Merge branch 'develop' into cmake
Showing
13.3 KB
22.4 KB
11.4 KB
18.0 KB
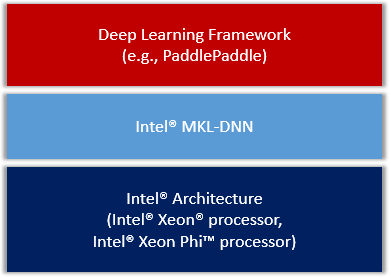
| W: | H:
| W: | H:
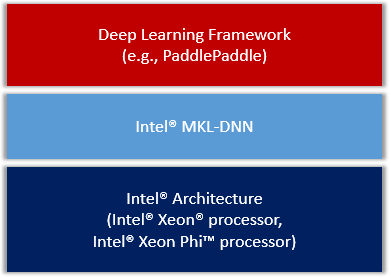
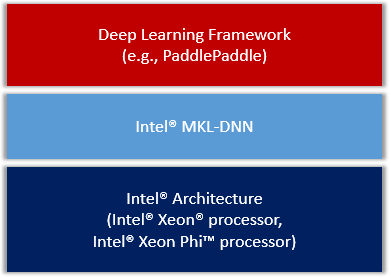
paddle/operators/nce_op.cc
0 → 100644
paddle/operators/nce_op.h
0 → 100644
13.3 KB
22.4 KB
11.4 KB
18.0 KB
9.7 KB | W: | H:
10.5 KB | W: | H: