
+# use score to do beam search
+current_score = pd.fc(input=current_state_with_lod,
+size=target_dict_dim,
+act='softmax')
+topk_scores, topk_indices = pd.topk(current_score, k=topk_size)
+selected_ids, selected_scores = pd.beam_search(
+pre_ids, topk_indices, topk_scores, beam_size, end_id=10, level=0)
+
+pd.increment(x=counter, value=1, in_place=True)
+
+# update the memories
+pd.array_write(current_state, array=state_array, i=counter)
+pd.array_write(selected_ids, array=ids_array, i=counter)
+pd.array_write(selected_scores, array=scores_array, i=counter)
+
+pd.less_than(x=counter, y=array_len, cond=cond)
+
+translation_ids, translation_scores = pd.beam_search_decode(
+ids=ids_array, scores=scores_array)
+
+return translation_ids, translation_scores
+```
+
+进而,我们定义一个`train_program`来使用`inference_program`计算出的结果,在标记数据的帮助下来计算误差。我们还定义了一个`optimizer_func`来定义优化器。
+
+```python
+def train_program(is_sparse):
+context = encoder(is_sparse)
+rnn_out = train_decoder(context, is_sparse)
+label = pd.data(
+name="target_language_next_word", shape=[1], dtype='int64', lod_level=1)
+cost = pd.cross_entropy(input=rnn_out, label=label)
+avg_cost = pd.mean(cost)
+return avg_cost
+
+
+def optimizer_func():
+return fluid.optimizer.Adagrad(
+learning_rate=1e-4,
+regularization=fluid.regularizer.L2DecayRegularizer(
+regularization_coeff=0.1))
+```
+
+## 训练模型
+
+### 定义训练环境
+定义您的训练环境,可以指定训练是发生在CPU还是GPU上。
+
+```python
+use_cuda = False
+place = fluid.CUDAPlace(0) if use_cuda else fluid.CPUPlace()
+```
+
+### 定义数据提供器
+下一步是为训练和测试定义数据提供器。提供器读入一个大小为 `BATCH_SIZE`的数据。`paddle.dataset.wmt.train` 每次会在乱序化后提供一个大小为`BATCH_SIZE`的数据,乱序化的大小为缓存大小`buf_size`。
+
+```python
+train_reader = paddle.batch(
+paddle.reader.shuffle(
+paddle.dataset.wmt14.train(dict_size), buf_size=1000),
+batch_size=batch_size)
+```
+
+### 构造训练器(trainer)
+训练器需要一个训练程序和一个训练优化函数。
+
+```python
+is_sparse = False
+trainer = fluid.Trainer(
+train_func=partial(train_program, is_sparse),
+place=place,
+optimizer_func=optimizer_func)
+```
+
+### 提供数据
+
+`feed_order`用来定义每条产生的数据和`paddle.layer.data`之间的映射关系。比如,`wmt14.train`产生的第一列的数据对应的是`src_word_id`这个特征。
+
+```python
+feed_order = [
+'src_word_id', 'target_language_word', 'target_language_next_word'
+]
+```
+
+### 事件处理器
+回调函数`event_handler`在一个之前定义好的事件发生后会被调用。例如,我们可以在每步训练结束后查看误差。
+
+```python
+def event_handler(event):
+if isinstance(event, fluid.EndStepEvent):
+if event.step % 10 == 0:
+print('pass_id=' + str(event.epoch) + ' batch=' + str(event.step))
+
+if event.step == 20:
+trainer.stop()
+```
+
+### 开始训练
+最后,我们传入训练循环数(`num_epoch`)和一些别的参数,调用 `trainer.train` 来开始训练。
+
+```python
+EPOCH_NUM = 1
+
+trainer.train(
+reader=train_reader,
+num_epochs=EPOCH_NUM,
+event_handler=event_handler,
+feed_order=feed_order)
+```
+
+## 应用模型
+
+### 定义解码部分
+
+使用上面定义的 `encoder` 和 `decoder` 函数来推测翻译后的对应id和分数.
+
+```python
+context = encoder(is_sparse)
+translation_ids, translation_scores = decode(context, is_sparse)
+```
+
+### 定义数据
+
+我们先初始化id和分数来生成tensors来作为输入数据。在这个预测例子中,我们用`wmt14.test`数据中的第一个记录来做推测,最后我们用"源字典"和"目标字典"来列印对应的句子结果。
+
+```python
+init_ids_data = np.array([1 for _ in range(batch_size)], dtype='int64')
+init_scores_data = np.array(
+[1. for _ in range(batch_size)], dtype='float32')
+init_ids_data = init_ids_data.reshape((batch_size, 1))
+init_scores_data = init_scores_data.reshape((batch_size, 1))
+init_lod = [1] * batch_size
+init_lod = [init_lod, init_lod]
+
+init_ids = fluid.create_lod_tensor(init_ids_data, init_lod, place)
+init_scores = fluid.create_lod_tensor(init_scores_data, init_lod, place)
+
+test_data = paddle.batch(
+paddle.reader.shuffle(
+paddle.dataset.wmt14.test(dict_size), buf_size=1000),
+batch_size=batch_size)
+
+feed_order = ['src_word_id']
+feed_list = [
+framework.default_main_program().global_block().var(var_name)
+for var_name in feed_order
+]
+feeder = fluid.DataFeeder(feed_list, place)
+
+src_dict, trg_dict = paddle.dataset.wmt14.get_dict(dict_size)
+```
+
+### 测试
+现在我们可以进行预测了。我们要在`feed_order`提供对应参数,放在`executor`上运行以取得id和分数结果
+
+```python
+exe = Executor(place)
+exe.run(framework.default_startup_program())
+
+for data in test_data():
+feed_data = map(lambda x: [x[0]], data)
+feed_dict = feeder.feed(feed_data)
+feed_dict['init_ids'] = init_ids
+feed_dict['init_scores'] = init_scores
+
+results = exe.run(
+framework.default_main_program(),
+feed=feed_dict,
+fetch_list=[translation_ids, translation_scores],
+return_numpy=False)
+
+result_ids = np.array(results[0])
+result_scores = np.array(results[1])
+
+print("Original sentence:")
+print(" ".join([src_dict[w] for w in feed_data[0][0]]))
+print("Translated sentence:")
+print(" ".join([trg_dict[w] for w in result_ids]))
+print("Corresponding score: ", result_scores)
+
+break
+```
+
+## 总结
+
+端到端的神经网络机器翻译是近几年兴起的一种全新的机器翻译方法。本章中,我们介绍了NMT中典型的“编码器-解码器”框架。由于NMT是一个典型的Seq2Seq(Sequence to Sequence,序列到序列)学习问题,因此,Seq2Seq中的query改写(query rewriting)、摘要、单轮对话等问题都可以用本教程的模型来解决。
+
+## 参考文献
+
+1. Koehn P. [Statistical machine translation](https://books.google.com.hk/books?id=4v_Cx1wIMLkC&printsec=frontcover&hl=zh-CN&source=gbs_ge_summary_r&cad=0#v=onepage&q&f=false)[M]. Cambridge University Press, 2009.
+2. Cho K, Van Merriënboer B, Gulcehre C, et al. [Learning phrase representations using RNN encoder-decoder for statistical machine translation](http://www.aclweb.org/anthology/D/D14/D14-1179.pdf)[C]//Proceedings of the 2014 Conference on Empirical Methods in Natural Language Processing (EMNLP), 2014: 1724-1734.
+3. Chung J, Gulcehre C, Cho K H, et al. [Empirical evaluation of gated recurrent neural networks on sequence modeling](https://arxiv.org/abs/1412.3555)[J]. arXiv preprint arXiv:1412.3555, 2014.
+4. Bahdanau D, Cho K, Bengio Y. [Neural machine translation by jointly learning to align and translate](https://arxiv.org/abs/1409.0473)[C]//Proceedings of ICLR 2015, 2015.
+5. Papineni K, Roukos S, Ward T, et al. [BLEU: a method for automatic evaluation of machine translation](http://dl.acm.org/citation.cfm?id=1073135)[C]//Proceedings of the 40th annual meeting on association for computational linguistics. Association for Computational Linguistics, 2002: 311-318.
+
+
+
本教程 由 PaddlePaddle 创作,采用 知识共享 署名-相同方式共享 4.0 国际 许可协议进行许可。
diff --git a/doc/fluid/new_docs/beginners_guide/basics/recommender_system/.gitignore b/doc/fluid/new_docs/beginners_guide/basics/recommender_system/.gitignore
new file mode 100644
index 0000000000000000000000000000000000000000..f23901aeb3a9e7cd12611fc556742670d04a9bb5
--- /dev/null
+++ b/doc/fluid/new_docs/beginners_guide/basics/recommender_system/.gitignore
@@ -0,0 +1,2 @@
+.idea
+.ipynb_checkpoints
diff --git a/doc/fluid/new_docs/beginners_guide/basics/recommender_system/image/Deep_candidate_generation_model_architecture.en.png b/doc/fluid/new_docs/beginners_guide/basics/recommender_system/image/Deep_candidate_generation_model_architecture.en.png
new file mode 100644
index 0000000000000000000000000000000000000000..c213608e769f69fb2cfe8597f8e696ee53730e3d
Binary files /dev/null and b/doc/fluid/new_docs/beginners_guide/basics/recommender_system/image/Deep_candidate_generation_model_architecture.en.png differ
diff --git a/doc/fluid/new_docs/beginners_guide/basics/recommender_system/image/Deep_candidate_generation_model_architecture.png b/doc/fluid/new_docs/beginners_guide/basics/recommender_system/image/Deep_candidate_generation_model_architecture.png
new file mode 100644
index 0000000000000000000000000000000000000000..8aedb2204371e7691140ceffa5992f6080bbf097
Binary files /dev/null and b/doc/fluid/new_docs/beginners_guide/basics/recommender_system/image/Deep_candidate_generation_model_architecture.png differ
diff --git a/doc/fluid/new_docs/beginners_guide/basics/recommender_system/image/YouTube_Overview.en.png b/doc/fluid/new_docs/beginners_guide/basics/recommender_system/image/YouTube_Overview.en.png
new file mode 100644
index 0000000000000000000000000000000000000000..4298567ac5600173343299999965b20612e7affe
Binary files /dev/null and b/doc/fluid/new_docs/beginners_guide/basics/recommender_system/image/YouTube_Overview.en.png differ
diff --git a/doc/fluid/new_docs/beginners_guide/basics/recommender_system/image/YouTube_Overview.png b/doc/fluid/new_docs/beginners_guide/basics/recommender_system/image/YouTube_Overview.png
new file mode 100644
index 0000000000000000000000000000000000000000..a98e7cc67606b31e4c945f7eb907563e46dcef56
Binary files /dev/null and b/doc/fluid/new_docs/beginners_guide/basics/recommender_system/image/YouTube_Overview.png differ
diff --git a/doc/fluid/new_docs/beginners_guide/basics/recommender_system/image/output_32_0.png b/doc/fluid/new_docs/beginners_guide/basics/recommender_system/image/output_32_0.png
new file mode 100644
index 0000000000000000000000000000000000000000..7fd97b9cc3a0b9105b41591af4e8f8e4646bd681
Binary files /dev/null and b/doc/fluid/new_docs/beginners_guide/basics/recommender_system/image/output_32_0.png differ
diff --git a/doc/fluid/new_docs/beginners_guide/basics/recommender_system/image/rec_regression_network.png b/doc/fluid/new_docs/beginners_guide/basics/recommender_system/image/rec_regression_network.png
new file mode 100644
index 0000000000000000000000000000000000000000..90c9b09fb78db98391ee199934f2d16efd6d6652
Binary files /dev/null and b/doc/fluid/new_docs/beginners_guide/basics/recommender_system/image/rec_regression_network.png differ
diff --git a/doc/fluid/new_docs/beginners_guide/basics/recommender_system/image/rec_regression_network_en.png b/doc/fluid/new_docs/beginners_guide/basics/recommender_system/image/rec_regression_network_en.png
new file mode 100755
index 0000000000000000000000000000000000000000..6fc8e11967000ec48c1c0a6fa3c2eaecb80cbb84
Binary files /dev/null and b/doc/fluid/new_docs/beginners_guide/basics/recommender_system/image/rec_regression_network_en.png differ
diff --git a/doc/fluid/new_docs/beginners_guide/basics/recommender_system/image/text_cnn.png b/doc/fluid/new_docs/beginners_guide/basics/recommender_system/image/text_cnn.png
new file mode 100644
index 0000000000000000000000000000000000000000..61e63d9147cbc2901706ef80776d706e5368c3c5
Binary files /dev/null and b/doc/fluid/new_docs/beginners_guide/basics/recommender_system/image/text_cnn.png differ
diff --git a/doc/fluid/new_docs/beginners_guide/basics/recommender_system/image/text_cnn_en.png b/doc/fluid/new_docs/beginners_guide/basics/recommender_system/image/text_cnn_en.png
new file mode 100644
index 0000000000000000000000000000000000000000..fbcae2be81141be955076e877b94b0ea5d7e4d4a
Binary files /dev/null and b/doc/fluid/new_docs/beginners_guide/basics/recommender_system/image/text_cnn_en.png differ
diff --git a/doc/fluid/new_docs/beginners_guide/basics/recommender_system/index.md b/doc/fluid/new_docs/beginners_guide/basics/recommender_system/index.md
new file mode 100644
index 0000000000000000000000000000000000000000..09a07f3dc30abc57ab3731af054dd83491acc9a6
--- /dev/null
+++ b/doc/fluid/new_docs/beginners_guide/basics/recommender_system/index.md
@@ -0,0 +1,528 @@
+# 个性化推荐
+
+本教程源代码目录在[book/recommender_system](https://github.com/PaddlePaddle/book/tree/develop/05.recommender_system), 初次使用请参考PaddlePaddle[安装教程](https://github.com/PaddlePaddle/book/blob/develop/README.cn.md#运行这本书)。
+
+## 背景介绍
+
+在网络技术不断发展和电子商务规模不断扩大的背景下,商品数量和种类快速增长,用户需要花费大量时间才能找到自己想买的商品,这就是信息超载问题。为了解决这个难题,推荐系统(Recommender System)应运而生。
+
+个性化推荐系统是信息过滤系统(Information Filtering System)的子集,它可以用在很多领域,如电影、音乐、电商和 Feed 流推荐等。推荐系统通过分析、挖掘用户行为,发现用户的个性化需求与兴趣特点,将用户可能感兴趣的信息或商品推荐给用户。与搜索引擎不同,推荐系统不需要用户准确地描述出自己的需求,而是根据分析历史行为建模,主动提供满足用户兴趣和需求的信息。
+
+传统的推荐系统方法主要有:
+
+- 协同过滤推荐(Collaborative Filtering Recommendation):该方法收集分析用户历史行为、活动、偏好,计算一个用户与其他用户的相似度,利用目标用户的相似用户对商品评价的加权评价值,来预测目标用户对特定商品的喜好程度。优点是可以给用户推荐未浏览过的新产品;缺点是对于没有任何行为的新用户存在冷启动的问题,同时也存在用户与商品之间的交互数据不够多造成的稀疏问题,会导致模型难以找到相近用户。
+- 基于内容过滤推荐[[1](#参考文献)](Content-based Filtering Recommendation):该方法利用商品的内容描述,抽象出有意义的特征,通过计算用户的兴趣和商品描述之间的相似度,来给用户做推荐。优点是简单直接,不需要依据其他用户对商品的评价,而是通过商品属性进行商品相似度度量,从而推荐给用户所感兴趣商品的相似商品;缺点是对于没有任何行为的新用户同样存在冷启动的问题。
+- 组合推荐[[2](#参考文献)](Hybrid Recommendation):运用不同的输入和技术共同进行推荐,以弥补各自推荐技术的缺点。
+
+其中协同过滤是应用最广泛的技术之一,它又可以分为多个子类:基于用户 (User-Based)的推荐[[3](#参考文献)] 、基于物品(Item-Based)的推荐[[4](#参考文献)]、基于社交网络关系(Social-Based)的推荐[[5](#参考文献)]、基于模型(Model-based)的推荐等。1994年明尼苏达大学推出的GroupLens系统[[3](#参考文献)]一般被认为是推荐系统成为一个相对独立的研究方向的标志。该系统首次提出了基于协同过滤来完成推荐任务的思想,此后,基于该模型的协同过滤推荐引领了推荐系统十几年的发展方向。
+
+深度学习具有优秀的自动提取特征的能力,能够学习多层次的抽象特征表示,并对异质或跨域的内容信息进行学习,可以一定程度上处理推荐系统冷启动问题[[6](#参考文献)]。本教程主要介绍个性化推荐的深度学习模型,以及如何使用PaddlePaddle实现模型。
+
+## 效果展示
+
+我们使用包含用户信息、电影信息与电影评分的数据集作为个性化推荐的应用场景。当我们训练好模型后,只需要输入对应的用户ID和电影ID,就可以得出一个匹配的分数(范围[0,5],分数越高视为兴趣越大),然后根据所有电影的推荐得分排序,推荐给用户可能感兴趣的电影。
+
+```
+Input movie_id: 1962
+Input user_id: 1
+Prediction Score is 4.25
+```
+
+## 模型概览
+
+本章中,我们首先介绍YouTube的视频推荐系统[[7](#参考文献)],然后介绍我们实现的融合推荐模型。
+
+### YouTube的深度神经网络推荐系统
+
+YouTube是世界上最大的视频上传、分享和发现网站,YouTube推荐系统为超过10亿用户从不断增长的视频库中推荐个性化的内容。整个系统由两个神经网络组成:候选生成网络和排序网络。候选生成网络从百万量级的视频库中生成上百个候选,排序网络对候选进行打分排序,输出排名最高的数十个结果。系统结构如图1所示:
+
+
+
+图1. YouTube 推荐系统结构
+
+
+#### 候选生成网络(Candidate Generation Network)
+
+候选生成网络将推荐问题建模为一个类别数极大的多类分类问题:对于一个Youtube用户,使用其观看历史(视频ID)、搜索词记录(search tokens)、人口学信息(如地理位置、用户登录设备)、二值特征(如性别,是否登录)和连续特征(如用户年龄)等,对视频库中所有视频进行多分类,得到每一类别的分类结果(即每一个视频的推荐概率),最终输出概率较高的几百个视频。
+
+首先,将观看历史及搜索词记录这类历史信息,映射为向量后取平均值得到定长表示;同时,输入人口学特征以优化新用户的推荐效果,并将二值特征和连续特征归一化处理到[0, 1]范围。接下来,将所有特征表示拼接为一个向量,并输入给非线形多层感知器(MLP,详见[识别数字](https://github.com/PaddlePaddle/book/blob/develop/02.recognize_digits/README.cn.md)教程)处理。最后,训练时将MLP的输出给softmax做分类,预测时计算用户的综合特征(MLP的输出)与所有视频的相似度,取得分最高的`$k$`个作为候选生成网络的筛选结果。图2显示了候选生成网络结构。
+
+
+
+图2. 候选生成网络结构
+
+
+对于一个用户`$U$`,预测此刻用户要观看的视频`$\omega$`为视频`$i$`的概率公式为:
+
+$$P(\omega=i|u)=\frac{e^{v_{i}u}}{\sum_{j \in V}e^{v_{j}u}}$$
+
+其中`$u$`为用户`$U$`的特征表示,`$V$`为视频库集合,`$v_i$`为视频库中第`$i$`个视频的特征表示。`$u$`和`$v_i$`为长度相等的向量,两者点积可以通过全连接层实现。
+
+考虑到softmax分类的类别数非常多,为了保证一定的计算效率:1)训练阶段,使用负样本类别采样将实际计算的类别数缩小至数千;2)推荐(预测)阶段,忽略softmax的归一化计算(不影响结果),将类别打分问题简化为点积(dot product)空间中的最近邻(nearest neighbor)搜索问题,取与`$u$`最近的`$k$`个视频作为生成的候选。
+
+#### 排序网络(Ranking Network)
+排序网络的结构类似于候选生成网络,但是它的目标是对候选进行更细致的打分排序。和传统广告排序中的特征抽取方法类似,这里也构造了大量的用于视频排序的相关特征(如视频 ID、上次观看时间等)。这些特征的处理方式和候选生成网络类似,不同之处是排序网络的顶部是一个加权逻辑回归(weighted logistic regression),它对所有候选视频进行打分,从高到底排序后将分数较高的一些视频返回给用户。
+
+### 融合推荐模型
+本节会使卷积神经网络(Convolutional Neural Networks)来学习电影名称的表示。下面会依次介绍文本卷积神经网络以及融合推荐模型。
+
+#### 文本卷积神经网络(CNN)
+
+卷积神经网络经常用来处理具有类似网格拓扑结构(grid-like topology)的数据。例如,图像可以视为二维网格的像素点,自然语言可以视为一维的词序列。卷积神经网络可以提取多种局部特征,并对其进行组合抽象得到更高级的特征表示。实验表明,卷积神经网络能高效地对图像及文本问题进行建模处理。
+
+卷积神经网络主要由卷积(convolution)和池化(pooling)操作构成,其应用及组合方式灵活多变,种类繁多。本小结我们以如图3所示的网络进行讲解:
+
+
+
+图3. 卷积神经网络文本分类模型
+
+
+假设待处理句子的长度为`$n$`,其中第`$i$`个词的词向量(word embedding)为`$x_i\in\mathbb{R}^k$`,`$k$`为维度大小。
+
+首先,进行词向量的拼接操作:将每`$h$`个词拼接起来形成一个大小为`$h$`的词窗口,记为`$x_{i:i+h-1}$`,它表示词序列`$x_{i},x_{i+1},\ldots,x_{i+h-1}$`的拼接,其中,`$i$`表示词窗口中第一个词在整个句子中的位置,取值范围从`$1$`到`$n-h+1$`,`$x_{i:i+h-1}\in\mathbb{R}^{hk}$`。
+
+其次,进行卷积操作:把卷积核(kernel)`$w\in\mathbb{R}^{hk}$`应用于包含`$h$`个词的窗口`$x_{i:i+h-1}$`,得到特征`$c_i=f(w\cdot x_{i:i+h-1}+b)$`,其中`$b\in\mathbb{R}$`为偏置项(bias),`$f$`为非线性激活函数,如`$sigmoid$`。将卷积核应用于句子中所有的词窗口`${x_{1:h},x_{2:h+1},\ldots,x_{n-h+1:n}}$`,产生一个特征图(feature map):
+
+$$c=[c_1,c_2,\ldots,c_{n-h+1}], c \in \mathbb{R}^{n-h+1}$$
+
+接下来,对特征图采用时间维度上的最大池化(max pooling over time)操作得到此卷积核对应的整句话的特征`$\hat c$`,它是特征图中所有元素的最大值:
+
+$$\hat c=max(c)$$
+
+#### 模型概览
+
+在融合推荐模型的电影推荐系统中:
+
+1. 首先,使用用户特征和电影特征作为神经网络的输入,其中:
+
+- 用户特征融合了四个属性信息,分别是用户ID、性别、职业和年龄。
+
+- 电影特征融合了三个属性信息,分别是电影ID、电影类型ID和电影名称。
+
+2. 对用户特征,将用户ID映射为维度大小为256的向量表示,输入全连接层,并对其他三个属性也做类似的处理。然后将四个属性的特征表示分别全连接并相加。
+
+3. 对电影特征,将电影ID以类似用户ID的方式进行处理,电影类型ID以向量的形式直接输入全连接层,电影名称用文本卷积神经网络得到其定长向量表示。然后将三个属性的特征表示分别全连接并相加。
+
+4. 得到用户和电影的向量表示后,计算二者的余弦相似度作为推荐系统的打分。最后,用该相似度打分和用户真实打分的差异的平方作为该回归模型的损失函数。
+
+
+
+图4. 融合推荐模型
+
+
+## 数据准备
+
+### 数据介绍与下载
+
+我们以 [MovieLens 百万数据集(ml-1m)](http://files.grouplens.org/datasets/movielens/ml-1m.zip)为例进行介绍。ml-1m 数据集包含了 6,000 位用户对 4,000 部电影的 1,000,000 条评价(评分范围 1~5 分,均为整数),由 GroupLens Research 实验室搜集整理。
+
+Paddle在API中提供了自动加载数据的模块。数据模块为 `paddle.dataset.movielens`
+
+
+```python
+import paddle
+movie_info = paddle.dataset.movielens.movie_info()
+print movie_info.values()[0]
+```
+
+
+```python
+# Run this block to show dataset's documentation
+# help(paddle.dataset.movielens)
+```
+
+在原始数据中包含电影的特征数据,用户的特征数据,和用户对电影的评分。
+
+例如,其中某一个电影特征为:
+
+
+```python
+movie_info = paddle.dataset.movielens.movie_info()
+print movie_info.values()[0]
+```
+
+
+
+
+这表示,电影的id是1,标题是《Toy Story》,该电影被分为到三个类别中。这三个类别是动画,儿童,喜剧。
+
+
+```python
+user_info = paddle.dataset.movielens.user_info()
+print user_info.values()[0]
+```
+
+
+
+
+这表示,该用户ID是1,女性,年龄比18岁还年轻。职业ID是10。
+
+
+其中,年龄使用下列分布
+* 1: "Under 18"
+* 18: "18-24"
+* 25: "25-34"
+* 35: "35-44"
+* 45: "45-49"
+* 50: "50-55"
+* 56: "56+"
+
+职业是从下面几种选项里面选则得出:
+* 0: "other" or not specified
+* 1: "academic/educator"
+* 2: "artist"
+* 3: "clerical/admin"
+* 4: "college/grad student"
+* 5: "customer service"
+* 6: "doctor/health care"
+* 7: "executive/managerial"
+* 8: "farmer"
+* 9: "homemaker"
+* 10: "K-12 student"
+* 11: "lawyer"
+* 12: "programmer"
+* 13: "retired"
+* 14: "sales/marketing"
+* 15: "scientist"
+* 16: "self-employed"
+* 17: "technician/engineer"
+* 18: "tradesman/craftsman"
+* 19: "unemployed"
+* 20: "writer"
+
+而对于每一条训练/测试数据,均为 <用户特征> + <电影特征> + 评分。
+
+例如,我们获得第一条训练数据:
+
+
+```python
+train_set_creator = paddle.dataset.movielens.train()
+train_sample = next(train_set_creator())
+uid = train_sample[0]
+mov_id = train_sample[len(user_info[uid].value())]
+print "User %s rates Movie %s with Score %s"%(user_info[uid], movie_info[mov_id], train_sample[-1])
+```
+
+User rates Movie with Score [5.0]
+
+
+即用户1对电影1193的评价为5分。
+
+## 模型配置说明
+
+下面我们开始根据输入数据的形式配置模型。首先引入所需的库函数以及定义全局变量。
+
+
+```python
+import math
+import sys
+import numpy as np
+import paddle
+import paddle.fluid as fluid
+import paddle.fluid.layers as layers
+import paddle.fluid.nets as nets
+
+IS_SPARSE = True
+USE_GPU = False
+BATCH_SIZE = 256
+```
+
+然后为我们的用户特征综合模型定义模型配置
+
+```python
+def get_usr_combined_features():
+
+USR_DICT_SIZE = paddle.dataset.movielens.max_user_id() + 1
+
+uid = layers.data(name='user_id', shape=[1], dtype='int64')
+
+usr_emb = layers.embedding(
+input=uid,
+dtype='float32',
+size=[USR_DICT_SIZE, 32],
+param_attr='user_table',
+is_sparse=IS_SPARSE)
+
+usr_fc = layers.fc(input=usr_emb, size=32)
+
+USR_GENDER_DICT_SIZE = 2
+
+usr_gender_id = layers.data(name='gender_id', shape=[1], dtype='int64')
+
+usr_gender_emb = layers.embedding(
+input=usr_gender_id,
+size=[USR_GENDER_DICT_SIZE, 16],
+param_attr='gender_table',
+is_sparse=IS_SPARSE)
+
+usr_gender_fc = layers.fc(input=usr_gender_emb, size=16)
+
+USR_AGE_DICT_SIZE = len(paddle.dataset.movielens.age_table)
+usr_age_id = layers.data(name='age_id', shape=[1], dtype="int64")
+
+usr_age_emb = layers.embedding(
+input=usr_age_id,
+size=[USR_AGE_DICT_SIZE, 16],
+is_sparse=IS_SPARSE,
+param_attr='age_table')
+
+usr_age_fc = layers.fc(input=usr_age_emb, size=16)
+
+USR_JOB_DICT_SIZE = paddle.dataset.movielens.max_job_id() + 1
+usr_job_id = layers.data(name='job_id', shape=[1], dtype="int64")
+
+usr_job_emb = layers.embedding(
+input=usr_job_id,
+size=[USR_JOB_DICT_SIZE, 16],
+param_attr='job_table',
+is_sparse=IS_SPARSE)
+
+usr_job_fc = layers.fc(input=usr_job_emb, size=16)
+
+concat_embed = layers.concat(
+input=[usr_fc, usr_gender_fc, usr_age_fc, usr_job_fc], axis=1)
+
+usr_combined_features = layers.fc(input=concat_embed, size=200, act="tanh")
+
+return usr_combined_features
+```
+
+如上述代码所示,对于每个用户,我们输入4维特征。其中包括user_id,gender_id,age_id,job_id。这几维特征均是简单的整数值。为了后续神经网络处理这些特征方便,我们借鉴NLP中的语言模型,将这几维离散的整数值,变换成embedding取出。分别形成usr_emb, usr_gender_emb, usr_age_emb, usr_job_emb。
+
+然后,我们对于所有的用户特征,均输入到一个全连接层(fc)中。将所有特征融合为一个200维度的特征。
+
+进而,我们对每一个电影特征做类似的变换,网络配置为:
+
+
+```python
+def get_mov_combined_features():
+
+MOV_DICT_SIZE = paddle.dataset.movielens.max_movie_id() + 1
+
+mov_id = layers.data(name='movie_id', shape=[1], dtype='int64')
+
+mov_emb = layers.embedding(
+input=mov_id,
+dtype='float32',
+size=[MOV_DICT_SIZE, 32],
+param_attr='movie_table',
+is_sparse=IS_SPARSE)
+
+mov_fc = layers.fc(input=mov_emb, size=32)
+
+CATEGORY_DICT_SIZE = len(paddle.dataset.movielens.movie_categories())
+
+category_id = layers.data(
+name='category_id', shape=[1], dtype='int64', lod_level=1)
+
+mov_categories_emb = layers.embedding(
+input=category_id, size=[CATEGORY_DICT_SIZE, 32], is_sparse=IS_SPARSE)
+
+mov_categories_hidden = layers.sequence_pool(
+input=mov_categories_emb, pool_type="sum")
+
+MOV_TITLE_DICT_SIZE = len(paddle.dataset.movielens.get_movie_title_dict())
+
+mov_title_id = layers.data(
+name='movie_title', shape=[1], dtype='int64', lod_level=1)
+
+mov_title_emb = layers.embedding(
+input=mov_title_id, size=[MOV_TITLE_DICT_SIZE, 32], is_sparse=IS_SPARSE)
+
+mov_title_conv = nets.sequence_conv_pool(
+input=mov_title_emb,
+num_filters=32,
+filter_size=3,
+act="tanh",
+pool_type="sum")
+
+concat_embed = layers.concat(
+input=[mov_fc, mov_categories_hidden, mov_title_conv], axis=1)
+
+mov_combined_features = layers.fc(input=concat_embed, size=200, act="tanh")
+
+return mov_combined_features
+```
+
+电影标题名称(title)是一个序列的整数,整数代表的是这个词在索引序列中的下标。这个序列会被送入 `sequence_conv_pool` 层,这个层会在时间维度上使用卷积和池化。因为如此,所以输出会是固定长度,尽管输入的序列长度各不相同。
+
+最后,我们定义一个`inference_program`来使用余弦相似度计算用户特征与电影特征的相似性。
+
+```python
+def inference_program():
+usr_combined_features = get_usr_combined_features()
+mov_combined_features = get_mov_combined_features()
+
+inference = layers.cos_sim(X=usr_combined_features, Y=mov_combined_features)
+scale_infer = layers.scale(x=inference, scale=5.0)
+
+return scale_infer
+```
+
+进而,我们定义一个`train_program`来使用`inference_program`计算出的结果,在标记数据的帮助下来计算误差。我们还定义了一个`optimizer_func`来定义优化器。
+
+```python
+def train_program():
+
+scale_infer = inference_program()
+
+label = layers.data(name='score', shape=[1], dtype='float32')
+square_cost = layers.square_error_cost(input=scale_infer, label=label)
+avg_cost = layers.mean(square_cost)
+
+return [avg_cost, scale_infer]
+
+
+def optimizer_func():
+return fluid.optimizer.SGD(learning_rate=0.2)
+```
+
+
+## 训练模型
+
+### 定义训练环境
+定义您的训练环境,可以指定训练是发生在CPU还是GPU上。
+
+```python
+use_cuda = False
+place = fluid.CUDAPlace(0) if use_cuda else fluid.CPUPlace()
+```
+
+### 定义数据提供器
+下一步是为训练和测试定义数据提供器。提供器读入一个大小为 `BATCH_SIZE`的数据。`paddle.dataset.movielens.train` 每次会在乱序化后提供一个大小为`BATCH_SIZE`的数据,乱序化的大小为缓存大小`buf_size`。
+
+```python
+train_reader = paddle.batch(
+paddle.reader.shuffle(
+paddle.dataset.movielens.train(), buf_size=8192),
+batch_size=BATCH_SIZE)
+
+test_reader = paddle.batch(
+paddle.dataset.movielens.test(), batch_size=BATCH_SIZE)
+```
+
+### 构造训练器(trainer)
+训练器需要一个训练程序和一个训练优化函数。
+
+```python
+trainer = fluid.Trainer(
+train_func=train_program, place=place, optimizer_func=optimizer_func)
+```
+
+### 提供数据
+
+`feed_order`用来定义每条产生的数据和`paddle.layer.data`之间的映射关系。比如,`movielens.train`产生的第一列的数据对应的是`user_id`这个特征。
+
+```python
+feed_order = [
+'user_id', 'gender_id', 'age_id', 'job_id', 'movie_id', 'category_id',
+'movie_title', 'score'
+]
+```
+
+### 事件处理器
+回调函数`event_handler`在一个之前定义好的事件发生后会被调用。例如,我们可以在每步训练结束后查看误差。
+
+```python
+# Specify the directory path to save the parameters
+params_dirname = "recommender_system.inference.model"
+
+from paddle.v2.plot import Ploter
+test_title = "Test cost"
+plot_cost = Ploter(test_title)
+
+
+def event_handler(event):
+if isinstance(event, fluid.EndStepEvent):
+avg_cost_set = trainer.test(
+reader=test_reader, feed_order=feed_order)
+
+# get avg cost
+avg_cost = np.array(avg_cost_set).mean()
+
+plot_cost.append(test_title, event.step, avg_cost_set[0])
+plot_cost.plot()
+
+print("avg_cost: %s" % avg_cost)
+print('BatchID {0}, Test Loss {1:0.2}'.format(event.epoch + 1,
+float(avg_cost)))
+
+if event.step == 20: # Adjust this number for accuracy
+trainer.save_params(params_dirname)
+trainer.stop()
+```
+
+### 开始训练
+最后,我们传入训练循环数(`num_epoch`)和一些别的参数,调用 `trainer.train` 来开始训练。
+
+```python
+trainer.train(
+num_epochs=1,
+event_handler=event_handler,
+reader=train_reader,
+feed_order=feed_order)
+```
+
+## 应用模型
+
+### 构建预测器
+传入`inference_program`和`params_dirname`来初始化一个预测器, `params_dirname`用来存放训练过程中的各个参数。
+
+```python
+inferencer = fluid.Inferencer(
+inference_program, param_path=params_dirname, place=place)
+```
+
+### 生成测试用输入数据
+使用 create_lod_tensor(data, lod, place) 的API来生成细节层次的张量。`data`是一个序列,每个元素是一个索引号的序列。`lod`是细节层次的信息,对应于`data`。比如,data = [[10, 2, 3], [2, 3]] 意味着它包含两个序列,长度分别是3和2。于是相应地 lod = [[3, 2]],它表明其包含一层细节信息,意味着 `data` 有两个序列,长度分别是3和2。
+
+在这个预测例子中,我们试着预测用户ID为1的用户对于电影'Hunchback of Notre Dame'的评分
+
+```python
+infer_movie_id = 783
+infer_movie_name = paddle.dataset.movielens.movie_info()[infer_movie_id].title
+user_id = fluid.create_lod_tensor([[1]], [[1]], place)
+gender_id = fluid.create_lod_tensor([[1]], [[1]], place)
+age_id = fluid.create_lod_tensor([[0]], [[1]], place)
+job_id = fluid.create_lod_tensor([[10]], [[1]], place)
+movie_id = fluid.create_lod_tensor([[783]], [[1]], place) # Hunchback of Notre Dame
+category_id = fluid.create_lod_tensor([[10, 8, 9]], [[3]], place) # Animation, Children's, Musical
+movie_title = fluid.create_lod_tensor([[1069, 4140, 2923, 710, 988]], [[5]],
+place) # 'hunchback','of','notre','dame','the'
+```
+
+### 测试
+现在我们可以进行预测了。我们要提供的`feed_order`应该和训练过程一致。
+
+
+```python
+results = inferencer.infer(
+{
+'user_id': user_id,
+'gender_id': gender_id,
+'age_id': age_id,
+'job_id': job_id,
+'movie_id': movie_id,
+'category_id': category_id,
+'movie_title': movie_title
+},
+return_numpy=False)
+```
+
+## 总结
+
+本章介绍了传统的推荐系统方法和YouTube的深度神经网络推荐系统,并以电影推荐为例,使用PaddlePaddle训练了一个个性化推荐神经网络模型。推荐系统几乎涵盖了电商系统、社交网络、广告推荐、搜索引擎等领域的方方面面,而在图像处理、自然语言处理等领域已经发挥重要作用的深度学习技术,也将会在推荐系统领域大放异彩。
+
+## 参考文献
+
+1. [Peter Brusilovsky](https://en.wikipedia.org/wiki/Peter_Brusilovsky) (2007). *The Adaptive Web*. p. 325.
+2. Robin Burke , [Hybrid Web Recommender Systems](http://www.dcs.warwick.ac.uk/~acristea/courses/CS411/2010/Book%20-%20The%20Adaptive%20Web/HybridWebRecommenderSystems.pdf), pp. 377-408, The Adaptive Web, Peter Brusilovsky, Alfred Kobsa, Wolfgang Nejdl (Ed.), Lecture Notes in Computer Science, Springer-Verlag, Berlin, Germany, Lecture Notes in Computer Science, Vol. 4321, May 2007, 978-3-540-72078-2.
+3. P. Resnick, N. Iacovou, etc. “[GroupLens: An Open Architecture for Collaborative Filtering of Netnews](http://ccs.mit.edu/papers/CCSWP165.html)”, Proceedings of ACM Conference on Computer Supported Cooperative Work, CSCW 1994. pp.175-186.
+4. Sarwar, Badrul, et al. "[Item-based collaborative filtering recommendation algorithms.](http://files.grouplens.org/papers/www10_sarwar.pdf)" *Proceedings of the 10th international conference on World Wide Web*. ACM, 2001.
+5. Kautz, Henry, Bart Selman, and Mehul Shah. "[Referral Web: combining social networks and collaborative filtering.](http://www.cs.cornell.edu/selman/papers/pdf/97.cacm.refweb.pdf)" Communications of the ACM 40.3 (1997): 63-65. APA
+6. Yuan, Jianbo, et al. ["Solving Cold-Start Problem in Large-scale Recommendation Engines: A Deep Learning Approach."](https://arxiv.org/pdf/1611.05480v1.pdf) *arXiv preprint arXiv:1611.05480* (2016).
+7. Covington P, Adams J, Sargin E. [Deep neural networks for youtube recommendations](https://static.googleusercontent.com/media/research.google.com/zh-CN//pubs/archive/45530.pdf)[C]//Proceedings of the 10th ACM Conference on Recommender Systems. ACM, 2016: 191-198.
+
+
+
+
本教程 由 PaddlePaddle 创作,采用 知识共享 署名-相同方式共享 4.0 国际 许可协议进行许可。
diff --git a/doc/fluid/new_docs/beginners_guide/basics/understand_sentiment/.gitignore b/doc/fluid/new_docs/beginners_guide/basics/understand_sentiment/.gitignore
new file mode 100644
index 0000000000000000000000000000000000000000..667762d327cb160376a4119fa9df9db41b6443b2
--- /dev/null
+++ b/doc/fluid/new_docs/beginners_guide/basics/understand_sentiment/.gitignore
@@ -0,0 +1,10 @@
+data/aclImdb
+data/imdb
+data/pre-imdb
+data/mosesdecoder-master
+*.log
+model_output
+dataprovider_copy_1.py
+model.list
+*.pyc
+.DS_Store
diff --git a/doc/fluid/new_docs/beginners_guide/basics/understand_sentiment/image/lstm.png b/doc/fluid/new_docs/beginners_guide/basics/understand_sentiment/image/lstm.png
new file mode 100644
index 0000000000000000000000000000000000000000..98fbea413a98a619004ca669c67f5f867fe974c9
Binary files /dev/null and b/doc/fluid/new_docs/beginners_guide/basics/understand_sentiment/image/lstm.png differ
diff --git a/doc/fluid/new_docs/beginners_guide/basics/understand_sentiment/image/lstm_en.png b/doc/fluid/new_docs/beginners_guide/basics/understand_sentiment/image/lstm_en.png
new file mode 100755
index 0000000000000000000000000000000000000000..d73a00bf2c1fca2f9b8c26bccf5ea844fa1db50b
Binary files /dev/null and b/doc/fluid/new_docs/beginners_guide/basics/understand_sentiment/image/lstm_en.png differ
diff --git a/doc/fluid/new_docs/beginners_guide/basics/understand_sentiment/image/rnn.png b/doc/fluid/new_docs/beginners_guide/basics/understand_sentiment/image/rnn.png
new file mode 100755
index 0000000000000000000000000000000000000000..26c904102a6e6c4e30f0048b81373ae8c148b355
Binary files /dev/null and b/doc/fluid/new_docs/beginners_guide/basics/understand_sentiment/image/rnn.png differ
diff --git a/doc/fluid/new_docs/beginners_guide/basics/understand_sentiment/image/stacked_lstm.jpg b/doc/fluid/new_docs/beginners_guide/basics/understand_sentiment/image/stacked_lstm.jpg
new file mode 100644
index 0000000000000000000000000000000000000000..6b2adf70f2b5112a2e82505da5cff9f5fd0c6298
Binary files /dev/null and b/doc/fluid/new_docs/beginners_guide/basics/understand_sentiment/image/stacked_lstm.jpg differ
diff --git a/doc/fluid/new_docs/beginners_guide/basics/understand_sentiment/image/stacked_lstm_en.png b/doc/fluid/new_docs/beginners_guide/basics/understand_sentiment/image/stacked_lstm_en.png
new file mode 100755
index 0000000000000000000000000000000000000000..8b5dbd726178b5555c513294e7b10a81acc96ff5
Binary files /dev/null and b/doc/fluid/new_docs/beginners_guide/basics/understand_sentiment/image/stacked_lstm_en.png differ
diff --git a/doc/fluid/new_docs/beginners_guide/basics/understand_sentiment/index.md b/doc/fluid/new_docs/beginners_guide/basics/understand_sentiment/index.md
new file mode 100644
index 0000000000000000000000000000000000000000..624de7e4d439953c7255481fb0c9d62ce94f3900
--- /dev/null
+++ b/doc/fluid/new_docs/beginners_guide/basics/understand_sentiment/index.md
@@ -0,0 +1,354 @@
+# 情感分析
+
+本教程源代码目录在[book/understand_sentiment](https://github.com/PaddlePaddle/book/tree/develop/06.understand_sentiment), 初次使用请参考PaddlePaddle[安装教程](https://github.com/PaddlePaddle/book/blob/develop/README.cn.md#运行这本书)。
+
+## 背景介绍
+
+在自然语言处理中,情感分析一般是指判断一段文本所表达的情绪状态。其中,一段文本可以是一个句子,一个段落或一个文档。情绪状态可以是两类,如(正面,负面),(高兴,悲伤);也可以是三类,如(积极,消极,中性)等等。情感分析的应用场景十分广泛,如把用户在购物网站(亚马逊、天猫、淘宝等)、旅游网站、电影评论网站上发表的评论分成正面评论和负面评论;或为了分析用户对于某一产品的整体使用感受,抓取产品的用户评论并进行情感分析等等。表格1展示了对电影评论进行情感分析的例子:
+
+| 电影评论 | 类别 |
+| -------- | ----- |
+| 在冯小刚这几年的电影里,算最好的一部的了| 正面 |
+| 很不好看,好像一个地方台的电视剧 | 负面 |
+| 圆方镜头全程炫技,色调背景美则美矣,但剧情拖沓,口音不伦不类,一直努力却始终无法入戏| 负面|
+|剧情四星。但是圆镜视角加上婺源的风景整个非常有中国写意山水画的感觉,看得实在太舒服了。。|正面|
+
+表格 1 电影评论情感分析
+
+在自然语言处理中,情感分析属于典型的**文本分类**问题,即把需要进行情感分析的文本划分为其所属类别。文本分类涉及文本表示和分类方法两个问题。在深度学习的方法出现之前,主流的文本表示方法为词袋模型BOW(bag of words),话题模型等等;分类方法有SVM(support vector machine), LR(logistic regression)等等。
+
+对于一段文本,BOW表示会忽略其词顺序、语法和句法,将这段文本仅仅看做是一个词集合,因此BOW方法并不能充分表示文本的语义信息。例如,句子“这部电影糟糕透了”和“一个乏味,空洞,没有内涵的作品”在情感分析中具有很高的语义相似度,但是它们的BOW表示的相似度为0。又如,句子“一个空洞,没有内涵的作品”和“一个不空洞而且有内涵的作品”的BOW相似度很高,但实际上它们的意思很不一样。
+
+本章我们所要介绍的深度学习模型克服了BOW表示的上述缺陷,它在考虑词顺序的基础上把文本映射到低维度的语义空间,并且以端对端(end to end)的方式进行文本表示及分类,其性能相对于传统方法有显著的提升\[[1](#参考文献)\]。
+
+## 模型概览
+本章所使用的文本表示模型为卷积神经网络(Convolutional Neural Networks)和循环神经网络(Recurrent Neural Networks)及其扩展。下面依次介绍这几个模型。
+
+### 文本卷积神经网络简介(CNN)
+
+我们在[推荐系统](https://github.com/PaddlePaddle/book/tree/develop/05.recommender_system)一节介绍过应用于文本数据的卷积神经网络模型的计算过程,这里进行一个简单的回顾。
+
+对卷积神经网络来说,首先使用卷积处理输入的词向量序列,产生一个特征图(feature map),对特征图采用时间维度上的最大池化(max pooling over time)操作得到此卷积核对应的整句话的特征,最后,将所有卷积核得到的特征拼接起来即为文本的定长向量表示,对于文本分类问题,将其连接至softmax即构建出完整的模型。在实际应用中,我们会使用多个卷积核来处理句子,窗口大小相同的卷积核堆叠起来形成一个矩阵,这样可以更高效的完成运算。另外,我们也可使用窗口大小不同的卷积核来处理句子,[推荐系统](https://github.com/PaddlePaddle/book/tree/develop/05.recommender_system)一节的图3作为示意画了四个卷积核,不同颜色表示不同大小的卷积核操作。
+
+对于一般的短文本分类问题,上文所述的简单的文本卷积网络即可达到很高的正确率\[[1](#参考文献)\]。若想得到更抽象更高级的文本特征表示,可以构建深层文本卷积神经网络\[[2](#参考文献),[3](#参考文献)\]。
+
+### 循环神经网络(RNN)
+
+循环神经网络是一种能对序列数据进行精确建模的有力工具。实际上,循环神经网络的理论计算能力是图灵完备的\[[4](#参考文献)\]。自然语言是一种典型的序列数据(词序列),近年来,循环神经网络及其变体(如long short term memory\[[5](#参考文献)\]等)在自然语言处理的多个领域,如语言模型、句法解析、语义角色标注(或一般的序列标注)、语义表示、图文生成、对话、机器翻译等任务上均表现优异甚至成为目前效果最好的方法。
+
+
+
+图1. 循环神经网络按时间展开的示意图
+
+
+循环神经网络按时间展开后如图1所示:在第`$t$`时刻,网络读入第`$t$`个输入`$x_t$`(向量表示)及前一时刻隐层的状态值`$h_{t-1}$`(向量表示,`$h_0$`一般初始化为`$0$`向量),计算得出本时刻隐层的状态值`$h_t$`,重复这一步骤直至读完所有输入。如果将循环神经网络所表示的函数记为`$f$`,则其公式可表示为:
+
+$$h_t=f(x_t,h_{t-1})=\sigma(W_{xh}x_t+W_{hh}h_{t-1}+b_h)$$
+
+其中`$W_{xh}$`是输入到隐层的矩阵参数,`$W_{hh}$`是隐层到隐层的矩阵参数,`$b_h$`为隐层的偏置向量(bias)参数,`$\sigma$`为`$sigmoid$`函数。
+
+在处理自然语言时,一般会先将词(one-hot表示)映射为其词向量(word embedding)表示,然后再作为循环神经网络每一时刻的输入`$x_t$`。此外,可以根据实际需要的不同在循环神经网络的隐层上连接其它层。如,可以把一个循环神经网络的隐层输出连接至下一个循环神经网络的输入构建深层(deep or stacked)循环神经网络,或者提取最后一个时刻的隐层状态作为句子表示进而使用分类模型等等。
+
+### 长短期记忆网络(LSTM)
+
+对于较长的序列数据,循环神经网络的训练过程中容易出现梯度消失或爆炸现象\[[6](#参考文献)\]。为了解决这一问题,Hochreiter S, Schmidhuber J. (1997)提出了LSTM(long short term memory\[[5](#参考文献)\])。
+
+相比于简单的循环神经网络,LSTM增加了记忆单元`$c$`、输入门`$i$`、遗忘门`$f$`及输出门`$o$`。这些门及记忆单元组合起来大大提升了循环神经网络处理长序列数据的能力。若将基于LSTM的循环神经网络表示的函数记为`$F$`,则其公式为:
+
+$$ h_t=F(x_t,h_{t-1})$$
+
+`$F$`由下列公式组合而成\[[7](#参考文献)\]:
+$$ i_t = \sigma{(W_{xi}x_t+W_{hi}h_{t-1}+W_{ci}c_{t-1}+b_i)} $$
+$$ f_t = \sigma(W_{xf}x_t+W_{hf}h_{t-1}+W_{cf}c_{t-1}+b_f) $$
+$$ c_t = f_t\odot c_{t-1}+i_t\odot tanh(W_{xc}x_t+W_{hc}h_{t-1}+b_c) $$
+$$ o_t = \sigma(W_{xo}x_t+W_{ho}h_{t-1}+W_{co}c_{t}+b_o) $$
+$$ h_t = o_t\odot tanh(c_t) $$
+其中,`$i_t, f_t, c_t, o_t$`分别表示输入门,遗忘门,记忆单元及输出门的向量值,带角标的`$W$`及`$b$`为模型参数,`$tanh$`为双曲正切函数,`$\odot$`表示逐元素(elementwise)的乘法操作。输入门控制着新输入进入记忆单元`$c$`的强度,遗忘门控制着记忆单元维持上一时刻值的强度,输出门控制着输出记忆单元的强度。三种门的计算方式类似,但有着完全不同的参数,它们各自以不同的方式控制着记忆单元`$c$`,如图2所示:
+
+
+
+图2. 时刻`$t$`的LSTM [7]
+
+
+LSTM通过给简单的循环神经网络增加记忆及控制门的方式,增强了其处理远距离依赖问题的能力。类似原理的改进还有Gated Recurrent Unit (GRU)\[[8](#参考文献)\],其设计更为简洁一些。**这些改进虽然各有不同,但是它们的宏观描述却与简单的循环神经网络一样(如图2所示),即隐状态依据当前输入及前一时刻的隐状态来改变,不断地循环这一过程直至输入处理完毕:**
+
+$$ h_t=Recrurent(x_t,h_{t-1})$$
+
+其中,`$Recrurent$`可以表示简单的循环神经网络、GRU或LSTM。
+
+### 栈式双向LSTM(Stacked Bidirectional LSTM)
+
+对于正常顺序的循环神经网络,`$h_t$`包含了`$t$`时刻之前的输入信息,也就是上文信息。同样,为了得到下文信息,我们可以使用反方向(将输入逆序处理)的循环神经网络。结合构建深层循环神经网络的方法(深层神经网络往往能得到更抽象和高级的特征表示),我们可以通过构建更加强有力的基于LSTM的栈式双向循环神经网络\[[9](#参考文献)\],来对时序数据进行建模。
+
+如图3所示(以三层为例),奇数层LSTM正向,偶数层LSTM反向,高一层的LSTM使用低一层LSTM及之前所有层的信息作为输入,对最高层LSTM序列使用时间维度上的最大池化即可得到文本的定长向量表示(这一表示充分融合了文本的上下文信息,并且对文本进行了深层次抽象),最后我们将文本表示连接至softmax构建分类模型。
+
+
+
+图3. 栈式双向LSTM用于文本分类
+
+
+
+## 数据集介绍
+
+我们以[IMDB情感分析数据集](http://ai.stanford.edu/%7Eamaas/data/sentiment/)为例进行介绍。IMDB数据集的训练集和测试集分别包含25000个已标注过的电影评论。其中,负面评论的得分小于等于4,正面评论的得分大于等于7,满分10分。
+```text
+aclImdb
+|- test
+|-- neg
+|-- pos
+|- train
+|-- neg
+|-- pos
+```
+Paddle在`dataset/imdb.py`中提实现了imdb数据集的自动下载和读取,并提供了读取字典、训练数据、测试数据等API。
+
+## 配置模型
+
+在该示例中,我们实现了两种文本分类算法,分别基于[推荐系统](https://github.com/PaddlePaddle/book/tree/develop/05.recommender_system)一节介绍过的文本卷积神经网络,以及[栈式双向LSTM](#栈式双向LSTM(Stacked Bidirectional LSTM))。我们首先引入要用到的库和定义全局变量:
+
+```python
+import paddle
+import paddle.fluid as fluid
+from functools import partial
+import numpy as np
+
+CLASS_DIM = 2
+EMB_DIM = 128
+HID_DIM = 512
+BATCH_SIZE = 128
+USE_GPU = False
+```
+
+
+### 文本卷积神经网络
+我们构建神经网络`convolution_net`,示例代码如下。
+需要注意的是:`fluid.nets.sequence_conv_pool` 包含卷积和池化层两个操作。
+
+```python
+def convolution_net(data, input_dim, class_dim, emb_dim, hid_dim):
+emb = fluid.layers.embedding(
+input=data, size=[input_dim, emb_dim], is_sparse=True)
+conv_3 = fluid.nets.sequence_conv_pool(
+input=emb,
+num_filters=hid_dim,
+filter_size=3,
+act="tanh",
+pool_type="sqrt")
+conv_4 = fluid.nets.sequence_conv_pool(
+input=emb,
+num_filters=hid_dim,
+filter_size=4,
+act="tanh",
+pool_type="sqrt")
+prediction = fluid.layers.fc(
+input=[conv_3, conv_4], size=class_dim, act="softmax")
+return prediction
+```
+
+网络的输入`input_dim`表示的是词典的大小,`class_dim`表示类别数。这里,我们使用[`sequence_conv_pool`](https://github.com/PaddlePaddle/Paddle/blob/develop/python/paddle/trainer_config_helpers/networks.py) API实现了卷积和池化操作。
+
+### 栈式双向LSTM
+
+栈式双向神经网络`stacked_lstm_net`的代码片段如下:
+
+```python
+def stacked_lstm_net(data, input_dim, class_dim, emb_dim, hid_dim, stacked_num):
+
+emb = fluid.layers.embedding(
+input=data, size=[input_dim, emb_dim], is_sparse=True)
+
+fc1 = fluid.layers.fc(input=emb, size=hid_dim)
+lstm1, cell1 = fluid.layers.dynamic_lstm(input=fc1, size=hid_dim)
+
+inputs = [fc1, lstm1]
+
+for i in range(2, stacked_num + 1):
+fc = fluid.layers.fc(input=inputs, size=hid_dim)
+lstm, cell = fluid.layers.dynamic_lstm(
+input=fc, size=hid_dim, is_reverse=(i % 2) == 0)
+inputs = [fc, lstm]
+
+fc_last = fluid.layers.sequence_pool(input=inputs[0], pool_type='max')
+lstm_last = fluid.layers.sequence_pool(input=inputs[1], pool_type='max')
+
+prediction = fluid.layers.fc(input=[fc_last, lstm_last],
+size=class_dim,
+act='softmax')
+return prediction
+```
+以上的栈式双向LSTM抽象出了高级特征并把其映射到和分类类别数同样大小的向量上。`paddle.activation.Softmax`函数用来计算分类属于某个类别的概率。
+
+重申一下,此处我们可以调用`convolution_net`或`stacked_lstm_net`的任何一个。我们以`convolution_net`为例。
+
+接下来我们定义预测程序(`inference_program`)。预测程序使用`convolution_net`来对`fluid.layer.data`的输入进行预测。
+
+```python
+def inference_program(word_dict):
+data = fluid.layers.data(
+name="words", shape=[1], dtype="int64", lod_level=1)
+
+dict_dim = len(word_dict)
+net = convolution_net(data, dict_dim, CLASS_DIM, EMB_DIM, HID_DIM)
+return net
+```
+
+我们这里定义了`training_program`。它使用了从`inference_program`返回的结果来计算误差。我们同时定义了优化函数`optimizer_func`。
+
+因为是有监督的学习,训练集的标签也在`paddle.layer.data`中定义了。在训练过程中,交叉熵用来在`paddle.layer.classification_cost`中作为损失函数。
+
+在测试过程中,分类器会计算各个输出的概率。第一个返回的数值规定为 损耗(cost)。
+
+```python
+def train_program(word_dict):
+prediction = inference_program(word_dict)
+label = fluid.layers.data(name="label", shape=[1], dtype="int64")
+cost = fluid.layers.cross_entropy(input=prediction, label=label)
+avg_cost = fluid.layers.mean(cost)
+accuracy = fluid.layers.accuracy(input=prediction, label=label)
+return [avg_cost, accuracy]
+
+
+def optimizer_func():
+return fluid.optimizer.Adagrad(learning_rate=0.002)
+```
+
+## 训练模型
+
+### 定义训练环境
+
+定义您的训练是在CPU上还是在GPU上:
+
+
+```python
+use_cuda = False
+place = fluid.CUDAPlace(0) if use_cuda else fluid.CPUPlace()
+```
+
+### 定义数据提供器
+
+下一步是为训练和测试定义数据提供器。提供器读入一个大小为 BATCH_SIZE的数据。paddle.dataset.imdb.train 每次会在乱序化后提供一个大小为BATCH_SIZE的数据,乱序化的大小为缓存大小buf_size。
+
+注意:读取IMDB的数据可能会花费几分钟的时间,请耐心等待。
+
+```python
+print("Loading IMDB word dict....")
+word_dict = paddle.dataset.imdb.word_dict()
+
+print ("Reading training data....")
+train_reader = paddle.batch(
+paddle.reader.shuffle(
+paddle.dataset.imdb.train(word_dict), buf_size=25000),
+batch_size=BATCH_SIZE)
+```
+
+### 构造训练器(trainer)
+训练器需要一个训练程序和一个训练优化函数。
+
+```python
+trainer = fluid.Trainer(
+train_func=partial(train_program, word_dict),
+place=place,
+optimizer_func=optimizer_func)
+```
+
+### 提供数据
+
+`feed_order`用来定义每条产生的数据和`paddle.layer.data`之间的映射关系。比如,`imdb.train`产生的第一列的数据对应的是`words`这个特征。
+
+```python
+feed_order = ['words', 'label']
+```
+
+### 事件处理器
+
+回调函数event_handler在一个之前定义好的事件发生后会被调用。例如,我们可以在每步训练结束后查看误差。
+
+```python
+# Specify the directory path to save the parameters
+params_dirname = "understand_sentiment_conv.inference.model"
+
+def event_handler(event):
+if isinstance(event, fluid.EndStepEvent):
+print("Step {0}, Epoch {1} Metrics {2}".format(
+event.step, event.epoch, map(np.array, event.metrics)))
+
+if event.step == 10:
+trainer.save_params(params_dirname)
+trainer.stop()
+```
+
+### 开始训练
+
+最后,我们传入训练循环数(num_epoch)和一些别的参数,调用 trainer.train 来开始训练。
+
+```python
+trainer.train(
+num_epochs=1,
+event_handler=event_handler,
+reader=train_reader,
+feed_order=feed_order)
+```
+
+## 应用模型
+
+### 构建预测器
+
+传入`inference_program`和`params_dirname`来初始化一个预测器, `params_dirname`用来存放训练过程中的各个参数。
+
+```python
+inferencer = fluid.Inferencer(
+inference_program, param_path=params_dirname, place=place)
+```
+
+### 生成测试用输入数据
+
+为了进行预测,我们任意选取3个评论。请随意选取您看好的3个。我们把评论中的每个词对应到`word_dict`中的id。如果词典中没有这个词,则设为`unknown`。
+然后我们用`create_lod_tensor`来创建细节层次的张量。
+
+```python
+reviews_str = [
+'read the book forget the movie', 'this is a great movie', 'this is very bad'
+]
+reviews = [c.split() for c in reviews_str]
+
+UNK = word_dict['']
+lod = []
+for c in reviews:
+lod.append([word_dict.get(words, UNK) for words in c])
+
+base_shape = [[len(c) for c in lod]]
+
+tensor_words = fluid.create_lod_tensor(lod, base_shape, place)
+```
+
+## 应用模型
+
+现在我们可以对每一条评论进行正面或者负面的预测啦。
+
+```python
+results = inferencer.infer({'words': tensor_words})
+
+for i, r in enumerate(results[0]):
+print("Predict probability of ", r[0], " to be positive and ", r[1], " to be negative for review \'", reviews_str[i], "\'")
+
+```
+
+
+## 总结
+
+本章我们以情感分析为例,介绍了使用深度学习的方法进行端对端的短文本分类,并且使用PaddlePaddle完成了全部相关实验。同时,我们简要介绍了两种文本处理模型:卷积神经网络和循环神经网络。在后续的章节中我们会看到这两种基本的深度学习模型在其它任务上的应用。
+
+
+## 参考文献
+1. Kim Y. [Convolutional neural networks for sentence classification](http://arxiv.org/pdf/1408.5882)[J]. arXiv preprint arXiv:1408.5882, 2014.
+2. Kalchbrenner N, Grefenstette E, Blunsom P. [A convolutional neural network for modelling sentences](http://arxiv.org/pdf/1404.2188.pdf?utm_medium=App.net&utm_source=PourOver)[J]. arXiv preprint arXiv:1404.2188, 2014.
+3. Yann N. Dauphin, et al. [Language Modeling with Gated Convolutional Networks](https://arxiv.org/pdf/1612.08083v1.pdf)[J] arXiv preprint arXiv:1612.08083, 2016.
+4. Siegelmann H T, Sontag E D. [On the computational power of neural nets](http://research.cs.queensu.ca/home/akl/cisc879/papers/SELECTED_PAPERS_FROM_VARIOUS_SOURCES/05070215382317071.pdf)[C]//Proceedings of the fifth annual workshop on Computational learning theory. ACM, 1992: 440-449.
+5. Hochreiter S, Schmidhuber J. [Long short-term memory](http://web.eecs.utk.edu/~itamar/courses/ECE-692/Bobby_paper1.pdf)[J]. Neural computation, 1997, 9(8): 1735-1780.
+6. Bengio Y, Simard P, Frasconi P. [Learning long-term dependencies with gradient descent is difficult](http://www-dsi.ing.unifi.it/~paolo/ps/tnn-94-gradient.pdf)[J]. IEEE transactions on neural networks, 1994, 5(2): 157-166.
+7. Graves A. [Generating sequences with recurrent neural networks](http://arxiv.org/pdf/1308.0850)[J]. arXiv preprint arXiv:1308.0850, 2013.
+8. Cho K, Van Merriënboer B, Gulcehre C, et al. [Learning phrase representations using RNN encoder-decoder for statistical machine translation](http://arxiv.org/pdf/1406.1078)[J]. arXiv preprint arXiv:1406.1078, 2014.
+9. Zhou J, Xu W. [End-to-end learning of semantic role labeling using recurrent neural networks](http://www.aclweb.org/anthology/P/P15/P15-1109.pdf)[C]//Proceedings of the Annual Meeting of the Association for Computational Linguistics. 2015.
+
+
+
本教程 由 PaddlePaddle 创作,采用 知识共享 署名-相同方式共享 4.0 国际 许可协议进行许可。
diff --git a/doc/fluid/new_docs/beginners_guide/basics/word2vec/.gitignore b/doc/fluid/new_docs/beginners_guide/basics/word2vec/.gitignore
new file mode 100644
index 0000000000000000000000000000000000000000..a620e0279c310d213d4e6d8e99e666962c11e352
--- /dev/null
+++ b/doc/fluid/new_docs/beginners_guide/basics/word2vec/.gitignore
@@ -0,0 +1,3 @@
+data/train.list
+data/test.list
+data/simple-examples*
diff --git a/doc/fluid/new_docs/beginners_guide/basics/word2vec/image/2d_similarity.png b/doc/fluid/new_docs/beginners_guide/basics/word2vec/image/2d_similarity.png
new file mode 100644
index 0000000000000000000000000000000000000000..384f59919a2c8dedb198e97d51434616648932e1
Binary files /dev/null and b/doc/fluid/new_docs/beginners_guide/basics/word2vec/image/2d_similarity.png differ
diff --git a/doc/fluid/new_docs/beginners_guide/basics/word2vec/image/cbow.png b/doc/fluid/new_docs/beginners_guide/basics/word2vec/image/cbow.png
new file mode 100644
index 0000000000000000000000000000000000000000..76b7d4bc0f99372465bd9aa34721513d39ad0776
Binary files /dev/null and b/doc/fluid/new_docs/beginners_guide/basics/word2vec/image/cbow.png differ
diff --git a/doc/fluid/new_docs/beginners_guide/basics/word2vec/image/cbow_en.png b/doc/fluid/new_docs/beginners_guide/basics/word2vec/image/cbow_en.png
new file mode 100755
index 0000000000000000000000000000000000000000..d985c393e618e9b79df05e4ff0ae57ccc93744d0
Binary files /dev/null and b/doc/fluid/new_docs/beginners_guide/basics/word2vec/image/cbow_en.png differ
diff --git a/doc/fluid/new_docs/beginners_guide/basics/word2vec/image/ngram.en.png b/doc/fluid/new_docs/beginners_guide/basics/word2vec/image/ngram.en.png
new file mode 100755
index 0000000000000000000000000000000000000000..2e16ab2f443732b8ef5404a8e7cd2457bc5eee23
Binary files /dev/null and b/doc/fluid/new_docs/beginners_guide/basics/word2vec/image/ngram.en.png differ
diff --git a/doc/fluid/new_docs/beginners_guide/basics/word2vec/image/ngram.png b/doc/fluid/new_docs/beginners_guide/basics/word2vec/image/ngram.png
new file mode 100644
index 0000000000000000000000000000000000000000..2449dce6a86b43b1b997ff418ed0dba56848463f
Binary files /dev/null and b/doc/fluid/new_docs/beginners_guide/basics/word2vec/image/ngram.png differ
diff --git a/doc/fluid/new_docs/beginners_guide/basics/word2vec/image/nnlm.png b/doc/fluid/new_docs/beginners_guide/basics/word2vec/image/nnlm.png
new file mode 100644
index 0000000000000000000000000000000000000000..1e0b40a8f7aefdf46d42761305511f281c08e595
Binary files /dev/null and b/doc/fluid/new_docs/beginners_guide/basics/word2vec/image/nnlm.png differ
diff --git a/doc/fluid/new_docs/beginners_guide/basics/word2vec/image/nnlm_en.png b/doc/fluid/new_docs/beginners_guide/basics/word2vec/image/nnlm_en.png
new file mode 100755
index 0000000000000000000000000000000000000000..158bd64b8f8729dea67834a8d591d21bce8b8564
Binary files /dev/null and b/doc/fluid/new_docs/beginners_guide/basics/word2vec/image/nnlm_en.png differ
diff --git a/doc/fluid/new_docs/beginners_guide/basics/word2vec/image/sentence_emb.png b/doc/fluid/new_docs/beginners_guide/basics/word2vec/image/sentence_emb.png
new file mode 100644
index 0000000000000000000000000000000000000000..ce4a8bf4769183cbaff91793753d2350a3ce936c
Binary files /dev/null and b/doc/fluid/new_docs/beginners_guide/basics/word2vec/image/sentence_emb.png differ
diff --git a/doc/fluid/new_docs/beginners_guide/basics/word2vec/image/skipgram.png b/doc/fluid/new_docs/beginners_guide/basics/word2vec/image/skipgram.png
new file mode 100644
index 0000000000000000000000000000000000000000..a3ab385845d3dc8b5c670bae91225bc8dd47a8bb
Binary files /dev/null and b/doc/fluid/new_docs/beginners_guide/basics/word2vec/image/skipgram.png differ
diff --git a/doc/fluid/new_docs/beginners_guide/basics/word2vec/image/skipgram_en.png b/doc/fluid/new_docs/beginners_guide/basics/word2vec/image/skipgram_en.png
new file mode 100755
index 0000000000000000000000000000000000000000..3c36c6d1f66eb98ea78c0673965d02a4ee3aa288
Binary files /dev/null and b/doc/fluid/new_docs/beginners_guide/basics/word2vec/image/skipgram_en.png differ
diff --git a/doc/fluid/new_docs/beginners_guide/basics/word2vec/index.md b/doc/fluid/new_docs/beginners_guide/basics/word2vec/index.md
new file mode 100644
index 0000000000000000000000000000000000000000..e73a6334ca1acd49379604f24d3d4e463192a902
--- /dev/null
+++ b/doc/fluid/new_docs/beginners_guide/basics/word2vec/index.md
@@ -0,0 +1,440 @@
+
+# 词向量
+
+本教程源代码目录在[book/word2vec](https://github.com/PaddlePaddle/book/tree/develop/04.word2vec), 初次使用请参考PaddlePaddle[安装教程](https://github.com/PaddlePaddle/book/blob/develop/README.cn.md#运行这本书)。
+
+## 背景介绍
+
+本章我们介绍词的向量表征,也称为word embedding。词向量是自然语言处理中常见的一个操作,是搜索引擎、广告系统、推荐系统等互联网服务背后常见的基础技术。
+
+在这些互联网服务里,我们经常要比较两个词或者两段文本之间的相关性。为了做这样的比较,我们往往先要把词表示成计算机适合处理的方式。最自然的方式恐怕莫过于向量空间模型(vector space model)。
+在这种方式里,每个词被表示成一个实数向量(one-hot vector),其长度为字典大小,每个维度对应一个字典里的每个词,除了这个词对应维度上的值是1,其他元素都是0。
+
+One-hot vector虽然自然,但是用处有限。比如,在互联网广告系统里,如果用户输入的query是“母亲节”,而有一个广告的关键词是“康乃馨”。虽然按照常理,我们知道这两个词之间是有联系的——母亲节通常应该送给母亲一束康乃馨;但是这两个词对应的one-hot vectors之间的距离度量,无论是欧氏距离还是余弦相似度(cosine similarity),由于其向量正交,都认为这两个词毫无相关性。 得出这种与我们相悖的结论的根本原因是:每个词本身的信息量都太小。所以,仅仅给定两个词,不足以让我们准确判别它们是否相关。要想精确计算相关性,我们还需要更多的信息——从大量数据里通过机器学习方法归纳出来的知识。
+
+在机器学习领域里,各种“知识”被各种模型表示,词向量模型(word embedding model)就是其中的一类。通过词向量模型可将一个 one-hot vector映射到一个维度更低的实数向量(embedding vector),如`$embedding(Mother's\ Day) = [0.3, 4.2, -1.5, ...], embedding(Carnation) = [0.2, 5.6, -2.3, ...]$`。在这个映射到的实数向量表示中,希望两个语义(或用法)上相似的词对应的词向量“更像”,这样如“母亲节”和“康乃馨”的对应词向量的余弦相似度就不再为零了。
+
+词向量模型可以是概率模型、共生矩阵(co-occurrence matrix)模型或神经元网络模型。在用神经网络求词向量之前,传统做法是统计一个词语的共生矩阵`$X$`。`$X$`是一个`$|V| \times |V|$` 大小的矩阵,`$X_{ij}$`表示在所有语料中,词汇表`V`(vocabulary)中第i个词和第j个词同时出现的词数,`$|V|$`为词汇表的大小。对`$X$`做矩阵分解(如奇异值分解,Singular Value Decomposition \[[5](#参考文献)\]),得到的`$U$`即视为所有词的词向量:
+
+$$X = USV^T$$
+
+但这样的传统做法有很多问题:
+1) 由于很多词没有出现,导致矩阵极其稀疏,因此需要对词频做额外处理来达到好的矩阵分解效果;
+2) 矩阵非常大,维度太高(通常达到`$10^6*10^6$`的数量级);
+3) 需要手动去掉停用词(如although, a,...),不然这些频繁出现的词也会影响矩阵分解的效果。
+
+
+基于神经网络的模型不需要计算存储一个在全语料上统计的大表,而是通过学习语义信息得到词向量,因此能很好地解决以上问题。在本章里,我们将展示基于神经网络训练词向量的细节,以及如何用PaddlePaddle训练一个词向量模型。
+
+
+## 效果展示
+
+本章中,当词向量训练好后,我们可以用数据可视化算法t-SNE\[[4](#参考文献)\]画出词语特征在二维上的投影(如下图所示)。从图中可以看出,语义相关的词语(如a, the, these; big, huge)在投影上距离很近,语意无关的词(如say, business; decision, japan)在投影上的距离很远。
+
+
+
+图1. 词向量的二维投影
+
+
+另一方面,我们知道两个向量的余弦值在`$[-1,1]$`的区间内:两个完全相同的向量余弦值为1, 两个相互垂直的向量之间余弦值为0,两个方向完全相反的向量余弦值为-1,即相关性和余弦值大小成正比。因此我们还可以计算两个词向量的余弦相似度:
+
+```
+similarity: 0.899180685161
+please input two words: big huge
+
+please input two words: from company
+similarity: -0.0997506977351
+```
+
+以上结果可以通过运行`calculate_dis.py`, 加载字典里的单词和对应训练特征结果得到,我们将在[应用模型](#应用模型)中详细描述用法。
+
+
+## 模型概览
+
+在这里我们介绍三个训练词向量的模型:N-gram模型,CBOW模型和Skip-gram模型,它们的中心思想都是通过上下文得到一个词出现的概率。对于N-gram模型,我们会先介绍语言模型的概念,并在之后的[训练模型](#训练模型)中,带大家用PaddlePaddle实现它。而后两个模型,是近年来最有名的神经元词向量模型,由 Tomas Mikolov 在Google 研发\[[3](#参考文献)\],虽然它们很浅很简单,但训练效果很好。
+
+### 语言模型
+
+在介绍词向量模型之前,我们先来引入一个概念:语言模型。
+语言模型旨在为语句的联合概率函数`$P(w_1, ..., w_T)$`建模, 其中`$w_i$`表示句子中的第i个词。语言模型的目标是,希望模型对有意义的句子赋予大概率,对没意义的句子赋予小概率。
+这样的模型可以应用于很多领域,如机器翻译、语音识别、信息检索、词性标注、手写识别等,它们都希望能得到一个连续序列的概率。 以信息检索为例,当你在搜索“how long is a football bame”时(bame是一个医学名词),搜索引擎会提示你是否希望搜索"how long is a football game", 这是因为根据语言模型计算出“how long is a football bame”的概率很低,而与bame近似的,可能引起错误的词中,game会使该句生成的概率最大。
+
+对语言模型的目标概率`$P(w_1, ..., w_T)$`,如果假设文本中每个词都是相互独立的,则整句话的联合概率可以表示为其中所有词语条件概率的乘积,即:
+
+$$P(w_1, ..., w_T) = \prod_{t=1}^TP(w_t)$$
+
+然而我们知道语句中的每个词出现的概率都与其前面的词紧密相关, 所以实际上通常用条件概率表示语言模型:
+
+$$P(w_1, ..., w_T) = \prod_{t=1}^TP(w_t | w_1, ... , w_{t-1})$$
+
+
+
+### N-gram neural model
+
+在计算语言学中,n-gram是一种重要的文本表示方法,表示一个文本中连续的n个项。基于具体的应用场景,每一项可以是一个字母、单词或者音节。 n-gram模型也是统计语言模型中的一种重要方法,用n-gram训练语言模型时,一般用每个n-gram的历史n-1个词语组成的内容来预测第n个词。
+
+Yoshua Bengio等科学家就于2003年在著名论文 Neural Probabilistic Language Models \[[1](#参考文献)\] 中介绍如何学习一个神经元网络表示的词向量模型。文中的神经概率语言模型(Neural Network Language Model,NNLM)通过一个线性映射和一个非线性隐层连接,同时学习了语言模型和词向量,即通过学习大量语料得到词语的向量表达,通过这些向量得到整个句子的概率。用这种方法学习语言模型可以克服维度灾难(curse of dimensionality),即训练和测试数据不同导致的模型不准。注意:由于“神经概率语言模型”说法较为泛泛,我们在这里不用其NNLM的本名,考虑到其具体做法,本文中称该模型为N-gram neural model。
+
+我们在上文中已经讲到用条件概率建模语言模型,即一句话中第`$t$`个词的概率和该句话的前`$t-1$`个词相关。可实际上越远的词语其实对该词的影响越小,那么如果考虑一个n-gram, 每个词都只受其前面`n-1`个词的影响,则有:
+
+$$P(w_1, ..., w_T) = \prod_{t=n}^TP(w_t|w_{t-1}, w_{t-2}, ..., w_{t-n+1})$$
+
+给定一些真实语料,这些语料中都是有意义的句子,N-gram模型的优化目标则是最大化目标函数:
+
+$$\frac{1}{T}\sum_t f(w_t, w_{t-1}, ..., w_{t-n+1};\theta) + R(\theta)$$
+
+其中`$f(w_t, w_{t-1}, ..., w_{t-n+1})$`表示根据历史n-1个词得到当前词`$w_t$`的条件概率,`$R(\theta)$`表示参数正则项。
+
+
+
+图2. N-gram神经网络模型
+
+
+图2展示了N-gram神经网络模型,从下往上看,该模型分为以下几个部分:
+- 对于每个样本,模型输入`$w_{t-n+1},...w_{t-1}$`, 输出句子第t个词为字典中`|V|`个词的概率。
+
+每个输入词`$w_{t-n+1},...w_{t-1}$`首先通过映射矩阵映射到词向量`$C(w_{t-n+1}),...C(w_{t-1})$`。
+
+- 然后所有词语的词向量连接成一个大向量,并经过一个非线性映射得到历史词语的隐层表示:
+
+$$g=Utanh(\theta^Tx + b_1) + Wx + b_2$$
+
+其中,`$x$`为所有词语的词向量连接成的大向量,表示文本历史特征;`$\theta$`、`$U$`、`$b_1$`、`$b_2$`和`$W$`分别为词向量层到隐层连接的参数。`$g$`表示未经归一化的所有输出单词概率,`$g_i$`表示未经归一化的字典中第`$i$`个单词的输出概率。
+
+- 根据softmax的定义,通过归一化`$g_i$`, 生成目标词`$w_t$`的概率为:
+
+$$P(w_t | w_1, ..., w_{t-n+1}) = \frac{e^{g_{w_t}}}{\sum_i^{|V|} e^{g_i}}$$
+
+- 整个网络的损失值(cost)为多类分类交叉熵,用公式表示为
+
+$$J(\theta) = -\sum_{i=1}^N\sum_{c=1}^{|V|}y_k^{i}log(softmax(g_k^i))$$
+
+其中`$y_k^i$`表示第`$i$`个样本第`$k$`类的真实标签(0或1),`$softmax(g_k^i)$`表示第i个样本第k类softmax输出的概率。
+
+
+
+### Continuous Bag-of-Words model(CBOW)
+
+CBOW模型通过一个词的上下文(各N个词)预测当前词。当N=2时,模型如下图所示:
+
+
+
+图3. CBOW模型
+
+
+具体来说,不考虑上下文的词语输入顺序,CBOW是用上下文词语的词向量的均值来预测当前词。即:
+
+$$context = \frac{x_{t-1} + x_{t-2} + x_{t+1} + x_{t+2}}{4}$$
+
+其中`$x_t$`为第`$t$`个词的词向量,分类分数(score)向量 `$z=U*context$`,最终的分类`$y$`采用softmax,损失函数采用多类分类交叉熵。
+
+### Skip-gram model
+
+CBOW的好处是对上下文词语的分布在词向量上进行了平滑,去掉了噪声,因此在小数据集上很有效。而Skip-gram的方法中,用一个词预测其上下文,得到了当前词上下文的很多样本,因此可用于更大的数据集。
+
+
+
+图4. Skip-gram模型
+
+
+如上图所示,Skip-gram模型的具体做法是,将一个词的词向量映射到`$2n$`个词的词向量(`$2n$`表示当前输入词的前后各`$n$`个词),然后分别通过softmax得到这`$2n$`个词的分类损失值之和。
+
+
+## 数据准备
+
+### 数据介绍
+
+本教程使用Penn Treebank (PTB)(经Tomas Mikolov预处理过的版本)数据集。PTB数据集较小,训练速度快,应用于Mikolov的公开语言模型训练工具\[[2](#参考文献)\]中。其统计情况如下:
+
+
+
+
+训练数据 |
+验证数据 |
+测试数据 |
+
+
+ptb.train.txt |
+ptb.valid.txt |
+ptb.test.txt |
+
+
+42068句 |
+3370句 |
+3761句 |
+
+
+
+
+
+### 数据预处理
+
+本章训练的是5-gram模型,表示在PaddlePaddle训练时,每条数据的前4个词用来预测第5个词。PaddlePaddle提供了对应PTB数据集的python包`paddle.dataset.imikolov`,自动做数据的下载与预处理,方便大家使用。
+
+预处理会把数据集中的每一句话前后加上开始符号``以及结束符号``。然后依据窗口大小(本教程中为5),从头到尾每次向右滑动窗口并生成一条数据。
+
+如"I have a dream that one day" 一句提供了5条数据:
+
+```text
+ I have a dream
+I have a dream that
+have a dream that one
+a dream that one day
+dream that one day
+```
+
+最后,每个输入会按其单词次在字典里的位置,转化成整数的索引序列,作为PaddlePaddle的输入。
+
+## 编程实现
+
+本配置的模型结构如下图所示:
+
+
+
+图5. 模型配置中的N-gram神经网络模型
+
+
+首先,加载所需要的包:
+
+```python
+import paddle
+import paddle.fluid as fluid
+import numpy
+from functools import partial
+import math
+import os
+import sys
+```
+
+然后,定义参数:
+```python
+EMBED_SIZE = 32 # word vector dimension
+HIDDEN_SIZE = 256 # hidden layer dimension
+N = 5 # train 5-gram
+BATCH_SIZE = 32 # batch size
+
+# can use CPU or GPU
+use_cuda = os.getenv('WITH_GPU', '0') != '0'
+
+word_dict = paddle.dataset.imikolov.build_dict()
+dict_size = len(word_dict)
+```
+
+不同于之前的PaddlePaddle v2版本,在新的Fluid版本里,我们不必再手动计算词向量。PaddlePaddle提供了一个内置的方法`fluid.layers.embedding`,我们就可以直接用它来构造 N-gram 神经网络。
+
+- 我们来定义我们的 N-gram 神经网络结构。这个结构在训练和预测中都会使用到。因为词向量比较稀疏,我们传入参数 `is_sparse == True`, 可以加速稀疏矩阵的更新。
+
+```python
+def inference_program(is_sparse):
+first_word = fluid.layers.data(name='firstw', shape=[1], dtype='int64')
+second_word = fluid.layers.data(name='secondw', shape=[1], dtype='int64')
+third_word = fluid.layers.data(name='thirdw', shape=[1], dtype='int64')
+fourth_word = fluid.layers.data(name='fourthw', shape=[1], dtype='int64')
+
+embed_first = fluid.layers.embedding(
+input=first_word,
+size=[dict_size, EMBED_SIZE],
+dtype='float32',
+is_sparse=is_sparse,
+param_attr='shared_w')
+embed_second = fluid.layers.embedding(
+input=second_word,
+size=[dict_size, EMBED_SIZE],
+dtype='float32',
+is_sparse=is_sparse,
+param_attr='shared_w')
+embed_third = fluid.layers.embedding(
+input=third_word,
+size=[dict_size, EMBED_SIZE],
+dtype='float32',
+is_sparse=is_sparse,
+param_attr='shared_w')
+embed_fourth = fluid.layers.embedding(
+input=fourth_word,
+size=[dict_size, EMBED_SIZE],
+dtype='float32',
+is_sparse=is_sparse,
+param_attr='shared_w')
+
+concat_embed = fluid.layers.concat(
+input=[embed_first, embed_second, embed_third, embed_fourth], axis=1)
+hidden1 = fluid.layers.fc(input=concat_embed,
+size=HIDDEN_SIZE,
+act='sigmoid')
+predict_word = fluid.layers.fc(input=hidden1, size=dict_size, act='softmax')
+return predict_word
+```
+
+- 基于以上的神经网络结构,我们可以如下定义我们的`训练`方法
+
+```python
+def train_program(is_sparse):
+# The declaration of 'next_word' must be after the invoking of inference_program,
+# or the data input order of train program would be [next_word, firstw, secondw,
+# thirdw, fourthw], which is not correct.
+predict_word = inference_program(is_sparse)
+next_word = fluid.layers.data(name='nextw', shape=[1], dtype='int64')
+cost = fluid.layers.cross_entropy(input=predict_word, label=next_word)
+avg_cost = fluid.layers.mean(cost)
+return avg_cost
+```
+
+- 现在我们可以开始训练啦。如今的版本较之以前就简单了许多。我们有现成的训练和测试集:`paddle.dataset.imikolov.train()`和`paddle.dataset.imikolov.test()`。两者都会返回一个读取器。在PaddlePaddle中,读取器是一个Python的函数,每次调用,会读取下一条数据。它是一个Python的generator。
+
+`paddle.batch` 会读入一个读取器,然后输出一个批次化了的读取器。`event_handler`亦可以一并传入`trainer.train`来时不时的输出每个步骤,批次的训练情况。
+
+```python
+def optimizer_func():
+# Note here we need to choose more sophisticated optimizers
+# such as AdaGrad with a decay rate. The normal SGD converges
+# very slowly.
+# optimizer=fluid.optimizer.SGD(learning_rate=0.001),
+return fluid.optimizer.AdagradOptimizer(
+learning_rate=3e-3,
+regularization=fluid.regularizer.L2DecayRegularizer(8e-4))
+
+
+def train(use_cuda, train_program, params_dirname):
+train_reader = paddle.batch(
+paddle.dataset.imikolov.train(word_dict, N), BATCH_SIZE)
+test_reader = paddle.batch(
+paddle.dataset.imikolov.test(word_dict, N), BATCH_SIZE)
+
+place = fluid.CUDAPlace(0) if use_cuda else fluid.CPUPlace()
+
+def event_handler(event):
+if isinstance(event, fluid.EndStepEvent):
+# We output cost every 10 steps.
+if event.step % 10 == 0:
+outs = trainer.test(
+reader=test_reader,
+feed_order=['firstw', 'secondw', 'thirdw', 'fourthw', 'nextw'])
+avg_cost = outs[0]
+
+print "Step %d: Average Cost %f" % (event.step, avg_cost)
+
+# If average cost is lower than 5.8, we consider the model good enough to stop.
+# Note 5.8 is a relatively high value. In order to get a better model, one should
+# aim for avg_cost lower than 3.5. But the training could take longer time.
+if avg_cost < 5.8:
+trainer.save_params(params_dirname)
+trainer.stop()
+
+if math.isnan(avg_cost):
+sys.exit("got NaN loss, training failed.")
+
+trainer = fluid.Trainer(
+train_func=train_program,
+optimizer_func=optimizer_func,
+place=place)
+
+trainer.train(
+reader=train_reader,
+num_epochs=1,
+event_handler=event_handler,
+feed_order=['firstw', 'secondw', 'thirdw', 'fourthw', 'nextw'])
+```
+
+- `trainer.train`将会开始训练。从`event_handler`返回的监控情况如下:
+
+```python
+Step 0: Average Cost 7.337213
+Step 10: Average Cost 6.136128
+Step 20: Average Cost 5.766995
+...
+```
+
+## 模型应用
+在模型训练后,我们可以用它做一些预测。
+
+### 预测下一个词
+我们可以用我们训练过的模型,在得知之前的 N-gram 后,预测下一个词。
+
+```python
+def infer(use_cuda, inference_program, params_dirname=None):
+place = fluid.CUDAPlace(0) if use_cuda else fluid.CPUPlace()
+inferencer = fluid.Inferencer(
+infer_func=inference_program, param_path=params_dirname, place=place)
+
+# Setup inputs by creating 4 LoDTensors representing 4 words. Here each word
+# is simply an index to look up for the corresponding word vector and hence
+# the shape of word (base_shape) should be [1]. The length-based level of
+# detail (lod) info of each LoDtensor should be [[1]] meaning there is only
+# one lod_level and there is only one sequence of one word on this level.
+# Note that lod info should be a list of lists.
+
+data1 = [[211]] # 'among'
+data2 = [[6]] # 'a'
+data3 = [[96]] # 'group'
+data4 = [[4]] # 'of'
+lod = [[1]]
+
+first_word = fluid.create_lod_tensor(data1, lod, place)
+second_word = fluid.create_lod_tensor(data2, lod, place)
+third_word = fluid.create_lod_tensor(data3, lod, place)
+fourth_word = fluid.create_lod_tensor(data4, lod, place)
+
+result = inferencer.infer(
+{
+'firstw': first_word,
+'secondw': second_word,
+'thirdw': third_word,
+'fourthw': fourth_word
+},
+return_numpy=False)
+
+print(numpy.array(result[0]))
+most_possible_word_index = numpy.argmax(result[0])
+print(most_possible_word_index)
+print([
+key for key, value in word_dict.iteritems()
+if value == most_possible_word_index
+][0])
+```
+
+在经历3分钟的短暂训练后,我们得到如下的预测。我们的模型预测 `among a group of` 的下一个词是`a`。这比较符合文法规律。如果我们训练时间更长,比如几个小时,那么我们会得到的下一个预测是 `workers`。
+
+
+```python
+[[0.00106646 0.0007907 0.00072041 ... 0.00049024 0.00041355 0.00084464]]
+6
+a
+```
+
+整个程序的入口很简单:
+
+```python
+def main(use_cuda, is_sparse):
+if use_cuda and not fluid.core.is_compiled_with_cuda():
+return
+
+params_dirname = "word2vec.inference.model"
+
+train(
+use_cuda=use_cuda,
+train_program=partial(train_program, is_sparse),
+params_dirname=params_dirname)
+
+infer(
+use_cuda=use_cuda,
+inference_program=partial(inference_program, is_sparse),
+params_dirname=params_dirname)
+
+
+main(use_cuda=use_cuda, is_sparse=True)
+```
+
+
+## 总结
+本章中,我们介绍了词向量、语言模型和词向量的关系、以及如何通过训练神经网络模型获得词向量。在信息检索中,我们可以根据向量间的余弦夹角,来判断query和文档关键词这二者间的相关性。在句法分析和语义分析中,训练好的词向量可以用来初始化模型,以得到更好的效果。在文档分类中,有了词向量之后,可以用聚类的方法将文档中同义词进行分组,也可以用 N-gram 来预测下一个词。希望大家在本章后能够自行运用词向量进行相关领域的研究。
+
+
+## 参考文献
+1. Bengio Y, Ducharme R, Vincent P, et al. [A neural probabilistic language model](http://www.jmlr.org/papers/volume3/bengio03a/bengio03a.pdf)[J]. journal of machine learning research, 2003, 3(Feb): 1137-1155.
+2. Mikolov T, Kombrink S, Deoras A, et al. [Rnnlm-recurrent neural network language modeling toolkit](http://www.fit.vutbr.cz/~imikolov/rnnlm/rnnlm-demo.pdf)[C]//Proc. of the 2011 ASRU Workshop. 2011: 196-201.
+3. Mikolov T, Chen K, Corrado G, et al. [Efficient estimation of word representations in vector space](https://arxiv.org/pdf/1301.3781.pdf)[J]. arXiv preprint arXiv:1301.3781, 2013.
+4. Maaten L, Hinton G. [Visualizing data using t-SNE](https://lvdmaaten.github.io/publications/papers/JMLR_2008.pdf)[J]. Journal of Machine Learning Research, 2008, 9(Nov): 2579-2605.
+5. https://en.wikipedia.org/wiki/Singular_value_decomposition
+
+
+
本教程 由 PaddlePaddle 创作,采用 知识共享 署名-相同方式共享 4.0 国际 许可协议进行许可。
diff --git a/doc/fluid/new_docs/beginners_guide/index.rst b/doc/fluid/new_docs/beginners_guide/index.rst
new file mode 100644
index 0000000000000000000000000000000000000000..e18933dcc0038129077a455892ddd785579f0003
--- /dev/null
+++ b/doc/fluid/new_docs/beginners_guide/index.rst
@@ -0,0 +1,15 @@
+########
+新手入门
+########
+
+.. todo::
+
+ 新手入门的导引文字,需要完善。
+
+.. toctree::
+ :maxdepth: 2
+
+ install/install_doc.rst
+ quick_start/index.rst
+ basics/index.rst
+ basics/learning_materials.md
diff --git a/doc/fluid/new_docs/beginners_guide/install/install_doc.rst b/doc/fluid/new_docs/beginners_guide/install/install_doc.rst
new file mode 100644
index 0000000000000000000000000000000000000000..8a66a95f45ea18dbfdc2450694517d5df8c47efd
--- /dev/null
+++ b/doc/fluid/new_docs/beginners_guide/install/install_doc.rst
@@ -0,0 +1,543 @@
+.. _how_to_install:
+
+安装说明
+^^^^^^^^
+
+若您的系统为Linux或Windows,您可以使用我们提供的安装包来安装PaddlePaddle。
+
+对于MacOS系统,我们暂未提供安装包,您可以使用 **从源码编译** 的方式安装。
+
+
+.. _install_linux:
+
+在Linux安装PaddlePaddle
+--------
+
+推荐您使用 `pip `_
+安装,它是Linux系统下最简单的安装方式。
+
+注意事项:
+
+- PaddlePaddle Python API 依赖Python 2.7版本。
+
+执行下面的命令即可在当前机器上安装PaddlePaddle的运行时环境,并自动下载安装依赖软件。
+
+ .. code-block:: bash
+
+ pip install paddlepaddle
+
+您可以通过指定版本号来安装其它版本,例如:
+
+ .. code-block:: bash
+
+ pip install paddlepaddle==0.13.0
+
+
+如果需要安装支持GPU的版本(cuda9.0_cudnn7_avx_openblas),需要执行:
+
+ .. code-block:: bash
+
+ pip install paddlepaddle-gpu
+
+PaddlePaddle针对不同需求提供了更多版本的安装包,部分列表如下:
+
+================================= ========================================
+版本号 版本说明
+================================= ========================================
+paddlepaddle-gpu==0.14.0 使用CUDA 9.0和cuDNN 7编译的0.14.0版本
+paddlepaddle-gpu==0.14.0.post87 使用CUDA 8.0和cuDNN 7编译的0.14.0版本
+paddlepaddle-gpu==0.14.0.post85 使用CUDA 8.0和cuDNN 5编译的0.14.0版本
+paddlepaddle-gpu==0.13.0 使用CUDA 9.0和cuDNN 7编译的0.13.0版本
+paddlepaddle-gpu==0.12.0 使用CUDA 8.0和cuDNN 5编译的0.12.0版本
+paddlepaddle-gpu==0.11.0.post87 使用CUDA 8.0和cuDNN 7编译的0.11.0版本
+paddlepaddle-gpu==0.11.0.post8 使用CUDA 8.0和cuDNN 5编译的0.11.0版本
+paddlepaddle-gpu==0.11.0 使用CUDA 7.5和cuDNN 5编译的0.11.0版本
+================================= ========================================
+
+您可以在 `Release History `_
+中找到paddlepaddle-gpu的各个发行版本。
+
+如果需要获取并安装最新的PaddlePaddle开发分支,可以从我们的 `CI系统 `_ 中下载最新的whl安装包和c-api开发包并安装。如需登录,请点击“Log in as guest”。
+
+.. _FAQ:
+
+安装常见问题和解决方法
+======================
+
+- paddlepaddle*.whl is not a supported wheel on this platform.
+
+出现这个问题的主要原因是,没有找到和当前系统匹配的paddlepaddle安装包。
+请检查Python版本是否为2.7系列。另外最新的pip官方源中的安装包默认是manylinux1标准,
+需要使用最新的pip (>9.0.0) 才可以安装。
+
+可以使用下面的命令更新您的pip:
+
+ .. code-block:: bash
+
+ pip install --upgrade pip
+
+如果仍然存在问题,可以执行:
+
+ .. code-block:: bash
+
+ python -c "import pip; print(pip.pep425tags.get_supported())"
+
+获取当前系统支持的安装包格式,并检查和需安装的包是否匹配。pypi安装包
+可以在 `这里 `_ 找到。
+
+如果系统支持的是 linux_x86_64 而安装包是 manylinux1_x86_64 ,需要升级pip版本到最新;
+如果系统支持 manylinux1_x86_64 而安装包(本地)是 linux_x86_64,
+可以重命名这个whl包为 manylinux1_x86_64 再安装。
+
+
+.. _install_windows:
+
+在Windows安装PaddlePaddle
+------------------------------
+Windows系统需要通过Docker来使用PaddleaPaddle。Docker是一个虚拟容器,使用Docker可以简化复杂的环境配置工作。
+
+我们提供了 `PaddlePaddle_Windows快速安装包 `_,
+它能够帮助您安装Docker和PaddlePaddle。
+
+* 安装包支持的系统:Windows7,Windows8的所有版本,Windows10的专业版、企业版。
+
+* 如果您希望使用GPU提升训练速度,请使用Linux系统安装,Windows系统暂不支持。
+
+.. _install_mac:
+
+在MacOS安装PaddlePaddle
+--------
+
+对于MacOS系统,我们暂未提供pip安装方式,您可以使用 **源码编译** 的方式安装。
+
+.. _others:
+
+其他安装方式
+-------------
+
+.. _source:
+源码编译(使用Docker镜像)
+==========
+
+.. _requirements:
+
+需要的软硬件
+"""""""""""""
+
+为了编译PaddlePaddle,我们需要
+
+1. 一台电脑,可以装的是 Linux, Windows 或者 MacOS 操作系统
+2. Docker
+
+不需要依赖其他任何软件了。即便是 Python 和 GCC 都不需要,因为我们会把所有编译工具都安装进一个 Docker 镜像里。
+
+.. _build_step:
+
+编译方法
+"""""""""""""
+
+PaddlePaddle需要使用Docker环境完成编译,这样可以免去单独安装编译依赖的步骤,可选的不同编译环境Docker镜像可以在 `这里 `_ 找到。
+
+
+**I. 编译CPU-Only版本的PaddlePaddle,需要执行:**
+
+.. code-block:: bash
+
+ # 1. 获取源码
+ git clone https://github.com/PaddlePaddle/Paddle.git
+ cd Paddle
+ # 2. 执行如下命令下载最新版本的docker镜像
+ docker run --name paddle-test -v $PWD:/paddle --network=host -it docker.paddlepaddlehub.com/paddle:latest-dev /bin/bash
+ # 3. 进入docker内执行如下命令编译CPU-Only的二进制安装包
+ mkdir -p /paddle/build && cd /paddle/build
+ cmake .. -DWITH_FLUID_ONLY=ON -DWITH_GPU=OFF -DWITH_TESTING=OFF
+ make -j$(nproc)
+
+**II. 编译GPU版本的PaddlePaddle,需要执行:**
+
+.. code-block:: bash
+
+ # 1. 获取源码
+ git clone https://github.com/PaddlePaddle/Paddle.git
+ cd Paddle
+ # 2. 安装nvidia-docker
+ apt-get install nvidia-docker
+ # 3. 执行如下命令下载支持GPU运行的docker容器
+ nvidia-docker run --name paddle-test-gpu -v $PWD:/paddle --network=host -it docker.paddlepaddlehub.com/paddle:latest-dev /bin/bash
+ # 4. 进入docker内执行如下命令编译GPU版本的PaddlePaddle
+ mkdir -p /paddle/build && cd /paddle/build
+ cmake .. -DWITH_FLUID_ONLY=ON -DWITH_GPU=ON -DWITH_TESTING=OFF
+ make -j$(nproc)
+
+**注意事项:**
+
+* 上述有关 :code:`docker` 的命令把当前目录(源码树根目录)映射为 container 里的 :code:`/paddle` 目录。
+* 进入 :code:`docker` 后执行 :code:`cmake` 命令,若是出现 :code:`patchelf not found, please install it.` 错误,则执行 :code:`apt-get install -y patchelf` 命令即可解决问题。
+* 若您在使用Docker编译PaddlePaddle遇到问题时, `这个issue `_ 可能会对您有所帮助。
+
+
+.. _source:
+源码编译(不使用Docker镜像)
+==========
+
+如果您选择不使用Docker镜像,则需要在本机安装下面章节列出的 `附录:编译依赖`_ 之后才能开始编译的步骤。
+
+.. _build_step:
+
+编译方法
+"""""""""""""
+
+在本机上编译CPU-Only版本的PaddlePaddle,需要执行如下命令:
+
+.. code-block:: bash
+
+ # 1. 使用virtualenvwrapper创建python虚环境并将工作空间切换到虚环境 [可选]
+ mkvirtualenv paddle-venv
+ workon paddle-venv
+ # 2. 获取源码
+ git clone https://github.com/PaddlePaddle/Paddle.git
+ cd Paddle
+ # 3. 执行下面的命令编译CPU-Only的二进制
+ mkdir build && cd build
+ cmake .. -DWITH_FLUID_ONLY=ON -DWITH_GPU=OFF -DWITH_TESTING=OFF
+ make -j4 # 根据机器配备CPU的核心数开启相应的多线程进行编译
+
+
+**注意事项:**
+
+* MacOS系统下因为默认安装了cblas库,所以编译时可能会遇到 :code:`use of undeclared identifier 'openblas_set_num_threads'` 错误。因此,在执行cmake命令时需要指定所使用openblas库的头文件路径,具体操作如下:
+
+ .. code-block:: bash
+
+ cd Paddle/build && rm -rf *
+ cmake .. -DWITH_FLUID_ONLY=ON -DWITH_GPU=OFF -DWITH_TESTING=OFF -DOPENBLAS_INC_DIR=/usr/local/Cellar/openblas/[本机所安装的openblas版本号]/include/
+ make -j4 # 根据机器配备CPU的核心数开启相应的多线程进行编译
+* 若您在MacOS系统下从源码编译PaddlePaddle遇到问题时, `这个issue `_ 可能会对您有所帮助。
+
+编译完成后会在build/python/dist目录下生成输出的whl包,可以选在在当前机器安装也可以拷贝到目标机器安装:
+
+.. code-block:: bash
+
+ pip install build/python/dist/*.whl
+
+如果机器中已经安装过PaddlePaddle,有两种方法:
+
+.. code-block:: bash
+
+ 1. 先卸载之前的版本,再重新安装
+ pip uninstall paddlepaddle
+ pip install build/python/dist/*.whl
+
+ 2. 直接升级到更新的版本
+ pip install build/python/dist/*.whl -U
+
+.. _run_test:
+
+执行单元测试
+"""""""""""""
+
+如果您期望在编译完成后立即执行所有的单元测试,可以按照下面的方法:
+
+设置 :code:`RUN_TEST=ON` 和 :code:`WITH_TESTING=ON` 就会在完成编译之后,立即执行单元测试。
+开启 :code:`WITH_GPU=ON` 可以指定同时执行GPU上的单元测试。
+
+.. code-block:: bash
+
+ docker run -it -v $PWD:/paddle -e "WITH_GPU=OFF" -e "WITH_TESTING=ON" -e "RUN_TEST=ON" docker.paddlepaddlehub.com/paddle:latest-dev bash -x /paddle/paddle/scripts/paddle_build.sh build
+
+如果期望执行其中一个单元测试,(比如 :code:`test_sum_op` ):
+
+.. code-block:: bash
+
+ docker run -it -v $PWD:/paddle -e "WITH_GPU=OFF" -e "WITH_TESTING=ON" -e "RUN_TEST=OFF" docker.paddlepaddlehub.com/paddle:latest-dev bash -x /paddle/paddle/scripts/paddle_build.sh build
+ cd /paddle/build
+ ctest -R test_sum_op -V
+
+.. _faq_docker:
+
+常见问题
+"""""""""""""
+
+- 什么是 Docker?
+
+ 如果您没有听说 Docker,可以把它想象为一个类似 virtualenv 的系统,但是虚拟的不仅仅是 Python 的运行环境。
+
+- Docker 还是虚拟机?
+
+ 有人用虚拟机来类比 Docker。需要强调的是:Docker 不会虚拟任何硬件,Docker container 里运行的编译工具实际上都是在本机的 CPU 和操作系统上直接运行的,性能和把编译工具安装在本机运行一样。
+
+- 为什么用 Docker?
+
+ 把工具和配置都安装在一个 Docker image 里可以标准化编译环境。这样如果遇到问题,其他人可以复现问题以便帮助。
+
+ 另外,对于习惯使用Windows和MacOS的开发者来说,使用Docker就不用配置交叉编译环境了。
+
+- 可以选择不用Docker吗?
+
+ 当然可以。大家可以用把开发工具安装进入 Docker image 一样的方式,把这些工具安装到本机。这篇文档介绍基于 Docker 的开发流程,是因为这个流程比其他方法都更简便。
+
+- 学习 Docker 有多难?
+
+ 理解 Docker 并不难,大概花十分钟看一下 `这篇文章 `_。
+ 这可以帮您省掉花一小时安装和配置各种开发工具,以及切换机器时需要新安装的辛苦。别忘了 PaddlePaddle 更新可能导致需要新的开发工具。更别提简化问题复现带来的好处了。
+
+- 可以用 IDE 吗?
+
+ 当然可以,因为源码就在本机上。IDE 默认调用 make 之类的程序来编译源码,我们只需要配置 IDE 来调用 Docker 命令编译源码即可。
+
+ 很多 PaddlePaddle 开发者使用 Emacs。他们在自己的 `~/.emacs` 配置文件里加两行
+
+ .. code-block:: bash
+
+ (global-set-key "\C-cc" 'compile)
+ (setq compile-command
+ "docker run --rm -it -v $(git rev-parse --show-toplevel):/paddle paddle:dev")
+
+ 就可以按 `Ctrl-C` 和 `c` 键来启动编译了。
+
+- 可以并行编译吗?
+
+ 是的。我们的 Docker image 运行一个 `Bash 脚本 `_。这个脚本调用 :code:`make -j$(nproc)` 来启动和 CPU 核一样多的进程来并行编译。
+
+- Docker 需要 sudo
+
+ 如果用自己的电脑开发,自然也就有管理员权限(sudo)了。如果用公用的电脑开发,需要请管理员安装和配置好 Docker。此外,PaddlePaddle 项目在努力开始支持其他不需要 sudo 的集装箱技术,比如 rkt。
+
+- 在 Windows/MacOS 上编译很慢
+
+ Docker 在 Windows 和 MacOS 都可以运行。不过实际上是运行在一个 Linux 虚拟机上。可能需要注意给这个虚拟机多分配一些 CPU 和内存,以保证编译高效。具体做法请参考 `这个issue `_。
+
+- 磁盘不够
+
+ 本文中的例子里, :code:`docker run` 命令里都用了 :code:`--rm` 参数,这样保证运行结束之后的 containers 不会保留在磁盘上。可以用 :code:`docker ps -a` 命令看到停止后但是没有删除的 containers。 :code:`docker build` 命令有时候会产生一些中间结果,是没有名字的 images,也会占用磁盘。可以参考 `这篇文章 `_ 来清理这些内容。
+
+
+.. _compile_deps:
+
+附录:编译依赖
+"""""""""""""
+
+PaddlePaddle编译需要使用到下面的依赖(包含但不限于),其他的依赖软件,会自动在编译时下载。
+
+.. csv-table:: PaddlePaddle编译依赖
+ :header: "依赖", "版本", "说明"
+ :widths: 10, 15, 30
+
+ "CMake", "3.4", ""
+ "GCC", "4.8.2", "推荐使用CentOS的devtools2"
+ "Python", "2.7.x", "依赖libpython2.7.so"
+ "SWIG", ">=2.0", ""
+ "wget","",""
+ "openblas","",""
+ "pip", ">=9.0", ""
+ "numpy", "", ""
+ "protobuf","3.1.0",""
+ "wheel","",""
+ "Go", ">=1.8", "可选"
+
+
+.. _build_options:
+
+附录:编译选项
+"""""""""""""
+
+PaddlePaddle的编译选项,包括生成CPU/GPU二进制文件、链接何种BLAS库等。
+用户可在调用cmake的时候设置它们,详细的cmake使用方法可以参考
+`官方文档 `_ 。
+
+在cmake的命令行中,通过使用 ``-D`` 命令设置该类编译选项,例如:
+
+.. code-block:: bash
+
+ cmake .. -DWITH_GPU=OFF
+
+.. csv-table:: 编译选项说明
+ :header: "选项", "说明", "默认值"
+ :widths: 1, 7, 2
+
+ "WITH_GPU", "是否支持GPU", "ON"
+ "WITH_C_API", "是否仅编译CAPI", "OFF"
+ "WITH_DOUBLE", "是否使用双精度浮点数", "OFF"
+ "WITH_DSO", "是否运行时动态加载CUDA动态库,而非静态加载CUDA动态库。", "ON"
+ "WITH_AVX", "是否编译含有AVX指令集的PaddlePaddle二进制文件", "ON"
+ "WITH_PYTHON", "是否内嵌PYTHON解释器", "ON"
+ "WITH_STYLE_CHECK", "是否编译时进行代码风格检查", "ON"
+ "WITH_TESTING", "是否开启单元测试", "OFF"
+ "WITH_DOC", "是否编译中英文文档", "OFF"
+ "WITH_SWIG_PY", "是否编译PYTHON的SWIG接口,该接口可用于预测和定制化训练", "Auto"
+ "WITH_GOLANG", "是否编译go语言的可容错parameter server", "OFF"
+ "WITH_MKL", "是否使用MKL数学库,如果为否则是用OpenBLAS", "ON"
+
+BLAS
++++++
+
+PaddlePaddle支持 `MKL `_ 和
+`OpenBlAS `_ 两种BLAS库。默认使用MKL。如果使用MKL并且机器含有AVX2指令集,
+还会下载MKL-DNN数学库,详细参考 `这里 `_ 。
+
+如果关闭MKL,则会使用OpenBLAS作为BLAS库。
+
+CUDA/cuDNN
++++++++++++
+
+PaddlePaddle在编译时/运行时会自动找到系统中安装的CUDA和cuDNN库进行编译和执行。
+使用参数 :code:`-DCUDA_ARCH_NAME=Auto` 可以指定开启自动检测SM架构,加速编译。
+
+PaddlePaddle可以使用cuDNN v5.1之后的任何一个版本来编译运行,但尽量请保持编译和运行使用的cuDNN是同一个版本。
+我们推荐使用最新版本的cuDNN。
+
+编译选项的设置
+++++++++++++++
+
+PaddePaddle通过编译时指定路径来实现引用各种BLAS/CUDA/cuDNN库。cmake编译时,首先在系统路径( :code:`/usr/lib:/usr/local/lib` )中搜索这几个库,同时也会读取相关路径变量来进行搜索。 通过使用 ``-D`` 命令可以设置,例如
+
+.. code-block:: bash
+
+ cmake .. -DWITH_GPU=ON -DWITH_TESTING=OFF -DCUDNN_ROOT=/opt/cudnnv5
+
+注意:这几个编译选项的设置,只在第一次cmake的时候有效。如果之后想要重新设置,推荐清理整个编译目录( :code:`rm -rf` )后,再指定。
+
+.. _install_docker:
+
+使用Docker安装运行
+==================
+
+使用Docker安装和运行PaddlePaddle可以无需考虑依赖环境。
+您可以在 `Docker官网 `_
+获得基本的Docker安装和使用方法。
+
+在了解Docker的基本使用方法之后,即可开始下面的步骤:
+
+.. _docker_pull:
+
+获取PaddlePaddle的Docker镜像
+""""""""""""""""""""""""""""
+
+执行下面的命令获取最新的PaddlePaddle Docker镜像,版本为cpu_avx_mkl:
+
+ .. code-block:: bash
+
+ docker pull paddlepaddle/paddle
+
+对于国内用户,我们提供了加速访问的镜像源:
+
+ .. code-block:: bash
+
+ docker pull docker.paddlepaddlehub.com/paddle
+
+下载GPU版本(cuda8.0_cudnn5_avx_mkl)的Docker镜像:
+
+ .. code-block:: bash
+
+ docker pull paddlepaddle/paddle:latest-gpu
+ docker pull docker.paddlepaddlehub.com/paddle:latest-gpu
+
+选择下载使用不同的BLAS库的Docker镜像:
+
+ .. code-block:: bash
+
+ # 默认是使用MKL的镜像
+ docker pull paddlepaddle/paddle
+ # 使用OpenBLAS的镜像
+ docker pull paddlepaddle/paddle:latest-openblas
+
+下载指定版本的Docker镜像,可以从 `DockerHub网站 `_ 获取可选的tag,并执行下面的命令:
+
+ .. code-block:: bash
+
+ docker pull paddlepaddle/paddle:[tag]
+ # 比如:
+ docker pull docker.paddlepaddlehub.com/paddle:0.11.0-gpu
+
+.. _docker_run:
+
+在Docker中执行PaddlePaddle训练程序
+"""""""""""""""""""""""""""""""""""
+
+假设您已经在当前目录(比如在/home/work)编写了一个PaddlePaddle的程序 :code:`train.py` (可以参考
+`PaddlePaddleBook `_
+编写),就可以使用下面的命令开始执行训练:
+
+ .. code-block:: bash
+
+ cd /home/work
+ docker run -it -v $PWD:/work paddlepaddle/paddle /work/train.py
+
+上述命令中, :code:`-it` 参数说明容器已交互式运行; :code:`-v $PWD:/work`
+指定将当前路径(Linux中$PWD变量会展开为当前路径的绝对路径)挂载到容器内部的 :code:`/work`
+目录; :code:`paddlepaddle/paddle` 指定需要使用的容器; 最后 :code:`/work/train.py`
+为容器内执行的命令,即运行训练程序。
+
+当然,您也可以进入到Docker容器中,以交互式的方式执行或调试您的代码:
+
+ .. code-block:: bash
+ docker run -it -v $PWD:/work paddlepaddle/paddle /bin/bash
+ cd /work
+ python train.py
+
+**注:PaddlePaddle Docker镜像为了减小体积,默认没有安装vim,您可以在容器中执行** :code:`apt-get install -y vim` **安装后,在容器中编辑代码。**
+
+.. _docker_run_book:
+
+使用Docker启动PaddlePaddle Book教程
+""""""""""""""""""""""""""""""""""""
+
+使用Docker可以快速在本地启动一个包含了PaddlePaddle官方Book教程的Jupyter Notebook,可以通过网页浏览。
+PaddlePaddle Book是为用户和开发者制作的一个交互式的Jupyter Notebook。
+如果您想要更深入了解deep learning,PaddlePaddle Book一定是您最好的选择。
+大家可以通过它阅读教程,或者制作和分享带有代码、公式、图表、文字的交互式文档。
+
+我们提供可以直接运行PaddlePaddle Book的Docker镜像,直接运行:
+
+ .. code-block:: bash
+
+ docker run -p 8888:8888 paddlepaddle/book
+
+国内用户可以使用下面的镜像源来加速访问:
+
+ .. code-block: bash
+
+ docker run -p 8888:8888 docker.paddlepaddlehub.com/book
+
+然后在浏览器中输入以下网址:
+
+ .. code-block:: text
+
+ http://localhost:8888/
+
+就这么简单,享受您的旅程!
+
+.. _docker_run_gpu:
+
+使用Docker执行GPU训练
+""""""""""""""""""""""""""""
+
+为了保证GPU驱动能够在镜像里面正常运行,我们推荐使用
+`nvidia-docker `_ 来运行镜像。
+请不要忘记提前在物理机上安装GPU最新驱动。
+
+ .. code-block:: bash
+
+ nvidia-docker run -it -v $PWD:/work paddlepaddle/paddle:latest-gpu /bin/bash
+
+**注: 如果没有安装nvidia-docker,可以尝试以下的方法,将CUDA库和Linux设备挂载到Docker容器内:**
+
+ .. code-block:: bash
+
+ export CUDA_SO="$(\ls /usr/lib64/libcuda* | xargs -I{} echo '-v {}:{}') $(\ls /usr/lib64/libnvidia* | xargs -I{} echo '-v {}:{}')"
+ export DEVICES=$(\ls /dev/nvidia* | xargs -I{} echo '--device {}:{}')
+ docker run ${CUDA_SO} ${DEVICES} -it paddlepaddle/paddle:latest-gpu
+
+**关于AVX:**
+
+AVX是一种CPU指令集,可以加速PaddlePaddle的计算。最新的PaddlePaddle Docker镜像默认
+是开启AVX编译的,所以,如果您的电脑不支持AVX,需要单独
+`编译 <./build_from_source_cn.html>`_ PaddlePaddle为no-avx版本。
+
+以下指令能检查Linux电脑是否支持AVX:
+
+ .. code-block:: bash
+
+ if cat /proc/cpuinfo | grep -i avx; then echo Yes; else echo No; fi
+
+如果输出是No,就需要选择使用no-AVX的镜像
diff --git a/doc/fluid/new_docs/beginners_guide/install/paddleci.png b/doc/fluid/new_docs/beginners_guide/install/paddleci.png
new file mode 100644
index 0000000000000000000000000000000000000000..16087ce059aa3c07ce8c927d983eb86351915825
Binary files /dev/null and b/doc/fluid/new_docs/beginners_guide/install/paddleci.png differ
diff --git a/doc/fluid/new_docs/beginners_guide/quick_start/fit_a_line/README.cn.md b/doc/fluid/new_docs/beginners_guide/quick_start/fit_a_line/README.cn.md
new file mode 100644
index 0000000000000000000000000000000000000000..ba43ada5100ed1db7192de9c795b4b8a6596d705
--- /dev/null
+++ b/doc/fluid/new_docs/beginners_guide/quick_start/fit_a_line/README.cn.md
@@ -0,0 +1,329 @@
+```eval_rst
+.. _quick_start_fit_a_line:
+```
+# 线性回归
+让我们从经典的线性回归(Linear Regression \[[1](#参考文献)\])模型开始这份教程。在这一章里,你将使用真实的数据集建立起一个房价预测模型,并且了解到机器学习中的若干重要概念。
+
+本教程源代码目录在[book/fit_a_line](https://github.com/PaddlePaddle/book/tree/develop/01.fit_a_line), 初次使用请参考PaddlePaddle[安装教程](https://github.com/PaddlePaddle/book/blob/develop/README.cn.md#运行这本书)。
+
+## 背景介绍
+给定一个大小为`$n$`的数据集 `${\{y_{i}, x_{i1}, ..., x_{id}\}}_{i=1}^{n}$`,其中`$x_{i1}, \ldots, x_{id}$`是第`$i$`个样本`$d$`个属性上的取值,`$y_i$`是该样本待预测的目标。线性回归模型假设目标`$y_i$`可以被属性间的线性组合描述,即
+
+$$y_i = \omega_1x_{i1} + \omega_2x_{i2} + \ldots + \omega_dx_{id} + b, i=1,\ldots,n$$
+
+例如,在我们将要建模的房价预测问题里,`$x_{ij}$`是描述房子`$i$`的各种属性(比如房间的个数、周围学校和医院的个数、交通状况等),而 `$y_i$`是房屋的价格。
+
+初看起来,这个假设实在过于简单了,变量间的真实关系很难是线性的。但由于线性回归模型有形式简单和易于建模分析的优点,它在实际问题中得到了大量的应用。很多经典的统计学习、机器学习书籍\[[2,3,4](#参考文献)\]也选择对线性模型独立成章重点讲解。
+
+## 效果展示
+我们使用从[UCI Housing Data Set](https://archive.ics.uci.edu/ml/datasets/Housing)获得的波士顿房价数据集进行模型的训练和预测。下面的散点图展示了使用模型对部分房屋价格进行的预测。其中,每个点的横坐标表示同一类房屋真实价格的中位数,纵坐标表示线性回归模型根据特征预测的结果,当二者值完全相等的时候就会落在虚线上。所以模型预测得越准确,则点离虚线越近。
+
+
+图1. 预测值 V.S. 真实值
+
+## 模型概览
+
+### 模型定义
+
+在波士顿房价数据集中,和房屋相关的值共有14个:前13个用来描述房屋相关的各种信息,即模型中的 `$x_i$`;最后一个值为我们要预测的该类房屋价格的中位数,即模型中的 `$y_i$`。因此,我们的模型就可以表示成:
+
+$$\hat{Y} = \omega_1X_{1} + \omega_2X_{2} + \ldots + \omega_{13}X_{13} + b$$
+
+`$\hat{Y}$` 表示模型的预测结果,用来和真实值`$Y$`区分。模型要学习的参数即:`$\omega_1, \ldots, \omega_{13}, b$`。
+
+建立模型后,我们需要给模型一个优化目标,使得学到的参数能够让预测值`$\hat{Y}$`尽可能地接近真实值`$Y$`。这里我们引入损失函数([Loss Function](https://en.wikipedia.org/wiki/Loss_function),或Cost Function)这个概念。 输入任意一个数据样本的目标值`$y_{i}$`和模型给出的预测值`$\hat{y_{i}}$`,损失函数输出一个非负的实值。这个实值通常用来反映模型误差的大小。
+
+对于线性回归模型来讲,最常见的损失函数就是均方误差(Mean Squared Error, [MSE](https://en.wikipedia.org/wiki/Mean_squared_error))了,它的形式是:
+
+$$MSE=\frac{1}{n}\sum_{i=1}^{n}{(\hat{Y_i}-Y_i)}^2$$
+
+即对于一个大小为`$n$`的测试集,`$MSE$`是`$n$`个数据预测结果误差平方的均值。
+
+### 训练过程
+
+定义好模型结构之后,我们要通过以下几个步骤进行模型训练
+1. 初始化参数,其中包括权重`$\omega_i$`和偏置`$b$`,对其进行初始化(如0均值,1方差)。
+2. 网络正向传播计算网络输出和损失函数。
+3. 根据损失函数进行反向误差传播 ([backpropagation](https://en.wikipedia.org/wiki/Backpropagation)),将网络误差从输出层依次向前传递, 并更新网络中的参数。
+4. 重复2~3步骤,直至网络训练误差达到规定的程度或训练轮次达到设定值。
+
+## 数据集
+
+### 数据集介绍
+这份数据集共506行,每行包含了波士顿郊区的一类房屋的相关信息及该类房屋价格的中位数。其各维属性的意义如下:
+
+
+
+
+
+ 属性名 |
+ 解释 |
+ 类型 |
+
+
+
+
+ CRIM |
+ 该镇的人均犯罪率 |
+ 连续值 |
+
+
+ ZN |
+ 占地面积超过25,000平方呎的住宅用地比例 |
+ 连续值 |
+
+
+ INDUS |
+ 非零售商业用地比例 |
+ 连续值 |
+
+
+ CHAS |
+ 是否邻近 Charles River |
+ 离散值,1=邻近;0=不邻近 |
+
+
+ NOX |
+ 一氧化氮浓度 |
+ 连续值 |
+
+
+ RM |
+ 每栋房屋的平均客房数 |
+ 连续值 |
+
+
+ AGE |
+ 1940年之前建成的自用单位比例 |
+ 连续值 |
+
+
+ DIS |
+ 到波士顿5个就业中心的加权距离 |
+ 连续值 |
+
+
+ RAD |
+ 到径向公路的可达性指数 |
+ 连续值 |
+
+
+ TAX |
+ 全值财产税率 |
+ 连续值 |
+
+
+ PTRATIO |
+ 学生与教师的比例 |
+ 连续值 |
+
+
+ B |
+ 1000(BK - 0.63)^2,其中BK为黑人占比 |
+ 连续值 |
+
+
+ LSTAT |
+ 低收入人群占比 |
+ 连续值 |
+
+
+ MEDV |
+ 同类房屋价格的中位数 |
+ 连续值 |
+
+
+
+
+
+### 数据预处理
+#### 连续值与离散值
+观察一下数据,我们的第一个发现是:所有的13维属性中,有12维的连续值和1维的离散值(CHAS)。离散值虽然也常使用类似0、1、2这样的数字表示,但是其含义与连续值是不同的,因为这里的差值没有实际意义。例如,我们用0、1、2来分别表示红色、绿色和蓝色的话,我们并不能因此说“蓝色和红色”比“绿色和红色”的距离更远。所以通常对一个有`$d$`个可能取值的离散属性,我们会将它们转为`$d$`个取值为0或1的二值属性或者将每个可能取值映射为一个多维向量。不过就这里而言,因为CHAS本身就是一个二值属性,就省去了这个麻烦。
+
+#### 属性的归一化
+另外一个稍加观察即可发现的事实是,各维属性的取值范围差别很大(如图2所示)。例如,属性B的取值范围是[0.32, 396.90],而属性NOX的取值范围是[0.3850, 0.8170]。这里就要用到一个常见的操作-归一化(normalization)了。归一化的目标是把各位属性的取值范围放缩到差不多的区间,例如[-0.5,0.5]。这里我们使用一种很常见的操作方法:减掉均值,然后除以原取值范围。
+
+做归一化(或 [Feature scaling](https://en.wikipedia.org/wiki/Feature_scaling))至少有以下3个理由:
+- 过大或过小的数值范围会导致计算时的浮点上溢或下溢。
+- 不同的数值范围会导致不同属性对模型的重要性不同(至少在训练的初始阶段如此),而这个隐含的假设常常是不合理的。这会对优化的过程造成困难,使训练时间大大的加长。
+- 很多的机器学习技巧/模型(例如L1,L2正则项,向量空间模型-Vector Space Model)都基于这样的假设:所有的属性取值都差不多是以0为均值且取值范围相近的。
+
+
+图2. 各维属性的取值范围
+
+#### 整理训练集与测试集
+我们将数据集分割为两份:一份用于调整模型的参数,即进行模型的训练,模型在这份数据集上的误差被称为**训练误差**;另外一份被用来测试,模型在这份数据集上的误差被称为**测试误差**。我们训练模型的目的是为了通过从训练数据中找到规律来预测未知的新数据,所以测试误差是更能反映模型表现的指标。分割数据的比例要考虑到两个因素:更多的训练数据会降低参数估计的方差,从而得到更可信的模型;而更多的测试数据会降低测试误差的方差,从而得到更可信的测试误差。我们这个例子中设置的分割比例为`$8:2$`
+
+
+在更复杂的模型训练过程中,我们往往还会多使用一种数据集:验证集。因为复杂的模型中常常还有一些超参数([Hyperparameter](https://en.wikipedia.org/wiki/Hyperparameter_optimization))需要调节,所以我们会尝试多种超参数的组合来分别训练多个模型,然后对比它们在验证集上的表现选择相对最好的一组超参数,最后才使用这组参数下训练的模型在测试集上评估测试误差。由于本章训练的模型比较简单,我们暂且忽略掉这个过程。
+
+## 训练
+
+`fit_a_line/trainer.py`演示了训练的整体过程。
+
+### 配置数据提供器(Datafeeder)
+首先我们引入必要的库:
+```python
+import paddle
+import paddle.fluid as fluid
+import numpy
+```
+
+我们通过uci_housing模块引入了数据集合[UCI Housing Data Set](https://archive.ics.uci.edu/ml/datasets/Housing)
+
+其中,在uci_housing模块中封装了:
+
+1. 数据下载的过程。下载数据保存在~/.cache/paddle/dataset/uci_housing/housing.data。
+2. [数据预处理](#数据预处理)的过程。
+
+接下来我们定义了用于训练和测试的数据提供器。提供器每次读入一个大小为`BATCH_SIZE`的数据批次。如果用户希望加一些随机性,她可以同时定义一个批次大小和一个缓存大小。这样的话,每次数据提供器会从缓存中随机读取批次大小那么多的数据。
+
+```python
+BATCH_SIZE = 20
+
+train_reader = paddle.batch(
+paddle.reader.shuffle(
+paddle.dataset.uci_housing.train(), buf_size=500),
+batch_size=BATCH_SIZE)
+
+test_reader = paddle.batch(
+paddle.reader.shuffle(
+paddle.dataset.uci_housing.test(), buf_size=500),
+batch_size=BATCH_SIZE)
+```
+
+### 配置训练程序
+训练程序的目的是定义一个训练模型的网络结构。对于线性回归来讲,它就是一个从输入到输出的简单的全连接层。更加复杂的结果,比如卷积神经网络,递归神经网络等会在随后的章节中介绍。训练程序必须返回`平均损失`作为第一个返回值,因为它会被后面反向传播算法所用到。
+
+```python
+def train_program():
+y = fluid.layers.data(name='y', shape=[1], dtype='float32')
+
+# feature vector of length 13
+x = fluid.layers.data(name='x', shape=[13], dtype='float32')
+y_predict = fluid.layers.fc(input=x, size=1, act=None)
+
+loss = fluid.layers.square_error_cost(input=y_predict, label=y)
+avg_loss = fluid.layers.mean(loss)
+
+return avg_loss
+```
+
+### 定义运算场所
+我们可以定义运算是发生在CPU还是GPU
+
+```python
+use_cuda = False
+place = fluid.CUDAPlace(0) if use_cuda else fluid.CPUPlace()
+```
+
+### 创建训练器
+训练器会读入一个训练程序和一些必要的其他参数:
+
+```python
+trainer = fluid.Trainer(
+train_func=train_program,
+place=place,
+optimizer_func=fluid.optimizer.SGD(learning_rate=0.001))
+```
+
+### 开始提供数据
+PaddlePaddle提供了读取数据者发生器机制来读取训练数据。读取数据者会一次提供多列数据,因此我们需要一个Python的list来定义读取顺序。
+
+```python
+feed_order=['x', 'y']
+```
+
+除此之外,可以定义一个事件相应器来处理类似`打印训练进程`的事件:
+
+```python
+# Specify the directory path to save the parameters
+params_dirname = "fit_a_line.inference.model"
+
+# Plot data
+from paddle.v2.plot import Ploter
+train_title = "Train cost"
+test_title = "Test cost"
+plot_cost = Ploter(train_title, test_title)
+
+step = 0
+
+# event_handler to print training and testing info
+def event_handler_plot(event):
+global step
+if isinstance(event, fluid.EndStepEvent):
+if event.step % 10 == 0: # every 10 batches, record a test cost
+test_metrics = trainer.test(
+reader=test_reader, feed_order=feed_order)
+
+plot_cost.append(test_title, step, test_metrics[0])
+plot_cost.plot()
+
+if test_metrics[0] < 10.0:
+# If the accuracy is good enough, we can stop the training.
+print('loss is less than 10.0, stop')
+trainer.stop()
+
+# We can save the trained parameters for the inferences later
+if params_dirname is not None:
+trainer.save_params(params_dirname)
+
+step += 1
+```
+
+### 开始训练
+我们现在可以通过调用`trainer.train()`来开始训练
+
+```python
+%matplotlib inline
+
+# The training could take up to a few minutes.
+trainer.train(
+reader=train_reader,
+num_epochs=100,
+event_handler=event_handler_plot,
+feed_order=feed_order)
+```
+
+
+
+## 预测
+提供一个`inference_program`和一个`params_dirname`来初始化预测器。`params_dirname`用来存储我们的参数。
+
+### 设定预测程序
+类似于`trainer.train`,预测器需要一个预测程序来做预测。我们可以稍加修改我们的训练程序来把预测值包含进来。
+
+
+```python
+def inference_program():
+x = fluid.layers.data(name='x', shape=[13], dtype='float32')
+y_predict = fluid.layers.fc(input=x, size=1, act=None)
+return y_predict
+```
+
+### 预测
+预测器会从`params_dirname`中读取已经训练好的模型,来对从未遇见过的数据进行预测。
+
+```python
+inferencer = fluid.Inferencer(
+infer_func=inference_program, param_path=params_dirname, place=place)
+
+batch_size = 10
+tensor_x = numpy.random.uniform(0, 10, [batch_size, 13]).astype("float32")
+
+results = inferencer.infer({'x': tensor_x})
+print("infer results: ", results[0])
+```
+
+## 总结
+在这章里,我们借助波士顿房价这一数据集,介绍了线性回归模型的基本概念,以及如何使用PaddlePaddle实现训练和测试的过程。很多的模型和技巧都是从简单的线性回归模型演化而来,因此弄清楚线性模型的原理和局限非常重要。
+
+
+## 参考文献
+1. https://en.wikipedia.org/wiki/Linear_regression
+2. Friedman J, Hastie T, Tibshirani R. The elements of statistical learning[M]. Springer, Berlin: Springer series in statistics, 2001.
+3. Murphy K P. Machine learning: a probabilistic perspective[M]. MIT press, 2012.
+4. Bishop C M. Pattern recognition[J]. Machine Learning, 2006, 128.
+
+
+
本教程 由 PaddlePaddle 创作,采用 知识共享 署名-相同方式共享 4.0 国际 许可协议进行许可。
diff --git a/doc/fluid/new_docs/beginners_guide/quick_start/fit_a_line/image/predictions.png b/doc/fluid/new_docs/beginners_guide/quick_start/fit_a_line/image/predictions.png
new file mode 100644
index 0000000000000000000000000000000000000000..27e4acb1313794f52ad9ad9e874cdadd197ff41f
Binary files /dev/null and b/doc/fluid/new_docs/beginners_guide/quick_start/fit_a_line/image/predictions.png differ
diff --git a/doc/fluid/new_docs/beginners_guide/quick_start/fit_a_line/image/ranges.png b/doc/fluid/new_docs/beginners_guide/quick_start/fit_a_line/image/ranges.png
new file mode 100644
index 0000000000000000000000000000000000000000..5d86b12715f46afbafb7d50e2938e184219b5b95
Binary files /dev/null and b/doc/fluid/new_docs/beginners_guide/quick_start/fit_a_line/image/ranges.png differ
diff --git a/doc/fluid/new_docs/beginners_guide/quick_start/fit_a_line/image/train_and_test.png b/doc/fluid/new_docs/beginners_guide/quick_start/fit_a_line/image/train_and_test.png
new file mode 100644
index 0000000000000000000000000000000000000000..bcd304a6a0baf30ecfbc43e08fc0aca179d05958
Binary files /dev/null and b/doc/fluid/new_docs/beginners_guide/quick_start/fit_a_line/image/train_and_test.png differ
diff --git a/doc/fluid/new_docs/beginners_guide/quick_start/index.rst b/doc/fluid/new_docs/beginners_guide/quick_start/index.rst
new file mode 100644
index 0000000000000000000000000000000000000000..f5889ba52b8016596108de48bad59f238c16afc0
--- /dev/null
+++ b/doc/fluid/new_docs/beginners_guide/quick_start/index.rst
@@ -0,0 +1,13 @@
+########
+快速入门
+########
+
+.. todo::
+
+ 概述
+
+.. toctree::
+ :maxdepth: 2
+
+ fit_a_line/README.cn.md
+ recognize_digits/README.cn.md
diff --git a/doc/fluid/new_docs/beginners_guide/quick_start/recognize_digits/README.cn.md b/doc/fluid/new_docs/beginners_guide/quick_start/recognize_digits/README.cn.md
new file mode 100644
index 0000000000000000000000000000000000000000..c04a949a3f6550048f2a3447070829aeb640b995
--- /dev/null
+++ b/doc/fluid/new_docs/beginners_guide/quick_start/recognize_digits/README.cn.md
@@ -0,0 +1,453 @@
+# 识别数字
+
+本教程源代码目录在[book/recognize_digits](https://github.com/PaddlePaddle/book/tree/develop/02.recognize_digits), 初次使用请参考PaddlePaddle[安装教程](https://github.com/PaddlePaddle/book/blob/develop/README.cn.md#运行这本书)。
+
+## 背景介绍
+当我们学习编程的时候,编写的第一个程序一般是实现打印"Hello World"。而机器学习(或深度学习)的入门教程,一般都是 [MNIST](http://yann.lecun.com/exdb/mnist/) 数据库上的手写识别问题。原因是手写识别属于典型的图像分类问题,比较简单,同时MNIST数据集也很完备。MNIST数据集作为一个简单的计算机视觉数据集,包含一系列如图1所示的手写数字图片和对应的标签。图片是28x28的像素矩阵,标签则对应着0~9的10个数字。每张图片都经过了大小归一化和居中处理。
+
+
+图1. MNIST图片示例
+
+MNIST数据集是从 [NIST](https://www.nist.gov/srd/nist-special-database-19) 的Special Database 3(SD-3)和Special Database 1(SD-1)构建而来。由于SD-3是由美国人口调查局的员工进行标注,SD-1是由美国高中生进行标注,因此SD-3比SD-1更干净也更容易识别。Yann LeCun等人从SD-1和SD-3中各取一半作为MNIST的训练集(60000条数据)和测试集(10000条数据),其中训练集来自250位不同的标注员,此外还保证了训练集和测试集的标注员是不完全相同的。
+
+Yann LeCun早先在手写字符识别上做了很多研究,并在研究过程中提出了卷积神经网络(Convolutional Neural Network),大幅度地提高了手写字符的识别能力,也因此成为了深度学习领域的奠基人之一。如今的深度学习领域,卷积神经网络占据了至关重要的地位,从最早Yann LeCun提出的简单LeNet,到如今ImageNet大赛上的优胜模型VGGNet、GoogLeNet、ResNet等(请参见[图像分类](https://github.com/PaddlePaddle/book/tree/develop/03.image_classification) 教程),人们在图像分类领域,利用卷积神经网络得到了一系列惊人的结果。
+
+有很多算法在MNIST上进行实验。1998年,LeCun分别用单层线性分类器、多层感知器(Multilayer Perceptron, MLP)和多层卷积神经网络LeNet进行实验,使得测试集上的误差不断下降(从12%下降到0.7%)\[[1](#参考文献)\]。此后,科学家们又基于K近邻(K-Nearest Neighbors)算法\[[2](#参考文献)\]、支持向量机(SVM)\[[3](#参考文献)\]、神经网络\[[4-7](#参考文献)\]和Boosting方法\[[8](#参考文献)\]等做了大量实验,并采用多种预处理方法(如去除歪曲、去噪、模糊等)来提高识别的准确率。
+
+本教程中,我们从简单的模型Softmax回归开始,带大家入门手写字符识别,并逐步进行模型优化。
+
+
+## 模型概览
+
+基于MNIST数据训练一个分类器,在介绍本教程使用的三个基本图像分类网络前,我们先给出一些定义:
+- `$X$`是输入:MNIST图片是`$28\times28$` 的二维图像,为了进行计算,我们将其转化为`$784$`维向量,即`$X=\left ( x_0, x_1, \dots, x_{783} \right )$`。
+- `$Y$`是输出:分类器的输出是10类数字(0-9),即`$Y=\left ( y_0, y_1, \dots, y_9 \right )$`,每一维`$y_i$`代表图片分类为第`$i$`类数字的概率。
+- `$L$`是图片的真实标签:`$L=\left ( l_0, l_1, \dots, l_9 \right )$`也是10维,但只有一维为1,其他都为0。
+
+### Softmax回归(Softmax Regression)
+
+最简单的Softmax回归模型是先将输入层经过一个全连接层得到的特征,然后直接通过softmax 函数进行多分类\[[9](#参考文献)\]。
+
+输入层的数据`$X$`传到输出层,在激活操作之前,会乘以相应的权重 `$W$` ,并加上偏置变量 `$b$` ,具体如下:
+
+$$ y_i = \text{softmax}(\sum_j W_{i,j}x_j + b_i) $$
+
+其中 `$ \text{softmax}(x_i) = \frac{e^{x_i}}{\sum_j e^{x_j}} $`
+
+对于有 `$N$` 个类别的多分类问题,指定 `$N$` 个输出节点,`$N$` 维结果向量经过softmax将归一化为 `$N$` 个[0,1]范围内的实数值,分别表示该样本属于这 `$N$` 个类别的概率。此处的 `$y_i$` 即对应该图片为数字 `$i$` 的预测概率。
+
+在分类问题中,我们一般采用交叉熵代价损失函数(cross entropy),公式如下:
+
+$$ \text{crossentropy}(label, y) = -\sum_i label_ilog(y_i) $$
+
+图2为softmax回归的网络图,图中权重用蓝线表示、偏置用红线表示、+1代表偏置参数的系数为1。
+
+
+图2. softmax回归网络结构图
+
+### 多层感知器(Multilayer Perceptron, MLP)
+
+Softmax回归模型采用了最简单的两层神经网络,即只有输入层和输出层,因此其拟合能力有限。为了达到更好的识别效果,我们考虑在输入层和输出层中间加上若干个隐藏层\[[10](#参考文献)\]。
+
+1. 经过第一个隐藏层,可以得到 `$ H_1 = \phi(W_1X + b_1) $`,其中`$\phi$`代表激活函数,常见的有sigmoid、tanh或ReLU等函数。
+2. 经过第二个隐藏层,可以得到 `$ H_2 = \phi(W_2H_1 + b_2) $`。
+3. 最后,再经过输出层,得到的`$Y=\text{softmax}(W_3H_2 + b_3)$`,即为最后的分类结果向量。
+
+
+图3为多层感知器的网络结构图,图中权重用蓝线表示、偏置用红线表示、+1代表偏置参数的系数为1。
+
+
+图3. 多层感知器网络结构图
+
+### 卷积神经网络(Convolutional Neural Network, CNN)
+
+在多层感知器模型中,将图像展开成一维向量输入到网络中,忽略了图像的位置和结构信息,而卷积神经网络能够更好的利用图像的结构信息。[LeNet-5](http://yann.lecun.com/exdb/lenet/)是一个较简单的卷积神经网络。图4显示了其结构:输入的二维图像,先经过两次卷积层到池化层,再经过全连接层,最后使用softmax分类作为输出层。下面我们主要介绍卷积层和池化层。
+
+
+图4. LeNet-5卷积神经网络结构
+
+#### 卷积层
+
+卷积层是卷积神经网络的核心基石。在图像识别里我们提到的卷积是二维卷积,即离散二维滤波器(也称作卷积核)与二维图像做卷积操作,简单的讲是二维滤波器滑动到二维图像上所有位置,并在每个位置上与该像素点及其领域像素点做内积。卷积操作被广泛应用与图像处理领域,不同卷积核可以提取不同的特征,例如边沿、线性、角等特征。在深层卷积神经网络中,通过卷积操作可以提取出图像低级到复杂的特征。
+
+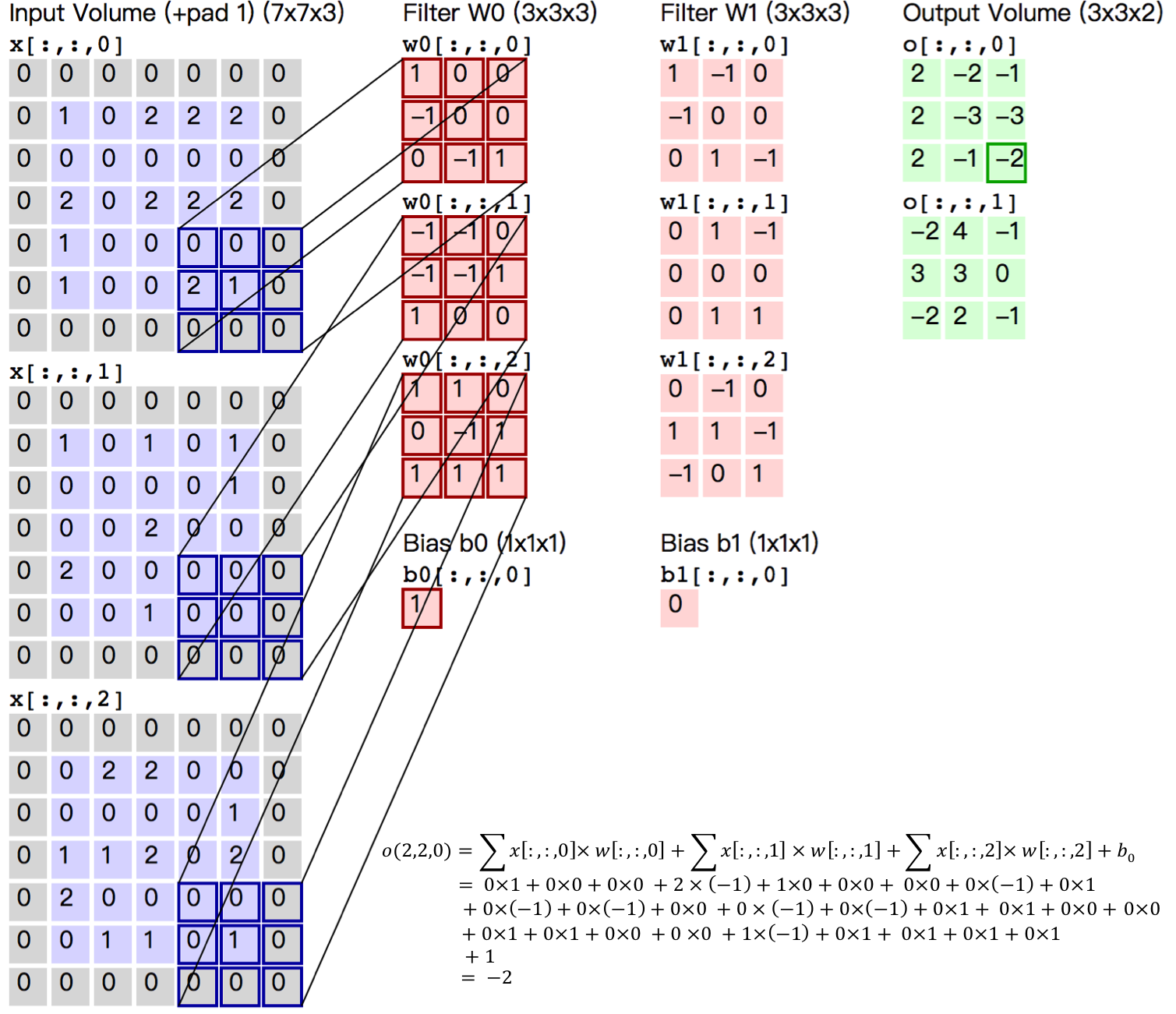
+图5. 卷积层图片
+
+图5给出一个卷积计算过程的示例图,输入图像大小为`$H=5,W=5,D=3$`,即`$5 \times 5$`大小的3通道(RGB,也称作深度)彩色图像。这个示例图中包含两(用`$K$`表示)组卷积核,即图中滤波器`$W_0$`和`$W_1$`。在卷积计算中,通常对不同的输入通道采用不同的卷积核,如图示例中每组卷积核包含(`$D=3$`)个`$3 \times 3$`(用`$F \times F$`表示)大小的卷积核。另外,这个示例中卷积核在图像的水平方向(`$W$`方向)和垂直方向(`$H$`方向)的滑动步长为2(用`$S$`表示);对输入图像周围各填充1(用`$P$`表示)个0,即图中输入层原始数据为蓝色部分,灰色部分是进行了大小为1的扩展,用0来进行扩展。经过卷积操作得到输出为`$3 \times 3 \times 2$`(用`$H_{o} \times W_{o} \times K$`表示)大小的特征图,即`$3 \times 3$`大小的2通道特征图,其中`$H_o$`计算公式为:`$H_o = (H - F + 2 \times P)/S + 1$`,`$W_o$`同理。 而输出特征图中的每个像素,是每组滤波器与输入图像每个特征图的内积再求和,再加上偏置`$b_o$`,偏置通常对于每个输出特征图是共享的。输出特征图`$o[:,:,0]$`中的最后一个`$-2$`计算如图5右下角公式所示。
+
+在卷积操作中卷积核是可学习的参数,经过上面示例介绍,每层卷积的参数大小为`$D \times F \times F \times K$`。在多层感知器模型中,神经元通常是全部连接,参数较多。而卷积层的参数较少,这也是由卷积层的主要特性即局部连接和共享权重所决定。
+
+- 局部连接:每个神经元仅与输入神经元的一块区域连接,这块局部区域称作感受野(receptive field)。在图像卷积操作中,即神经元在空间维度(spatial dimension,即上图示例H和W所在的平面)是局部连接,但在深度上是全部连接。对于二维图像本身而言,也是局部像素关联较强。这种局部连接保证了学习后的过滤器能够对于局部的输入特征有最强的响应。局部连接的思想,也是受启发于生物学里面的视觉系统结构,视觉皮层的神经元就是局部接受信息的。
+
+- 权重共享:计算同一个深度切片的神经元时采用的滤波器是共享的。例如图4中计算`$o[:,:,0]$`的每个每个神经元的滤波器均相同,都为`$W_0$`,这样可以很大程度上减少参数。共享权重在一定程度上讲是有意义的,例如图片的底层边缘特征与特征在图中的具体位置无关。但是在一些场景中是无意的,比如输入的图片是人脸,眼睛和头发位于不同的位置,希望在不同的位置学到不同的特征 (参考[斯坦福大学公开课]( http://cs231n.github.io/convolutional-networks/))。请注意权重只是对于同一深度切片的神经元是共享的,在卷积层,通常采用多组卷积核提取不同特征,即对应不同深度切片的特征,不同深度切片的神经元权重是不共享。另外,偏重对同一深度切片的所有神经元都是共享的。
+
+通过介绍卷积计算过程及其特性,可以看出卷积是线性操作,并具有平移不变性(shift-invariant),平移不变性即在图像每个位置执行相同的操作。卷积层的局部连接和权重共享使得需要学习的参数大大减小,这样也有利于训练较大卷积神经网络。
+
+#### 池化层
+
+
+图6. 池化层图片
+
+池化是非线性下采样的一种形式,主要作用是通过减少网络的参数来减小计算量,并且能够在一定程度上控制过拟合。通常在卷积层的后面会加上一个池化层。池化包括最大池化、平均池化等。其中最大池化是用不重叠的矩形框将输入层分成不同的区域,对于每个矩形框的数取最大值作为输出层,如图6所示。
+
+更详细的关于卷积神经网络的具体知识可以参考[斯坦福大学公开课]( http://cs231n.github.io/convolutional-networks/ )和[图像分类](https://github.com/PaddlePaddle/book/blob/develop/image_classification/README.md)教程。
+
+### 常见激活函数介绍
+- sigmoid激活函数: `$ f(x) = sigmoid(x) = \frac{1}{1+e^{-x}} $`
+
+- tanh激活函数: `$ f(x) = tanh(x) = \frac{e^x-e^{-x}}{e^x+e^{-x}} $`
+
+实际上,tanh函数只是规模变化的sigmoid函数,将sigmoid函数值放大2倍之后再向下平移1个单位:tanh(x) = 2sigmoid(2x) - 1 。
+
+- ReLU激活函数: `$ f(x) = max(0, x) $`
+
+更详细的介绍请参考[维基百科激活函数](https://en.wikipedia.org/wiki/Activation_function)。
+
+## 数据介绍
+
+PaddlePaddle在API中提供了自动加载[MNIST](http://yann.lecun.com/exdb/mnist/)数据的模块`paddle.dataset.mnist`。加载后的数据位于`/home/username/.cache/paddle/dataset/mnist`下:
+
+
+
+
+
+ 文件名称 |
+ 说明 |
+
+
+
+
+
+ train-images-idx3-ubyte |
+ 训练数据图片,60,000条数据 |
+
+
+ train-labels-idx1-ubyte |
+ 训练数据标签,60,000条数据 |
+
+
+ t10k-images-idx3-ubyte |
+ 测试数据图片,10,000条数据 |
+
+
+ t10k-labels-idx1-ubyte |
+ 测试数据标签,10,000条数据 |
+
+
+
+
+
+## Fluid API 概述
+
+演示将使用最新的 `Fluid API`。Fluid API是最新的 PaddlePaddle API。它在不牺牲性能的情况下简化了模型配置。
+我们建议使用 Fluid API,因为它更容易学起来。
+
+下面是快速的 Fluid API 概述。
+1. `inference_program`:指定如何从数据输入中获得预测的函数。
+这是指定网络流的地方。
+
+1. `train_program`:指定如何从 `inference_program` 和`标签值`中获取 `loss` 的函数。
+这是指定损失计算的地方。
+
+1. `optimizer_func`: “指定优化器配置的函数。优化器负责减少损失并驱动培训。Paddle 支持多种不同的优化器。
+
+1. `Trainer`:PaddlePaddle Trainer 管理由 `train_program` 和 `optimizer` 指定的训练过程。
+通过 `event_handler` 回调函数,用户可以监控培训的进展。
+
+1. `Inferencer`:Fluid inferencer 加载 `inference_program` 和由 Trainer 训练的参数。
+然后,它可以推断数据和返回预测。
+
+在这个演示中,我们将深入了解它们。
+
+## 配置说明
+加载 PaddlePaddle 的 Fluid API 包。
+
+```python
+import paddle
+import paddle.fluid as fluid
+```
+
+### Program Functions 配置
+
+我们需要设置“推理程序”函数。我们想用这个程序来演示三个不同的分类器,每个分类器都定义为 Python 函数。
+我们需要将图像数据馈送到分类器。Paddle 为读取数据提供了一个特殊的层 `layer.data` 层。
+让我们创建一个数据层来读取图像并将其连接到分类网络。
+
+- Softmax回归:只通过一层简单的以softmax为激活函数的全连接层,就可以得到分类的结果。
+
+```python
+def softmax_regression():
+img = fluid.layers.data(name='img', shape=[1, 28, 28], dtype='float32')
+predict = fluid.layers.fc(
+input=img, size=10, act='softmax')
+return predict
+```
+
+- 多层感知器:下面代码实现了一个含有两个隐藏层(即全连接层)的多层感知器。其中两个隐藏层的激活函数均采用ReLU,输出层的激活函数用Softmax。
+
+```python
+def multilayer_perceptron():
+img = fluid.layers.data(name='img', shape=[1, 28, 28], dtype='float32')
+# 第一个全连接层,激活函数为ReLU
+hidden = fluid.layers.fc(input=img, size=200, act='relu')
+# 第二个全连接层,激活函数为ReLU
+hidden = fluid.layers.fc(input=hidden, size=200, act='relu')
+# 以softmax为激活函数的全连接输出层,输出层的大小必须为数字的个数10
+prediction = fluid.layers.fc(input=hidden, size=10, act='softmax')
+return prediction
+```
+
+- 卷积神经网络LeNet-5: 输入的二维图像,首先经过两次卷积层到池化层,再经过全连接层,最后使用以softmax为激活函数的全连接层作为输出层。
+
+```python
+def convolutional_neural_network():
+img = fluid.layers.data(name='img', shape=[1, 28, 28], dtype='float32')
+# 第一个卷积-池化层
+conv_pool_1 = fluid.nets.simple_img_conv_pool(
+input=img,
+filter_size=5,
+num_filters=20,
+pool_size=2,
+pool_stride=2,
+act="relu")
+conv_pool_1 = fluid.layers.batch_norm(conv_pool_1)
+# 第二个卷积-池化层
+conv_pool_2 = fluid.nets.simple_img_conv_pool(
+input=conv_pool_1,
+filter_size=5,
+num_filters=50,
+pool_size=2,
+pool_stride=2,
+act="relu")
+# 以softmax为激活函数的全连接输出层,输出层的大小必须为数字的个数10
+prediction = fluid.layers.fc(input=conv_pool_2, size=10, act='softmax')
+return prediction
+```
+
+#### Train Program 配置
+然后我们需要设置训练程序 `train_program`。它首先从分类器中进行预测。
+在训练期间,它将从预测中计算 `avg_cost`。
+
+**注意:** 训练程序应该返回一个数组,第一个返回参数必须是 `avg_cost`。训练器使用它来计算梯度。
+
+请随意修改代码,测试 Softmax 回归 `softmax_regression`, `MLP` 和 卷积神经网络 `convolutional neural network` 分类器之间的不同结果。
+
+```python
+def train_program():
+label = fluid.layers.data(name='label', shape=[1], dtype='int64')
+
+# predict = softmax_regression() # uncomment for Softmax回归
+# predict = multilayer_perceptron() # uncomment for 多层感知器
+predict = convolutional_neural_network() # uncomment for LeNet5卷积神经网络
+cost = fluid.layers.cross_entropy(input=predict, label=label)
+avg_cost = fluid.layers.mean(cost)
+acc = fluid.layers.accuracy(input=predict, label=label)
+return [avg_cost, acc]
+
+
+# 该模型运行在单个CPU上
+```
+
+#### Optimizer Function 配置
+
+在下面的 `Adam optimizer`,`learning_rate` 是训练的速度,与网络的训练收敛速度有关系。
+
+```python
+def optimizer_program():
+return fluid.optimizer.Adam(learning_rate=0.001)
+```
+
+### 数据集 Feeders 配置
+
+下一步,我们开始训练过程。`paddle.dataset.movielens.train()`和`paddle.dataset.movielens.test()`分别做训练和测试数据集。这两个函数各自返回一个reader——PaddlePaddle中的reader是一个Python函数,每次调用的时候返回一个Python yield generator。
+
+下面`shuffle`是一个reader decorator,它接受一个reader A,返回另一个reader B —— reader B 每次读入`buffer_size`条训练数据到一个buffer里,然后随机打乱其顺序,并且逐条输出。
+
+`batch`是一个特殊的decorator,它的输入是一个reader,输出是一个batched reader —— 在PaddlePaddle里,一个reader每次yield一条训练数据,而一个batched reader每次yield一个minibatch。
+
+```python
+train_reader = paddle.batch(
+paddle.reader.shuffle(
+paddle.dataset.mnist.train(), buf_size=500),
+batch_size=64)
+
+test_reader = paddle.batch(
+paddle.dataset.mnist.test(), batch_size=64)
+```
+
+### Trainer 配置
+
+现在,我们需要配置 `Trainer`。`Trainer` 需要接受训练程序 `train_program`, `place` 和优化器 `optimizer`。
+
+```python
+# 该模型运行在单个CPU上
+use_cuda = False # set to True if training with GPU
+place = fluid.CUDAPlace(0) if use_cuda else fluid.CPUPlace()
+
+trainer = fluid.Trainer(
+train_func=train_program, place=place, optimizer_func=optimizer_program)
+```
+
+#### Event Handler 配置
+
+Fluid API 在训练期间为回调函数提供了一个钩子。用户能够通过机制监控培训进度。
+我们将在这里演示两个 `event_handler` 程序。请随意修改 Jupyter 笔记本 ,看看有什么不同。
+
+`event_handler` 用来在训练过程中输出训练结果
+
+```python
+# Save the parameter into a directory. The Inferencer can load the parameters from it to do infer
+params_dirname = "recognize_digits_network.inference.model"
+lists = []
+def event_handler(event):
+if isinstance(event, fluid.EndStepEvent):
+if event.step % 100 == 0:
+# event.metrics maps with train program return arguments.
+# event.metrics[0] will yeild avg_cost and event.metrics[1] will yeild acc in this example.
+print "Pass %d, Batch %d, Cost %f" % (
+event.step, event.epoch, event.metrics[0])
+
+if isinstance(event, fluid.EndEpochEvent):
+avg_cost, acc = trainer.test(
+reader=test_reader, feed_order=['img', 'label'])
+
+print("Test with Epoch %d, avg_cost: %s, acc: %s" % (event.epoch, avg_cost, acc))
+
+# save parameters
+trainer.save_params(params_dirname)
+lists.append((event.epoch, avg_cost, acc))
+```
+
+`event_handler_plot` 可以用来在训练过程中画图如下:
+
+
+
+```python
+from paddle.v2.plot import Ploter
+
+train_title = "Train cost"
+test_title = "Test cost"
+cost_ploter = Ploter(train_title, test_title)
+step = 0
+lists = []
+
+# event_handler to plot a figure
+def event_handler_plot(event):
+global step
+if isinstance(event, fluid.EndStepEvent):
+if step % 100 == 0:
+# event.metrics maps with train program return arguments.
+# event.metrics[0] will yeild avg_cost and event.metrics[1] will yeild acc in this example.
+cost_ploter.append(train_title, step, event.metrics[0])
+cost_ploter.plot()
+step += 1
+if isinstance(event, fluid.EndEpochEvent):
+# save parameters
+trainer.save_params(params_dirname)
+
+avg_cost, acc = trainer.test(
+reader=test_reader, feed_order=['img', 'label'])
+cost_ploter.append(test_title, step, avg_cost)
+lists.append((event.epoch, avg_cost, acc))
+```
+
+#### 开始训练
+
+既然我们设置了 `event_handler` 和 `data reader`,我们就可以开始训练模型了。
+
+`feed_order` 用于将数据目录映射到 `train_program`
+
+```python
+trainer.train(
+num_epochs=5,
+event_handler=event_handler,
+reader=train_reader,
+feed_order=['img', 'label'])
+```
+
+训练过程是完全自动的,event_handler里打印的日志类似如下所示:
+
+```
+Pass 0, Batch 0, Cost 0.125650
+Pass 100, Batch 0, Cost 0.161387
+Pass 200, Batch 0, Cost 0.040036
+Pass 300, Batch 0, Cost 0.023391
+Pass 400, Batch 0, Cost 0.005856
+Pass 500, Batch 0, Cost 0.003315
+Pass 600, Batch 0, Cost 0.009977
+Pass 700, Batch 0, Cost 0.020959
+Pass 800, Batch 0, Cost 0.105560
+Pass 900, Batch 0, Cost 0.239809
+Test with Epoch 0, avg_cost: 0.053097883707459624, acc: 0.9822850318471338
+```
+
+训练之后,检查模型的预测准确度。用 MNIST 训练的时候,一般 softmax回归模型的分类准确率为约为 92.34%,多层感知器为97.66%,卷积神经网络可以达到 99.20%。
+
+
+## 应用模型
+
+可以使用训练好的模型对手写体数字图片进行分类,下面程序展示了如何使用 `fluid.Inferencer` 接口进行推断。
+
+### Inference 配置
+
+`Inference` 需要一个 `infer_func` 和 `param_path` 来设置网络和经过训练的参数。
+我们可以简单地插入在此之前定义的分类器。
+
+```python
+inferencer = fluid.Inferencer(
+# infer_func=softmax_regression, # uncomment for softmax regression
+# infer_func=multilayer_perceptron, # uncomment for MLP
+infer_func=convolutional_neural_network, # uncomment for LeNet5
+param_path=params_dirname,
+place=place)
+```
+
+### 生成预测输入数据
+
+`infer_3.png` 是数字 3 的一个示例图像。把它变成一个 numpy 数组以匹配数据馈送格式。
+
+```python
+# Prepare the test image
+import os
+import numpy as np
+from PIL import Image
+def load_image(file):
+im = Image.open(file).convert('L')
+im = im.resize((28, 28), Image.ANTIALIAS)
+im = np.array(im).reshape(1, 1, 28, 28).astype(np.float32)
+im = im / 255.0 * 2.0 - 1.0
+return im
+
+cur_dir = cur_dir = os.getcwd()
+img = load_image(cur_dir + '/image/infer_3.png')
+```
+
+### 预测
+
+现在我们准备做预测。
+
+```python
+results = inferencer.infer({'img': img})
+lab = np.argsort(results) # probs and lab are the results of one batch data
+print "Label of image/infer_3.png is: %d" % lab[0][0][-1]
+```
+
+## 总结
+
+本教程的softmax回归、多层感知器和卷积神经网络是最基础的深度学习模型,后续章节中复杂的神经网络都是从它们衍生出来的,因此这几个模型对之后的学习大有裨益。同时,我们也观察到从最简单的softmax回归变换到稍复杂的卷积神经网络的时候,MNIST数据集上的识别准确率有了大幅度的提升,原因是卷积层具有局部连接和共享权重的特性。在之后学习新模型的时候,希望大家也要深入到新模型相比原模型带来效果提升的关键之处。此外,本教程还介绍了PaddlePaddle模型搭建的基本流程,从dataprovider的编写、网络层的构建,到最后的训练和预测。对这个流程熟悉以后,大家就可以用自己的数据,定义自己的网络模型,并完成自己的训练和预测任务了。
+
+## 参考文献
+
+1. LeCun, Yann, Léon Bottou, Yoshua Bengio, and Patrick Haffner. ["Gradient-based learning applied to document recognition."](http://ieeexplore.ieee.org/abstract/document/726791/) Proceedings of the IEEE 86, no. 11 (1998): 2278-2324.
+2. Wejéus, Samuel. ["A Neural Network Approach to Arbitrary SymbolRecognition on Modern Smartphones."](http://www.diva-portal.org/smash/record.jsf?pid=diva2%3A753279&dswid=-434) (2014).
+3. Decoste, Dennis, and Bernhard Schölkopf. ["Training invariant support vector machines."](http://link.springer.com/article/10.1023/A:1012454411458) Machine learning 46, no. 1-3 (2002): 161-190.
+4. Simard, Patrice Y., David Steinkraus, and John C. Platt. ["Best Practices for Convolutional Neural Networks Applied to Visual Document Analysis."](http://citeseerx.ist.psu.edu/viewdoc/download?doi=10.1.1.160.8494&rep=rep1&type=pdf) In ICDAR, vol. 3, pp. 958-962. 2003.
+5. Salakhutdinov, Ruslan, and Geoffrey E. Hinton. ["Learning a Nonlinear Embedding by Preserving Class Neighbourhood Structure."](http://www.jmlr.org/proceedings/papers/v2/salakhutdinov07a/salakhutdinov07a.pdf) In AISTATS, vol. 11. 2007.
+6. Cireşan, Dan Claudiu, Ueli Meier, Luca Maria Gambardella, and Jürgen Schmidhuber. ["Deep, big, simple neural nets for handwritten digit recognition."](http://www.mitpressjournals.org/doi/abs/10.1162/NECO_a_00052) Neural computation 22, no. 12 (2010): 3207-3220.
+7. Deng, Li, Michael L. Seltzer, Dong Yu, Alex Acero, Abdel-rahman Mohamed, and Geoffrey E. Hinton. ["Binary coding of speech spectrograms using a deep auto-encoder."](http://citeseerx.ist.psu.edu/viewdoc/download?doi=10.1.1.185.1908&rep=rep1&type=pdf) In Interspeech, pp. 1692-1695. 2010.
+8. Kégl, Balázs, and Róbert Busa-Fekete. ["Boosting products of base classifiers."](http://dl.acm.org/citation.cfm?id=1553439) In Proceedings of the 26th Annual International Conference on Machine Learning, pp. 497-504. ACM, 2009.
+9. Rosenblatt, Frank. ["The perceptron: A probabilistic model for information storage and organization in the brain."](http://psycnet.apa.org/journals/rev/65/6/386/) Psychological review 65, no. 6 (1958): 386.
+10. Bishop, Christopher M. ["Pattern recognition."](http://users.isr.ist.utl.pt/~wurmd/Livros/school/Bishop%20-%20Pattern%20Recognition%20And%20Machine%20Learning%20-%20Springer%20%202006.pdf) Machine Learning 128 (2006): 1-58.
+
+
+
本教程 由 PaddlePaddle 创作,采用 知识共享 署名-相同方式共享 4.0 国际 许可协议进行许可。
diff --git a/doc/fluid/new_docs/beginners_guide/quick_start/recognize_digits/image/cnn.png b/doc/fluid/new_docs/beginners_guide/quick_start/recognize_digits/image/cnn.png
new file mode 100644
index 0000000000000000000000000000000000000000..3f5cdaacdc6acce41c5c6c99649be46685cf9903
Binary files /dev/null and b/doc/fluid/new_docs/beginners_guide/quick_start/recognize_digits/image/cnn.png differ
diff --git a/doc/fluid/new_docs/beginners_guide/quick_start/recognize_digits/image/cnn_train_log.png b/doc/fluid/new_docs/beginners_guide/quick_start/recognize_digits/image/cnn_train_log.png
new file mode 100644
index 0000000000000000000000000000000000000000..65bd17eacd41bbdbdb042bd1ba366eb53663b410
Binary files /dev/null and b/doc/fluid/new_docs/beginners_guide/quick_start/recognize_digits/image/cnn_train_log.png differ
diff --git a/doc/fluid/new_docs/beginners_guide/quick_start/recognize_digits/image/infer_3.png b/doc/fluid/new_docs/beginners_guide/quick_start/recognize_digits/image/infer_3.png
new file mode 100644
index 0000000000000000000000000000000000000000..030cd60d3b4af9aecd4941204da4ad15f6e1189f
Binary files /dev/null and b/doc/fluid/new_docs/beginners_guide/quick_start/recognize_digits/image/infer_3.png differ
diff --git a/doc/fluid/new_docs/beginners_guide/quick_start/recognize_digits/image/max_pooling.png b/doc/fluid/new_docs/beginners_guide/quick_start/recognize_digits/image/max_pooling.png
new file mode 100644
index 0000000000000000000000000000000000000000..90b02fa2a735cfcc9efb2de90906325dedcb358c
Binary files /dev/null and b/doc/fluid/new_docs/beginners_guide/quick_start/recognize_digits/image/max_pooling.png differ
diff --git a/doc/fluid/new_docs/beginners_guide/quick_start/recognize_digits/image/mlp.png b/doc/fluid/new_docs/beginners_guide/quick_start/recognize_digits/image/mlp.png
new file mode 100644
index 0000000000000000000000000000000000000000..9f4d26cd8da32201d0a5e9c72d466301dd2b42a1
Binary files /dev/null and b/doc/fluid/new_docs/beginners_guide/quick_start/recognize_digits/image/mlp.png differ
diff --git a/doc/fluid/new_docs/beginners_guide/quick_start/recognize_digits/image/mlp_train_log.png b/doc/fluid/new_docs/beginners_guide/quick_start/recognize_digits/image/mlp_train_log.png
new file mode 100644
index 0000000000000000000000000000000000000000..f5a478fdc24f29c17555a2f1451f3f5a079faed9
Binary files /dev/null and b/doc/fluid/new_docs/beginners_guide/quick_start/recognize_digits/image/mlp_train_log.png differ
diff --git a/doc/fluid/new_docs/beginners_guide/quick_start/recognize_digits/image/mnist_example_image.png b/doc/fluid/new_docs/beginners_guide/quick_start/recognize_digits/image/mnist_example_image.png
new file mode 100644
index 0000000000000000000000000000000000000000..4edd7cabf8a2282f6392ac1421c7ca4afb288589
Binary files /dev/null and b/doc/fluid/new_docs/beginners_guide/quick_start/recognize_digits/image/mnist_example_image.png differ
diff --git a/doc/fluid/new_docs/beginners_guide/quick_start/recognize_digits/image/softmax_regression.png b/doc/fluid/new_docs/beginners_guide/quick_start/recognize_digits/image/softmax_regression.png
new file mode 100644
index 0000000000000000000000000000000000000000..40b98298288b9c406fce1cbca9c913753020a94d
Binary files /dev/null and b/doc/fluid/new_docs/beginners_guide/quick_start/recognize_digits/image/softmax_regression.png differ
diff --git a/doc/fluid/new_docs/beginners_guide/quick_start/recognize_digits/image/softmax_train_log.png b/doc/fluid/new_docs/beginners_guide/quick_start/recognize_digits/image/softmax_train_log.png
new file mode 100644
index 0000000000000000000000000000000000000000..47204941af7f22e68386a70a06ec4f122b83e262
Binary files /dev/null and b/doc/fluid/new_docs/beginners_guide/quick_start/recognize_digits/image/softmax_train_log.png differ
diff --git a/doc/fluid/new_docs/beginners_guide/quick_start/recognize_digits/image/train_and_test.png b/doc/fluid/new_docs/beginners_guide/quick_start/recognize_digits/image/train_and_test.png
new file mode 100644
index 0000000000000000000000000000000000000000..5cb87b450d0398bcfaec0e647c362052069797e7
Binary files /dev/null and b/doc/fluid/new_docs/beginners_guide/quick_start/recognize_digits/image/train_and_test.png differ
diff --git a/doc/fluid/new_docs/faq/faq.rst b/doc/fluid/new_docs/faq/faq.rst
new file mode 100644
index 0000000000000000000000000000000000000000..3b4bd4f895162fa3b0ba12e785e38ad694590b25
--- /dev/null
+++ b/doc/fluid/new_docs/faq/faq.rst
@@ -0,0 +1,12 @@
+###################
+编译安装与单元测试
+###################
+
+1. 通过pip安装的PaddlePaddle在 :code:`import paddle.fluid` 报找不到 :code:`libmkldnn.so` 或 :code:`libmklml_intel.so`
+------------------------------------------------------------------------------------------
+出现这种问题的原因是在导入 :code:`paddle.fluid` 时需要加载 :code:`libmkldnn.so` 和 :code:`libmklml_intel.so`,
+但是系统没有找到该文件。一般通过pip安装PaddlePaddle时会将 :code:`libmkldnn.so` 和 :code:`libmklml_intel.so`
+拷贝到 :code:`/usr/local/lib` 路径下,所以解决办法是将该路径加到 :code:`LD_LIBRARY_PATH` 环境变量下,
+即: :code:`export LD_LIBRARY_PATH=/usr/local/lib:$LD_LIBRARY_PATH` 。
+
+**注意**:如果是在虚拟环境中安装PaddlePaddle, :code:`libmkldnn.so` 和 :code:`libmklml_intel.so` 可能不在 :code:`/usr/local/lib` 路径下。
diff --git a/doc/fluid/new_docs/faq/index_cn.rst b/doc/fluid/new_docs/faq/index_cn.rst
new file mode 100644
index 0000000000000000000000000000000000000000..bb2ed99217609d3a9edd179d4f98ad5b8b649860
--- /dev/null
+++ b/doc/fluid/new_docs/faq/index_cn.rst
@@ -0,0 +1,9 @@
+FAQ
+====
+
+本文档对关于PaddlePaddle的一些常见问题提供了解答。如果您的问题未在此处,请您到 `PaddlePaddle社区 `_ 查找答案或直接提 `issue `_ ,我们会及时进行回复。
+
+.. toctree::
+ :maxdepth: 1
+
+ faq.rst
diff --git a/doc/fluid/new_docs/user_guides/howto/basic_concept/fluid_basic_concept.rst b/doc/fluid/new_docs/user_guides/howto/basic_concept/fluid_basic_concept.rst
new file mode 100644
index 0000000000000000000000000000000000000000..55c3c761f932713ffa2b462b35f9f46a8edae536
--- /dev/null
+++ b/doc/fluid/new_docs/user_guides/howto/basic_concept/fluid_basic_concept.rst
@@ -0,0 +1,392 @@
+================================
+PaddleFluid设计思想和基本使用概念
+================================
+
+
+
+Paddle Fluid 是用来让用户像 PyTorch 和 Tensorflow Eager Execution 一样执行程序。
+在这些系统中,不再有模型这个概念,应用也不再包含一个用于描述 Operator 图或者一系列层的符号描述,
+而是像通用程序那样描述训练或者预测的过程。
+
+
+深度学习平台的演化
+================
+
+时至今日,深度学习已成为事实上最流行的机器学习技术。学术界多年研究加上工业界的长期实践提出了若干有效的基本建模单元:
+全连接,卷积,循环神经网络等;设计各类训练技巧:初始化方法,跨层连接,各类 norm 技术等;
+发明了各种新的优化算法:Adadelta,Adam 等;
+各类固定的网络结构:highway, residual, attention 等纷纷涌现,不胜枚举。
+学术界工业界多年的付出共同促成了深度学习方法今日的影响力。
+
+学术研究和生产实践中积累了大量的知识,能够很好的解释神经网络中基本模块各自独的学习能力和特性。
+基本模块和训练技术的组合能够搭建出千变万化的神经网络模型。
+基本模块和训练技术是有限的,但他们的组合却是千变万化,这是深度学习方法的魅力所在,也是难度所在。
+
+正是这样高度的模块化特性,研究者和工程师们都在努力避免重复造轮子以提高研究和生产的效率,
+又进一步催生了深度学习平台技术的发展,深度学习框架已演变成为 AI 基础设施中重要的一部分。
+从 Theano,到 DistBelief,到 TensorFlow;从 Caffe 到 Caffe2;
+从 Torch 到 PyTorch;从 PaddlePaddle 到 PaddleFluid,
+深度学习平台技术也经历了两代的演化,并向着第三代平台技术迈进。
+
+站在历史发展的今天,当我们准备切换尝试使用一个新的深度学习平台作为支持自己学习和研究的工具时,
+平台技术都发生了哪些演化,能够为我们的带来什么便利呢?
+
+先让我们来看看深度学习框架解决的三大问题:
+
+- 如何描述计算以支持未来潜在会出现的新模型?
+- 如何高效利用异构设备最大化算力?
+- 如何利用网络中的计算机进行分布式计算来处理千万亿级别的数据?
+
+以上三个问题中的第一个和使用者研究者最为密切相关。
+这篇文章我们通过分析 PaddleFluid的设计理念,
+来了解一个深度学习框架如何抽象深度学习模型,来看看我们的使用经验如何在不同深度学习平台之间过度和迁移。
+
+如何描述计算
+=============
+
+让我们首先来看看 PaddleFluid 如何描述机器学习模型
+
+
+PaddleFluid之 :code:`Program`
+
+如何描述计算很大程度决定了一个神经网络框架计算功能的完备性。
+深度学习模型和方法历经二十多年的发展:“依次执行一组计算的前向,
+再以和前向计算相反的顺序执行反向计算,中间无分支无交互”,
+这样的模型结构已经无法满足研究者和千千万万框架使用者的想象力。
+
+从 `PaddleFluid 的设计目标 `_ 来看,
+在如何描述机器学习模型这一核心问题上,PaddleFluid 的目标是:
+创造一种新的计算描述方式,不但能够描述至今为止人们已知的主流神经网络模型,并且能够支持未来会出现的任意模型。
+
+PaddleFluid 是如何做到支持未来出现的新模型这一目标呢?PaddleFluid 的设计选择是:
+对用户来说,用一段 :code:`Program` (在 PaddleFluid 内部会被转化为一种叫作 :code:`ProgramDesc` 的描述语言),
+而不是用计算图来描述机器学习模型。 :code:`Program` 用符合用户使用直觉的方式,
+提供一种新的描述语言能够描述任意复杂的机器学习模型。
+
+对所有计算机专业同学学习编程语言的第一课一定是建立对“程序语言的三种执行结构:顺序执行,条件选择和循环执行”的认识。
+计算机世界的所有可计算逻辑都是由这三种执行结构表示,用这三种结构描述的逻辑是可计算的。那么同样道理,
+对一个神经网络框架来说,如果可以和程序语言一样提供对这三种执行结构的支持,那么将可以描述任意复杂的,
+可被计算机计算的机器学习模型。PaddleFluid通过提供对这三种执行结构的支持,来做到对任意复杂模型的描述。
+
+具体来说:
+
+1. Fluid 的核心设计理念都可以类比到程序语言,如果已经有写程序的经验,那么使用 Fluid 构建神经网络模型的体验,将非常接近写程序;
+
+2. 在 PaddleFluid 中,用户不会显示地感知“计算图”这样的概念,一个机器学习模型被描述为一个 Fluid :code:`Program` (Fluid 内部称之为 :code:`ProgramDesc` );
+
+- 一个 Fluid :code:`Program` 由一组嵌套的 :code:`Block` 构成。 :code:`Block` 的概念可以类比到 C++ 或是 Java 中的一对大括号,或是 Python 语言中的一个缩进快;
+- :code:`Block` 中的计算由顺序执行、条件选择或者循环执行三种方式组合,构成复杂的计算逻辑。
+
+3. Fluid :code:`Program` 中包含对计算和计算对象的描述。计算的描述称之为 Operator;计算作用的对象(或者说 Operator 的输入和输出)被统一为 Tensor。
+
+在描述计算和计算的作用对象这一问题上,各个深度学习框架的选择是相同的,如果有一个平台的使用经验,那么将非常容易在各个平台之间进行迁移。
+
+核心使用概念
+=============
+
+下面,我们将更详细地了解核心使用概念在PaddlePaddle的使用方法。
+
+数据表示和计算的对象:Tensor
+--------------------------
+
+Tensor 是向量矩阵概念的扩展,是神经网络模型计算操作的基本对象。这在是今天所有主流深度学习平台的共同选择。
+
+可以简单地将 Tensor 理解为一个 N 维向量,它可以有任意多的维度。一个 Tensor 具有两个基本特征:
+
+1. 数据类型:每个 Tensor 的所有元素具有同样的、已知的数据类型;
+
+2. 大小(或者说形状):即维度的个数(rank,阶)以及各维度的长度。
+
+Tensor 某些维度的长度在定义模型阶段可能是未知的,在实际算法执行时才能确定。例如一个 mini-batch 中包含的样本数目(batch size),或者是一个 mini-batch 中序列的最大长度。
+
+PaddleFluid中的Tensor
+""""""""""""""""""""""
+
+PaddleFluid 中也使用 Tensor 作为神经网络中输入输出数据的统一表示。Tensor 的概念在今天主流的深度学习平台中都是完全相同,可以在各个深度学习框架之间直接无缝迁移。
+
+在 Fluid 中也同样存在三种特殊的 Tensor:
+
+1. 模型中的可学习参数
+
+模型中的可学习参数生存期和整个训练任务一样长,会接受优化算法的更新。在 PaddleFluid 中同样以 :code:`Variable` 表示;
+用户在绝大多数情况下都不需要自己来创建网络中的可学习参数,Fluid 为几乎常见的神经网络基本计算模块都提供了封装。
+以最简单的全连接模型为例,下面的代码片段会直接为全连接层创建连接权值 WW 和偏置( :code:`bias` )两个可学习参数,
+无需显示地调用 variable 相关接口创建可学习参数。
+
+
+::
+
+ import paddle.fluid as fluid
+
+ y = fluid.layers.fc(input=x, size=128, bias_attr=True)
+
+2. 输入输出Tensor
+
+整个神经网络的输入数据也是一个特殊的 Tensor,在这个 Tensor 中,
+一些维度的大小在定义模型时无法确定(通常包括:batch size;
+如果 mini-batch 之间,数据可变,也会包括序列的最大长度,图片的宽度和高度等),在定义模型时需要占位;
+PaddleFluid 中使用 :code:`fluid.layers.data` 来接入输入数据, :code:`fluid.layer.data` 需要提供输入 Tensor 的 形状信息,
+当遇到无法确定的维度 时, 相应维度指定为 None ,如下面的代码片段所示:
+
+::
+
+ import paddle.fluid as fluid
+
+ x = fluid.layers.data(name="x", shape=[2, None, 3], dtype="int64")
+
+3. 常量 Tensor 在 PaddleFluid 中需要通过组合 Tensor 和 :code:`fluid.layers.assign` 来实现。
+
+
+计算原语:Operation/Operator
+----------------------------
+
+Tensor 是今天所有主流深度学习框架的统一数据表示(输入、输出、中间计算结果、模型的可学习参数都是 Tensor)。
+另一方面,对数据的操作,在主流深度学习框架中也高度统一为:Operator/Operation。
+在中文中,通常我们会习惯将其称之为算子。
+
+注:在 PaddleFluid 中使用 Operator 称呼对 Tensor 的操作。
+
+Operation/Operator 接受多个 Tensor 作为输入,输出若干个 Tensor,表示了从输入到输出的变化。
+
+PaddleFluid中的Operator
+""""""""""""""""""""""""
+
+PaddleFluid 支持的所有算子,可以在 `API 帮助文档 `_ 中查看。
+
+为了便于用户使用,在 Python 端,Fluid 中的 Operator 被进一步封装入 :code:`paddle.fluid.layers` ,
+:code:`paddle.fluid.networks` 等模块。这是因为:一些常见的对Tensor的操作可能是有更多基础操作构成,
+例如:l2 norm 内部由 reduce、elementwise_add,scale 等多个 Operator 组合计算逻辑完成,
+为了提高使用的便利性,框架内部对基础 Operator 进行了一些封装,包括创建 Operator 依赖可学习参数,
+可学习参数的初始化细节等,减少用户重复开发的成本。
+
+对所有深度学习框架都面临同样的封装,在绝大多数情况下,用户很少会直接与框架底层的 Operator 直接打交道,而是使用框架提供的 layers,networks 等模块,降低开发的代码量。不论是什么样的概念,他们在各框架之间的本质和作用都是相同的:对 Tensor 的变换。
+
+总结
+>>>>>>
+
+不论叫作 Operation、Operator 还是 layers,他们在各深度学习平台中的含义和作用都是相同的:对 Tensor 的变换。是一个深度学习平台提供的基础计算能力。可以在每个平台各自的 API 帮助文档中查到。
+
+在各个深度学习平台都已加入 ONNX 项目的今天,每个深度学习平台提供给大家的基本算子都已趋同,与此同时,每个平台也各有其特点,会提供一些独特的算子,方便某一类任务的开发。
+
+构建模型并执行
+--------------
+
+整个训练任务运行方法如下:
+
+Fluid中的Program和Executor
+"""""""""""""""""""""""""""
+
+1. Fluid 使用 :code:`Program` 描述神经网络模型,对用户来说,并没有计算图的概念。
+用户定义的所有 Tensor 以及对 Tensor 的操作:Operator 都会被加入一段 :code:`Program` 中;
+
+一段 Program 由嵌套的 :code:`Block` 构成,但用户无需显示地创建 :code:`Block` 或是显示地注意到 :code:`Block` 的存在;
+在 Fluid 程序中, :code:`Block` 是在调用 :code:`while_op` , :code:`if_op` , :code:`parallel_do` 等特殊 :code:`Operator` 时,由这些 :code:`Operator` 来创建;
+对用户使用来说,只需要知道自己正在向一段 Fluid Program 中添加变量( :code:`Tensor` )和操作( :code:`Operator` )即可。
+
+2. Fluid 利用 :code:`Executor` 来执行一段 Fluid :code:`Program` 。
+
+为进一步理解 Fluid 中 :code:`Executor` 的作用,需要先解释一下 Fluid 程序的执行流程。 下图展示单机上,Fluid 程序的执行流程:
+
+.. figure:: fluid_local_train.jpeg
+
+ :scale: 50%
+ :align: center
+
+ Figure.1
+
+ Fluid本地训练任务执行流程图
+
+1. Fluid 设计思想和灵感非常类似于程序设计语言,和高级编译语言 C++/Java 编写程序的过程非常类似,Fluid 程序执行分为两个重要阶段:编译时和运行时;
+
+2. 编译期,用户通过调用 Fluid 提供的算子,向一段 :code:`Program` 中添加变量(Tensor)以及对变量的操作(Operators 或者 Layers)。用户只需要描述核心的前向计算,不需要关心反向计算,分布式下,异构设备下如何计算;
+
+3. 原始的 :code:`Program` 在平台内部转换为中间描述语言: :code:`ProgramDesc` ;
+
+4. 编译期最重要的一个功能模块是 Transpiler。Transpiler 接受一段 :code:`ProgramDesc` ,输出一段变化后的 :code:`ProgramDesc` ,作为后端 Executor 最终需要执行的 :code:`Fluid Program` ;
+
+最为常用的 Transipler 包括:
+
+1. 内存优化 Transipler:通过对变量读写依赖关系分析,插入内存回收 Operator 以维持运行过程中较小的内存开销;
+
+2. 分布式环境下的 Transpiler:接受用户定义的 local Program ,生成 Parameter Client 和 Parameter Server 执行的两段 :code:`Program` 。
+
+3. 后端 Executor 接受 Transpiler 输出的这段 :code:`Program` ,依次执行其中的 Operator(可以类比为程序语言中的指令),在执行过程中会为 Operator 创建所需的输入输出并进行管理。
+
+从上面的过程中可以看到,Fluid 程序的执行过程分为:编译器的定义 :code:`Program` ,和创建 :code:`Executor` 运行 :code:`Program` 。
+ :code:`Executor` 执行一段 :code:`Program` 的过程是不可交互和不可中断的。
+
+在 Fluid 中,可以创建多余一段 :code:`Program` 。默认情况,一个 PaddleFluid 程序中存在 2 段 Program:
+
+1. :code:`fluid.framework.default_startup_program` :其中定义了创建模型参数,输入输出,以及模型中可学习参数的初始化等各种操作;
+
+- :code:`default_startup_program` 可以由框架自动生成,使用时无需显示地创建;
+- 如果调用修改了参数的默认初始化方式,框架会自动的将相关的修改加入 :code:`default_startup_program` 。
+
+2. :code:`fluid.framework.default_main_program` :定义了神经网络模型,前向反向计算,以及优化算法对网络中可学习参数的更新;
+
+- 使用 Fluid 的核心就是构建起 :code:`default_main_program` 。
+
+3. PaddleFluid 中的 :code:`Scope` 类似于 TensorFlow 中的 collection 这一概念,但在 Fluid 中 :code:`Scope` 是框架后端概念,用户无法直接操作。因此,在使用框架时无需关心。
+
+总结
+"""""
+
+Fluid 中通过 Executor 来执行一段用户定义的 Fluid :code:`Program` 。
+1. Executor 连接了 Fluid 的前端和后端;
+
+2. Executor 接受用户定义的原始模型(一段 :code:`Program` ),通过调用系统中不同功能更的 :code:`Transpiler` 完成对原始 :code:`Program` 的变化,进行优化。
+
+完整实例:如何完成一个机器学习模型的训练
+===================================
+
+
+
+这一节,我们以 MNIST 手写数字识别问题 —— 机器学习任务的“Hello World”问题和数据,为例,通过一个可以运行的完整实例,来学习上文介绍的概念如何在PaddleFluid 平台使用。
+
+步骤1:定义数据
+----------------
+
+PaddleFluid 中以 :code:`fluid.layers.data` 来接收输入数据。
+
+::
+
+ import numpy as np
+
+ import paddle.fluid as fluid
+ import paddle.v2 as paddle
+
+ # define the input layers for the network.
+ x = fluid.layers.data(name="img", shape=[1, 28, 28], dtype="float32")
+ y_ = fluid.layers.data(name="label", shape=[1], dtype="int64")
+
+Fluid 中 Tensor 的第 0 维度固定为 batch size。在上面代码段中,图像输入 :code:`x` 的形状为:[1, 28, 28]。这三个维度的含义分别是:channel 数目,图像的高度和宽度。
+
+实际上 Fluid 框架内部,一幅图像输入是一个 4-D Tensor,所有 Tensor 的第 0 维固定为 batch size。框架内部会自动为batch size进行填充占位。无需对batch size指定填充占位。
+
+如果除去 batch size(第 0 维度)外,如果 Tensor 某一维度的大小只能在运行时确定,可以在该位置上直接指定 :code:`None` 进行占位。
+
+步骤2:定义模型
+--------------
+
+通过调用 Fluid 提供的算子定义含有一个隐层的神经网络。Fluid 模型的分为模型结构和优化方法两部分。这一点与 TensorFlow 程序十分相似似,使用概念可以直接对应进行迁移。
+
+::
+
+ # define the network topology.
+ y = fluid.layers.fc(input=x, size=10, act="softmax")
+ loss = fluid.layers.cross_entropy(input=y, label=y_)
+ avg_loss = fluid.layers.mean(loss)
+
+ # define the optimization algorithm.
+ optimizer = fluid.optimizer.Adam(learning_rate=1e-3)
+ optimizer.minimize(avg_loss)
+
+Fluid 使用 Program 而不是计算图描述模型,一般情况下,用户无需关心 Program 的细节,当调用以上 layers 时,会向一个全局的 Program: :code:`fluid.framework.default_main_program` 中插入变量(Tensor)和对变量的操作(上述代码段中的 layers 和 optimzier)。
+
+步骤3:参数初始化
+----------------
+
+如上文介绍,Fluid 程序中的 Executor 是连接 Fluid 前端和后端的接口。
+
+默认一个Fluid模型存在至少两段 Program。用于初始化网络中的可学习参数的那一段 :code:`Program` 叫作 :code:`fluid.default_startup_program()` 。
+
+只有执行器 executor 可以执行 Fluid Program,因此,在初始化网络中的可学习参数之前,需要首先创建一个 Fluid executor。
+
+::
+
+ # define the executor.
+ place = fluid.CPUPlace()
+ exe = fluid.Executor(place)
+ exe.run(fluid.default_startup_program())
+
+在以上代码段中, :code:`place` 用于告诉 executor 一段 Fluid Program 在何种设备上执行,
+常见的有 :code:`fluid.CPUPlace()` 和 :code:`fluid.CUDAPlace()` 。
+
+步骤4:数据输入 + 执行模型训练
+----------------------------
+
+我们在步骤 2 中定义的神经网络模型最终被插入一段叫做 :code:`fluid.framework.default_main_program` 的 Fluid Program 中。
+
+网络可学习参数初始化之后,可以通过让执行器 Executor 执行这段 :code:`fluid.framework.default_main_program` 来进行训练。
+
+::
+
+ train_reader = paddle.batch(
+ paddle.reader.shuffle(paddle.dataset.mnist.train(), buf_size=5000),
+ batch_size=BATCH_SIZE)
+ feeder = fluid.DataFeeder(place=place, feed_list=[x, y_])
+
+ for pass_id in range(100):
+ for batch_id, data in enumerate(train_reader()):
+ loss = exe.run(
+ fluid.framework.default_main_program(),
+ feed=feeder.feed(data),
+ fetch_list=[avg_loss])
+ print("Cur Cost : %f" % (np.array(loss[0])[0]))
+
+从上面的代码片段中可以看到,Fluid 程序的训练过程和 TensorFlow 程序的训练过程非常接近,
+都放在一个 :code:`for` 循环中,循环读取一个 mini-batch 数据,
+调用执行器执行 Fluid :code:`default_main_program` :接收 mini-batch 输入,在其上进行前向,反向和参数更新计算。
+
+`注:上面程序使用了 Fluid 内置的 MNIST 数据,和我们提供给 TensorFlow 示例程序的 MNIST 数据完全一样。`
+
+步骤5:观察模型效果
+-----------------
+
+以上步骤已经构成了完整的 Tensorflow 模型训练程序,每个 batch 观察一次 loss,可以直观看到模型的迭代效果:
+
+.. figure:: fluid_mnist.png
+
+ :scale: 40%
+ :align: center
+
+ Figure.2
+
+ Fluid MNIST手写数字识别任务代价下降曲线
+
+附:完整代码
+------------
+
+::
+
+ import numpy as np
+
+ import paddle.fluid as fluid
+ import paddle.v2 as paddle
+
+
+ def main():
+ BATCH_SIZE = 128
+
+ # define the input layers for the network.
+ x = fluid.layers.data(name="img", shape=[1, 28, 28], dtype="float32")
+ y_ = fluid.layers.data(name="label", shape=[1], dtype="int64")
+
+ # define the network topology.
+ y = fluid.layers.fc(input=x, size=10, act="softmax")
+ loss = fluid.layers.cross_entropy(input=y, label=y_)
+ avg_loss = fluid.layers.mean(loss)
+
+ optimizer = fluid.optimizer.Adam(learning_rate=5e-3)
+ optimizer.minimize(avg_loss)
+
+ # define the executor.
+ place = fluid.CPUPlace()
+ exe = fluid.Executor(place)
+ exe.run(fluid.default_startup_program())
+
+ train_reader = paddle.batch(
+ paddle.reader.shuffle(paddle.dataset.mnist.train(), buf_size=5000),
+ batch_size=BATCH_SIZE)
+ feeder = fluid.DataFeeder(place=place, feed_list=[x, y_])
+
+ for pass_id in range(100):
+ for batch_id, data in enumerate(train_reader()):
+ loss = exe.run(
+ fluid.framework.default_main_program(),
+ feed=feeder.feed(data),
+ fetch_list=[avg_loss])
+ print("Cur Cost : %f" % (np.array(loss[0])[0]))
+
+ if __name__ == "__main__":
+ main()
diff --git a/doc/fluid/new_docs/user_guides/howto/basic_concept/fluid_local_train.jpeg b/doc/fluid/new_docs/user_guides/howto/basic_concept/fluid_local_train.jpeg
new file mode 100644
index 0000000000000000000000000000000000000000..0a495901fafb85987e34acc3c454fb87e8160fca
Binary files /dev/null and b/doc/fluid/new_docs/user_guides/howto/basic_concept/fluid_local_train.jpeg differ
diff --git a/doc/fluid/new_docs/user_guides/howto/basic_concept/fluid_mnist.png b/doc/fluid/new_docs/user_guides/howto/basic_concept/fluid_mnist.png
new file mode 100644
index 0000000000000000000000000000000000000000..e5ad0ba058c863cf68ef0789e58fcf67b3115fdb
Binary files /dev/null and b/doc/fluid/new_docs/user_guides/howto/basic_concept/fluid_mnist.png differ
diff --git a/doc/fluid/new_docs/user_guides/howto/configure_simple_model/index.rst b/doc/fluid/new_docs/user_guides/howto/configure_simple_model/index.rst
new file mode 100644
index 0000000000000000000000000000000000000000..5946a2ccb7e43004eae39ec4b3c6112c66c1fd04
--- /dev/null
+++ b/doc/fluid/new_docs/user_guides/howto/configure_simple_model/index.rst
@@ -0,0 +1,88 @@
+.. _user_guide_configure_simple_model:
+
+##############
+配置简单的网络
+##############
+
+在解决实际问题时,可以先从逻辑层面对问题进行建模,明确模型所需要的 **输入数据类型**、**计算逻辑**、**求解目标** 以及 **优化算法**。PaddlePaddle提供了丰富的算子来实现模型逻辑。下面以一个简单回归任务举例说明如何使用PaddlePaddle构建模型。该例子完整代码参见 `fit_a_line `_。
+
+问题描述及定义
+##############
+
+问题描述: 给定一组数据 :math:``,求解出函数 :math:`f`,使得 :math:`y=f(x)`,其中 :math:`x\subset X` 表示一条样本的特征,为 :math:`13` 维的实数向量;:math:`y \subset Y` 为一实数表示该样本对应的值。
+
+我们可以尝试用回归模型来对问题建模,回归问题的损失函数有很多,这里选择常用的均方误差。为简化问题,这里假定 :math:`f` 为简单的线性变换函数,同时选用随机梯度下降算法来求解模型。
+
++----------------+----------------------------------------------+
+| 输入数据类型 | 样本特征: 13 维 实数 |
++ +----------------------------------------------+
+| | 样本标签: 1 维 实数 |
++----------------+----------------------------------------------+
+| 计算逻辑 | 使用线性模型,产生 1维实数作为模型的预测输出 |
++----------------+----------------------------------------------+
+| 求解目标 | 最小化模型预测输出与样本标签间的均方误差 |
++----------------+----------------------------------------------+
+| 优化算法 | 随机梯度下降 |
++----------------+----------------------------------------------+
+
+使用PaddlePadle建模
+###################
+
+从逻辑层面明确了输入数据格式、模型结构、损失函数以及优化算法后,需要使用PaddlePaddle提供的API及算子来实现模型逻辑。一个典型的模型主要包含4个部分,分别是:输入数据格式定义,模型前向计算逻辑,损失函数以及优化算法。
+
+数据层
+------
+
+PaddlePaddle提供了 :code:`fluid.layers.data()` 算子来描述输入数据的格式。
+
+:code:`fluid.layers.data()` 算子的输出是一个Variable。这个Variable的实际类型是Tensor。Tensor具有强大的表征能力,可以表示多维数据。为了精确描述数据结构,通常需要指定数据shape以及数值类型type。其中shape为一个整数向量,type可以是一个字符串类型。目前支持的数据类型参考 :ref:`user_guide_paddle_support_data_types` 。 模型训练一般会使用batch的方式读取数据,而batch的size在训练过程中可能不固定。data算子会依据实际数据来推断batch size,所以这里提供shape时不用关心batch size,只需关心一条样本的shape即可,更高级用法请参考 :ref:`user_guide_customize_batch_size_rank`。从上知,:math:`x` 为 :math:`13` 维的实数向量,:math:`y` 为实数,可使用下面代码定义数据层:
+
+.. code-block:: python
+
+ x = fluid.layers.data(name='x', shape=[13], dtype='float32')
+ y = fluid.layers.data(name='y', shape=[1], dtype='float32')
+
+该模型使用的数据比较简单,事实上data算子还可以描述变长的、嵌套的序列数据。也可以使用 :code:`open_files` 打开文件进行训练。更详细的文档可参照 :ref:`user_guide_prepare_data`。
+
+前向计算逻辑
+------------
+
+实现一个模型最重要的部分是实现计算逻辑,PaddlePaddle提供了丰富的算子。这些算子的封装粒度不同,通常对应一种或一组变换逻辑。算子输出即为对输入数据执行变换后的结果。用户可以灵活使用算子来完成复杂的模型逻辑。比如图像相关任务中会使用较多的卷积算子、序列任务中会使用LSTM/GRU等算子。复杂模型通常会组合多种算子,以完成复杂的变换。PaddlePaddle提供了非常自然的方式来组合算子,一般地可以使用下面的方式:
+
+.. code-block:: python
+
+ op_1_out = fluid.layers.op_1(input=op_1_in, ...)
+ op_2_out = fluid.layers.op_2(input=op_1_out, ...)
+ ...
+
+其中op_1和op_2表示算子类型,可以是fc来执行线性变换(全连接),也可以是conv来执行卷积变换等。通过算子的输入输出的连接来定义算子的计算顺序以及数据流方向。上面的例子中,op_1的输出是op_2的输入,那么在执行计算时,会先计算op_1,然后计算op_2。更复杂的模型可能需要使用控制流算子,依据输入数据来动态执行,针对这种情况,PaddlePaddle提供了IfElseOp和WhileOp等。算子的文档可参考 :code:`fluid.layers`。具体到这个任务, 我们使用一个fc算子:
+
+.. code-block:: python
+
+ y_predict = fluid.layers.fc(input=x, size=1, act=None)
+
+损失函数
+--------
+
+损失函数对应求解目标,我们可以通过最小化损失来求解模型。大多数模型使用的损失函数,输出是一个实数值。但是PaddlePaddle提供的损失算子一般是针对一条样本计算。当输入一个batch的数据时,损失算子的输出有多个值,每个值对应一条样本的损失,所以通常会在损失算子后面使用mean等算子,来对损失做归约。模型在一次前向迭代后会得到一个损失值,PaddlePaddle会自动执行链式求导法则计算模型里面每个参数和变量对应的梯度值。这里使用均方误差损失:
+
+.. code-block:: python
+
+ cost = fluid.layers.square_error_cost(input=y_predict, label=y)
+ avg_cost = fluid.layers.mean(cost)
+
+优化方法
+--------
+
+确定损失函数后,可以通过前向计算得到损失值,然后通过链式求导法则得到参数的梯度值。获取梯度值后需要更新参数,最简单的算法是随机梯度下降法::math:`w=w - \eta \cdot g`。但是普通的随机梯度下降算法存在一些问题: 比如收敛不稳定等。为了改善模型的训练速度以及效果,学术界先后提出了很多优化算法,包括: :code:`Momentum`、:code:`RMSProp`、:code:`Adam` 等。这些优化算法采用不同的策略来更新模型参数,一般可以针对具体任务和具体模型来选择优化算法。不管使用何种优化算法,学习率一般是一个需要指定的比较重要的超参数,需要通过实验仔细调整。这里采用随机梯度下降算法:
+
+.. code-block:: python
+
+ sgd_optimizer = fluid.optimizer.SGD(learning_rate=0.001)
+
+更多优化算子可以参考 :code:`fluid.optimizer()` 。
+
+下一步做什么?
+##############
+
+使用PaddlePaddle实现模型时需要关注 **数据层**、**前向计算逻辑**、**损失函数** 和 **优化方法**。不同的任务需要的数据格式不同,涉及的计算逻辑不同,损失函数不同,优化方法也不同。PaddlePaddle提供了丰富的模型示例,可以以这些示例为参考来构建自己的模型结构。用户可以访问 `模型库 `_ 查看官方提供的示例。
diff --git a/doc/fluid/new_docs/user_guides/howto/debug/index.rst b/doc/fluid/new_docs/user_guides/howto/debug/index.rst
new file mode 100644
index 0000000000000000000000000000000000000000..0878e17b4069be6b08bc85a35e77ba6421633218
--- /dev/null
+++ b/doc/fluid/new_docs/user_guides/howto/debug/index.rst
@@ -0,0 +1,10 @@
+############
+Debug 工具
+############
+
+PaddlePaddle 提供了如下方式方便 Debug 训练 情况
+
+.. toctree::
+ :maxdepth: 2
+
+ visualdl.md
diff --git a/doc/fluid/new_docs/user_guides/howto/debug/visualdl.md b/doc/fluid/new_docs/user_guides/howto/debug/visualdl.md
new file mode 100644
index 0000000000000000000000000000000000000000..a2f30823a6fcd379f94e6e98d043b0d00681827f
--- /dev/null
+++ b/doc/fluid/new_docs/user_guides/howto/debug/visualdl.md
@@ -0,0 +1,218 @@
+# VisualDL (Visualize the Deep Learning)
+
+
+
+
+## 介绍
+VisualDL是一个面向深度学习任务设计的可视化工具,包含了scalar、参数分布、模型结构、图像可视化等功能,项目正处于高速迭代中,新的组件会不断加入。
+
+目前大多数DNN平台均使用Python作为配置语言,VisualDL原生支持python的使用,
+通过在模型的Python配置中添加几行,便可以为训练过程提供丰富的可视化支持。
+
+除了Python SDK之外,VisualDL底层采用C++编写,其暴露的C++ SDK也可以集成到其他平台中,
+实现原生的性能和定制效果。
+
+## 组件
+VisualDL 目前支持4种组件:
+
+- graph
+- scalar
+- image
+- histogram
+
+### Graph
+兼容 ONNX(Open Neural Network Exchange)[https://github.com/onnx/onnx], 通过与 python SDK的结合,VisualDL可以兼容包括 PaddlePaddle, pytorch, mxnet在内的大部分主流DNN平台。
+
+
+
+
+
+### Scalar
+可以用于展示训练测试的误差趋势
+
+
+
+
+
+### Image
+可以用于可视化任何tensor,或模型生成的图片
+
+
+
+
+
+### Histogram
+
+用于可视化任何tensor中元素分布的变化趋势
+
+
+
+
+
+## 快速尝试
+请使用下面的命令,来快速测试 VisualDL。
+
+```
+# 安装,建議是在虚拟环境或anaconda下。
+pip install --upgrade visualdl
+
+# 运行一个例子,vdl_create_scratch_log 将创建测试日志
+vdl_create_scratch_log
+visualDL --logdir=scratch_log --port=8080
+
+# 访问 http://127.0.0.1:8080
+```
+
+如果以上步骤出现问题,很可能是因为python或pip不同版本或不同位置所致,以下安装方法能解决。
+
+## 使用 virtualenv 安装
+
+[Virtualenv](https://virtualenv.pypa.io/en/stable/) 能创建独立Python环境,也能确保Python和pip的相对位置正确。
+
+在macOS上,安装pip和virtualenv如下:
+```
+sudo easy_install pip
+pip install --upgrade virtualenv
+```
+
+在Linux上,安装pip和virtualenv如下:
+```
+sudo apt-get install python3-pip python3-dev python-virtualenv
+```
+
+然后创建一个虚拟环境:
+```
+virtualenv ~/vdl # for Python2.7
+virtualenv -p python3 ~/vdl for Python 3.x
+```
+
+```~/vdl``` 是你的Virtualenv目录, 你也可以选择任一目录。
+
+激活虚拟环境如下:
+```
+source ~/vdl/bin/activate
+```
+
+现在再安装 VisualDL 和运行范例:
+
+```
+pip install --upgrade visualdl
+
+# 运行一个例子,vdl_create_scratch_log 将创建测试日志
+vdl_create_scratch_log
+visualDL --logdir=scratch_log --port=8080
+
+# 访问 http://127.0.0.1:8080
+```
+
+如果在虚拟环境下仍然遇到安装问题,请尝试以下方法。
+
+
+## 使用 Anaconda 安装
+
+Anaconda是一个用于科学计算的Python发行版,提供了包管理与环境管理的功能,可以很方便地解决多版本python并存、切换以及各种第三方包安装问题。
+
+请根据[Anaconda下载网站](https://www.anaconda.com/download) 的指示去下载和安装Anaconda.
+下载Python 3.6版本的command-Line installer.
+
+创建conda环境名字为```vdl```或任何名字:
+```
+conda create -n vdl pip python=2.7 # or python=3.3, etc.
+```
+
+激活conda环境如下:
+```
+source activate vdl
+```
+
+现在再安装 VisualDL 和运行范例:
+
+```
+pip install --upgrade visualdl
+
+# 运行一个例子,vdl_create_scratch_log 将创建测试日志
+vdl_create_scratch_log
+visualDL --logdir=scratch_log --port=8080
+
+# 访问 http://127.0.0.1:8080
+```
+
+如果仍然遇到安装问题,请尝试以下用源代码安装方法。
+
+### 使用代码安装
+```
+#建議是在虚拟环境或anaconda下。
+git clone https://github.com/PaddlePaddle/VisualDL.git
+cd VisualDL
+
+python setup.py bdist_wheel
+pip install --upgrade dist/visualdl-*.whl
+```
+
+如果打包和安装遇到其他问题,不安装只想运行Visual DL可以看[这里](https://github.com/PaddlePaddle/VisualDL/blob/develop/docs/how_to_dev_frontend_en.md)
+
+
+## SDK
+VisualDL 同时提供了python SDK 和 C++ SDK 来实现不同方式的使用。
+
+### Python SDK
+VisualDL 现在支持 Python 2和 Python 3。
+
+以最简单的Scalar组件为例,尝试创建一个scalar组件并插入多个时间步的数据:
+
+```python
+import random
+from visualdl import LogWriter
+
+logdir = "./tmp"
+logger = LogWriter(logdir, sync_cycle=10000)
+
+# mark the components with 'train' label.
+with logger.mode("train"):
+ # create a scalar component called 'scalars/scalar0'
+ scalar0 = logger.scalar("scalars/scalar0")
+
+# add some records during DL model running.
+for step in range(100):
+ scalar0.add_record(step, random.random())
+```
+
+### C++ SDK
+上面 Python SDK 中代码完全一致的C++ SDK用法如下
+```c++
+#include
+#include
+#include "visualdl/sdk.h"
+
+namespace vs = visualdl;
+namespace cp = visualdl::components;
+
+int main() {
+ const std::string dir = "./tmp";
+ vs::LogWriter logger(dir, 10000);
+
+ logger.SetMode("train");
+ auto tablet = logger.AddTablet("scalars/scalar0");
+
+ cp::Scalar scalar0(tablet);
+
+ for (int step = 0; step < 1000; step++) {
+ float v = (float)std::rand() / RAND_MAX;
+ scalar0.AddRecord(step, v);
+ }
+
+ return 0;
+}
+```
+## 启动Board
+当训练过程中已经产生了日志数据,就可以启动board进行实时预览可视化信息
+
+```
+visualDL --logdir
+```
+
+board 还支持一下参数来实现远程的访问:
+
+- `--host` 设定IP
+- `--port` 设定端口
+- `--model_pb` 指定 ONNX 格式的模型文件
diff --git a/doc/fluid/new_docs/user_guides/howto/evaluation/index.rst b/doc/fluid/new_docs/user_guides/howto/evaluation/index.rst
new file mode 100644
index 0000000000000000000000000000000000000000..6f6698cadcba4d9645fdc4a8a74d899598b96d99
--- /dev/null
+++ b/doc/fluid/new_docs/user_guides/howto/evaluation/index.rst
@@ -0,0 +1,10 @@
+############
+模型评估和调试
+############
+
+PaddlePaddle Fluid提供了常用的模型评估指标,并提供了VisualDL工具可视化模型效果。
+
+.. toctree::
+ :maxdepth: 2
+
+ metrics
diff --git a/doc/fluid/new_docs/user_guides/howto/evaluation/metrics.rst b/doc/fluid/new_docs/user_guides/howto/evaluation/metrics.rst
new file mode 100644
index 0000000000000000000000000000000000000000..f37968a50350a90e698cb1a63bd501635753e7fb
--- /dev/null
+++ b/doc/fluid/new_docs/user_guides/howto/evaluation/metrics.rst
@@ -0,0 +1,62 @@
+############
+模型评估
+############
+
+模型评估是用指标反映模型在预期目标下精度,根据模型任务决定观察指标,作为在训练中调整超参数,评估模型效果的重要依据。
+metric函数的输入为当前模型的预测preds和labels,输出是自定义的。metric函数和loss函数非常相似,但是metric并不是模型训练网络组成部分。
+
+用户可以通过训练网络得到当前的预测preds和labels,在Python端定制metric函数;也可以通过定制c++ Operator的方式,在GPU上加速metric计算。
+
+paddle.fluid.metrics模块包含该功能
+
+
+常用指标
+############
+
+metric函数根据模型任务不同,指标构建方法因任务而异。
+
+回归类型任务labels是实数,因此loss和metric函数构建相同,可参考MSE的方法。
+分类任务常用指标为分类指标,本文提到的一般是二分类指标,多分类和多标签需要查看对应的API文档。例如排序指标auc,多分类可以作为0,1分类任务,auc指标仍然适用。
+Fluid中包含了常用分类指标,例如Precision, Recall, Accuracy等,更多请阅读API文档。以 :ref:`Precision` 为例,具体方法为
+
+.. code-block:: python
+
+ >>> import paddle.fluid as fluid
+ >>> labels = fluid.layers.data(name="data", shape=[1], dtype="int32")
+ >>> data = fluid.layers.data(name="data", shape=[32, 32], dtype="int32")
+ >>> pred = fluid.layers.fc(input=data, size=1000, act="tanh")
+ >>> acc = fluid.metrics.Precision()
+ >>> for pass in range(PASSES):
+ >>> acc.reset()
+ >>> for data in train_reader():
+ >>> loss, preds, labels = exe.run(fetch_list=[cost, preds, labels])
+ >>> acc.update(preds=preds, labels=labels)
+ >>> numpy_acc = acc.eval()
+
+
+其他任务例如MultiTask Learning,Metric Learning,Learning To Rank各种指标构造方法请参考API文档。
+
+自定义指标
+############
+Fluid支持自定义指标,灵活支持各类计算任务。下文通过一个简单的计数器metric函数,实现对模型的评估。
+其中preds是模型预测值,labels是给定的标签。
+
+.. code-block:: python
+
+ >>> class MyMetric(MetricBase):
+ >>> def __init__(self, name=None):
+ >>> super(MyMetric, self).__init__(name)
+ >>> self.counter = 0 # simple counter
+
+ >>> def reset(self):
+ >>> self.counter = 0
+
+ >>> def update(self, preds, labels):
+ >>> if not _is_numpy_(preds):
+ >>> raise ValueError("The 'preds' must be a numpy ndarray.")
+ >>> if not _is_numpy_(labels):
+ >>> raise ValueError("The 'labels' must be a numpy ndarray.")
+ >>> self.counter += sum(preds == labels)
+
+ >>> def eval(self):
+ >>> return self.counter
diff --git a/doc/fluid/new_docs/user_guides/howto/modification/foo.rst b/doc/fluid/new_docs/user_guides/howto/modification/foo.rst
new file mode 100644
index 0000000000000000000000000000000000000000..9d43c91a8544c3b281b2e8d556cb8b8e069d7e0a
--- /dev/null
+++ b/doc/fluid/new_docs/user_guides/howto/modification/foo.rst
@@ -0,0 +1,3 @@
+###
+FAQ
+###
diff --git a/doc/fluid/new_docs/user_guides/howto/prepare_data/feeding_data.rst b/doc/fluid/new_docs/user_guides/howto/prepare_data/feeding_data.rst
new file mode 100644
index 0000000000000000000000000000000000000000..c3bf033bb8316eeb4901c0cdc61e0556c8816dac
--- /dev/null
+++ b/doc/fluid/new_docs/user_guides/howto/prepare_data/feeding_data.rst
@@ -0,0 +1,169 @@
+.. _user_guide_use_numpy_array_as_train_data:
+
+###########################
+使用Numpy Array作为训练数据
+###########################
+
+PaddlePaddle Fluid支持使用 :code:`fluid.layers.data()` 配置数据层;
+再使用 Numpy Array 或者直接使用Python创建C++的
+:code:`fluid.LoDTensor` , 通过 :code:`Executor.run(feed=...)` 传给
+:code:`fluid.Executor` 或 :code:`fluid.ParallelExecutor` 。
+
+数据层配置
+##########
+
+通过 :code:`fluid.layers.data()` 可以配置神经网络中需要的数据层。具体方法为:
+
+.. code-block:: python
+
+ import paddle.fluid as fluid
+
+ image = fluid.layers.data(name="image", shape=[3, 224, 224])
+ label = fluid.layers.data(name="label", shape=[1], dtype="int64")
+
+ # use image/label as layer input
+ prediction = fluid.layers.fc(input=image, size=1000, act="softmax")
+ loss = fluid.layers.cross_entropy(input=prediction, label=label)
+ ...
+
+上段代码中,:code:`image` 和 :code:`label` 是通过 :code:`fluid.layers.data`
+创建的两个输入数据层。其中 :code:`image` 是 :code:`[3, 224, 224]` 维度的浮点数据;
+:code:`label` 是 :code:`[1]` 维度的整数数据。这里需要注意的是:
+
+1. Fluid中默认使用 :code:`-1` 表示 batch size 维度,默认情况下会在 :code:`shape`
+ 的第一个维度添加 :code:`-1` 。 所以 上段代码中, 我们可以接受将一个
+ :code:`[32, 3, 224, 224]` 的numpy array传给 :code:`image` 。 如果想自定义batch size
+ 维度的位置的话,请设置 :code:`fluid.layers.data(append_batch_size=False)` 。
+ 请参考进阶使用中的 :ref:`user_guide_customize_batch_size_rank` 。
+
+
+2. Fluid中用来做类别标签的数据类型是 :code:`int64`,并且标签从0开始。可用数据类型请参考 :ref:`user_guide_paddle_support_data_types`。
+
+.. _user_guide_feed_data_to_executor:
+
+传递训练数据给执行器
+####################
+
+:code:`Executor.run` 和 :code:`ParallelExecutor.run` 都接受一个 :code:`feed` 参数。
+这个参数是一个Python的字典。它的键是数据层的名字,例如上文代码中的 :code:`image`。
+它的值是对应的numpy array。
+
+例如:
+
+.. code-block:: python
+
+ exe = fluid.Executor(fluid.CPUPlace())
+ exe.run(feed={
+ "image": numpy.random.random(size=(32, 3, 224, 224)).astype('float32'),
+ "label": numpy.random.random(size=(32, 1)).astype('int64')
+ })
+
+进阶使用
+########
+
+如何传入序列数据
+----------------
+
+序列数据是PaddlePaddle Fluid支持的特殊数据类型,可以使用 :code:`LoDTensor` 作为
+输入数据类型。它需要用户: 1. 传入一个mini-batch需要被训练的所有数据;
+2.每个序列的长度信息。
+用户可以使用 :code:`fluid.create_lod_tensor` 来创建 :code:`LoDTensor`。
+
+传入序列信息的时候,需要设置序列嵌套深度,:code:`lod_level`。
+例如训练数据是词汇组成的句子,:code:`lod_level=1`;训练数据是 词汇先组成了句子,
+句子再组成了段落,那么 :code:`lod_level=2`。
+
+例如:
+
+.. code-block:: python
+
+ sentence = fluid.layers.data(name="sentence", dtype="int64", shape=[1], lod_level=1)
+
+ ...
+
+ exe.run(feed={
+ "sentence": create_lod_tensor(
+ data=numpy.array([1, 3, 4, 5, 3, 6, 8], dtype='int64').reshape(-1, 1),
+ lod=[4, 1, 2],
+ place=fluid.CPUPlace()
+ )
+ })
+
+训练数据 :code:`sentence` 包含三个样本,他们的长度分别是 :code:`4, 1, 2`。
+他们分别是 :code:`data[0:4]`, :code:`data[4:5]` 和 :code:`data[5:7]`。
+
+如何分别设置ParallelExecutor中每个设备的训练数据
+------------------------------------------------
+
+用户将数据传递给使用 :code:`ParallelExecutor.run(feed=...)` 时,
+可以显示指定每一个训练设备(例如GPU)上的数据。
+用户需要将一个列表传递给 :code:`feed` 参数,列表中的每一个元素都是一个字典。
+这个字典的键是数据层的名字,值是数据层的值。
+
+例如:
+
+.. code-block:: python
+
+ parallel_executor = fluid.ParallelExecutor()
+ parallel_executor.run(
+ feed=[
+ {
+ "image": numpy.random.random(size=(32, 3, 224, 224)).astype('float32'),
+ "label": numpy.random.random(size=(32, 1)).astype('int64')
+ },
+ {
+ "image": numpy.random.random(size=(16, 3, 224, 224)).astype('float32'),
+ "label": numpy.random.random(size=(16, 1)).astype('int64')
+ },
+ ]
+ )
+
+上述代码中,GPU0会训练 32 个样本,而 GPU1训练 16 个样本。
+
+
+.. _user_guide_customize_batch_size_rank:
+
+自定义BatchSize维度
+-------------------
+
+PaddlePaddle Fluid默认batch size是数据的第一维度,以 :code:`-1` 表示。但是在高级
+使用中,batch_size 可以固定,也可以是其他维度或者多个维度来表示。这都需要设置
+:code:`fluid.layers.data(append_batch_size=False)` 来完成。
+
+1. 固定batch size维度
+
+ .. code-block:: python
+
+ image = fluid.layers.data(name="image", shape=[32, 784], append_batch_size=False)
+
+ 这里,:code:`image` 永远是一个 :code:`[32, 784]` 大小的矩阵。
+
+2. 使用其他维度表示batch size
+
+ .. code-block:: python
+
+ sentence = fluid.layers.data(name="sentence",
+ shape=[80, -1, 1],
+ append_batch_size=False,
+ dtype="int64")
+
+ 这里 :code:`sentence` 的中间维度是batch size。这种数据排布会用在定长的循环神经
+ 网络中。
+
+
+.. _user_guide_paddle_support_data_types:
+
+Fluid目前支持的数据类型
+-----------------------
+
+PaddlePaddle Fluid目前支持的数据类型包括:
+
+ * float16: 部分操作支持
+ * float32: 主要实数类型
+ * float64: 次要实数类型,支持大部分操作
+ * int32: 次要标签类型
+ * int64: 主要标签类型
+ * uint64: 次要标签类型
+ * bool: 控制流数据类型
+ * int16: 次要标签类型
+ * uint8: 输入数据类型,可用于图像像素
\ No newline at end of file
diff --git a/doc/fluid/new_docs/user_guides/howto/prepare_data/index.rst b/doc/fluid/new_docs/user_guides/howto/prepare_data/index.rst
new file mode 100644
index 0000000000000000000000000000000000000000..56fa928029903f1e3bd3e8064c146797f01b2b85
--- /dev/null
+++ b/doc/fluid/new_docs/user_guides/howto/prepare_data/index.rst
@@ -0,0 +1,52 @@
+.. _user_guide_prepare_data:
+
+########
+准备数据
+########
+
+PaddlePaddle Fluid支持两种传入数据的方式:
+
+1. 用户需要使用 :code:`fluid.layers.data`
+配置数据输入层,并在 :code:`fluid.Executor` 或 :code:`fluid.ParallelExecutor`
+中,使用 :code:`executor.run(feed=...)` 传入训练数据。
+
+2. 用户需要先将训练数据
+转换成 Paddle 识别的 :code:`fluid.recordio_writer` , 再使用
+:code:`fluid.layers.open_files` 以及 :code:`fluid.layers.reader` 配置数据读取。
+
+这两种准备数据方法的比较如下:
+
+.. _user_guide_prepare_data_comparision:
+
++------------+----------------------------------+---------------------------------------+
+| | Feed数据 | 使用Reader |
++============+==================================+=======================================+
+| API接口 | :code:`executor.run(feed=...)` | :code:`fluid.layers.reader` |
++------------+----------------------------------+---------------------------------------+
+| 数据格式 | Numpy Array | :code:`fluid.recordio_writer` |
++------------+----------------------------------+---------------------------------------+
+| 数据增强 | Python端使用其他库完成 | 使用Fluid中的Operator 完成 |
++------------+----------------------------------+---------------------------------------+
+| 速度 | 慢 | 快 |
++------------+----------------------------------+---------------------------------------+
+| 推荐用途 | 调试模型 | 工业训练 |
++------------+----------------------------------+---------------------------------------+
+
+这些准备数据的详细使用方法,请参考:
+
+.. toctree::
+ :maxdepth: 2
+
+ feeding_data
+ use_recordio_reader
+
+Python Reader
+#############
+
+为了方便用户在Python中定义数据处理流程,PaddlePaddle Fluid支持 Python Reader,
+具体请参考:
+
+.. toctree::
+ :maxdepth: 2
+
+ reader.md
diff --git a/doc/fluid/new_docs/user_guides/howto/prepare_data/reader.md b/doc/fluid/new_docs/user_guides/howto/prepare_data/reader.md
new file mode 100644
index 0000000000000000000000000000000000000000..aa50e4d26166536eaf8044d527debd8ad46060f6
--- /dev/null
+++ b/doc/fluid/new_docs/user_guides/howto/prepare_data/reader.md
@@ -0,0 +1,210 @@
+```eval_rst
+.. _user_guide_reader:
+```
+
+# Python Reader
+
+During the training and testing phases, PaddlePaddle programs need to read data. To help the users write code that performs reading input data, we define the following:
+
+- A *reader*: A function that reads data (from file, network, random number generator, etc) and yields the data items.
+- A *reader creator*: A function that returns a reader function.
+- A *reader decorator*: A function, which takes in one or more readers, and returns a reader.
+- A *batch reader*: A function that reads data (from *reader*, file, network, random number generator, etc) and yields a batch of data items.
+
+and also provide a function which can convert a reader to a batch reader, frequently used reader creators and reader decorators.
+
+## Data Reader Interface
+
+*Data reader* doesn't have to be a function that reads and yields data items. It can just be any function without any parameters that creates an iterable (anything can be used in `for x in iterable`) as follows:
+
+```
+iterable = data_reader()
+```
+
+The item produced from the iterable should be a **single** entry of data and **not** a mini batch. The entry of data could be a single item or a tuple of items. Item should be of one of the [supported types](http://www.paddlepaddle.org/doc/ui/data_provider/pydataprovider2.html?highlight=dense_vector#input-types) (e.g., numpy 1d array of float32, int, list of int etc.)
+
+An example implementation for single item data reader creator is as follows:
+
+```python
+def reader_creator_random_image(width, height):
+ def reader():
+ while True:
+ yield numpy.random.uniform(-1, 1, size=width*height)
+ return reader
+```
+
+An example implementation for multiple item data reader creator is as follows:
+```python
+def reader_creator_random_image_and_label(width, height, label):
+ def reader():
+ while True:
+ yield numpy.random.uniform(-1, 1, size=width*height), label
+ return reader
+```
+
+## Batch Reader Interface
+
+*Batch reader* can be any function without any parameters that creates an iterable (anything can be used in `for x in iterable`). The output of the iterable should be a batch (list) of data items. Each item inside the list should be a tuple.
+
+Here are some valid outputs:
+
+```python
+# a mini batch of three data items. Each data item consist three columns of data, each of which is 1.
+[(1, 1, 1),
+(2, 2, 2),
+(3, 3, 3)]
+
+# a mini batch of three data items, each data item is a list (single column).
+[([1,1,1],),
+([2,2,2],),
+([3,3,3],)]
+```
+
+Please note that each item inside the list must be a tuple, below is an invalid output:
+```python
+ # wrong, [1,1,1] needs to be inside a tuple: ([1,1,1],).
+ # Otherwise it is ambiguous whether [1,1,1] means a single column of data [1, 1, 1],
+ # or three columns of data, each of which is 1.
+[[1,1,1],
+[2,2,2],
+[3,3,3]]
+```
+
+It is easy to convert from a reader to a batch reader:
+
+```python
+mnist_train = paddle.dataset.mnist.train()
+mnist_train_batch_reader = paddle.batch(mnist_train, 128)
+```
+
+It is also straight forward to create a custom batch reader:
+
+```python
+def custom_batch_reader():
+ while True:
+ batch = []
+ for i in xrange(128):
+ batch.append((numpy.random.uniform(-1, 1, 28*28),)) # note that it's a tuple being appended.
+ yield batch
+
+mnist_random_image_batch_reader = custom_batch_reader
+```
+
+## Usage
+
+Following is how we can use the reader with PaddlePaddle:
+The batch reader, a mapping from item(s) to data layer, the batch size and the number of total passes will be passed into `paddle.train` as follows:
+
+```python
+# two data layer is created:
+image_layer = paddle.layer.data("image", ...)
+label_layer = paddle.layer.data("label", ...)
+
+# ...
+batch_reader = paddle.batch(paddle.dataset.mnist.train(), 128)
+paddle.train(batch_reader, {"image":0, "label":1}, 128, 10, ...)
+```
+
+## Data Reader Decorator
+
+The *Data reader decorator* takes in a single reader or multiple data readers and returns a new data reader. It is similar to a [python decorator](https://wiki.python.org/moin/PythonDecorators), but it does not use `@` in the syntax.
+
+Since we have a strict interface for data readers (no parameters and return a single data item), a data reader can be used in a flexible way using data reader decorators. Following are a few examples:
+
+### Prefetch Data
+
+Since reading data may take some time and training can not proceed without data, it is generally a good idea to prefetch the data.
+
+Use `paddle.reader.buffered` to prefetch data:
+
+```python
+buffered_reader = paddle.reader.buffered(paddle.dataset.mnist.train(), 100)
+```
+
+`buffered_reader` will try to buffer (prefetch) `100` data entries.
+
+### Compose Multiple Data Readers
+
+For example, if we want to use a source of real images (say reusing mnist dataset), and a source of random images as input for [Generative Adversarial Networks](https://arxiv.org/abs/1406.2661).
+
+We can do the following :
+
+```python
+def reader_creator_random_image(width, height):
+ def reader():
+ while True:
+ yield numpy.random.uniform(-1, 1, size=width*height)
+ return reader
+
+def reader_creator_bool(t):
+ def reader:
+ while True:
+ yield t
+ return reader
+
+true_reader = reader_creator_bool(True)
+false_reader = reader_creator_bool(False)
+
+reader = paddle.reader.compose(paddle.dataset.mnist.train(), data_reader_creator_random_image(20, 20), true_reader, false_reader)
+# Skipped 1 because paddle.dataset.mnist.train() produces two items per data entry.
+# And we don't care about the second item at this time.
+paddle.train(paddle.batch(reader, 128), {"true_image":0, "fake_image": 2, "true_label": 3, "false_label": 4}, ...)
+```
+
+### Shuffle
+
+Given the shuffle buffer size `n`, `paddle.reader.shuffle` returns a data reader that buffers `n` data entries and shuffles them before a data entry is read.
+
+Example:
+```python
+reader = paddle.reader.shuffle(paddle.dataset.mnist.train(), 512)
+```
+
+## Q & A
+
+### Why does a reader return only a single entry, and not a mini batch?
+
+Returning a single entry makes reusing existing data readers much easier (for example, if an existing reader returns 3 entries instead if a single entry, the training code will be more complicated because it need to handle cases like a batch size 2).
+
+We provide a function: `paddle.batch` to turn (a single entry) reader into a batch reader.
+
+### Why do we need a batch reader, isn't is sufficient to give the reader and batch_size as arguments during training ?
+
+In most of the cases, it would be sufficient to give the reader and batch_size as arguments to the train method. However sometimes the user wants to customize the order of data entries inside a mini batch, or even change the batch size dynamically. For these cases using a batch reader is very efficient and helpful.
+
+### Why use a dictionary instead of a list to provide mapping?
+
+Using a dictionary (`{"image":0, "label":1}`) instead of a list (`["image", "label"]`) gives the advantage that the user can easily reuse the items (e.g., using `{"image_a":0, "image_b":0, "label":1}`) or even skip an item (e.g., using `{"image_a":0, "label":2}`).
+
+### How to create a custom data reader creator ?
+
+```python
+def image_reader_creator(image_path, label_path, n):
+ def reader():
+ f = open(image_path)
+ l = open(label_path)
+ images = numpy.fromfile(
+ f, 'ubyte', count=n * 28 * 28).reshape((n, 28 * 28)).astype('float32')
+ images = images / 255.0 * 2.0 - 1.0
+ labels = numpy.fromfile(l, 'ubyte', count=n).astype("int")
+ for i in xrange(n):
+ yield images[i, :], labels[i] # a single entry of data is created each time
+ f.close()
+ l.close()
+ return reader
+
+# images_reader_creator creates a reader
+reader = image_reader_creator("/path/to/image_file", "/path/to/label_file", 1024)
+paddle.train(paddle.batch(reader, 128), {"image":0, "label":1}, ...)
+```
+
+### How is `paddle.train` implemented
+
+An example implementation of paddle.train is:
+
+```python
+def train(batch_reader, mapping, batch_size, total_pass):
+ for pass_idx in range(total_pass):
+ for mini_batch in batch_reader(): # this loop will never end in online learning.
+ do_forward_backward(mini_batch, mapping)
+```
diff --git a/doc/fluid/new_docs/user_guides/howto/prepare_data/use_recordio_reader.rst b/doc/fluid/new_docs/user_guides/howto/prepare_data/use_recordio_reader.rst
new file mode 100644
index 0000000000000000000000000000000000000000..dfda33f1b03516fe2c704f55d095955282b19109
--- /dev/null
+++ b/doc/fluid/new_docs/user_guides/howto/prepare_data/use_recordio_reader.rst
@@ -0,0 +1,167 @@
+.. _user_guide_use_recordio_as_train_data:
+
+############################
+使用RecordIO文件作为训练数据
+############################
+
+相比于 :ref:`user_guide_use_numpy_array_as_train_data`,
+:ref:`user_guide_use_recordio_as_train_data` 的性能更好;
+但是用户需要先将训练数据集转换成RecordIO文件格式,再使用
+:code:`fluid.layers.open_files()` 层在神经网络配置中导入 RecordIO 文件。
+用户还可以使用 :code:`fluid.layers.double_buffer()` 加速数据从内存到显存的拷贝,
+使用 :code:`fluid.layers.Preprocessor` 工具进行数据增强。
+
+将训练数据转换成RecordIO文件格式
+################################
+
+:code:`fluid.recordio_writer` 中,每个记录都是一个
+:code:`vector`, 即一个支持序列信息的Tensor数组。这个数组包括训练所需
+的所有特征。例如对于图像分类来说,这个数组可以包含图片和分类标签。
+
+用户可以使用 :code:`fluid.recordio_writer.convert_reader_to_recordio_file()` 可以将
+:ref:`user_guide_reader` 转换成一个RecordIO文件。或者可以使用
+:code:`fluid.recordio_writer.convert_reader_to_recordio_files()` 将一个
+:ref:`user_guide_reader` 转换成多个RecordIO文件。
+
+具体使用方法为:
+
+.. code-block:: python
+
+ import paddle.fluid as fluid
+ import numpy
+
+ def reader_creator():
+ def __impl__():
+ for i in range(1000):
+ yield [
+ numpy.random.random(size=[3,224,224], dtype="float32"),
+ numpy.random.random(size=[1], dtype="int64")
+ ]
+ return __impl__
+
+ img = fluid.layers.data(name="image", shape=[3, 224, 224])
+ label = fluid.layers.data(name="label", shape=[1], dtype="int64")
+ feeder = fluid.DataFeeder(feed_list=[img, label], place=fluid.CPUPlace())
+
+ BATCH_SIZE = 32
+ reader = paddle.batch(reader_creator(), batch_size=BATCH_SIZE)
+ fluid.recordio_writer.convert_reader_to_recordio_file(
+ "train.recordio", feeder=feeder, reader_creator=reader)
+
+其中 :code:`reader_creator` 创建了一个 :code:`Reader`。
+:ref:`_api_fluid_data_feeder_DataFeeder`
+是将 :code:`Reader` 转换成 :code:`LoDTensor` 的工具。详细请参考
+:ref:`user_guide_reader` 。
+
+上述程序将 :code:`reader_creator` 的数据转换成了 :code:`train.recordio` 文件,
+其中每一个record 含有 32 条样本。如果batch size会在训练过程中调整,
+用户可以将每一个Record的样本数设置成1。并参考
+:ref:`user_guide_use_recordio_as_train_data_use_op_create_batch`。
+
+
+配置神经网络, 打开RecordIO文件
+##############################
+
+RecordIO文件转换好之后,用户可以使用 :code:`fluid.layers.open_files()`
+打开文件,并使用 :code:`fluid.layers.read_file` 读取文件内容。
+简单使用方法如下:
+
+.. code-block:: python
+
+ import paddle.fluid as fluid
+
+ file_obj = fluid.layers.open_files(
+ filenames=["train.recordio"],
+ shape=[[3, 224, 224], [1]],
+ lod_levels=[0, 0],
+ dtypes=["float32", "int64"],
+ pass_num=100
+ )
+
+ image, label = fluid.layers.read_file(file_obj)
+
+其中如果设置了 :code:`pass_num` ,那么当所有数据读完后,会重新读取数据,
+直到读取了 :code:`pass_num` 遍。
+
+
+
+进阶使用
+########
+
+
+使用 :code:`fluid.layers.double_buffer()`
+------------------------------------------
+
+:code:`Double buffer` 使用双缓冲技术,将训练数据从内存中复制到显存中。配置双缓冲
+需要使用 :code:`fluid.layers.double_buffer()` 修饰文件对象。 例如:
+
+.. code-block:: python
+
+ import paddle.fliud as fluid
+ file_obj = fluid.layers.open_files(...)
+ file_obj = fluid.layers.double_buffer(file_obj)
+
+ image, label = fluid.layers.read_file(file_obj)
+
+双缓冲技术可以参考
+`Multiple buffering `_ 。
+
+配置数据增强
+------------
+
+使用 :code:`fluid.layers.Preprocessor` 可以配置文件的数据增强方法。例如
+
+.. code-block:: python
+
+ import paddle.fluid as fluid
+ file_obj = fluid.layers.open_files(...)
+ preprocessor = fluid.layers.Preprocessor(reader=data_file)
+ with preprocessor.block():
+ image, label = preprocessor.inputs()
+ image = image / 2
+ label = label + 1
+ preprocessor.outputs(image, label)
+
+如上代码所示,使用 :code:`Preprocessor` 定义了一个数据增强模块,并在
+:code:`with preprocessor.block()` 中定义了数据增强的具体操作。 用户通过配置
+:code:`preprocessor.inputs()` 获得数据文件中的各个字段。 并用
+:code:`preprocessor.outputs()` 标记预处理后的输出。
+
+.. _user_guide_use_recordio_as_train_data_use_op_create_batch:
+
+使用Op组batch
+-------------
+
+使用 :code:`fluid.layers.batch()` 可以在训练的过程中动态的组batch。例如
+
+.. code-block:: python
+
+ import paddle.fluid as fluid
+ file_obj = fluid.layers.open_files(...)
+ file_obj = fluid.layers.batch(file_obj, batch_size=32)
+
+ img, label = fluid.layers.read_file(file_obj)
+
+需要注意的是,如果数据集中的最后几个样本不能组成 :code:`batch_size` 大小的批量数据,
+那么这几个样本直接组成一个批量数据进行训练。
+
+读入数据的shuffle
+-----------------
+
+使用 :code:`fluid.layers.shuffle()` 可以在训练过程中动态重排训练数据。例如
+
+.. code-block:: python
+
+ import paddle.fluid as fluid
+ file_obj = fluid.layers.open_files(...)
+ file_obj = fliud.layers.shuffle(file_obj, buffer_size=8192)
+
+ img, label = fliud.layers.read_file(file_obj)
+
+需要注意的是:
+
+1. :code:`shuffle` 实现方法是:
+先读入 :code:`buffer_size` 条样本,再随机的选出样本进行训练。
+
+2. :code:`shuffle` 中 :code:`buffer_size` 会占用训练内存,需要确定训练过程中内存
+足够支持缓存 :code:`buffer_size` 条数据。
diff --git a/doc/fluid/new_docs/user_guides/howto/training/checkpoint_doc_cn.md b/doc/fluid/new_docs/user_guides/howto/training/checkpoint_doc_cn.md
new file mode 100644
index 0000000000000000000000000000000000000000..c4afd536c67b24a17e4437ecedf779ddcddcbc98
--- /dev/null
+++ b/doc/fluid/new_docs/user_guides/howto/training/checkpoint_doc_cn.md
@@ -0,0 +1,60 @@
+# Checkpoint功能使用指南
+
+## 背景
+单机/多机在训练过程中会由于软件/硬件的问题出现异常,导致训练中断,进而导致训练无结果或结果不可用,浪费大量时间和机器性能。
+
+## 目的
+Checkpoint功能能够在训练中途对训练数据中间数据进行保存,出现异常恢复训练的时候能够加载中途保存的数据继续训练, 实现单机/多机的容错训练的功能。
+
+## 说明
+### 目前已实现的参数保存:
+1. 基于Trainer 0 实现训练过程中的参数保存
+2. 基于PServer 实现了```Distribute Lookup Table```相关参数保存
+### Fluid Checkpoint 保存数据目录结构:
+
+```
+checkpoint_dir (用户定义的checkpoint目录)
+├── checkpoint_0 (第一次保存)
+│ ├── __lockup_table__ (Distribute Lookup Table 目录)
+│ │ ├── table_pserver_0 (Pserver 0 号保存的lookup table 数据)
+│ │ └── table_pserver_1
+│ ├── __model__ (model 目录)
+│ │ └── var.w_1
+│ └── trainer_0 (trainer 自有数据保存)
+│ ├── epoch_id
+│ └── step_id
+└── checkpoint_1 (第二次保存)
+```
+
+## 使用方法
+### 声明Fluid.CheckpointConfig
+用户对checkpoint功能的配置,主要是配置对象```Fluid```中的```CheckpointConfig```.
+
+```CheckpointConfig``` 包括4个参数:
+
+| 参数 | 类型 | 说明 |
+| - | :-: | - |
+| checkpoint_dir | int| checkpoint存储目录 |
+| max_num_checkpoints | int | 最大保存的checkpoint副本数 |
+| epoch_interval | int | 每隔epoch_interval轮epoch |
+| step_interval | int | 每隔step_interval轮step |
+
+### 在Fluid.Trainer对象的声明中加入Fluid.CheckpointConfig的声明
+Trainer的__init__方法的参数中包含了对```CheckpointConfig```, 需要传入在声明Trainer前声明的```CheckpointConfig```对象。
+如:
+```python
+config = CheckpointConfig(
+ checkpoint_dir = "/tmp/ckpt", max_num_checkpoints = 2,
+ epoch_interval = 2, step_interval = 10)
+trainer = Trainer(..., checkpoint_config=config)
+```
+定义和声明完成后, 训练在运行过程中就会在指定的step和epoch处进行保存,出现异常时,就会自动从最新的checkpoint目录进行参数恢复啦!
+
+## 相关API
+[Trainer API 说明](https://github.com/PaddlePaddle/Paddle/blob/develop/python/paddle/fluid/trainer.py)
+
+## 注意
+1. 保证每个训练的```checkpoint_dir``` 与其他训练独立。
+2. 最大副本数量```max_num_checkpoints```需要根据磁盘容量以及模型的大小进行调整, 保证磁盘的可用性。
+3. ```epoch_interval``` 和 ```step_interval``` 不宜过小, 频繁的进行checkpoint会拖慢训练速度。
+4. **分布式训练**的过程中:每个Trainer都会在```checkpoint_dir```目录中保存当前Trainer的参数(只有Trainer 0会保存模型的参数),需要**分布式文件系统(HDFS等)**将同```checkpoint_dir```目录的数据进行合并才能得到完整的数据,恢复训练的时候需要用完整的数据进行恢复。
diff --git a/doc/fluid/new_docs/user_guides/howto/training/checkpoint_doc_en.md b/doc/fluid/new_docs/user_guides/howto/training/checkpoint_doc_en.md
new file mode 100644
index 0000000000000000000000000000000000000000..14d37246ca0cab8715e244fda9624d0d59f8ec5f
--- /dev/null
+++ b/doc/fluid/new_docs/user_guides/howto/training/checkpoint_doc_en.md
@@ -0,0 +1,62 @@
+# Checkpoint User Guide
+
+## Background
+In many cases, Stand-alone training and Distributed training can be aborted by the software problem or hardware problem. More seriously, we waste so much time and the performance of the machine but get nothing, which makes us frustrating and we have to restart it again.
+
+## Purpose
+The feature of ```Checkpoint``` can save Intermediate model variables, lookup table variable, and other needs data in checkpoint directory. When the exception occurs, we can load these variables from the checkpoint directory immediately.
+## Introduce
+### Complete Features Currently:
+1. The Trainer 0 will save model variables in training.
+2. Each of the Trainer will save its own arguments needed.
+3. Each of the Parameter Server will save ```Distribute Lookup Table``` variables in training.
+### Fluid Checkpoint directory structure:
+
+```
+checkpoint_dir (the checkpoint directory user define)
+├── checkpoint_0 (the first save directory)
+│ ├── __lockup_table__ (Distribute Lookup Table directory)
+│ │ ├── table_pserver_0 (Lookup table's data about Pserver 0)
+│ │ └── table_pserver_1
+│ ├── __model__ (model directory)
+│ │ └── var.w_1
+│ └── trainer_0 (each trainer will save its own data)
+│ ├── epoch_id
+│ └── step_id
+└── checkpoint_1 (the second save directory)
+```
+
+## usage
+### Fluid.CheckpointConfig construct
+When the user wants to use ```Checkpoint``` feature, the main thing user have to do is declare ```CheckpointConfig``` and construct it.
+
+```CheckpointConfig``` has 4 member variables need to be initialized:
+
+| Member Variable | Type | Comment |
+| - | :-: | - |
+| checkpoint_dir | int| checkpoint directory |
+| max_num_checkpoints | int | Maximum number of checkpoint copies |
+| epoch_interval | int | epoch interval times |
+| step_interval | int | step interval times |
+
+### Add Fluid.CheckpointConfig's declaration in Fluid.Trainer
+Because the initialization of Trainer needs an instance of ```CheckpointConfig```., we should declare ```CheckpointConfig``` in ```Fluid``` first.
+
+For example:
+```python
+config = CheckpointConfig(
+ checkpoint_dir = "/tmp/ckpt", max_num_checkpoints = 2,
+ epoch_interval = 2, step_interval = 10)
+trainer = Trainer(..., checkpoint_config=config)
+```
+
+After all the things done, the train will save checkpoint at the specified epoch and step, when the train is aborted, the user can restart it, the train will restore from the latest copy.
+
+## Related API
+[Related Trainer API](https://github.com/PaddlePaddle/Paddle/blob/develop/python/paddle/fluid/trainer.py)
+
+## Attention
+1. Make the ```checkpoint_dir``` only be used by one train job.
+2. The number of ```max_num_checkpoints``` need to be adjusted by the disk size and model size.
+3. Too frequently to slow down the train speed, so too ```small epoch_interval``` and ```step_interval``` are not suitable.
+4. **In distributed train**, each Trainer will save arguments in its ```checkpoint_dir``` (Only Trainer 0 will save model variables). We need **distributed file system (HDFS, etc)** to merge all the ```checkpoint_dir``` to get the whole data.
diff --git a/doc/fluid/new_docs/user_guides/howto/training/cluster_howto.rst b/doc/fluid/new_docs/user_guides/howto/training/cluster_howto.rst
new file mode 100644
index 0000000000000000000000000000000000000000..00ec9e819c81fae3263b1f1e6bcedf524f2b3991
--- /dev/null
+++ b/doc/fluid/new_docs/user_guides/howto/training/cluster_howto.rst
@@ -0,0 +1,160 @@
+.. _cluster_howto
+
+Fluid分布式训练使用手册
+====================
+
+分布式训练基本思想
+---------------
+
+分布式深度学习训练通常分为两种并行化方法:数据并行,模型并行,参考下图:
+
+.. image:: src/parallelism.png
+
+在模型并行方式下,模型的层和参数将被分布在多个节点上,模型在一个mini-batch的前向和反向训练中,将经过多次跨\
+节点之间的通信。每个节点只保存整个模型的一部分;在数据并行方式下,每个节点保存有完整的模型的层和参数,每个节点\
+独自完成前向和反向计算,然后完成梯度的聚合并同步的更新所有节点上的参数。Fluid目前版本仅提供数据并行方式,另外\
+诸如模型并行的特例实现(超大稀疏模型训练)功能将在后续的文档中予以说明。
+
+在数据并行模式的训练中,Fluid使用了两种通信模式,用于应对不同训练任务对分布式训练的要求,分别为RPC通信和Collective
+通信。其中RPC通信方式使用 `gRPC `_ ,Collective通信方式使用
+`NCCL2 `_ 。
+
+.. csv-table:: 下面是一个RPC通信和Collective通信的横向对比:
+ :header: "Feature", "Coolective", "RPC"
+
+ "Ring-Based通信", "Yes", "No"
+ "异步训练", "Yes", "Yes"
+ "分布式模型", "No", "Yes"
+ "容错训练", "No", "Yes"
+ "性能", "Faster", "Fast"
+
+- RPC通信方式的结构:
+
+ .. image:: src/dist_train_pserver.png
+
+ 使用RPC通信方式的数据并行分布式训练,会启动多个pserver进程和多个trainer进程,每个pserver进程\
+ 会保存一部分模型参数,并负责接收从trainer发送的梯度并更新这些模型参数;每个trainer进程会保存一份\
+ 完整的模型,并使用一部分数据进行训练,然后向pserver发送梯度,最后从pserver拉取更新后的参数。
+
+ pserver进程可以在和trainer完全不同的计算节点上,也可以和trainer公用节点。一个分布式任务所需要的\
+ pserver进程个数通常需要根据实际情况调整,已达到最佳的性能,然而通常来说pserver的进程不会比trainer\
+ 更多。
+
+ 在使用GPU训练时,pserver可以选择使用GPU或只使用CPU,如果pserver也使用GPU,则会增加一次从CPU拷贝\
+ 接收到的梯度数据到GPU的开销,在某些情况下会导致整体训练性能降低。
+
+- NCCL2通信方式的结构:
+
+ .. image:: src/dist_train_nccl2.png
+
+ 使用NCCL2(Collective通信方式)进行分布式训练,是不需要启动pserver进程的,每个trainer进程都保存\
+ 一份完整的模型参数,在完成计算梯度之后通过trainer之间的相互通信,Reduce梯度数据到所有节点的所有设备\
+ 然后每个节点在各自完成参数更新。
+
+使用parameter server方式的训练
+------------------------------
+
+使用 :code:`trainer` API,程序可以自动的通过识别环境变量决定是否已分布式方式执行。
+
+.. csv-table:: 需要在您的分布式环境中配置的环境变量包括:
+ :header: "环境变量", "说明"
+
+ "PADDLE_TRAINING_ROLE", "当前进程的角色,可以是PSERVER或TRAINER"
+ "PADDLE_PSERVER_PORT", "parameter使用的端口"
+ "PADDLE_PSERVER_IPS", "parameter server的IP地址列表,用逗号分开"
+ "PADDLE_TRAINERS", "分布式任务中trainer节点的个数"
+ "PADDLE_CURRENT_IP", "当前节点的IP"
+ "PADDLE_TRAINER_ID", "trainer节点的id,从0~n-1,不能有重复"
+
+使用更加底层的 :code:`transpiler` API可以提供自定义的分布式训练的方法,比如可以在同一台机器上,
+启动多个pserver和trainer进行训练,使用底层API的方法可以参考下面的样例代码:
+
+.. code-block:: python
+
+ role = "PSERVER"
+ trainer_id = 0
+ pserver_endpoints = "127.0.0.1:6170,127.0.0.1:6171"
+ current_endpoint = "127.0.0.1:6170"
+ trainers = 4
+ t = fluid.DistributeTranspiler()
+ t.transpile(trainer_id, pservers=pserver_endpoints, trainers=trainers)
+ if role == "PSERVER":
+ pserver_prog = t.get_pserver_program(current_endpoint)
+ pserver_startup = t.get_startup_program(current_endpoint,
+ pserver_prog)
+ exe.run(pserver_startup)
+ exe.run(pserver_prog)
+ elif role == "TRAINER":
+ train_loop(t.get_trainer_program())
+
+
+选择同步或异步训练
+++++++++++++++++++
+
+Fluid分布式任务可以支持同步训练或异步训练,在同步训练方式下,所有的trainer节点,会在每个mini-batch
+同步地合并所有节点的梯度数据并发送给parameter server完成更新,在异步训练方式下,每个trainer没有相互\
+同步等待的过程,可以独立的parameter server的参数。通常情况下,使用异步训练方式,可以在trainer节点\
+更多的时候比同步训练方式有更高的总体吞吐量。
+
+在调用 :code:`transpile` 函数时,默认会生成同步训练的分布式程序,通过指定 :code:`sync_mode=False`
+参数即可生成异步训练的程序:
+
+.. code-block:: python
+
+ t.transpile(trainer_id, pservers=pserver_endpoints, trainers=trainers, sync_mode=False)
+
+
+选择参数分布方法
+++++++++++++++++
+
+参数 :code:`split_method` 可以指定参数在parameter server上的分布方式。
+
+Fluid默认使用 `RoundRobin `_
+方式将参数分布在多个parameter server上。此方式在默认未关闭参数切分的情况下,参数会较平均的分布在所有的
+parameter server上。如果需要使用其他,可以传入其他的方法,目前可选的方法有: :code:`RoundRobin` 和
+:code:`HashName` 。也可以使用自定义的分布方式,只需要参考
+`这里 `_
+编写自定义的分布函数。
+
+
+关闭切分参数
+++++++++++++
+
+参数 :code:`slice_var_up` 指定是否将较大(大于8192个元素)的参数切分到多个parameter server已均衡计算负载,默认为开启。
+
+当模型中的可训练参数体积比较均匀或者使用自定义的参数分布方法是参数均匀分布在多个parameter server上,
+可以选择关闭切分参数,这样可以降低切分和重组带来的计算和拷贝开销:
+
+.. code-block:: python
+
+ t.transpile(trainer_id, pservers=pserver_endpoints, trainers=trainers, slice_var_up=False)
+
+
+使用NCCL2通信方式的训练
+--------------------
+
+注NCCL2模式目前仅支持trainer API,NCCL2方式并没有很多可选项,也没有"transpiler",所以并没有底层API。
+使用NCCL2方式同样需要配置每个节点的环境变量,此处与parameter server模式有所不同,并不需要启动独立的\
+parameter server的进程,只需要启动多个trainer进程即可。
+
+
+.. csv-table:: NCCL2模式环境变量说明:
+ :header: "环境变量", "说明"
+
+ "PADDLE_TRAINER_IPS", "所有Trainer节点的IP列表,用逗号分隔"
+ "PADDLE_TRAINER_ID", "trainer节点的id,从0~n-1,不能有重复"
+ "PADDLE_PSERVER_PORT", "一个端口,用于在NCCL2初始化时,广播NCCL ID"
+ "PADDLE_CURRENT_IP", "当前节点的IP"
+
+目前使用NCCL2进行分布式训练仅支持同步训练方式。使用NCCL2方式的分布式训练,更适合模型体积较大,并需要使用\
+同步训练和GPU训练,如果硬件设备支持RDMA和GPU Direct,可以达到很高的分布式训练性能。
+
+注意如果系统中有多个网络设备,需要手动指定NCCL2使用的设备,
+假设需要使用 :code:`eth2` 为通信设备,需要设定如下环境变量:
+
+.. code-block:: bash
+
+ export NCCL_SOCKET_IFNAME=eth2
+
+另外NCCL2提供了其他的开关环境变量,比如指定是否开启GPU Direct,是否使用RDMA等,详情可以参考
+`ncclknobs `_ 。
diff --git a/doc/fluid/new_docs/user_guides/howto/training/cluster_quick_start.rst b/doc/fluid/new_docs/user_guides/howto/training/cluster_quick_start.rst
new file mode 100644
index 0000000000000000000000000000000000000000..6131c92d6f5386c7e91b2917d25dd7ae830ff182
--- /dev/null
+++ b/doc/fluid/new_docs/user_guides/howto/training/cluster_quick_start.rst
@@ -0,0 +1,143 @@
+.. _cluster_quick_start:
+
+分布式训练快速开始
+==================
+
+准备工作
+--------
+
+在本篇文章中,我们将会在介绍如何快速在一个集群中启动一个 PaddlePaddle
+的分布式训练任务,在开始之前,请按如下步骤做些准备工作:
+
+1. 准备一个至少4个节点的集群,并且保证网络可以联通,在本文中我们使用
+ ``*.paddlepaddle.com`` 来表示每个节点的主机名称,您可以根据集群的实际情况来修改它。
+
+2. 在开始之前确保已经阅读过 :ref:`how_to_install`
+ 并且可以在集群的所有节点上可以正常运行 PaddlePaddle。
+
+启动集群训练任务
+----------------
+
+在启动集群训练脚本时,需要在不同的节点上指定不同的环境变量,具体如下:
+
++-----------------+-----------------+-----------------+---------------------+
+| 环境变量 | 数据类型 | 样例 | 描述 |
++=================+=================+=================+=====================+
+| PADDLE_TRAINING | str | PSERVER,TRAINER | 训练节点的角色 |
+| _ROLE | | | |
++-----------------+-----------------+-----------------+---------------------+
+| PADDLE_PSERVER_ | str | ps0.paddlepaddl | 所有 pserver |
+| IPS | | e.com,ps1.paddl | 节点的 IP |
+| | | epaddle.com… | 地址或 |
+| | | | hostname, |
+| | | | 用“,”分隔 |
++-----------------+-----------------+-----------------+---------------------+
+| PADDLE_PSERVER_ | int | 6174 | pserver |
+| PORT | | | 节点监听的端口 |
++-----------------+-----------------+-----------------+---------------------+
+| PADDLE_TRAINERS | int | 2 | 训练任务中 |
+| | | | trainer |
+| | | | 节点的数量 |
++-----------------+-----------------+-----------------+---------------------+
+| PADDLE_CURRENT_ | str | ps0.paddlepaddl | 当前 pserver |
+| IP | | e.com | 节点的 IP |
+| | | | 地址或 hostanme |
++-----------------+-----------------+-----------------+---------------------+
+| PADDLE_TRAINER_ | int | 0 | 当前 trainer |
+| ID | | | 节点的唯一 ID, |
+| | | | 取值范围为从0开始到 |
+| | | | PADDLE_TRAINERS-1 |
++-----------------+-----------------+-----------------+---------------------+
+
+样例代码
+~~~~~~~~
+
+将下面程序代码保存为 ``fluid_dist.py``
+
+.. code:: python
+
+ import paddle
+ import paddle.fluid as fluid
+ import contextlib
+ import numpy
+ import unittest
+
+ # train reader
+ BATCH_SIZE = 20
+
+ train_reader = paddle.batch(
+ paddle.reader.shuffle(
+ paddle.dataset.uci_housing.train(), buf_size=500),
+ batch_size=BATCH_SIZE)
+
+ test_reader = paddle.batch(
+ paddle.reader.shuffle(
+ paddle.dataset.uci_housing.test(), buf_size=500),
+ batch_size=BATCH_SIZE)
+
+
+ def train_program():
+ y = fluid.layers.data(name='y', shape=[1], dtype='float32')
+ x = fluid.layers.data(name='x', shape=[13], dtype='float32')
+ y_predict = fluid.layers.fc(input=x, size=1, act=None)
+
+ loss = fluid.layers.square_error_cost(input=y_predict, label=y)
+ avg_loss = fluid.layers.mean(loss)
+
+ return avg_loss
+
+ def optimizer_func():
+ return fluid.optimizer.SGD(learning_rate=0.001)
+
+ def train(use_cuda, train_program):
+ place = fluid.CUDAPlace(0) if use_cuda else fluid.CPUPlace()
+
+ trainer = fluid.Trainer(
+ train_func=train_program, place=place, optimizer_func=optimizer_func)
+
+ def event_handler(event):
+ if isinstance(event, fluid.EndStepEvent):
+ if event.step == 10:
+ test_metrics = trainer.test(
+ reader=test_reader, feed_order=['x', 'y'])
+ print("step {0}, loss: {1}".format(event.step, test_metrics))
+ trainer.stop()
+
+ trainer.train(
+ reader=train_reader,
+ num_epochs=100,
+ event_handler=event_handler,
+ feed_order=['x', 'y'])
+
+ train(False, train_program)
+
+启动trainer节点和pserver节点
+~~~~~~~~~~~~~~~~~~~~~~~~~~~~
+
+.. list-table::
+ :header-rows: 1
+
+ * - 启动节点
+ - 启动命令
+ - 说明
+ * - ps0.paddlepaddle.com
+ - :code:`PADDLE_TRAINING_ROLE=PSERVER PADDLE_CURRENT_IP=ps0.paddlepaddle.com PADDLE_PSERVER_IPS=ps0.paddlepaddle.com,ps1.paddlepaddle.com PADDLE_TRAINERS=2 PADDLE_PSERVER_PORT=6174 python fluid_dist.py`
+ - 启动 pserver 节点
+ * - ps1.paddlepaddle.com
+ - :code:`PADDLE_TRAINING_ROLE=PSERVER PADDLE_CURRENT_IP=ps1.paddlepaddle.com PADDLE_PSERVER_IPS=ps0.paddlepaddle.com,ps1.paddlepaddle.com PADDLE_TRAINERS=2 PADDLE_PSERVER_PORT=6174 python fluid_dist.py`
+ - 启动 pserver 节点
+ * - trainer0.paddlepaddle.com
+ - :code:`PADDLE_TRAINING_ROLE=TRAINER PADDLE_PSERVER_IPS=ps0.paddlepaddle.com,ps1.paddlepaddle.com PADDLE_TRAINERS=2 PADDLE_TRAINER_ID=0 PADDLE_PSERVER_PORT=6174 python fluid_dist.py`
+ - 启动第0号 trainer 节点
+ * - trainer1.paddlepaddle.com
+ - :code:`PADDLE_TRAINING_ROLE=TRAINER PADDLE_PSERVER_IPS=ps0.paddlepaddle.com,ps1.paddlepaddle.com PADDLE_TRAINERS=2 PADDLE_TRAINER_ID=1 PADDLE_PSERVER_PORT=6174 python fluid_dist.py`
+ - 启动第1号 trainer 节点
+
+**注意**
+
+- 需要先启动pserver节点再启动trainer节点
+- 看到trainer节点输出如下日志表示训练任务执行正确
+
+ .. code:: bash
+
+ step 10, loss: [258.2326202392578]
diff --git a/doc/fluid/new_docs/user_guides/howto/training/index.rst b/doc/fluid/new_docs/user_guides/howto/training/index.rst
new file mode 100644
index 0000000000000000000000000000000000000000..68475101e26b3f695c8003995cc1c6a95426ff27
--- /dev/null
+++ b/doc/fluid/new_docs/user_guides/howto/training/index.rst
@@ -0,0 +1,12 @@
+############
+训练神经网络
+############
+
+PaddlePaddle Fluid支持单机训练,和多节点训练。每种训练模式下,都支持多种训练方法。
+
+.. toctree::
+ :maxdepth: 2
+
+ single_node
+ multi_node
+ save_load_variables
diff --git a/doc/fluid/new_docs/user_guides/howto/training/multi_node.rst b/doc/fluid/new_docs/user_guides/howto/training/multi_node.rst
new file mode 100644
index 0000000000000000000000000000000000000000..24316f0be0d8f211e680fa15cb432732b5967c79
--- /dev/null
+++ b/doc/fluid/new_docs/user_guides/howto/training/multi_node.rst
@@ -0,0 +1,9 @@
+########
+多机训练
+########
+
+.. toctree::
+ :maxdepth: 2
+
+ cluster_quick_start.rst
+ cluster_howto.rst
diff --git a/doc/fluid/new_docs/user_guides/howto/training/save_load_variables.rst b/doc/fluid/new_docs/user_guides/howto/training/save_load_variables.rst
new file mode 100644
index 0000000000000000000000000000000000000000..a96776f4a17a1d6da170bdff9d81771c38912bb5
--- /dev/null
+++ b/doc/fluid/new_docs/user_guides/howto/training/save_load_variables.rst
@@ -0,0 +1,172 @@
+.. _user_guide_save_load_vars:
+
+##################
+保存与载入模型变量
+##################
+
+模型变量分类
+############
+
+在PaddlePaddle Fluid中,所有的模型变量都用 :code:`fluid.Variable()` 作为基类进行表示。
+在该基类之下,模型变量主要可以分为以下几种类别:
+
+1. 模型参数
+ 模型参数是深度学习模型中被训练和学习的变量,在训练过程中,训练框架根据反向传播算法计算出每一个模型参数当前的梯度,
+ 并用优化器根据梯度对参数进行更新。模型的训练过程本质上可以看做是模型参数不断迭代更新的过程。
+ 在PaddlePaddle Fluid中,模型参数用 :code:`fluid.framework.Parameter` 来表示,
+ 这是一个 :code:`fluid.Variable()` 的派生类,除了 :code:`fluid.Variable()` 具有的各项性质以外,
+ :code:`fluid.framework.Parameter` 还可以配置自身的初始化方法、更新率等属性。
+
+2. 长期变量
+ 长期变量指的是在整个训练过程中持续存在、不会因为一个迭代的结束而被销毁的变量,例如动态调节的全局学习率等。
+ 在PaddlePaddle Fluid中,长期变量通过将 :code:`fluid.Variable()` 的 :code:`persistable`
+ 属性设置为 :code:`True` 来表示。所有的模型参数都是长期变量,但并非所有的长期变量都是模型参数。
+
+3. 临时变量
+ 不属于上面两个类别的所有模型变量都是临时变量,这种类型的变量只在一个训练迭代中存在,在每一个迭代结束后,
+ 所有的临时变量都会被销毁,然后在下一个迭代开始之前,又会先构造出新的临时变量供本轮迭代使用。
+ 一般情况下模型中的大部分变量都属于这一类别,例如输入的训练数据、一个普通的layer的输出等等。
+
+
+
+如何保存模型变量
+################
+
+根据用途的不同,我们需要保存的模型变量也是不同的。例如,如果我们只是想保存模型用来进行以后的预测,
+那么只保存模型参数就够用了。但如果我们需要保存一个checkpoint以备将来恢复训练,
+那么我们应该将各种长期变量都保存下来,甚至还需要记录一下当前的epoch和step的id。
+因为一些模型变量虽然不是参数,但对于模型的训练依然必不可少。
+
+因此,根据需求的不同,我们提供了两套API来分别进行模型的参数和checkpoint的保存。
+
+保存模型用于对新样本的预测
+==========================
+
+如果我们保存模型的目的是用于对新样本的预测,那么只保存模型参数就足够了。我们可以使用
+:code:`fluid.io.save_params()` 接口来进行模型参数的保存。
+
+例如:
+
+.. code-block:: python
+
+ import paddle.fluid as fluid
+
+ exe = fluid.Executor(fluid.CPUPlace())
+ param_path = "./my_paddle_model"
+ prog = fluid.default_main_program()
+ fluid.io.save_params(executor=exe, dirname=param_path, main_program=None)
+
+上面的例子中,通过调用 :code:`fluid.io.save_params` 函数,PaddlePaddle Fluid会对默认
+:code:`fluid.Program` 也就是 :code:`prog` 中的所有模型变量进行扫描,
+筛选出其中所有的模型参数,并将这些模型参数保存到指定的 :code:`param_path` 之中。
+
+
+保存checkpoint用于将来恢复训练
+==============================
+
+在训练过程中,我们可能希望在一些节点上将当前的训练状态保存下来,
+以便在将来需要的时候恢复训练环境继续进行训练。这一般被称作“checkpoint”。
+想要保存checkpoint,可以使用 :code:`fluid.io.save_checkpiont()` 接口。
+
+例如:
+
+.. code-block:: python
+
+ import paddle.fluid as fluid
+
+ exe = fluid.Executor(fluid.CPUPlace())
+ path = "./checkpoints"
+ prog = fluid.default_main_program()
+ trainer_args = {"epoch_id": 200,
+ "step_id": 20} # just an example
+ fluid.io.save_checkpoint(executor=exe,
+ checkpoint_dir=path,
+ trainer_id=0,
+ trainer_args=trainer_args,
+ main_program=prog,
+ max_num_checkpoints=3)
+
+上面的例子中,通过调用 :code:`fluid.io.save_checkpoint` 函数,PaddlePaddle Fluid会对默认
+:code:`fluid.Program` 也就是 :code:`prog` 中的所有模型变量进行扫描,
+根据一系列内置的规则自动筛选出其中所有需要保存的变量,并将他们保存到指定的 :code:`path` 目录下。
+
+:code:`fluid.io.save_checkpoint` 的各个参数中, :code:`trainer_id` 在单机情况下设置为0即可; :code:`trainer_args`
+为一个Python dict,用于给定当前的epoch_id和step_id;
+:code:`max_num_checkpoints` 用于表示的最大checkpoint数量,
+如果目录中已经存在的checkpoint数量超过这个值,那最早的checkpoint将被删除。
+
+如何载入模型变量
+################
+
+与模型变量的保存相对应,我们提供了两套API来分别载入模型的参数和载入模型的checkpoint。
+
+载入模型用于对新样本的预测
+==========================
+
+对于通过 :code:`fluid.io.save_params` 保存的模型,可以使用 :code:`fluid.io.load_params`
+来进行载入。
+
+例如:
+
+.. code-block:: python
+
+ import paddle.fluid as fluid
+
+ exe = fluid.Executor(fluid.CPUPlace())
+ param_path = "./my_paddle_model"
+ prog = fluid.default_main_program()
+ fluid.io.load_params(executor=exe, dirname=param_path,
+ main_program=prog)
+
+上面的例子中,通过调用 :code:`fluid.io.load_params` 函数,PaddlePaddle Fluid会对
+:code:`prog` 中的所有模型变量进行扫描,筛选出其中所有的模型参数,
+并尝试从 :code:`param_path` 之中读取加载它们。
+
+需要格外注意的是,这里的 :code:`prog` 必须和调用 :code:`fluid.io.save_params`
+时所用的 :code:`prog` 中的前向部分完全一致,且不能包含任何参数更新的操作。如果两者存在不一致,
+那么可能会导致一些变量未被正确加载;如果错误地包含了参数更新操作,那可能会导致正常预测过程中参数被更改。
+这两个 :code:`fluid.Program` 之间的关系类似于训练 :code:`fluid.Program`
+和测试 :code:`fluid.Program` 之间的关系,详见: :ref:`user_guide_test_while_training`。
+
+另外,需特别注意运行 :code:`fluid.default_startup_program()` 必须在调用 :code:`fluid.io.load_params`
+之前。如果在之后运行,可能会覆盖已加载的模型参数导致错误。
+
+
+载入checkpoint用于恢复训练
+==========================
+
+对于通过 :code:`fluid.io.save_checkpoint` 保存的模型,可以使用 :code:`fluid.io.load_checkpoint`
+来进行载入。
+
+例如:
+
+.. code-block:: python
+
+ import paddle.fluid as fluid
+
+ exe = fluid.Executor(fluid.CPUPlace())
+ path = "./checkpoints"
+ prog = fluid.default_main_program()
+ fluid.io.load_checkpoint(executor=exe, checkpoint_dir=path,
+ serial=9, main_program=prog)
+
+上面的例子中,通过调用 :code:`fluid.io.save_checkpoint` 函数,PaddlePaddle Fluid会对
+:code:`prog` 中的所有模型变量进行扫描,根据内置规则自动筛选出需要加载的变量,
+并尝试从 :code:`path` 之中加载它们。
+
+参数 :code:`serial` 用来标记具体要加载的checkpoint的版本号。在保存checkpoint的时候,
+一个checkpoint会被保存在一个子目录中,并在目录名上体现出自己的版本号。
+一般越大的版本号表示这个checkpoint越新。
+
+这里的 :code:`prog` 必须和调用 :code:`fluid.io.save_checkpoint` 时所用的 :code:`prog`
+完全一致,否则会导致变量加载错误或者未加载。另外,与 :code:`fluid.io.save_params` 类似,
+运行 :code:`fluid.default_startup_program()` 也必须在 :code:`fluid.io.load_checkpoint`
+之前进行。
+
+多机checkpoint保存
+##################
+
+.. toctree::
+ :maxdepth: 2
+
+ checkpoint_doc_cn.md
\ No newline at end of file
diff --git a/doc/fluid/new_docs/user_guides/howto/training/single_node.rst b/doc/fluid/new_docs/user_guides/howto/training/single_node.rst
new file mode 100644
index 0000000000000000000000000000000000000000..23eac0f831f2d6d052b7fc35b536d4ab633df851
--- /dev/null
+++ b/doc/fluid/new_docs/user_guides/howto/training/single_node.rst
@@ -0,0 +1,119 @@
+########
+单机训练
+########
+
+准备工作
+########
+
+要进行PaddlePaddle Fluid单机训练,需要先 :ref:`user_guide_prepare_data` 和
+:ref:`user_guide_configure_simple_model` 。当\
+:ref:`user_guide_configure_simple_model` 完毕后,可以得到两个\
+:code:`fluid.Program`, :code:`startup_program` 和 :code:`main_program`。
+默认情况下,可以使用 :code:`fluid.default_startup_program()` 与\ :code:`fluid.default_main_program()` 获得全局的 :code:`fluid.Program`。
+
+例如:
+
+.. code-block:: python
+
+ import paddle.fluid as fluid
+
+ image = fluid.layers.data(name="image", shape=[784])
+ label = fluid.layers.data(name="label", shape=[1])
+ hidden = fluid.layers.fc(input=image, size=100, act='relu')
+ prediction = fluid.layers.fc(input=hidden, size=10, act='softmax')
+ loss = fluid.layers.mean(
+ fluid.layers.cross_entropy(
+ input=prediction,
+ label=label
+ )
+ )
+
+ sgd = fluid.optimizer.SGD(learning_rate=0.001)
+ sgd.minimize(loss)
+
+ # Here the fluid.default_startup_program() and fluid.default_main_program()
+ # has been constructed.
+
+在上述模型配置执行完毕后, :code:`fluid.default_startup_program()` 与\
+:code:`fluid.default_main_program()` 配置完毕了。
+
+初始化参数
+##########
+
+参数随机初始化
+==============
+
+用户配置完模型后,参数初始化操作会被写入到\
+:code:`fluid.default_startup_program()` 中。使用 :code:`fluid.Executor()` 运行
+这一程序,即可在全局 :code:`fluid.global_scope()` 中随机初始化参数。例如:
+
+.. code-block:: python
+
+ exe = fluid.Executor(fluid.CUDAPlace(0))
+ exe.run(program=fluid.default_startup_program())
+
+值得注意的是: 如果使用多GPU训练,参数需要先在GPU0上初始化,再经由\
+:code:`fluid.ParallelExecutor` 分发到多张显卡上。
+
+
+载入预定义参数
+==============
+
+在神经网络训练过程中,经常会需要载入预定义模型,进而继续进行训练。\
+如何载入预定义参数,请参考 :ref:`user_guide_save_load_vars`。
+
+
+单卡训练
+########
+
+执行单卡训练可以使用 :code:`fluid.Executor()` 中的 :code:`run()` 方法,运行训练\
+:code:`fluid.Program` 即可。在运行的时候,用户可以通过 :code:`run(feed=...)`\
+参数传入数据;用户可以通过 :code:`run(fetch=...)` 获取持久的数据。例如:\
+
+.. code-block:: python
+
+ ...
+ loss = fluid.layers.mean(...)
+
+ exe = fluid.Executor(...)
+ # the result is an numpy array
+ result = exe.run(feed={"image": ..., "label": ...}, fetch_list=[loss])
+
+这里有几点注意事项:
+
+1. feed的数据格式,请参考文章 :ref:`user_guide_feed_data_to_executor`。
+2. :code:`Executor.run` 的返回值是 :code:`fetch_list=[...]` 的variable值。被fetch\
+ 的Variable必须是persistable的。 :code:`fetch_list` 可以传入Variable的列表,\
+ 也可以传入Variable的名字列表。:code:`Executor.run` 返回Fetch结果列表。
+3. 如果需要取回的数据包含序列信息,可以设置
+ :code:`exe.run(return_numpy=False, ...)` 直接返回 :code:`fluid.LoDTensor`
+ 。用户可以直接访问 :code:`fluid.LoDTensor` 中的信息。
+
+多卡训练
+########
+
+执行多卡训练可以使用 :code:`fluid.ParallelExecutor` 运行训练
+:code:`fluid.Program`。例如:
+
+.. code-block:: python
+
+ train_exe = fluid.ParallelExecutor(use_cuda=True, loss_name=loss.name,
+ main_program=fluid.default_main_program())
+ train_exe.run(fetch_list=[loss.name], feed={...})
+
+这里有几点注意事项:
+
+1. :code:`ParallelExecutor` 的构造函数需要指明要执行的 :code:`fluid.Program` ,
+ 并在执行过程中不能修改。默认值是 :code:`fluid.default_main_program()` 。
+2. :code:`ParallelExecutor` 需要明确指定是否使用 CUDA 显卡进行训练。在显卡训练\
+ 模式下会占用全部显卡。用户可以配置 `CUDA_VISIBLE_DEVICES `_ 来修改占用\
+ 的显卡。
+
+进阶使用
+########
+
+.. toctree::
+ :maxdepth: 2
+
+ test_while_training
+ save_load_variables
diff --git a/doc/fluid/new_docs/user_guides/howto/training/src/dist_train_nccl2.graffle b/doc/fluid/new_docs/user_guides/howto/training/src/dist_train_nccl2.graffle
new file mode 100644
index 0000000000000000000000000000000000000000..16f6b8835c4ffb82babca56b62ba44494fd6a947
Binary files /dev/null and b/doc/fluid/new_docs/user_guides/howto/training/src/dist_train_nccl2.graffle differ
diff --git a/doc/fluid/new_docs/user_guides/howto/training/src/dist_train_nccl2.png b/doc/fluid/new_docs/user_guides/howto/training/src/dist_train_nccl2.png
new file mode 100644
index 0000000000000000000000000000000000000000..587a1a48affdde6809d7f8bf77e1055db7cd8c14
Binary files /dev/null and b/doc/fluid/new_docs/user_guides/howto/training/src/dist_train_nccl2.png differ
diff --git a/doc/fluid/new_docs/user_guides/howto/training/src/dist_train_pserver.graffle b/doc/fluid/new_docs/user_guides/howto/training/src/dist_train_pserver.graffle
new file mode 100644
index 0000000000000000000000000000000000000000..046c4903231e8ca441884674c08b381766c0bbae
Binary files /dev/null and b/doc/fluid/new_docs/user_guides/howto/training/src/dist_train_pserver.graffle differ
diff --git a/doc/fluid/new_docs/user_guides/howto/training/src/dist_train_pserver.png b/doc/fluid/new_docs/user_guides/howto/training/src/dist_train_pserver.png
new file mode 100644
index 0000000000000000000000000000000000000000..cd2f92ad1a14ac12efc2c257c8aa3d1ae403b2b1
Binary files /dev/null and b/doc/fluid/new_docs/user_guides/howto/training/src/dist_train_pserver.png differ
diff --git a/doc/fluid/new_docs/user_guides/howto/training/src/parallelism.png b/doc/fluid/new_docs/user_guides/howto/training/src/parallelism.png
new file mode 100644
index 0000000000000000000000000000000000000000..6c078b5241559a05219447db67b5d8a35aeefd3f
Binary files /dev/null and b/doc/fluid/new_docs/user_guides/howto/training/src/parallelism.png differ
diff --git a/doc/fluid/new_docs/user_guides/howto/training/test_while_training.rst b/doc/fluid/new_docs/user_guides/howto/training/test_while_training.rst
new file mode 100644
index 0000000000000000000000000000000000000000..37d5c0d78179ccead7a81dffb4ae2f0d835a5949
--- /dev/null
+++ b/doc/fluid/new_docs/user_guides/howto/training/test_while_training.rst
@@ -0,0 +1,120 @@
+.. _user_guide_test_while_training:
+
+##################
+训练过程中评测模型
+##################
+
+模型的测试评价与训练的 :code:`fluid.Program` 不同。在测试评价中:
+
+1. 评价测试不进行反向传播,不优化更新参数。
+2. 评价测试执行的操作可以不同。
+
+ * 例如 BatchNorm 操作,在训练和测试时执行不同的算法。
+
+ * 评价模型与训练相比可以是完全不同的模型。
+
+生成测试 :code:`fluid.Program`
+#################################
+
+通过克隆训练 :code:`fluid.Program` 生成测试 :code:`fluid.Program`
+=======================================================================
+
+:code:`Program.clone()` 方法可以复制出新的 :code:`fluid.Program` 。 通过设置
+:code:`Program.clone(for_test=True)` 复制含有用于测试的操作Program。简单的使用方法如下:
+
+.. code-block:: python
+
+ import paddle.fluid as fluid
+
+ img = fluid.layers.data(name="image", shape=[784])
+ prediction = fluid.layers.fc(
+ input=fluid.layers.fc(input=img, size=100, act='relu'),
+ size=10,
+ act='softmax'
+ )
+ label = fluid.layers.data(name="label", shape=[1], dtype="int64")
+ loss = fluid.layers.mean(fluid.layers.cross_entropy(input=prediction, label=label))
+ acc = fluid.layers.accuracy(input=prediction, label=label)
+
+ test_program = fluid.default_main_program().clone(for_test=True)
+
+ adam = fluid.optimizer.Adam(learning_rate=0.001)
+ adam.minimize(loss)
+
+在使用 :code:`Optimizer` 之前,将 :code:`fluid.default_main_program()` 复制\
+成一个 :code:`test_program` 。之后使用测试数据运行 :code:`test_program`,\
+就可以做到运行测试程序,而不影响训练结果。
+
+分别配置训练 :code:`fluid.Program` 和测试 :code:`fluid.Program`
+=====================================================================
+
+如果训练程序和测试程序相差较大时,用户也可以通过完全定义两个不同的
+:code:`fluid.Program`,分别进行训练和测试。在PaddlePaddle Fluid中,\
+所有的参数都有名字。如果两个不同的操作,甚至两个不同的网络使用了同样名字的参数,\
+那么他们的值和内存空间都是共享的。
+
+PaddlePaddle Fluid中使用 :code:`fluid.unique_name` 包来随机初始化用户未定义的\
+参数名称。通过 :code:`fluid.unique_name.guard` 可以确保多次调用某函数\
+参数初始化的名称一致。
+
+例如:
+
+.. code-block:: python
+
+ import paddle.fluid as fluid
+
+ def network(is_test):
+ file_obj = fluid.layers.open_files(filenames=["test.recordio"] if is_test else ["train.recordio"], ...)
+ img, label = fluid.layers.read_file(file_obj)
+ hidden = fluid.layers.fc(input=img, size=100, act="relu")
+ hidden = fluid.layers.batch_norm(input=hidden, is_test=is_test)
+ ...
+ return loss
+
+ with fluid.unique_name.guard():
+ train_loss = network(is_test=False)
+ sgd = fluid.optimizer.SGD(0.001)
+ sgd.minimize(train_loss)
+
+ test_program = fluid.Program()
+ with fluid.unique_name.guard():
+ with fluid.program_gurad(test_program, fluid.Program()):
+ test_loss = network(is_test=True)
+
+ # fluid.default_main_program() is the train program
+ # fluid.test_program is the test program
+
+执行测试 :code:`fluid.Program`
+#################################
+
+使用 :code:`Executor` 执行测试 :code:`fluid.Program`
+=======================================================
+
+用户可以使用 :code:`Executor.run(program=...)` 来执行测试
+:code:`fluid.Program`。
+
+例如
+
+.. code-block:: python
+
+ exe = fluid.Executor(fluid.CPUPlace())
+ test_acc = exe.run(program=test_program, feed=test_data_batch, fetch_list=[acc])
+ print 'Test accuracy is ', test_acc
+
+使用 :code:`ParallelExecutor` 执行测试 :code:`fluid.Program`
+===============================================================
+
+用户可以使用训练用的 :code:`ParallelExecutor` 与测试 :code:`fluid.Program`
+一起新建一个测试的 :code:`ParallelExecutor` ;再使用测试
+:code:`ParallelExecutor.run` 来执行测试。
+
+例如:
+
+.. code-block:: python
+
+ train_exec = fluid.ParallelExecutor(use_cuda=True, loss_name=loss.name)
+
+ test_exec = fluid.ParallelExecutor(use_cuda=True, share_vars_from=train_exec,
+ main_program=test_program)
+ test_acc = test_exec.run(fetch_list=[acc], ...)
+
diff --git a/doc/fluid/new_docs/user_guides/index.rst b/doc/fluid/new_docs/user_guides/index.rst
new file mode 100644
index 0000000000000000000000000000000000000000..453cb71cfdf72e031ce0f0517e2db936eca38dfc
--- /dev/null
+++ b/doc/fluid/new_docs/user_guides/index.rst
@@ -0,0 +1,18 @@
+########
+使用指南
+########
+
+
+.. todo::
+
+ 完善导引介绍
+
+.. toctree::
+ :maxdepth: 2
+
+ howto/prepare_data/index
+ howto/configure_simple_model/index
+ howto/training/index
+ howto/debug/index
+ howto/evaluation/index
+ models/index.rst
diff --git a/doc/fluid/new_docs/user_guides/models/index.rst b/doc/fluid/new_docs/user_guides/models/index.rst
new file mode 100644
index 0000000000000000000000000000000000000000..998e95c4885dc313d9449f5466f80c53d34fe82a
--- /dev/null
+++ b/doc/fluid/new_docs/user_guides/models/index.rst
@@ -0,0 +1,137 @@
+Fluid 模型库
+============
+
+图像分类
+--------
+
+图像分类是根据图像的语义信息对不同类别图像进行区分,是计算机视觉中重要的基础问题,是物体检测、图像分割、物体跟踪、行为分析、人脸识别等其他高层视觉任务的基础,在许多领域都有着广泛的应用。如:安防领域的人脸识别和智能视频分析等,交通领域的交通场景识别,互联网领域基于内容的图像检索和相册自动归类,医学领域的图像识别等。
+
+在深度学习时代,图像分类的准确率大幅度提升,在图像分类任务中,我们向大家介绍了如何在经典的数据集ImageNet上,训练常用的模型,包括AlexNet、VGG、GoogLeNet、ResNet、Inception-v4、MobileNet、DPN(Dual
+Path
+Network)、SE-ResNeXt模型,也开源了\ `训练的模型 `__\ 方便用户下载使用。同时提供了能够将Caffe模型转换为PaddlePaddle
+Fluid模型配置和参数文件的工具。
+
+- `AlexNet `__
+- `VGG `__
+- `GoogleNet `__
+- `Residual
+ Network `__
+- `Inception-v4 `__
+- `MobileNet `__
+- `Dual Path
+ Network `__
+- `SE-ResNeXt `__
+- `Caffe模型转换为Paddle
+ Fluid配置和模型文件工具 `__
+
+目标检测
+--------
+
+目标检测任务的目标是给定一张图像或是一个视频帧,让计算机找出其中所有目标的位置,并给出每个目标的具体类别。对于人类来说,目标检测是一个非常简单的任务。然而,计算机能够“看到”的是图像被编码之后的数字,很难解图像或是视频帧中出现了人或是物体这样的高层语义概念,也就更加难以定位目标出现在图像中哪个区域。与此同时,由于目标会出现在图像或是视频帧中的任何位置,目标的形态千变万化,图像或是视频帧的背景千差万别,诸多因素都使得目标检测对计算机来说是一个具有挑战性的问题。
+
+在目标检测任务中,我们介绍了如何基于\ `PASCAL
+VOC `__\ 、\ `MS
+COCO `__\ 数据训练通用物体检测模型,当前介绍了SSD算法,SSD全称Single Shot MultiBox Detector,是目标检测领域较新且效果较好的检测算法之一,具有检测速度快且检测精度高的特点。
+
+开放环境中的检测人脸,尤其是小的、模糊的和部分遮挡的人脸也是一个具有挑战的任务。我们也介绍了如何基于 `WIDER FACE `_ 数据训练百度自研的人脸检测PyramidBox模型,该算法于2018年3月份在WIDER FACE的多项评测中均获得 `第一名 `_。
+
+- `Single Shot MultiBox
+ Detector `__
+- `Face Detector: PyramidBox `_
+
+图像语义分割
+------------
+
+图像语意分割顾名思义是将图像像素按照表达的语义含义的不同进行分组/分割,图像语义是指对图像内容的理解,例如,能够描绘出什么物体在哪里做了什么事情等,分割是指对图片中的每个像素点进行标注,标注属于哪一类别。近年来用在无人车驾驶技术中分割街景来避让行人和车辆、医疗影像分析中辅助诊断等。
+
+在图像语义分割任务中,我们介绍如何基于图像级联网络(Image Cascade
+Network,ICNet)进行语义分割,相比其他分割算法,ICNet兼顾了准确率和速度。
+
+- `ICNet `__
+
+场景文字识别
+------------
+
+许多场景图像中包含着丰富的文本信息,对理解图像信息有着重要作用,能够极大地帮助人们认知和理解场景图像的内容。场景文字识别是在图像背景复杂、分辨率低下、字体多样、分布随意等情况下,将图像信息转化为文字序列的过程,可认为是一种特别的翻译过程:将图像输入翻译为自然语言输出。场景图像文字识别技术的发展也促进了一些新型应用的产生,如通过自动识别路牌中的文字帮助街景应用获取更加准确的地址信息等。
+
+在场景文字识别任务中,我们介绍如何将基于CNN的图像特征提取和基于RNN的序列翻译技术结合,免除人工定义特征,避免字符分割,使用自动学习到的图像特征,完成端到端地无约束字符定位和识别。当前,介绍了CRNN-CTC模型,后续会引入基于注意力机制的序列到序列模型。
+
+- `CRNN-CTC模型 `__
+
+语音识别
+--------
+
+自动语音识别(Automatic Speech Recognition,
+ASR)是将人类声音中的词汇内容转录成计算机可输入的文字的技术。语音识别的相关研究经历了漫长的探索过程,在HMM/GMM模型之后其发展一直较为缓慢,随着深度学习的兴起,其迎来了春天。在多种语言识别任务中,将深度神经网络(DNN)作为声学模型,取得了比GMM更好的性能,使得
+ASR
+成为深度学习应用最为成功的领域之一。而由于识别准确率的不断提高,有越来越多的语言技术产品得以落地,例如语言输入法、以智能音箱为代表的智能家居设备等
+—— 基于语言的交互方式正在深刻的改变人类的生活。
+
+与 `DeepSpeech `__
+中深度学习模型端到端直接预测字词的分布不同,本实例更接近传统的语言识别流程,以音素为建模单元,关注语言识别中声学模型的训练,利用\ `kaldi `__\ 进行音频数据的特征提取和标签对齐,并集成
+kaldi 的解码器完成解码。
+
+- `DeepASR `__
+
+机器翻译
+--------
+
+机器翻译(Machine
+Translation)将一种自然语言(源语言)转换成一种自然语言(目标语音),是自然语言处理中非常基础和重要的研究方向。在全球化的浪潮中,机器翻译在促进跨语言文明的交流中所起的重要作用是不言而喻的。其发展经历了统计机器翻译和基于神经网络的神经机器翻译(Nueural
+Machine Translation, NMT)等阶段。在 NMT
+成熟后,机器翻译才真正得以大规模应用。而早阶段的 NMT
+主要是基于循环神经网络 RNN
+的,其训练过程中当前时间步依赖于前一个时间步的计算,时间步之间难以并行化以提高训练速度。因此,非
+RNN 结构的 NMT 得以应运而生,例如基于卷积神经网络 CNN
+的结构和基于自注意力机制(Self-Attention)的结构。
+
+本实例所实现的 Transformer
+就是一个基于自注意力机制的机器翻译模型,其中不再有RNN或CNN结构,而是完全利用
+Attention 学习语言中的上下文依赖。相较于RNN/CNN,
+这种结构在单层内计算复杂度更低、易于并行化、对长程依赖更易建模,最终在多种语言之间取得了最好的翻译效果。
+
+- `Transformer `__
+
+强化学习
+--------
+
+强化学习是近年来一个愈发重要的机器学习方向,特别是与深度学习相结合而形成的深度强化学习(Deep
+Reinforcement Learning,
+DRL),取得了很多令人惊异的成就。人们所熟知的战胜人类顶级围棋职业选手的
+AlphaGo 就是 DRL
+应用的一个典型例子,除游戏领域外,其它的应用还包括机器人、自然语言处理等。
+
+深度强化学习的开山之作是在Atari视频游戏中的成功应用,
+其可直接接受视频帧这种高维输入并根据图像内容端到端地预测下一步的动作,所用到的模型被称为深度Q网络(Deep
+Q-Network, DQN)。本实例就是利用PaddlePaddle Fluid这个灵活的框架,实现了
+DQN 及其变体,并测试了它们在 Atari 游戏中的表现。
+
+- `DeepQNetwork `__
+
+中文词法分析
+------------
+
+中文分词(Word Segmentation)是将连续的自然语言文本,切分出具有语义合理性和完整性的词汇序列的过程。因为在汉语中,词是承担语义的最基本单位,切词是文本分类、情感分析、信息检索等众多自然语言处理任务的基础。 词性标注(Part-of-speech Tagging)是为自然语言文本中的每一个词汇赋予一个词性的过程,这里的词性包括名词、动词、形容词、副词等等。 命名实体识别(Named Entity Recognition,NER)又称作“专名识别”,是指识别自然语言文本中具有特定意义的实体,主要包括人名、地名、机构名、专有名词等。 我们将这三个任务统一成一个联合任务,称为词法分析任务,基于深度神经网络,利用海量标注语料进行训练,提供了一个端到端的解决方案。
+
+我们把这个联合的中文词法分析解决方案命名为LAC。LAC既可以认为是Lexical Analysis of Chinese的首字母缩写,也可以认为是LAC Analyzes Chinese的递归缩写。
+
+- `LAC `__
+
+情感倾向分析
+------------
+
+情感倾向分析针对带有主观描述的中文文本,可自动判断该文本的情感极性类别并给出相应的置信度。情感类型分为积极、消极、 中性。情感倾向分析能够帮助企业理解用户消费习惯、分析热点话题和危机舆情监控,为企业提供有力的决策支持。本次我们开放 AI开放平台中情感倾向分析采用的模型(http://ai.baidu.com/tech/nlp/sentiment_classify ), 提供给用户使用。
+
+- `Senta `__
+
+AnyQ
+----
+
+`AnyQ `__\ (ANswer Your Questions)
+开源项目主要包含面向FAQ集合的问答系统框架、文本语义匹配工具SimNet。
+问答系统框架采用了配置化、插件化的设计,各功能均通过插件形式加入,当前共开放了20+种插件。开发者可以使用AnyQ系统快速构建和定制适用于特定业务场景的FAQ问答系统,并加速迭代和升级。
+
+SimNet是百度自然语言处理部于2013年自主研发的语义匹配框架,该框架在百度各产品上广泛应用,主要包括BOW、CNN、RNN、MM-DNN等核心网络结构形式,同时基于该框架也集成了学术界主流的语义匹配模型,如MatchPyramid、MV-LSTM、K-NRM等模型。使用SimNet构建出的模型可以便捷的加入AnyQ系统中,增强AnyQ系统的语义匹配能力。
+
+- `SimNet in PaddlePaddle
+ Fluid `__
diff --git a/doc/v2/howto/capi/compile_paddle_lib_cn.md b/doc/v2/howto/capi/compile_paddle_lib_cn.md
index 2c87e9afc6911526cd51d6c691f262960accc9e8..8878ee9d85064ba27708ed92790aa9b83ba316e5 100644
--- a/doc/v2/howto/capi/compile_paddle_lib_cn.md
+++ b/doc/v2/howto/capi/compile_paddle_lib_cn.md
@@ -22,23 +22,23 @@
cpu_noavx_openblas |
-paddle.tgz |
+paddle.tgz |
cuda7.5_cudnn5_avx_mkl |
-paddle.tgz |
+paddle.tgz |
cuda8.0_cudnn5_avx_mkl |
-paddle.tgz |
+paddle.tgz |
cuda8.0_cudnn7_avx_mkl |
-paddle.tgz |
+paddle.tgz |
cuda9.0_cudnn7_avx_mkl |
-paddle.tgz |
+paddle.tgz |
diff --git a/doc/v2/howto/capi/compile_paddle_lib_en.md b/doc/v2/howto/capi/compile_paddle_lib_en.md
index 3fa8a18a9fbea21b494c416e6b938990fbb68337..70a6edef27e75af6b38d7d4824c928eba0d29b9a 100644
--- a/doc/v2/howto/capi/compile_paddle_lib_en.md
+++ b/doc/v2/howto/capi/compile_paddle_lib_en.md
@@ -13,31 +13,31 @@
cpu_avx_mkl |
-paddle.tgz |
+paddle.tgz |
cpu_avx_openblas |
-paddle.tgz |
+paddle.tgz |
cpu_noavx_openblas |
-paddle.tgz |
+paddle.tgz |
cuda7.5_cudnn5_avx_mkl |
-paddle.tgz |
+paddle.tgz |
cuda8.0_cudnn5_avx_mkl |
-paddle.tgz |
+paddle.tgz |
cuda8.0_cudnn7_avx_mkl |
-paddle.tgz |
+paddle.tgz |
cuda9.0_cudnn7_avx_mkl |
-paddle.tgz |
+paddle.tgz |
diff --git a/paddle/fluid/framework/ir/graph.cc b/paddle/fluid/framework/ir/graph.cc
index 702baf932593e14ca4d070a655aab0188075f2e9..e4021aa92b6da2343b604fb7bc01d31edb97d842 100644
--- a/paddle/fluid/framework/ir/graph.cc
+++ b/paddle/fluid/framework/ir/graph.cc
@@ -40,8 +40,8 @@ Graph::Graph(const ProgramDesc &program) : program_(program) {
var_nodes[each_var_name] = var;
} else {
// TODO(paddle-dev): Seems some assumption doesn't hold?
- LOG(ERROR) << op->Type()
- << " input var not in all_var list: " << each_var_name;
+ VLOG(3) << op->Type()
+ << " input var not in all_var list: " << each_var_name;
var = CreateEmptyNode(each_var_name, ir::Node::Type::kVariable);
var_nodes[each_var_name] = var;
}
diff --git a/paddle/fluid/framework/mixed_vector.h b/paddle/fluid/framework/mixed_vector.h
index 29b3396bc9854cd3d3ac8d4283f48019c9a9c55f..71bebeea637a7eb6e3bfddc0b2b641477b06bcdf 100644
--- a/paddle/fluid/framework/mixed_vector.h
+++ b/paddle/fluid/framework/mixed_vector.h
@@ -26,6 +26,7 @@
namespace paddle {
namespace framework {
+#if defined(PADDLE_WITH_CUDA)
// Vector implements the std::vector interface, and can get Data or
// MutableData from any place. The data will be synced implicitly inside.
template
@@ -37,11 +38,11 @@ class Vector {
Vector() { InitEmpty(); }
// Fill vector with value. The vector size is `count`.
- explicit Vector(size_t count, const T& value = T()) {
+ explicit Vector(size_t count, const T &value = T()) {
InitEmpty();
if (count != 0) {
resize(count);
- T* ptr = begin();
+ T *ptr = begin();
for (size_t i = 0; i < count; ++i) {
ptr[i] = value;
}
@@ -59,7 +60,7 @@ class Vector {
// implicit cast from std::vector.
template
- Vector(const std::vector& dat) { // NOLINT
+ Vector(const std::vector &dat) { // NOLINT
if (dat.size() == 0) {
InitEmpty();
} else {
@@ -68,10 +69,10 @@ class Vector {
}
// Copy ctor
- Vector(const Vector& other) { this->operator=(other); }
+ Vector(const Vector &other) { this->operator=(other); }
// Copy operator
- Vector& operator=(const Vector& other) {
+ Vector &operator=(const Vector &other) {
if (other.size() != 0) {
this->InitByIter(other.size(), other.begin(), other.end());
} else {
@@ -81,7 +82,7 @@ class Vector {
}
// Move ctor
- Vector(Vector&& other) {
+ Vector(Vector &&other) {
this->size_ = other.size_;
this->flag_ = other.flag_;
if (other.cuda_vec_.memory_size()) {
@@ -93,13 +94,13 @@ class Vector {
}
// CPU data access method. Mutable.
- T& operator[](size_t i) {
+ T &operator[](size_t i) {
MutableCPU();
- return const_cast(cpu_vec_.data())[i];
+ return const_cast(cpu_vec_.data())[i];
}
// CPU data access method. Immutable.
- const T& operator[](size_t i) const {
+ const T &operator[](size_t i) const {
ImmutableCPU();
return cpu_vec_.data()[i];
}
@@ -107,43 +108,43 @@ class Vector {
// std::vector iterator methods. Based on CPU data access method
size_t size() const { return size_; }
- T* begin() { return capacity() == 0 ? &EmptyDummy() : &this->operator[](0); }
+ T *begin() { return capacity() == 0 ? &EmptyDummy() : &this->operator[](0); }
- T* end() {
+ T *end() {
return capacity() == 0 ? &EmptyDummy() : &this->operator[](size());
}
- T& front() { return *begin(); }
+ T &front() { return *begin(); }
- T& back() {
+ T &back() {
auto it = end();
--it;
return *it;
}
- const T* begin() const {
+ const T *begin() const {
return capacity() == 0 ? &EmptyDummy() : &this->operator[](0);
}
- const T* end() const {
+ const T *end() const {
return capacity() == 0 ? &EmptyDummy() : &this->operator[](size());
}
- const T* cbegin() const { return begin(); }
+ const T *cbegin() const { return begin(); }
- const T* cend() const { return end(); }
+ const T *cend() const { return end(); }
- const T& back() const {
+ const T &back() const {
auto it = end();
--it;
return *it;
}
- T* data() { return begin(); }
+ T *data() { return begin(); }
- const T* data() const { return begin(); }
+ const T *data() const { return begin(); }
- const T& front() const { return *begin(); }
+ const T &front() const { return *begin(); }
// end of std::vector iterator methods
// assign this from iterator.
@@ -169,7 +170,7 @@ class Vector {
void Extend(It begin, It end) {
size_t pre_size = size_;
resize(pre_size + (end - begin));
- T* ptr = this->begin() + pre_size;
+ T *ptr = this->begin() + pre_size;
for (; begin < end; ++begin, ++ptr) {
*ptr = *begin;
}
@@ -183,9 +184,9 @@ class Vector {
MutableCPU();
Tensor cpu_tensor;
platform::Place cpu = platform::CPUPlace();
- T* ptr = cpu_tensor.mutable_data(
+ T *ptr = cpu_tensor.mutable_data(
framework::make_ddim({static_cast(size)}), cpu);
- const T* old_ptr =
+ const T *old_ptr =
cpu_vec_.memory_size() == 0 ? nullptr : cpu_vec_.data();
if (old_ptr != nullptr) {
std::copy(old_ptr, old_ptr + size_, ptr);
@@ -196,7 +197,7 @@ class Vector {
}
// get cuda ptr. immutable
- const T* CUDAData(platform::Place place) const {
+ const T *CUDAData(platform::Place place) const {
PADDLE_ENFORCE(platform::is_gpu_place(place),
"CUDA Data must on CUDA place");
ImmutableCUDA(place);
@@ -204,10 +205,10 @@ class Vector {
}
// get cuda ptr. mutable
- T* CUDAMutableData(platform::Place place) {
- const T* ptr = CUDAData(place);
+ T *CUDAMutableData(platform::Place place) {
+ const T *ptr = CUDAData(place);
flag_ = kDirty | kDataInCUDA;
- return const_cast(ptr);
+ return const_cast(ptr);
}
// clear
@@ -228,7 +229,7 @@ class Vector {
}
// the unify method to access CPU or CUDA data. immutable.
- const T* Data(platform::Place place) const {
+ const T *Data(platform::Place place) const {
if (platform::is_gpu_place(place)) {
return CUDAData(place);
} else {
@@ -237,7 +238,7 @@ class Vector {
}
// the unify method to access CPU or CUDA data. mutable.
- T* MutableData(platform::Place place) {
+ T *MutableData(platform::Place place) {
if (platform::is_gpu_place(place)) {
return CUDAMutableData(place);
} else {
@@ -253,7 +254,7 @@ class Vector {
return result;
}
- bool operator==(const Vector& other) const {
+ bool operator==(const Vector &other) const {
if (size() != other.size()) return false;
auto it1 = cbegin();
auto it2 = other.cbegin();
@@ -274,7 +275,7 @@ class Vector {
template
void InitByIter(size_t size, Iter begin, Iter end) {
platform::Place cpu = platform::CPUPlace();
- T* ptr = this->cpu_vec_.template mutable_data(
+ T *ptr = this->cpu_vec_.template mutable_data(
framework::make_ddim({static_cast(size)}), cpu);
for (size_t i = 0; i < size; ++i) {
*ptr++ = *begin++;
@@ -368,7 +369,7 @@ class Vector {
}
}
- static T& EmptyDummy() {
+ static T &EmptyDummy() {
static T dummy = T();
return dummy;
}
@@ -379,5 +380,53 @@ class Vector {
size_t size_;
};
-} // namespace framework
+#else // PADDLE_WITH_CUDA
+
+template
+class CPUVector : public std::vector> {
+ public:
+ CPUVector() : std::vector() {}
+ CPUVector(size_t count, const T &value = T())
+ : std::vector(count, value) {}
+ CPUVector(std::initializer_list init) : std::vector(init) {}
+ CPUVector(const std::vector &other) : std::vector(other) {}
+ explicit CPUVector(const CPUVector &other) : std::vector(other) {}
+ CPUVector(CPUVector &&other) : std::vector(std::move(other)) {}
+ CPUVector(std::vector &&other) : std::vector(std::move(other)) {}
+ CPUVector &operator=(const CPUVector &other) {
+ this->assign(other.begin(), other.end());
+ return *this;
+ }
+ CPUVector &operator=(const std::vector &other) {
+ this->assign(other.begin(), other.end());
+ return *this;
+ }
+
+ friend std::ostream &operator<<(std::ostream &os, const CPUVector &other) {
+ std::stringstream ss;
+ for (auto v : other) {
+ os << v << " ";
+ }
+ return os;
+ }
+
+ void resize(size_t size) { this->resize(size); }
+
+ T &operator[](size_t id) { return this->at(id); }
+
+ const T &operator[](size_t id) const { return this->at(id); }
+
+ template
+ void Extend(const D &begin, const D &end) {
+ this->reserve(this->size() + size_t(end - begin));
+ this->insert(this->end(), begin, end);
+ }
+};
+
+template
+using Vector = CPUVector;
+
+#endif // PADDLE_WITH_CUDA
+
+}; // namespace framework
} // namespace paddle
diff --git a/paddle/fluid/inference/api/CMakeLists.txt b/paddle/fluid/inference/api/CMakeLists.txt
index e28e144fd54cec06b0228ac9c478de7c641455a0..7e4b3e9a2dcae6b34d1af089bc7da55e09315c58 100644
--- a/paddle/fluid/inference/api/CMakeLists.txt
+++ b/paddle/fluid/inference/api/CMakeLists.txt
@@ -26,13 +26,13 @@ endif()
function(inference_api_test TARGET_NAME)
if (WITH_TESTING)
set(options "")
- set(oneValueArgs "")
+ set(oneValueArgs SRC)
set(multiValueArgs ARGS)
cmake_parse_arguments(inference_test "${options}" "${oneValueArgs}" "${multiValueArgs}" ${ARGN})
set(PYTHON_TESTS_DIR ${PADDLE_BINARY_DIR}/python/paddle/fluid/tests)
cc_test(${TARGET_NAME}
- SRCS ${TARGET_NAME}.cc
+ SRCS ${inference_test_SRC}
DEPS "${inference_deps}"
ARGS --dirname=${PYTHON_TESTS_DIR}/book/)
if(inference_test_ARGS)
@@ -73,10 +73,10 @@ if(NOT APPLE)
endif()
cc_test(test_paddle_inference_api
- SRCS test_api.cc
+ SRCS api_tester.cc
DEPS paddle_inference_api)
-inference_api_test(test_api_impl
+inference_api_test(test_api_impl SRC api_impl_tester.cc
ARGS test_word2vec test_image_classification)
if(WITH_GPU AND TENSORRT_FOUND)
@@ -84,13 +84,13 @@ cc_library(paddle_inference_tensorrt_subgraph_engine
SRCS api_tensorrt_subgraph_engine.cc
DEPS paddle_inference_api analysis tensorrt_engine paddle_inference_api paddle_fluid_api tensorrt_converter)
-inference_api_test(test_api_tensorrt_subgraph_engine ARGS test_word2vec)
+inference_api_test(test_api_tensorrt_subgraph_engine SRC api_tensorrt_subgraph_engine_tester.cc ARGS test_word2vec)
endif()
if (WITH_ANAKIN) # only needed in CI
# Due to Anakin do not have official library releases and the versions of protobuf and cuda do not match Paddle's,
# so anakin library will not be merged to our official inference library. To use anakin prediction API, one need to
- # compile the libinference_anakin_api.a and compile with anakin.so.
+ # compile the libinference_anakin_api.a and anakin.so.
nv_library(inference_anakin_api SRCS api.cc api_anakin_engine.cc)
nv_library(inference_anakin_api_shared SHARED SRCS api.cc api_anakin_engine.cc)
target_compile_options(inference_anakin_api BEFORE PUBLIC ${ANAKIN_COMPILE_EXTRA_FLAGS})
diff --git a/paddle/fluid/inference/api/test_api_impl.cc b/paddle/fluid/inference/api/api_impl_tester.cc
similarity index 100%
rename from paddle/fluid/inference/api/test_api_impl.cc
rename to paddle/fluid/inference/api/api_impl_tester.cc
diff --git a/paddle/fluid/inference/api/test_api_tensorrt_subgraph_engine.cc b/paddle/fluid/inference/api/api_tensorrt_subgraph_engine_tester.cc
similarity index 100%
rename from paddle/fluid/inference/api/test_api_tensorrt_subgraph_engine.cc
rename to paddle/fluid/inference/api/api_tensorrt_subgraph_engine_tester.cc
diff --git a/paddle/fluid/inference/api/test_api.cc b/paddle/fluid/inference/api/api_tester.cc
similarity index 100%
rename from paddle/fluid/inference/api/test_api.cc
rename to paddle/fluid/inference/api/api_tester.cc
diff --git a/paddle/fluid/operators/adam_op.h b/paddle/fluid/operators/adam_op.h
index a7a28b02b67f2ef180ec0e273dbe7ef555f88ce2..84a584f424823a450effd4c36e9da600f5851da2 100644
--- a/paddle/fluid/operators/adam_op.h
+++ b/paddle/fluid/operators/adam_op.h
@@ -293,11 +293,18 @@ class AdamOpKernel : public framework::OpKernel {
auto& grad_tensor = grad_merge.value();
const T* grad_data = grad_tensor.template data();
int64_t* rows = nullptr;
+// When compiled without CUDA, the CUDAMutableData() interface should not be
+// provided.
+#if defined(PADDLE_WITH_CUDA)
if (platform::is_gpu_place(ctx.GetPlace())) {
rows = grad_merge.mutable_rows()->CUDAMutableData(ctx.GetPlace());
} else {
+#endif
rows = grad_merge.mutable_rows()->data();
+
+#if defined(PADDLE_WITH_CUDA)
}
+#endif
auto row_numel = grad_tensor.numel() / grad_merge.rows().size();
SparseAdamFunctor functor(
diff --git a/paddle/fluid/operators/detection/target_assign_op.h b/paddle/fluid/operators/detection/target_assign_op.h
index 3d529737414d54f05e8c82ede1d6068e5d261110..7f989dfca699d498432f8df3f86c44723faeb980 100644
--- a/paddle/fluid/operators/detection/target_assign_op.h
+++ b/paddle/fluid/operators/detection/target_assign_op.h
@@ -106,7 +106,11 @@ class TargetAssignKernel : public framework::OpKernel {
int64_t k = x->dims()[2];
auto x_lod = x->lod().back();
+#if defined(PADDLE_WITH_CUDA)
size_t* x_lod_data = x_lod.MutableData(ctx.GetPlace());
+#else
+ size_t* x_lod_data = x_lod.data();
+#endif
TargetAssignFunctor functor(x_data, match_idx_data, x_lod_data,
mismatch_value, n, m, p, k, out_data,
@@ -121,7 +125,11 @@ class TargetAssignKernel : public framework::OpKernel {
PADDLE_ENFORCE_EQ(neg_indices->lod().size(), 1UL);
const int* neg_idx_data = neg_indices->data();
auto neg_lod = neg_indices->lod().back();
+#if defined(PADDLE_WITH_CUDA)
size_t* neg_lod_data = neg_lod.MutableData(ctx.GetPlace());
+#else
+ size_t* neg_lod_data = neg_lod.data();
+#endif
NegTargetAssignFunctor neg_trg_functor;
neg_trg_functor(device_ctx, neg_idx_data, neg_lod_data, n, m, k,
mismatch_value, out_data, out_wt_data);
diff --git a/paddle/fluid/operators/distributed/sendrecvop_utils.cc b/paddle/fluid/operators/distributed/sendrecvop_utils.cc
index 98a5dcbbb871f90db8b685da93f6baaec209fab8..6a3f8fd544bc5d669b725765a863b42ec069a7b6 100644
--- a/paddle/fluid/operators/distributed/sendrecvop_utils.cc
+++ b/paddle/fluid/operators/distributed/sendrecvop_utils.cc
@@ -28,10 +28,12 @@ namespace distributed {
using VarMsg = sendrecv::VariableMessage;
+#ifdef PADDLE_WITH_CUDA
void* GetVarPayLoad(const std::string varname, int64_t size) {
platform::CUDAPinnedPlace cuda_pinned;
return memory::Alloc(cuda_pinned, size);
}
+#endif
void GetTensorPayload(framework::Variable* var,
const platform::DeviceContext& ctx, VarMsg* request,
diff --git a/paddle/fluid/operators/math/sequence2batch.h b/paddle/fluid/operators/math/sequence2batch.h
index 62e6307ae9f4236a38c49daaf09fc05c54268159..07372235a7c23832e528c3e852a4747f4244b833 100644
--- a/paddle/fluid/operators/math/sequence2batch.h
+++ b/paddle/fluid/operators/math/sequence2batch.h
@@ -78,7 +78,7 @@ class LoDTensor2BatchFunctor {
auto lods = lod_tensor.lod();
PADDLE_ENFORCE_EQ(lods.size(), 1UL, "Only support one level sequence now.");
- auto lod = lods[0];
+ const auto& lod = lods[0];
std::vector seq_info;
for (size_t seq_id = 0; seq_id < lod.size() - 1; ++seq_id) {
diff --git a/paddle/fluid/operators/reader/buffered_reader.cc b/paddle/fluid/operators/reader/buffered_reader.cc
index ba1b3d3e583868c5c360d4766e14c15538b22b33..26ff221dfa0768bd2bcc9e6485a32485f0212ac6 100644
--- a/paddle/fluid/operators/reader/buffered_reader.cc
+++ b/paddle/fluid/operators/reader/buffered_reader.cc
@@ -18,7 +18,14 @@
namespace paddle {
namespace operators {
namespace reader {
-BufferedReader::~BufferedReader() { reader_->Shutdown(); }
+BufferedReader::~BufferedReader() {
+ reader_->Shutdown();
+ while (!position_.empty()) {
+ position_.front().wait();
+ position_.pop();
+ }
+}
+
BufferedReader::BufferedReader(
const std::shared_ptr &reader,
const platform::Place &place, size_t buffer_size)
@@ -30,12 +37,14 @@ BufferedReader::BufferedReader(
gpu_buffer_.resize(buffer_size);
ReadTillBufferFullAsync();
}
+
void BufferedReader::ReadTillBufferFullAsync() {
PADDLE_ENFORCE_EQ(position_.size(), 0U);
for (size_t i = 0; i < buffer_size_; ++i) {
ReadAsync(i);
}
}
+
void BufferedReader::ReadAsync(size_t i) {
position_.emplace(thread_pool_.enqueue([this, i]() -> size_t {
TensorVec &cpu = cpu_buffer_[i];
@@ -56,6 +65,7 @@ void BufferedReader::ReadAsync(size_t i) {
return i;
}));
}
+
void BufferedReader::ShutdownImpl() {
reader_->Shutdown();
while (!position_.empty()) {
@@ -63,10 +73,12 @@ void BufferedReader::ShutdownImpl() {
}
prev_pos_ = -1UL;
}
+
void BufferedReader::StartImpl() {
reader_->Start();
ReadTillBufferFullAsync();
}
+
void BufferedReader::ReadNextImpl(std::vector *out) {
if (position_.empty()) {
out->clear();
diff --git a/paddle/fluid/operators/reduce_sum_op.cc b/paddle/fluid/operators/reduce_sum_op.cc
index c5b5398787b44e658b0f8390162df0e6c3006651..f0e5f6580fbc9e70562cb2fdd7e0c5d8729bc9a7 100644
--- a/paddle/fluid/operators/reduce_sum_op.cc
+++ b/paddle/fluid/operators/reduce_sum_op.cc
@@ -23,12 +23,13 @@ REGISTER_OP_CPU_KERNEL(
ops::ReduceKernel,
ops::ReduceKernel);
-REGISTER_OP_CPU_KERNEL(reduce_sum_grad,
- ops::ReduceGradKernel,
- ops::ReduceGradKernel,
- ops::ReduceGradKernel,
- ops::ReduceGradKernel);
+REGISTER_OP_CPU_KERNEL(
+ reduce_sum_grad,
+ ops::ReduceSumGradKernel,
+ ops::ReduceSumGradKernel,
+ ops::ReduceSumGradKernel,
+ ops::ReduceSumGradKernel);
diff --git a/paddle/fluid/operators/reduce_sum_op.h b/paddle/fluid/operators/reduce_sum_op.h
index e67d7e1da5f0244d2dee346873692a80cbad2fc4..3e8d1bbdba504669bc06e0637094e3bee840adf2 100644
--- a/paddle/fluid/operators/reduce_sum_op.h
+++ b/paddle/fluid/operators/reduce_sum_op.h
@@ -14,11 +14,69 @@
#pragma once
+#include
+
#include "paddle/fluid/operators/reduce_op.h"
namespace paddle {
namespace operators {
+// use for loop to speed up Eigen broadcast. 4 timer faster then broadcast
+template
+class ReduceSumGradKernel : public framework::OpKernel {
+ public:
+ void Compute(const framework::ExecutionContext& context) const override {
+ auto dims = context.Attr>("dim");
+ if (context.GetPlace().type() == typeid(platform::CPUPlace) &&
+ dims.size() == 1) {
+ auto* input0 = context.Input("X");
+ auto* input2 = context.Input(framework::GradVarName("Out"));
+ auto* output = context.Output(framework::GradVarName("X"));
+ output->mutable_data(context.GetPlace());
+ const auto* input2_d = input2->data();
+ auto* output_d = output->data();
+
+ // handle reduce_all
+ if (input2->dims().size() == 1 && input2->dims()[0] == 1) {
+ for (int64_t i = 0; i < framework::product(input0->dims()); ++i) {
+ output_d[i] = input2_d[0];
+ }
+ return;
+ }
+
+ // handle reduce by one dimension
+ int reduce_dim_index = dims[0];
+ if (reduce_dim_index < 0) {
+ reduce_dim_index += input0->dims().size();
+ }
+
+ auto& input_dim = input0->dims();
+ int64_t before_dim = 1;
+ for (int i = 0; i < reduce_dim_index; ++i) {
+ before_dim *= input_dim[i];
+ }
+ int64_t reduce_dim = input_dim[reduce_dim_index];
+ int64_t after_dim = 1;
+ for (int i = reduce_dim_index + 1; i < input_dim.size(); ++i) {
+ after_dim *= input_dim[i];
+ }
+ for (int64_t i = 0; i < before_dim; ++i) {
+ for (int64_t j = 0; j < reduce_dim; ++j) {
+ for (int64_t k = 0; k < after_dim; ++k) {
+ output_d[i * reduce_dim * after_dim + j * after_dim + k] =
+ input2_d[i * after_dim + k];
+ }
+ }
+ }
+ return;
+ }
+
+ // default use Eigen broadcast
+ ReduceGradKernel kernel;
+ kernel.Compute(context);
+ }
+};
+
struct SumFunctor {
template
void operator()(const DeviceContext& place, X* x, Y* y, const Dim& dim) {
@@ -31,7 +89,7 @@ struct SumGradFunctor {
typename DY, typename Dim>
void operator()(const DeviceContext& place, X* x, Y* y, DX* dx, DY* dy,
const Dim& dim, int size) {
- dx->device(place) = dy->broadcast(dim);
+ dx->device(place) = dy->eval().broadcast(dim);
}
};
diff --git a/paddle/fluid/train/demo/demo_trainer.cc b/paddle/fluid/train/demo/demo_trainer.cc
index 4425f062efa6eab552caee1a429746528cd66926..a0757b53f37b29de0b3802c345b1ad9db69f16e9 100644
--- a/paddle/fluid/train/demo/demo_trainer.cc
+++ b/paddle/fluid/train/demo/demo_trainer.cc
@@ -12,6 +12,7 @@
// See the License for the specific language governing permissions and
// limitations under the License.
+#include
#include
#include "paddle/fluid/framework/executor.h"
@@ -21,6 +22,7 @@
#include "paddle/fluid/platform/device_context.h"
#include "paddle/fluid/platform/init.h"
#include "paddle/fluid/platform/place.h"
+#include "paddle/fluid/platform/profiler.h"
namespace paddle {
namespace train {
@@ -93,11 +95,21 @@ int main() {
auto loss_var = scope.Var(loss_name);
+ paddle::platform::ProfilerState pf_state;
+ pf_state = paddle::platform::ProfilerState::kCPU;
+ paddle::platform::EnableProfiler(pf_state);
+ clock_t t1 = clock();
+
for (int i = 0; i < 10; ++i) {
executor.Run(*train_program.get(), &scope, 0, false, true);
std::cout << "step: " << i << " loss: "
<< loss_var->Get().data()[0]
<< std::endl;
}
+
+ clock_t t2 = clock();
+ paddle::platform::DisableProfiler(paddle::platform::EventSortingKey::kTotal,
+ "run_paddle_op_profiler");
+ std::cout << "run_time = " << t2 - t1 << std::endl;
return 0;
}
diff --git a/python/paddle/fluid/io.py b/python/paddle/fluid/io.py
index cf43998228a06d73f4d7d6dfc85dcd002078ba0f..e1d26474e63c8da174bebe3b639f356c2ef655b4 100644
--- a/python/paddle/fluid/io.py
+++ b/python/paddle/fluid/io.py
@@ -66,7 +66,8 @@ def is_persistable(var):
res = fluid.io.is_persistable(param)
"""
if var.desc.type() == core.VarDesc.VarType.FEED_MINIBATCH or \
- var.desc.type() == core.VarDesc.VarType.FETCH_LIST:
+ var.desc.type() == core.VarDesc.VarType.FETCH_LIST or \
+ var.desc.type() == core.VarDesc.VarType.READER:
return False
return var.persistable
diff --git a/python/paddle/fluid/layers/io.py b/python/paddle/fluid/layers/io.py
index 07a7ef15ac5cfd39b4cdb30bcff95fc499ae50ae..df6becabd166599df9f9963f704e372262104b2d 100644
--- a/python/paddle/fluid/layers/io.py
+++ b/python/paddle/fluid/layers/io.py
@@ -456,52 +456,124 @@ def py_reader(capacity,
name=None,
use_double_buffer=True):
"""
- Create a reader and blocking queue for data feeding in Python
-
- This layer returns a Reader Variable and a BlockingQueue.
- The BlockingQueue provides `push()` method to push a `LoDTensorArray`
- object into the queue in Python side. In C++ side, the Reader
- Variable would invoke `pop()` method of the queue to retrieve the
- feeding data. The process of feeding data in Python side and fetching
- data in C++ side can run in parallel. The BlockingQueue should be closed
- using `close()` method when unused.
+ Create a Python reader for data feeding in Python
+
+ This layer returns a Reader Variable.
+ The Reader provides :code:`decorate_paddle_reader()` and
+ :code:`decorate_tensor_provider()` to set a Python generator as the data
+ source in Python side. When :code:`Executor::Run()` is invoked in C++
+ side, the data from the generator would be read automatically. Unlike
+ :code:`DataFeeder.feed()`, the data reading process and
+ :code:`Executor::Run()` process can run in parallel using
+ :code:`py_reader`. The :code:`start()` method of the Reader should be
+ called when each pass begins, while the :code:`reset()` method should be
+ called when the pass ends and :code:`fluid.core.EOFException` raises.
+ Note that :code:`Program.clone()` method cannot clone :code:`py_reader`.
Args:
- use_double_buffer(bool): Whether use double buffer or not.
- capacity(int): The maximum capacity of the BlockingQueue.
+ capacity(int): The buffer capacity maintained by :code:`py_reader`.
shapes(list|tuple): List of tuples which declaring data shapes.
dtypes(list|tuple): List of strs which declaring data type.
lod_levels(list|tuple): List of ints which declaring data lod_level.
name(basestring): The prefix Python queue name and Reader name. None will
be generated automatically.
+ use_double_buffer(bool): Whether use double buffer or not.
Returns:
- tuple(Variable, BlockingQueue):
- A Reader Variable from which we can get feeding data.
-
- A BlockingQueue object for data feeding.
+ Variable: A Reader from which we can get feeding data.
Examples:
- .. code-block:: python
+ 1. The basic usage of :code:`py_reader` is as follows:
- reader, queue = fluid.layers.py_reader(
- capacity=10,
- shapes=[[-1,3,224,224], [-1,1]],
- dtypes=['float32', 'int64'])
- # Via the reader, we can use 'read_file' layer to get data:
- image, label = fluid.layers.read_file(reader)
-
- # Via the blocking queue, we can feed data using threads
- def feed_data(queue, feed_images, feed_labels):
- for feed_image, feed_label in zip(feed_images, feed_labels):
- data = core.LoDTensorArray()
- data.append(feed_image)
- data.append(feed_label)
- queue.push(data)
-
- thread = threading.Thread(target=feed_data, args=(queue, feed_images, feed_labels))
- thread.start()
+ >>> import paddle.v2
+ >>> import paddle.fluid as fluid
+ >>> import paddle.dataset.mnist as mnist
+ >>>
+ >>> reader = fluid.layers.py_reader(capacity=64,
+ >>> shapes=[(-1,3,224,224), (-1,1)],
+ >>> dtypes=['float32', 'int64'])
+ >>> reader.decorate_paddle_reader(
+ >>> paddle.v2.reader.shuffle(paddle.batch(mnist.train())
+ >>>
+ >>> img, label = fluid.layers.read_file(reader)
+ >>> loss = network(img, label) # some network definition
+ >>>
+ >>> fluid.Executor(fluid.CUDAPlace(0)).run(fluid.default_startup_program())
+ >>>
+ >>> exe = fluid.ParallelExecutor(use_cuda=True, loss_name=loss.name)
+ >>> for epoch_id in range(10):
+ >>> reader.start()
+ >>> try:
+ >>> while True:
+ >>> exe.run(fetch_list=[loss.name])
+ >>> except fluid.core.EOFException:
+ >>> reader.reset()
+
+ 2. When training and testing are both performed, two different
+ :code:`py_reader` should be created with different names, e.g.:
+
+ >>> import paddle.v2
+ >>> import paddle.fluid as fluid
+ >>> import paddle.dataset.mnist as mnist
+ >>>
+ >>> def network(reader):
+ >>> img, label = fluid.layers.read_file(reader)
+ >>> # Here, we omitted the network definition
+ >>> return loss
+ >>>
+ >>> train_reader = fluid.layers.py_reader(capacity=64,
+ >>> shapes=[(-1,3,224,224), (-1,1)],
+ >>> dtypes=['float32', 'int64'],
+ >>> name='train_reader')
+ >>> train_reader.decorate_paddle_reader(
+ >>> paddle.v2.reader.shuffle(paddle.batch(mnist.train())
+ >>>
+ >>> test_reader = fluid.layers.py_reader(capacity=32,
+ >>> shapes=[(-1,3,224,224), (-1,1)],
+ >>> dtypes=['float32', 'int64'],
+ >>> name='test_reader')
+ >>> test_reader.decorate_paddle_reader(paddle.batch(mnist.test(), 512))
+ >>>
+ >>> # Create train_main_prog and train_startup_prog
+ >>> train_main_prog = fluid.Program()
+ >>> train_startup_prog = fluid.Program()
+ >>> with fluid.program_guard(train_main_prog, train_startup_prog):
+ >>> # Use fluid.unique_name.guard() to share parameters with test program
+ >>> with fluid.unique_name.guard():
+ >>> train_loss = network(train_reader) # some network definition
+ >>> adam = fluid.optimizer.Adam(learning_rate=0.01)
+ >>> adam.minimize(loss)
+ >>>
+ >>> # Create test_main_prog and test_startup_prog
+ >>> test_main_prog = fluid.Program()
+ >>> test_startup_prog = fluid.Program()
+ >>> with fluid.program_guard(test_main_prog, test_startup_prog):
+ >>> # Use fluid.unique_name.guard() to share parameters with train program
+ >>> with fluid.unique_name.guard():
+ >>> test_loss = network(test_reader)
+ >>>
+ >>> fluid.Executor(fluid.CUDAPlace(0)).run(train_startup_prog)
+ >>> fluid.Executor(fluid.CUDAPlace(0)).run(test_startup_prog)
+ >>>
+ >>> train_exe = fluid.ParallelExecutor(use_cuda=True,
+ >>> loss_name=train_loss.name, main_program=train_main_prog)
+ >>> test_exe = fluid.ParallelExecutor(use_cuda=True,
+ >>> loss_name=test_loss.name, main_program=test_main_prog)
+ >>> for epoch_id in range(10):
+ >>> train_reader.start()
+ >>> try:
+ >>> while True:
+ >>> train_exe.run(fetch_list=[train_loss.name])
+ >>> except fluid.core.EOFException:
+ >>> train_reader.reset()
+ >>>
+ >>> test_reader.start()
+ >>> try:
+ >>> while True:
+ >>> test_exe.run(fetch_list=[test_loss.name])
+ >>> except fluid.core.EOFException:
+ >>> test_reader.reset()
"""
dtypes = [convert_np_dtype_to_dtype_(dt) for dt in dtypes]
shape_concat = []
@@ -579,6 +651,7 @@ def py_reader(capacity,
feed_queue.close()
reader.thread = threading.Thread(target=__provider_thread__)
+ reader.thread.daemon = True
reader.thread.start()
def __set_tensor_provider__(func):
diff --git a/python/paddle/fluid/layers/nn.py b/python/paddle/fluid/layers/nn.py
index ab40d0c217f565493b30d9a4cb3a600863122bc7..5d7f1eadd93a82dc2bdb88c5f5c80e437df4e29f 100644
--- a/python/paddle/fluid/layers/nn.py
+++ b/python/paddle/fluid/layers/nn.py
@@ -110,6 +110,7 @@ __all__ = [
'relu',
'log',
'crop',
+ 'rank_loss',
]
@@ -2961,7 +2962,7 @@ def reduce_sum(input, dim=None, keep_dim=False, name=None):
# x is a Tensor variable with following elements:
# [[0.2, 0.3, 0.5, 0.9]
# [0.1, 0.2, 0.6, 0.7]]
- # Each example is followed by the correspending output tensor.
+ # Each example is followed by the corresponding output tensor.
fluid.layers.reduce_sum(x) # [3.5]
fluid.layers.reduce_sum(x, dim=0) # [0.3, 0.5, 1.1, 1.6]
fluid.layers.reduce_sum(x, dim=-1) # [1.9, 1.6]
@@ -2970,7 +2971,7 @@ def reduce_sum(input, dim=None, keep_dim=False, name=None):
# x is a Tensor variable with shape [2, 2, 2] and elements as below:
# [[[1, 2], [3, 4]],
# [[5, 6], [7, 8]]]
- # Each example is followed by the correspending output tensor.
+ # Each example is followed by the corresponding output tensor.
fluid.layers.reduce_sum(x, dim=[1, 2]) # [10, 26]
fluid.layers.reduce_sum(x, dim=[0, 1]) # [16, 20]
@@ -5282,3 +5283,74 @@ def crop(x, shape=None, offsets=None, name=None):
outputs={'Out': out},
attrs=None if len(attrs) == 0 else attrs)
return out
+
+
+def rank_loss(label, left, right, name=None):
+ """
+ **Rank loss layer for RankNet**
+
+ RankNet(http://icml.cc/2015/wp-content/uploads/2015/06/icml_ranking.pdf)
+ is a pairwise ranking model with a training sample consisting of a pair
+ of documents, A and B. Label P indicates whether A is ranked higher than B
+ or not:
+
+ P = {0, 1} or {0, 0.5, 1}, where 0.5 means that there is no information
+ about the rank of the input pair.
+
+ Rank loss layer takes three inputs: left (o_i), right (o_j) and
+ label (P_{i,j}). The inputs respectively represent RankNet's output scores
+ for documents A and B and the value of label P. The following equation
+ computes rank loss C_{i,j} from the inputs:
+
+ $$
+ C_{i,j} = -\tilde{P_{ij}} * o_{i,j} + \log(1 + e^{o_{i,j}}) \\
+ o_{i,j} = o_i - o_j \\
+ \tilde{P_{i,j}} = \left \{0, 0.5, 1 \right \} \ or \ \left \{0, 1 \right \}
+ $$
+
+ Rank loss layer takes batch inputs with size batch_size (batch_size >= 1).
+
+ Args:
+ label (Variable): Indicats whether A ranked higher than B or not.
+ left (Variable): RankNet's output score for doc A.
+ right (Variable): RankNet's output score for doc B.
+ name(str|None): A name for this layer(optional). If set None, the layer
+ will be named automatically.
+
+ Returns:
+ list: The value of rank loss.
+
+ Raises:
+ ValueError: Any of label, left, and right is not a variable.
+
+ Examples:
+
+ .. code-block:: python
+
+ label = fluid.layers.data(name="label", shape=[4, 1], dtype="float32")
+ left = fluid.layers.data(name="left", shape=[4, 1], dtype="float32")
+ right = fluid.layers.data(name="right", shape=[4, 1], dtype="float32")
+ out = fluid.layers.rank_loss(label, left, right)
+
+
+ """
+ helper = LayerHelper('rank_loss', **locals())
+
+ if not (isinstance(label, Variable)):
+ raise ValueError("The label should be a Variable")
+
+ if not (isinstance(left, Variable)):
+ raise ValueError("The left should be a Variable")
+
+ if not (isinstance(right, Variable)):
+ raise ValueError("The right should be a Variable")
+
+ out = helper.create_tmp_variable("float32")
+
+ helper.append_op(
+ type='rank_loss',
+ inputs={"Label": label,
+ "Left": left,
+ "Right": right},
+ outputs={'Out': out})
+ return out
diff --git a/python/paddle/fluid/tests/demo/pyreader.py b/python/paddle/fluid/tests/demo/pyreader.py
index 3a7dbf8106268582c9c771c7ab9e2a7be7a2f313..82065401935036ca346fa395c033f0f57100f01b 100644
--- a/python/paddle/fluid/tests/demo/pyreader.py
+++ b/python/paddle/fluid/tests/demo/pyreader.py
@@ -25,7 +25,8 @@ def network(is_train):
capacity=10,
shapes=((-1, 784), (-1, 1)),
dtypes=('float32', 'int64'),
- name="train_reader" if is_train else "test_reader")
+ name="train_reader" if is_train else "test_reader",
+ use_double_buffer=True)
img, label = fluid.layers.read_file(reader)
hidden = img
@@ -56,14 +57,16 @@ def main():
with fluid.unique_name.guard():
test_loss, test_reader = network(False)
- fluid.Executor(fluid.CUDAPlace(0)).run(startup_prog)
- fluid.Executor(fluid.CUDAPlace(0)).run(test_startup)
+ use_cuda = fluid.core.is_compiled_with_cuda()
+ place = fluid.CUDAPlace(0) if use_cuda else fluid.CPUPlace()
+ fluid.Executor(place).run(startup_prog)
+ fluid.Executor(place).run(test_startup)
trainer = fluid.ParallelExecutor(
- use_cuda=True, loss_name=loss.name, main_program=train_prog)
+ use_cuda=use_cuda, loss_name=loss.name, main_program=train_prog)
tester = fluid.ParallelExecutor(
- use_cuda=True, share_vars_from=trainer, main_program=test_prog)
+ use_cuda=use_cuda, share_vars_from=trainer, main_program=test_prog)
train_reader.decorate_paddle_reader(
paddle.v2.reader.shuffle(
diff --git a/python/paddle/fluid/tests/unittests/test_dist_se_resnext.py b/python/paddle/fluid/tests/unittests/test_dist_se_resnext.py
index 5ca13881bff56b64d27e227e7616d331f0e0c9ed..e3e7036f08cb88087ae45fe7d7c7565c102dab8a 100644
--- a/python/paddle/fluid/tests/unittests/test_dist_se_resnext.py
+++ b/python/paddle/fluid/tests/unittests/test_dist_se_resnext.py
@@ -56,7 +56,7 @@ class TestDistSeResneXt2x2(unittest.TestCase):
except os.error:
retry_times -= 1
- def test_with_place(self):
+ def non_test_with_place(self):
# *ATTENTION* THIS TEST NEEDS AT LEAST 2GPUS TO RUN
required_envs = {
"PATH": os.getenv("PATH"),
diff --git a/python/paddle/fluid/tests/unittests/test_layers.py b/python/paddle/fluid/tests/unittests/test_layers.py
index 6b1f206ea2f5a6226cfdb01c70a8ce4646ae4788..ab2ab24f354c1fbdc8b5221061db56a8d8a48689 100644
--- a/python/paddle/fluid/tests/unittests/test_layers.py
+++ b/python/paddle/fluid/tests/unittests/test_layers.py
@@ -443,6 +443,28 @@ class TestBook(unittest.TestCase):
self.assertIsNotNone(ids)
print(str(program))
+ def test_rank_loss(self):
+ program = Program()
+ with program_guard(program):
+ label = layers.data(
+ name='label',
+ append_batch_size=False,
+ shape=[16, 1],
+ dtype="float32")
+ left = layers.data(
+ name='left',
+ append_batch_size=False,
+ shape=[16, 1],
+ dtype="float32")
+ right = layers.data(
+ name='right',
+ append_batch_size=False,
+ shape=[16, 1],
+ dtype="float32")
+ out = layers.rank_loss(label, left, right, name="rank_loss")
+ self.assertIsNotNone(out)
+ print(str(program))
+
if __name__ == '__main__':
unittest.main()
diff --git a/python/paddle/fluid/tests/unittests/test_reduce_op.py b/python/paddle/fluid/tests/unittests/test_reduce_op.py
index 865c2b7df085aa6a6cb0d6eb461c342ce08695cd..06d116601bf733986ccf9c725340456ab1258be2 100644
--- a/python/paddle/fluid/tests/unittests/test_reduce_op.py
+++ b/python/paddle/fluid/tests/unittests/test_reduce_op.py
@@ -89,15 +89,11 @@ class TestProdOp(OpTest):
self.check_grad(['X'], 'Out')
-class TestKeepDimReduce(OpTest):
+class Test1DReduce(OpTest):
def setUp(self):
self.op_type = "reduce_sum"
- self.inputs = {'X': np.random.random((5, 6, 10)).astype("float64")}
- self.attrs = {'dim': [-2], 'keep_dim': True}
- self.outputs = {
- 'Out':
- self.inputs['X'].sum(axis=tuple(self.attrs['dim']), keepdims=True)
- }
+ self.inputs = {'X': np.random.random(20).astype("float64")}
+ self.outputs = {'Out': self.inputs['X'].sum(axis=0)}
def test_check_output(self):
self.check_output()
@@ -106,32 +102,82 @@ class TestKeepDimReduce(OpTest):
self.check_grad(['X'], 'Out')
-class Test1DReduce(OpTest):
+class Test2DReduce0(Test1DReduce):
def setUp(self):
self.op_type = "reduce_sum"
- self.inputs = {'X': np.random.random(20).astype("float64")}
+ self.attrs = {'dim': [0]}
+ self.inputs = {'X': np.random.random((20, 10)).astype("float64")}
self.outputs = {'Out': self.inputs['X'].sum(axis=0)}
- def test_check_output(self):
- self.check_output()
- def test_check_grad(self):
- self.check_grad(['X'], 'Out')
+class Test2DReduce1(Test1DReduce):
+ def setUp(self):
+ self.op_type = "reduce_sum"
+ self.attrs = {'dim': [1]}
+ self.inputs = {'X': np.random.random((20, 10)).astype("float64")}
+ self.outputs = {
+ 'Out': self.inputs['X'].sum(axis=tuple(self.attrs['dim']))
+ }
-class TestReduceAll(OpTest):
+class Test3DReduce0(Test1DReduce):
+ def setUp(self):
+ self.op_type = "reduce_sum"
+ self.attrs = {'dim': [1]}
+ self.inputs = {'X': np.random.random((5, 6, 7)).astype("float64")}
+ self.outputs = {
+ 'Out': self.inputs['X'].sum(axis=tuple(self.attrs['dim']))
+ }
+
+
+class Test3DReduce1(Test1DReduce):
+ def setUp(self):
+ self.op_type = "reduce_sum"
+ self.attrs = {'dim': [2]}
+ self.inputs = {'X': np.random.random((5, 6, 7)).astype("float64")}
+ self.outputs = {
+ 'Out': self.inputs['X'].sum(axis=tuple(self.attrs['dim']))
+ }
+
+
+class Test3DReduce2(Test1DReduce):
+ def setUp(self):
+ self.op_type = "reduce_sum"
+ self.attrs = {'dim': [-2]}
+ self.inputs = {'X': np.random.random((5, 6, 7)).astype("float64")}
+ self.outputs = {
+ 'Out': self.inputs['X'].sum(axis=tuple(self.attrs['dim']))
+ }
+
+
+class Test3DReduce3(Test1DReduce):
+ def setUp(self):
+ self.op_type = "reduce_sum"
+ self.attrs = {'dim': [1, 2]}
+ self.inputs = {'X': np.random.random((5, 6, 7)).astype("float64")}
+ self.outputs = {
+ 'Out': self.inputs['X'].sum(axis=tuple(self.attrs['dim']))
+ }
+
+
+class TestKeepDimReduce(Test1DReduce):
+ def setUp(self):
+ self.op_type = "reduce_sum"
+ self.inputs = {'X': np.random.random((5, 6, 10)).astype("float64")}
+ self.attrs = {'dim': [1], 'keep_dim': True}
+ self.outputs = {
+ 'Out': self.inputs['X'].sum(axis=tuple(self.attrs['dim']),
+ keepdims=self.attrs['keep_dim'])
+ }
+
+
+class TestReduceAll(Test1DReduce):
def setUp(self):
self.op_type = "reduce_sum"
self.inputs = {'X': np.random.random((5, 6, 2, 10)).astype("float64")}
self.attrs = {'reduce_all': True}
self.outputs = {'Out': self.inputs['X'].sum()}
- def test_check_output(self):
- self.check_output()
-
- def test_check_grad(self):
- self.check_grad(['X'], 'Out')
-
## reduction in multi dims
class TestReduceMeanOpMultiAxises(OpTest):
diff --git a/python/paddle/fluid/transpiler/memory_optimization_transpiler.py b/python/paddle/fluid/transpiler/memory_optimization_transpiler.py
index dd90d66110e6233806b04bb726636a915f2ad84a..353c82f71632c0fa398bcfcf836cc382e7e501f7 100644
--- a/python/paddle/fluid/transpiler/memory_optimization_transpiler.py
+++ b/python/paddle/fluid/transpiler/memory_optimization_transpiler.py
@@ -324,6 +324,8 @@ def _process_sub_block_pair(pdesc, sub_block_pair):
sub_op_output = set()
sub_op_output.update(sub_op_dict[fwd_id].output_arg_names())
sub_op_output.update(sub_op_dict[grad_id].output_arg_names())
+ sub_op_output.update(sub_op_dict[fwd_id].input_arg_names())
+ sub_op_output.update(sub_op_dict[grad_id].input_arg_names())
ops_list.append((sub_block_ops, block_op_size, sub_op_output))
# Process rest fwd_op block ops
@@ -335,6 +337,7 @@ def _process_sub_block_pair(pdesc, sub_block_pair):
sub_block_ops.append(sub_block.op(i))
sub_op_output = set()
sub_op_output.update(sub_op_dict[fwd_id].output_arg_names())
+ sub_op_output.update(sub_op_dict[fwd_id].input_arg_names())
ops_list.append((sub_block_ops, sub_block_op_size, sub_op_output))
return ops_list
@@ -349,13 +352,17 @@ def _get_cfgs(input_program):
pdesc = input_program.get_desc()
block_desc = pdesc.block(0)
op_size = block_desc.op_size()
- # Get global block ops
- ops_list.append(
- ([block_desc.op(i) for i in range(op_size)], op_size, set()))
# Only process one level of nested subblock.
ops_list.extend(_process_sub_block_pair(pdesc, SUB_BLOCK_PAIR))
+ skip_opt_set = set()
+ for _, _, skip_opt in ops_list:
+ skip_opt_set.update(skip_opt)
+
+ # Get global block ops
+ ops_list.insert(
+ 0, ([block_desc.op(i) for i in range(op_size)], op_size, skip_opt_set))
cfgs = [
ControlFlowGraph(input_program, ops, forward_num, skip_opt)
for ops, forward_num, skip_opt in ops_list